Best Ai Platform for Academic Research
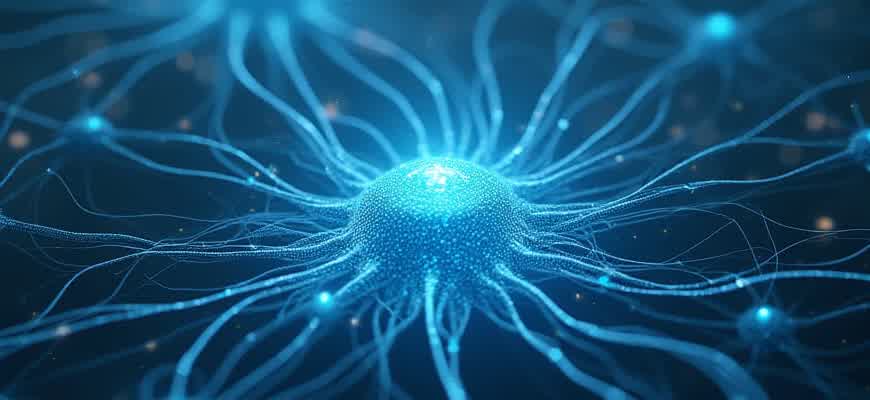
With the rise of artificial intelligence in research, selecting the right platform is crucial for enhancing productivity and accelerating scientific discoveries. These platforms offer a range of tools tailored to assist in data analysis, literature review, and collaboration. Below, we explore some of the leading AI platforms that are making a significant impact on academic research.
Key Features to Consider:
- Data processing capabilities
- Integration with research tools
- Collaboration features
- Accuracy and reliability of AI models
"Choosing the right AI platform can streamline workflows, improve data insights, and ultimately foster more efficient research collaboration."
Comparison of Leading AI Platforms:
Platform | Key Features | Best For |
---|---|---|
Platform A | Natural Language Processing, Predictive Analytics | Data analysis and pattern recognition |
Platform B | Literature Review Automation, Collaborative Tools | Reviewing large datasets and collaborative research |
Platform C | AI-Driven Experimentation, Visual Analytics | Experimentation and data visualization |
Top AI Platforms for Research in Academia
In the rapidly advancing field of academic research, AI platforms play an increasingly vital role in enhancing the efficiency and scope of scholarly work. These platforms provide researchers with a variety of tools that can aid in data analysis, content generation, literature review, and experimentations, thereby reducing the time and effort spent on routine tasks. Selecting the best AI platform depends on specific needs, whether it be for managing large datasets, producing high-quality papers, or aiding in complex simulations.
Several AI platforms have emerged as leaders in academic research, offering a combination of robust features, user-friendly interfaces, and specific applications tailored to the needs of scholars. Below is a breakdown of the most notable AI tools available to researchers today.
Key AI Platforms for Research
- Google Scholar AI - Focused on enhancing literature search capabilities, Google Scholar AI uses advanced algorithms to refine search results and recommend relevant papers.
- IBM Watson for Research - Known for its powerful data analysis tools, Watson aids researchers in extracting insights from large datasets, performing complex analytics, and generating predictive models.
- DeepAI - A comprehensive AI platform offering solutions for generating academic papers, analyzing trends, and visualizing data through deep learning models.
Features to Consider When Choosing an AI Research Platform
- Data Processing Capabilities: The platform should support large-scale data analysis, allowing for the processing of structured and unstructured datasets.
- Customizable Tools: Choose a platform that offers tools tailored to specific research disciplines, such as bioinformatics, physics, or social sciences.
- Collaboration Features: AI tools that facilitate seamless collaboration among teams of researchers can enhance productivity and result in more robust findings.
"AI tools in academic research are not just about automation but also about expanding the intellectual reach and capabilities of researchers." - Expert Review
Comparison Table
Platform | Main Features | Best For |
---|---|---|
Google Scholar AI | Advanced search algorithms, citation recommendations, literature discovery | Literature reviews and academic research discovery |
IBM Watson for Research | Data analysis, machine learning models, predictive analytics | Complex data analytics and simulations |
DeepAI | Paper generation, trend analysis, deep learning tools | Content generation and trend visualization |
How AI Platforms Simplify Data Collection for Research
Collecting data is a cornerstone of any research project, but the traditional process can be time-consuming and prone to human error. Artificial intelligence platforms offer solutions to automate and streamline the entire data collection process, making it more efficient and accurate. These platforms leverage machine learning algorithms to sift through vast amounts of data, extract relevant information, and organize it into useful formats for researchers.
One key advantage is the ability to gather data from a wide variety of sources, including academic databases, online repositories, and social media platforms. AI systems can quickly scan, analyze, and aggregate data, allowing researchers to focus on more complex aspects of their work rather than manual data entry and cleaning.
Benefits of AI in Data Collection
- Speed and Efficiency: AI tools can gather and organize data in a fraction of the time it would take a human researcher.
- Accuracy: Machine learning models are capable of reducing errors in data collection by automating repetitive tasks.
- Data Variety: AI can handle data from multiple sources, such as text, images, and even structured data like spreadsheets.
"AI platforms are revolutionizing the research field by making data collection faster, more accurate, and less dependent on manual input."
Key Features of AI Platforms for Data Collection
Feature | Description |
---|---|
Data Integration | AI systems can combine datasets from various platforms, creating a unified data source for analysis. |
Real-Time Data Collection | AI tools can monitor and collect data in real time, ensuring that research stays up-to-date. |
Data Validation | AI can verify the quality and consistency of data, reducing the need for post-collection cleaning. |
Steps Involved in AI-Powered Data Collection
- Data Scraping: AI tools automatically extract data from various sources, such as academic papers or websites.
- Data Filtering: The system filters out irrelevant or redundant information based on predefined criteria.
- Data Structuring: AI organizes the extracted data into a structured format, such as tables or datasets, making it easier to analyze.
Automating Literature Review with AI Tools
One of the most time-consuming tasks in academic research is conducting a comprehensive literature review. Traditionally, this process requires researchers to manually search for, read, and analyze numerous studies across various databases. However, with the advancement of AI technologies, this process is becoming increasingly automated, enabling researchers to save valuable time and focus more on analysis and synthesis rather than data collection.
AI-based platforms can efficiently automate literature reviews by processing large volumes of academic articles, extracting key insights, and providing summaries. This allows researchers to quickly identify relevant studies, track trends, and even identify gaps in the existing research.
Key Benefits of AI in Literature Review
- Time Efficiency: AI tools significantly reduce the time spent on manual searching, reading, and summarizing academic papers.
- Accuracy: AI algorithms can extract relevant data with greater precision, minimizing human error and subjectivity in the review process.
- Comprehensive Search: AI can scan a wider range of sources, including papers from less commonly cited journals, thus improving the thoroughness of the review.
- Trend Identification: AI tools can analyze vast amounts of research to identify emerging trends, research gaps, and areas of under-explored potential.
AI Tools for Automating Literature Review
- EndNote: A reference management software that uses AI to help researchers organize and categorize sources for easy retrieval and analysis.
- ResearchGate AI: A platform that uses machine learning algorithms to recommend articles and identify key papers related to specific research topics.
- Rayyan: A tool for systematic reviews that uses AI to assist in the screening and tagging of relevant articles from large datasets.
AI platforms can help academic researchers overcome the challenge of information overload by automating literature searches, providing context-aware summaries, and ensuring that no important studies are overlooked.
AI Tools Comparison Table
Tool | Primary Feature | Best Use Case |
---|---|---|
EndNote | Reference management and citation organization | Researchers focusing on efficient citation and source management |
ResearchGate AI | Article recommendations based on machine learning | Researchers looking for related articles in their field |
Rayyan | Automated screening and tagging of articles | Systematic reviewers performing literature selection |
Using AI for Data Analysis and Interpretation in Research
In modern academic research, AI-driven tools have become integral to efficiently process vast amounts of data. These platforms leverage machine learning and deep learning algorithms to perform complex analyses that would otherwise require extensive manual labor and computational time. By automating the process of data collection, cleaning, and analysis, AI allows researchers to focus on interpreting results and formulating hypotheses rather than getting bogged down in tedious preprocessing tasks.
The primary benefit of using AI for data interpretation is its ability to detect patterns and relationships within large datasets that are often invisible to the human eye. AI models can be trained to identify correlations, trends, and anomalies that may go unnoticed in traditional statistical analyses. This results in more accurate predictions and deeper insights, enhancing the overall quality of research.
Key AI Applications in Data Analysis
- Data Preprocessing: AI tools automate the cleaning, normalization, and transformation of raw data, ensuring it is ready for further analysis.
- Pattern Recognition: Machine learning algorithms are capable of identifying hidden patterns in large datasets, which is crucial for fields like genomics or social sciences.
- Predictive Analytics: AI helps researchers predict future outcomes by analyzing historical data and identifying trends.
AI Methods for Data Interpretation
- Supervised Learning: Uses labeled datasets to train algorithms for making predictions based on new, unseen data.
- Unsupervised Learning: Discovers hidden structures in unlabeled data, often used for clustering and anomaly detection.
- Natural Language Processing (NLP): Used for analyzing textual data, extracting meaning from research papers, or summarizing large volumes of information.
"AI empowers researchers to analyze data at a speed and scale that was previously unimaginable, unlocking new frontiers in scientific discovery."
Comparison of AI Techniques in Research
Method | Strengths | Weaknesses |
---|---|---|
Supervised Learning | Highly accurate predictions when trained with sufficient labeled data. | Requires large amounts of labeled data, which can be costly and time-consuming to collect. |
Unsupervised Learning | Effective in identifying hidden patterns without needing labeled data. | May produce results that are harder to interpret or validate without expert knowledge. |
NLP | Helps in extracting insights from unstructured textual data. | Can struggle with context, nuances, and ambiguous language in research texts. |
Enhancing Collaboration with AI-Driven Research Platforms
AI-powered research platforms have revolutionized the way academic teams collaborate on complex research projects. By leveraging machine learning algorithms and data analytics, these platforms offer a centralized space where researchers can seamlessly share insights, data, and findings. The integration of AI tools can help streamline communication and decision-making, reducing the time spent on repetitive tasks and ensuring a more efficient workflow.
Moreover, AI-enabled platforms can provide tailored recommendations, suggest relevant articles, and even predict potential breakthroughs. These features enhance not only the research process but also foster collaboration by aligning team members with the right resources and tools. As a result, researchers can focus more on innovation and discovery, knowing that the administrative and analytical tasks are being handled by the AI system.
Key Features of AI-Driven Collaboration
- Real-time Data Sharing: AI platforms allow for the immediate exchange of research data, facilitating quicker collaboration across different locations.
- Automated Literature Review: Machine learning algorithms scan vast databases and provide summarized findings, reducing the time spent on literature searches.
- Research Trend Prediction: AI can analyze existing data and predict future trends, offering valuable insights to guide research direction.
AI-Enhanced Tools for Research Teams
- Collaborative Annotation: AI platforms enable real-time annotation of documents, allowing multiple researchers to add insights and comments simultaneously.
- Data Integration: AI tools combine datasets from various sources, enabling comprehensive analyses and fostering cross-discipline collaborations.
- Language Processing: Advanced AI algorithms support translation and semantic analysis, breaking down language barriers and enhancing global collaboration.
Benefits for Academic Collaboration
Benefit | Description |
---|---|
Increased Efficiency | AI platforms automate mundane tasks like data entry and literature searches, freeing up time for more impactful work. |
Cross-disciplinary Collaboration | AI tools facilitate the merging of insights across different fields, promoting a more holistic approach to research. |
Global Reach | AI systems enhance remote collaboration by providing easy access to resources, no matter the geographical location. |
"AI-driven collaboration tools are changing the academic landscape, allowing researchers to connect, innovate, and achieve more than ever before."
How AI Helps in Reducing Research Time and Costs
Artificial intelligence has revolutionized the landscape of academic research by offering tools that streamline various stages of the research process. By automating repetitive tasks, AI enables researchers to focus on more critical aspects of their work, ultimately reducing the overall time spent on research projects. This efficiency also leads to a significant decrease in associated costs, making research more accessible and scalable across different fields.
One of the primary ways AI accelerates research is by enhancing data collection and analysis. Traditionally, researchers spend a considerable amount of time gathering and sorting data. AI-powered platforms can rapidly process vast amounts of information, identify patterns, and even suggest hypotheses, all of which would typically require manual labor and months of work. These advances in data handling translate into faster project timelines and lower costs for research institutions.
Key Areas Where AI Reduces Research Time and Expenses
- Data Processing: AI tools can analyze and synthesize large datasets at speeds far exceeding human capabilities, enabling quicker insights and eliminating the need for extensive manual analysis.
- Literature Review Automation: AI platforms can scan academic journals, books, and other sources to extract relevant information, significantly reducing the time needed for literature review.
- Data Visualization: AI-driven software generates high-quality visual representations of complex data, saving researchers time in manually creating graphs and charts.
Cost Reduction Through AI Integration
- Labor Savings: By automating time-consuming tasks such as data cleaning and analysis, AI reduces the need for extensive human labor, lowering operational costs.
- Enhanced Collaboration: AI platforms often provide tools that allow for seamless collaboration between researchers, cutting down on the cost of communication and project coordination.
- Reduced Trial and Error: AI can help predict outcomes and suggest improvements early in the research process, reducing the likelihood of costly mistakes during experimentation.
"AI allows researchers to complete their work in a fraction of the time, which ultimately reduces research expenditures and accelerates the pace of discovery."
AI in Academic Research: A Comparative Analysis
Traditional Method | AI-Enhanced Method |
---|---|
Manual data collection and analysis | Automated data processing and pattern recognition |
Long hours spent on literature reviews | AI-driven automated literature search and review |
High labor costs for data analysis and visualization | AI tools for data visualization and analysis, reducing manual labor |
Integrating Artificial Intelligence with Research Management Tools
The integration of AI technologies with research management systems is revolutionizing how academic projects are conducted. By merging AI's advanced capabilities in data processing with the efficiency of research management software, researchers can streamline various aspects of their work. This includes automating repetitive tasks, improving data analysis, and facilitating collaboration. AI enables enhanced decision-making by providing insights based on large datasets, significantly reducing human error and saving time.
As research projects grow more complex, AI-driven tools help in organizing data, tracking progress, and optimizing workflows. When implemented into research management platforms, AI offers customizable solutions that can handle everything from literature review automation to grant application assessments. This not only boosts the productivity of researchers but also enhances the overall quality of the research output.
Key Benefits of AI Integration
- Automated Data Analysis: AI can process and analyze large datasets more efficiently than humans, providing faster insights.
- Streamlined Workflow: By automating administrative tasks, researchers can focus on high-value activities such as experimentation and writing.
- Enhanced Collaboration: AI tools can facilitate real-time communication between teams, improving project coordination.
AI-Powered Features in Research Management Software
- Smart Literature Review: AI-powered algorithms can quickly scan and categorize research papers, identifying key findings and trends.
- Project Management Tools: AI aids in assigning tasks, tracking deadlines, and managing resources efficiently.
- Grant and Funding Analysis: AI assists in finding relevant funding opportunities by matching projects with suitable grants based on keywords and themes.
"AI integration into research management tools allows for smarter, data-driven decision-making that accelerates research processes."
Example: AI in Research Data Organization
Feature | AI Impact |
---|---|
Data Classification | AI can categorize research data based on context, making it easier to retrieve and analyze later. |
Data Insights | AI analyzes trends and patterns within datasets to predict future research outcomes. |
Collaboration Enhancement | AI tools recommend optimal team structures and task delegation based on project needs. |
Leveraging AI to Enhance Research Precision and Credibility
AI tools are increasingly shaping the landscape of academic research by enabling more precise data analysis and more reliable results. Researchers can now automate time-consuming tasks such as data collection, sorting, and preliminary analysis, allowing them to focus on complex hypothesis testing and interpretation. By reducing human error, AI helps to refine the research process and increase overall accuracy.
Furthermore, AI-powered platforms offer advanced capabilities for identifying patterns in large datasets that may not be immediately obvious to human researchers. These tools enhance the quality of findings by ensuring more comprehensive and robust analysis, which contributes to more reliable academic outcomes.
Key Benefits of AI in Research Precision
- Data Cleaning: AI can automatically detect and correct errors in raw data, ensuring that researchers work with high-quality information.
- Statistical Analysis: Sophisticated AI algorithms can perform complex statistical tests more efficiently than traditional methods, increasing the reliability of research conclusions.
- Pattern Recognition: Machine learning models can identify hidden patterns in vast datasets that researchers may overlook, leading to more insightful findings.
AI Tools Supporting Research Reliability
- Natural Language Processing (NLP): NLP technologies can help researchers analyze large volumes of literature and extract relevant information, reducing bias and improving data synthesis.
- Automated Peer Review: AI-based systems can assist in the peer review process by checking for inconsistencies or errors in research submissions.
- Reproducibility Checks: AI platforms can verify that research methods and results can be replicated, ensuring greater transparency and credibility.
AI-powered systems can reduce the impact of human biases in research, ensuring that findings are driven by objective data and analysis.
Table: Comparison of AI Tools in Research
AI Tool | Function | Impact on Research |
---|---|---|
DataCleaner | Automates data cleaning and error correction | Improves data quality and accuracy |
ML Model Analyzer | Performs advanced statistical analysis and pattern recognition | Enhances predictive accuracy and insight generation |
PeerReviewBot | Assists in automated peer review and checks for inconsistencies | Boosts credibility by reducing human error and bias |