Ai for Design Optimization
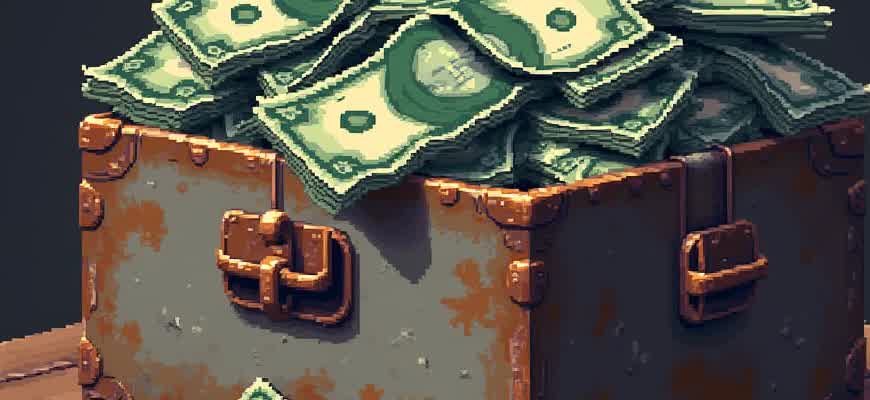
Artificial intelligence (AI) has revolutionized the field of design by introducing powerful tools that enhance the optimization process. Through advanced algorithms and machine learning techniques, AI allows designers to improve efficiency, reduce errors, and create more innovative solutions. The integration of AI in design tools can significantly reduce the time required to iterate through design concepts and optimize them for various objectives, such as performance, cost, or user experience.
Key Areas Where AI Improves Design:
- Automating repetitive tasks and reducing manual effort
- Enhancing the decision-making process with predictive analytics
- Generating innovative design alternatives through algorithmic approaches
AI-driven design tools enable a deeper analysis of design constraints, leading to more informed and data-driven decisions.
Common Techniques Used for Optimization:
- Generative design algorithms that explore a wide range of possibilities
- Topology optimization to ensure structural efficiency
- Parametric design tools that adapt to changes in real-time
Benefits of AI in Design Optimization:
Benefit | Impact |
---|---|
Time Efficiency | Reduces design cycle time by automating repetitive tasks |
Improved Accuracy | Minimizes human error and enhances precision in design calculations |
Innovation | Generates creative and unconventional design alternatives |
AI for Design Optimization
Artificial Intelligence (AI) is transforming the design process by offering innovative solutions for optimization. In industries ranging from architecture to product design, AI algorithms are being integrated to create more efficient, cost-effective, and functional designs. By analyzing vast amounts of data, AI can suggest design modifications that may not be apparent through traditional methods, speeding up decision-making and reducing human error.
One of the core advantages of using AI in design optimization is its ability to simulate various scenarios quickly, identifying the most effective solutions. Whether it's improving structural integrity, enhancing energy efficiency, or fine-tuning user experience, AI tools can adapt to evolving requirements, offering real-time adjustments based on live feedback.
How AI Enhances the Design Process
- Data-Driven Insights: AI systems analyze large data sets to provide insights on how designs can be improved. This leads to informed decisions, reducing guesswork and trial-and-error.
- Automation of Repetitive Tasks: AI can handle time-consuming tasks such as generating multiple iterations of designs, allowing designers to focus on creative aspects.
- Cost and Time Efficiency: Through predictive analysis, AI identifies the most cost-effective materials and designs, helping save both time and money during the production phase.
Examples of AI Applications in Design
- Architecture: AI-driven tools optimize building designs for energy consumption, structural stability, and material efficiency.
- Product Design: AI aids in simulating user interactions and manufacturing processes to create products that meet both user needs and production constraints.
- Fashion: AI helps designers predict trends and create customized patterns based on consumer data and preferences.
"AI is not only enhancing design capabilities but also pushing the boundaries of what’s possible in every field, from architecture to consumer goods."
AI vs. Traditional Design Methods
Factor | AI-Driven Design | Traditional Design |
---|---|---|
Speed | Quick simulations and iterative changes | Time-consuming with limited iterations |
Precision | Highly accurate predictions based on data | Relies on designer experience and intuition |
Cost | Reduces material waste and errors | Potential for higher costs due to mistakes and inefficiencies |
Accelerating Product Development with AI Integration
Artificial intelligence (AI) plays a transformative role in speeding up product development processes by automating complex tasks and enabling rapid iterations. By using AI to analyze vast amounts of data, companies can uncover insights that inform better decision-making at each phase of the design cycle. This leads to a more efficient workflow and faster time-to-market for innovative products. AI also helps in minimizing manual errors and reducing dependency on human expertise in repetitive tasks, allowing teams to focus on higher-value activities.
Incorporating AI tools into product development is a key driver for enhancing design optimization. AI algorithms can simulate various design scenarios in real-time, which accelerates the process of evaluating multiple prototypes. This enables teams to test and refine designs quickly without the need for expensive physical prototypes. Additionally, AI can predict product performance, identify potential issues, and suggest improvements, resulting in a more streamlined development cycle.
Key Benefits of AI in Product Development
- Reduced Time to Market: AI-driven automation and optimization significantly shorten development timelines.
- Enhanced Design Quality: AI tools can identify design flaws early, leading to higher-quality products.
- Improved Collaboration: AI facilitates seamless integration of various teams working on different aspects of product development.
AI-Powered Tools for Design Optimization
- Generative Design: AI-driven software can generate thousands of design alternatives based on predefined parameters, accelerating the concept phase.
- Predictive Analytics: AI models predict potential issues and help in making data-backed decisions during the design process.
- Machine Learning for Testing: AI can simulate real-world conditions to evaluate how designs perform under various scenarios.
"AI is not just a tool for improving existing processes; it is a catalyst for creating entirely new ways of designing and developing products."
AI's Impact on Design Iterations
The ability to run simulations and tests at scale allows design teams to perform multiple iterations in a fraction of the time traditionally required. With AI, these iterations can be optimized continuously as new data and feedback are integrated, ensuring that each version is closer to the final product without unnecessary delays.
AI Tool | Purpose | Impact on Development |
---|---|---|
Generative Design | Create multiple design alternatives based on defined parameters | Reduces time spent on brainstorming, leading to faster concept validation |
Predictive Analytics | Anticipates potential issues during development | Helps avoid costly mistakes and delays by addressing problems before they occur |
Machine Learning for Testing | Simulate product performance under varied conditions | Improves the accuracy and reliability of designs before physical testing |
How AI Algorithms Enhance Design Accuracy and Precision
AI-driven algorithms have revolutionized the way design accuracy and precision are achieved, especially in industries that require exacting standards. By utilizing machine learning and advanced data processing techniques, AI systems can identify patterns, predict outcomes, and optimize designs far beyond the capabilities of traditional methods. This allows for significant improvements in product performance, material usage, and overall design efficiency.
One of the primary benefits of AI in design optimization is its ability to analyze vast amounts of data and quickly identify the most effective design solutions. This level of analysis ensures that designs are not only more accurate but also tailored to meet specific performance criteria. AI tools can simulate real-world conditions and adjust designs accordingly, minimizing the need for costly physical prototypes and reducing the risk of human error.
Key Advantages of AI in Design Precision
- Predictive Modeling: AI algorithms can simulate a wide range of variables, predicting how changes in one part of the design will affect the whole system.
- Automated Adjustments: Machine learning systems can automatically modify designs based on real-time feedback and performance data.
- Data-Driven Insights: AI processes large datasets to uncover hidden patterns that help in refining design parameters for better accuracy.
Applications in Design Optimization
- Architecture: AI tools can optimize structural elements, minimizing material waste while ensuring safety and durability.
- Automotive Industry: By predicting how vehicles will perform under various conditions, AI helps create more precise designs, enhancing safety features and fuel efficiency.
- Electronics: AI algorithms optimize circuit board designs, improving efficiency while reducing component failure rates.
"AI algorithms not only enhance the accuracy of designs but also enable a higher degree of customization and adaptability in real-time."
Comparison of Traditional Design vs AI-Optimized Design
Feature | Traditional Design | AI-Optimized Design |
---|---|---|
Design Time | Longer due to manual testing and iterations | Faster with automated simulations and adjustments |
Accuracy | Prone to human error and approximation | High precision through data-driven insights |
Material Usage | Often inefficient, leading to waste | Optimized for minimal waste and cost |
Automating Design Iterations with Machine Learning Tools
Machine learning (ML) is revolutionizing the way design processes are approached, enabling faster, more efficient iterations and improvements. By integrating machine learning algorithms into the design workflow, engineers and designers can create better solutions without the need for manual adjustments at every stage. These tools can analyze vast amounts of data from previous iterations, learn from patterns, and predict the most effective design changes, saving time and reducing errors.
The automation of design iterations through machine learning significantly enhances productivity and accuracy. ML tools allow designers to focus more on creative aspects by offloading the repetitive, time-consuming tasks. These systems work by continuously improving upon initial designs, using previous outputs to guide future changes. This process ensures that designs evolve quickly while meeting predefined performance criteria.
Key Benefits of ML-Driven Design Iteration
- Efficiency: Automates repetitive tasks, reducing manual effort and speeding up the design process.
- Precision: Utilizes data-driven insights to optimize designs with greater accuracy than humanly possible.
- Scalability: Can handle large-scale projects with numerous design variables, making it suitable for complex tasks.
- Cost-Effective: Reduces the need for costly prototypes and testing by simulating results virtually.
Example Workflow for Machine Learning in Design
- Data Collection: Gather historical data from previous designs or simulation results.
- Training: Feed the collected data into an ML algorithm to identify patterns and correlations.
- Iteration: Automatically adjust design parameters based on the algorithm's predictions.
- Evaluation: Assess the updated design using predefined performance metrics.
- Finalization: Integrate the optimized design into the final product prototype.
"By leveraging machine learning, we eliminate the need for trial-and-error design approaches, drastically improving the overall workflow efficiency."
Machine Learning Tools in Design
Tool | Functionality | Use Case |
---|---|---|
TensorFlow | Deep learning framework for model training and design optimization | Used in creating predictive models for performance optimization in product design |
AutoML | Automates the model selection, training, and optimization process | Speeds up the design iteration cycle by automating the process of generating models |
Genetic Algorithms | Optimization algorithm inspired by natural selection | Used to evolve design alternatives through iterative testing and selection |
Using Artificial Intelligence to Detect and Remove Design Issues Early
Artificial intelligence has the potential to significantly improve design processes by identifying potential flaws at the early stages. By analyzing vast amounts of design data, AI tools can pinpoint weaknesses or inefficiencies that might not be evident to human designers, enabling proactive corrections. This approach not only reduces costly revisions later in the process but also enhances the overall quality of the final product.
By leveraging machine learning algorithms and predictive analytics, AI can optimize various aspects of design, from structural integrity to user experience. Through continuous learning, AI systems can identify patterns and correlations that humans may miss, leading to faster and more precise problem detection. The goal is to integrate AI into the design workflow, allowing designers to focus on creativity and innovation while minimizing the risk of errors.
Key Benefits of AI in Early Design Flaw Detection
- Faster Detection: AI can instantly analyze designs against pre-established criteria to highlight potential issues.
- Cost Reduction: Early identification of flaws reduces the need for expensive modifications in later stages of production.
- Improved Accuracy: AI's data-driven approach ensures that no detail is overlooked, preventing human error.
- Predictive Insights: Machine learning models predict how design flaws might evolve in real-world scenarios.
Process of Using AI to Detect Design Flaws
- Data Collection: Gather extensive data from previous designs, including user feedback, performance metrics, and failure cases.
- Model Training: Train AI algorithms using this data to recognize patterns associated with common design flaws.
- Automated Evaluation: Run the AI system on new designs to evaluate them against learned models, identifying potential flaws.
- Real-Time Feedback: Provide immediate feedback to designers, allowing them to make adjustments before moving forward.
"AI’s ability to learn from past designs and adapt in real-time helps ensure that issues are caught early, leading to faster development cycles and higher-quality outputs."
Examples of AI-Driven Tools for Flaw Detection
Tool | Functionality | Impact on Design |
---|---|---|
Generative Design Software | Generates multiple design alternatives based on specific parameters, optimizing for weight, strength, and material usage. | Helps eliminate inefficiencies and suboptimal designs early. |
Simulation-Based AI | Simulates real-world stress tests and performance to predict failures. | Reduces prototyping costs by predicting weak points in the design. |
AI-Powered CAD Tools | Analyzes design geometry for potential issues, including structural weaknesses or ergonomic inefficiencies. | Improves overall design quality by detecting flaws that may not be visible to the designer. |
Integrating AI with CAD Systems for Enhanced Design Process
In recent years, the integration of artificial intelligence (AI) with computer-aided design (CAD) software has revolutionized how designers approach complex projects. AI offers new capabilities to streamline workflows, improve precision, and optimize designs in ways that traditional CAD tools alone cannot achieve. Through AI-driven tools, designers can automatically generate design alternatives, detect potential issues early, and simulate real-world conditions, enhancing both efficiency and quality.
The use of AI in CAD systems not only speeds up the design process but also introduces advanced features like generative design, predictive analysis, and smart automation. By automating repetitive tasks and providing real-time suggestions, AI helps designers focus on higher-level decision-making while ensuring more robust and accurate outputs. The combined power of AI and CAD results in smarter, more innovative products with reduced time-to-market.
Key Benefits of AI in CAD Integration
- Generative Design: AI can create multiple design options based on specified constraints, such as material, cost, and performance, allowing designers to explore alternatives quickly.
- Predictive Analysis: AI algorithms can predict the performance of a design under various conditions, helping to identify potential flaws before physical testing.
- Automation of Routine Tasks: AI can automate repetitive and time-consuming tasks, such as part optimization, documentation generation, and error detection, freeing up designers to focus on more creative aspects.
Example Applications in Design
- Structural Design Optimization: AI-powered CAD tools can optimize the geometry and material selection for structures, ensuring maximum strength while minimizing weight and cost.
- 3D Model Generation: AI can assist in the generation of 3D models from basic sketches or even 2D drawings, reducing the manual effort required to create accurate representations.
- Customization for Mass Production: AI allows for the automatic adjustment of designs based on consumer preferences, enabling more efficient mass customization.
Challenges and Considerations
Challenge | Solution |
---|---|
Data Complexity | Advanced AI models require large datasets and high-quality data to be effective in design optimization. |
Integration with Existing Tools | Seamless integration of AI with existing CAD tools may require custom solutions and training for design teams. |
AI integration with CAD systems is not just about automating processes, but enabling new levels of creativity, precision, and efficiency in design.
How AI Optimizes Customization and Personalization in Design
Artificial intelligence has transformed the design industry by offering advanced tools that enable deeper customization and personalization. AI algorithms can analyze large datasets, including customer preferences and behavioral patterns, to create highly personalized design solutions. This allows for a more tailored approach to design, moving away from one-size-fits-all models to solutions that cater to individual needs and tastes. The application of AI enhances the user experience by generating designs that are not only functional but also aesthetically aligned with the client’s identity and expectations.
Through machine learning, AI can predict trends and automate the adaptation of designs based on specific parameters, such as user interactions and historical data. This dynamic customization goes beyond static templates, providing real-time adjustments to ensure that the design remains relevant and user-focused. The resulting products can better satisfy consumer demands, boosting both engagement and satisfaction.
Key Approaches to Personalization in Design
- Data-Driven Customization: AI utilizes user data to generate unique design options that reflect individual preferences and needs.
- Real-Time Adaptation: AI systems can modify designs on the fly, responding to changes in user behavior or environmental factors.
- Automated Design Variations: AI generates multiple design iterations, allowing users to select or tweak designs based on their personal tastes.
Examples of AI-Driven Customization
- Fashion design platforms using AI to recommend personalized clothing styles based on past purchases and browsing behavior.
- Home decor tools that offer personalized interior design suggestions based on room dimensions and user preferences.
- Web design tools that automatically adjust color schemes and layouts based on user demographics and site performance data.
AI Personalization: Key Benefits
Benefit | Description |
---|---|
Enhanced User Engagement | Personalized designs improve user satisfaction, encouraging deeper interaction with products or services. |
Efficiency in Design Creation | AI automates much of the design process, significantly reducing time and effort spent on customization tasks. |
Scalable Personalization | AI enables companies to offer personalized design experiences at scale, without compromising on quality. |
AI-driven personalization helps create designs that are not just functional, but emotionally resonant, offering users a truly unique experience that reflects their individual needs and preferences.
Optimizing Resource Allocation in Design Projects with AI
AI can significantly enhance resource distribution in design projects, ensuring that every aspect of the process is effectively managed. By analyzing data from previous projects, AI can predict which resources–whether human, financial, or material–are needed at specific stages. This enables design teams to allocate resources more efficiently, minimizing waste and reducing the overall project cost. AI tools that leverage predictive analytics can identify potential bottlenecks and suggest optimal strategies to ensure resources are used where they will have the most impact.
Moreover, AI can assist in dynamic decision-making. As project requirements evolve or unexpected issues arise, AI systems can adapt resource plans in real-time. This level of flexibility allows design managers to respond to changes promptly, avoiding delays and maintaining productivity throughout the project's lifecycle.
Key Benefits of AI in Resource Allocation
- Data-Driven Insights: AI analyzes historical project data to forecast future needs and trends.
- Cost Reduction: Through efficient allocation, AI minimizes the risk of overspending on unnecessary resources.
- Improved Time Management: AI optimizes scheduling, reducing downtime and enhancing project flow.
- Real-Time Adaptation: AI adjusts to unforeseen project changes, ensuring resources remain aligned with shifting goals.
Example of AI-Based Resource Allocation
Resource | Optimized Allocation | Result |
---|---|---|
Design Team | AI predicts peak workload times and distributes tasks accordingly. | Increased productivity and reduced burnout. |
Software Tools | AI assesses software tool usage and recommends the most efficient tools for specific tasks. | Faster design iterations with minimal tool switching. |
Budget | AI monitors expenses, ensuring spending aligns with project phases. | Reduced risk of budget overruns and better financial control. |
"AI empowers design teams to allocate resources in a way that maximizes efficiency, ensuring that every element of the project is supported appropriately."