Ai-powered Meaning
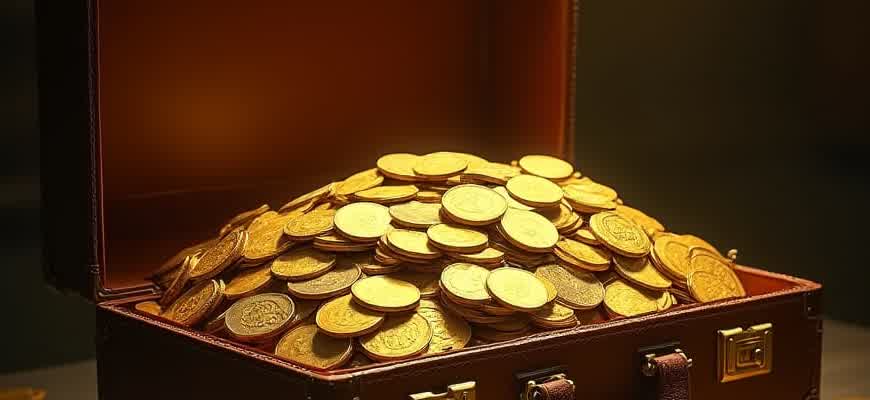
Artificial Intelligence (AI) is transforming the way we approach the idea of meaning in various contexts. It enables machines to interpret and generate value, making sense of data and processes that were traditionally managed by humans. The integration of AI into areas like natural language processing, decision-making, and automated reasoning has led to the emergence of AI-powered significance across multiple domains.
Key Areas Where AI Creates Meaning:
- Natural Language Understanding: AI systems can process vast amounts of text to identify context, sentiment, and deeper meaning.
- Data Interpretation: Machine learning models extract patterns and trends from complex data sets, generating actionable insights.
- Automated Decision-Making: AI makes sense of situations and environments, leading to decisions with minimal human input.
"AI has revolutionized how meaning is derived from complex datasets, shifting the landscape of human interaction with technology."
Types of AI Models Contributing to Meaning Creation:
Model Type | Application |
---|---|
Neural Networks | Used for pattern recognition and natural language processing. |
Decision Trees | Assist in making decisions based on past data and patterns. |
Generative Models | Generate new data based on learned patterns, such as text or images. |
AI-Driven Meaning: A Practical Guide
Understanding the concept of meaning in the context of artificial intelligence (AI) has become increasingly important as AI systems evolve. AI technologies, especially in the realms of natural language processing (NLP) and machine learning, are being used to derive and interpret meaning from data, text, and interactions. This guide will break down how AI systems interpret and generate meaningful content, offering practical insights into how you can leverage AI for various applications.
To effectively harness the power of AI in understanding meaning, it is essential to first grasp the fundamental techniques AI employs. These methods include data modeling, semantic analysis, and context recognition. By learning these processes, businesses and individuals can optimize their workflows, create more intuitive user experiences, and automate complex tasks related to data interpretation.
Key Concepts in AI-Powered Meaning
- Semantic Understanding: AI systems break down language into smaller, interpretable units, such as words and phrases, to extract meaning.
- Contextual Awareness: AI considers the broader context in which data or language appears, improving the accuracy of interpretation.
- Machine Learning Algorithms: These algorithms help AI learn from past data and improve its ability to derive meaning over time.
Steps to Apply AI for Meaning Extraction
- Data Collection: Gather large sets of data that will serve as the foundation for AI models.
- Preprocessing: Clean and structure the data to make it compatible with AI models.
- Model Training: Use machine learning to train models that can predict, classify, or generate meaningful insights.
- Deployment: Implement the trained models into real-world applications to derive meaning in real time.
"AI's ability to process vast amounts of data and recognize subtle patterns makes it an invaluable tool for understanding meaning and generating insights that would otherwise be impossible to detect."
Example of AI in Action: Sentiment Analysis
Sentiment | AI's Interpretation |
---|---|
Positive | AI recognizes keywords and tone to conclude that the message conveys a positive sentiment. |
Neutral | AI identifies a lack of strong emotional indicators, interpreting the message as neutral. |
Negative | AI detects negative words and context, interpreting the message as expressing dissatisfaction. |
How AI-Driven Tools Uncover Deep Insights from Text
AI-driven tools have significantly enhanced our ability to analyze large volumes of text data. These technologies use advanced algorithms to detect patterns, extract key concepts, and identify relationships between words and phrases. By processing text at scale, AI tools help organizations uncover insights that would be difficult or time-consuming for humans to find manually.
One of the primary advantages of AI in text analysis is its capacity to process and understand the underlying meaning within complex documents. AI algorithms are designed to go beyond surface-level interpretation, capturing nuances such as sentiment, intent, and context. This allows businesses to make more informed decisions based on a deeper understanding of textual data.
Techniques Used to Extract Insights from Text
- Natural Language Processing (NLP) – AI tools use NLP to analyze and understand human language. This includes part-of-speech tagging, named entity recognition, and syntactic parsing.
- Topic Modeling – This method groups similar words and phrases together to identify overarching themes in a body of text.
- Sentiment Analysis – AI identifies the emotional tone behind the text, such as whether a statement is positive, negative, or neutral.
- Text Summarization – AI can automatically generate concise summaries of large texts, extracting only the most important information.
Benefits of AI-Driven Text Analysis
- Scalability – AI can process vast amounts of text data quickly, making it ideal for large-scale analysis.
- Accuracy – AI-powered tools can uncover patterns that may be overlooked by human analysts.
- Real-time Insights – AI enables organizations to gain immediate feedback from text data, making it useful for customer service, social media monitoring, and more.
AI tools can analyze text data in ways that go beyond basic keyword matching, enabling businesses to make strategic decisions based on deeper insights into customer behavior, market trends, and competitive landscapes.
AI Techniques in Action: A Comparison
Technique | Application | Example |
---|---|---|
Sentiment Analysis | Assess customer feedback | Understanding whether reviews are positive or negative |
Topic Modeling | Content categorization | Grouping similar news articles or blog posts |
Text Summarization | Automated report generation | Summarizing research papers for quick insights |
Integrating AI into Your Existing Content Strategy
Incorporating artificial intelligence into your content strategy can significantly enhance its effectiveness. AI tools are capable of streamlining content creation, optimizing distribution, and improving user engagement through personalized experiences. The challenge lies in merging these advanced technologies with your current workflows, ensuring that the integration does not disrupt your existing content pipeline but instead complements it.
To successfully integrate AI, it's essential to start by identifying areas where AI can add the most value. Whether it’s through content generation, data analysis, or audience targeting, AI can assist in automating repetitive tasks, freeing up resources for more creative and strategic efforts. The key is balancing human creativity with AI capabilities to maximize impact.
Steps to Integrate AI into Your Content Strategy
- Define the Objectives: Clearly outline what you want to achieve with AI. Whether it’s improving content quality, increasing audience reach, or enhancing engagement, having specific goals will guide the integration process.
- Choose the Right Tools: Select AI tools that align with your goals. For example, natural language processing tools can assist in content creation, while AI analytics platforms help refine your targeting strategies.
- Train Your Team: Ensure your team understands the AI tools they’ll be working with. This might involve training on new software or updating current workflows to accommodate AI capabilities.
- Monitor and Optimize: Continuously track the performance of AI-driven initiatives. Gather data, measure results, and make necessary adjustments to improve content relevance and effectiveness.
"AI should enhance, not replace, human creativity. The goal is to create a synergistic environment where both work together for more impactful content."
Key AI Tools for Content Strategy
AI Tool | Use Case | Benefits |
---|---|---|
AI Content Generators | Automate content creation for blogs, social media posts, and product descriptions | Speeds up content production, maintains consistency, and reduces manual effort |
AI Analytics Platforms | Analyze audience behavior and content performance | Offers insights for better-targeted content, improving engagement and conversions |
AI Personalization Engines | Deliver personalized content recommendations based on user behavior | Enhances user experience, increasing time on site and customer loyalty |
Tips for a Smooth Integration
- Start with small, manageable projects to test AI's impact on your content strategy.
- Ensure seamless collaboration between your content team and data scientists to create a data-driven approach.
- Regularly review and update your AI tools to ensure they remain relevant as your strategy evolves.
Boosting Customer Engagement with AI-Based Contextual Understanding
AI-driven solutions offer a new way to create personalized customer experiences. By leveraging contextual awareness, AI can anticipate customer needs, preferences, and behaviors, providing more meaningful interactions. This enhances customer engagement and fosters long-term loyalty by delivering relevant content at the right time.
Contextual understanding allows AI to not only process customer data but also to interpret it in real-time. This leads to dynamic, responsive engagement strategies that adapt to individual customer journeys. The ability to recognize nuances in language, sentiment, and intent makes interactions more intuitive and valuable.
Key Benefits of AI-Based Contextual Engagement
- Personalized Experiences: AI adapts to each customer's unique behavior, providing tailored recommendations and solutions.
- Real-Time Interaction: By analyzing real-time data, AI can instantly respond to customer queries and requests.
- Increased Conversion Rates: Contextually aware engagement boosts customer satisfaction, leading to higher conversion and retention rates.
How It Works
- Data Collection: AI gathers data from various touchpoints such as web browsing, social media, and purchase history.
- Contextual Analysis: It interprets the data to understand customer intent, preferences, and current needs.
- Personalized Responses: AI then generates responses or actions that are highly relevant to the customer’s specific context.
"AI's ability to process and understand context in real time allows businesses to offer relevant and timely solutions, fostering a stronger connection with customers."
Example of Contextual AI in Action
Action | AI-Generated Response |
---|---|
Customer browsing a product page | AI suggests related items based on previous purchase history. |
Customer expresses frustration with a product | AI provides instant troubleshooting advice or an offer for a refund. |
Improving Content Relevance with AI-Powered Language Understanding
Artificial intelligence, particularly in the domain of Natural Language Processing (NLP), plays a critical role in enhancing the relevance of content. By analyzing vast amounts of textual data, AI can grasp not only the meaning but also the context and nuances behind the words. This enables automated systems to generate content that is more aligned with user intent, interests, and needs.
With advanced language models, content creation and delivery can be fine-tuned to provide a more personalized experience. Whether it's through improving SEO practices or generating more precise recommendations, AI-driven NLP helps ensure that the right message reaches the right audience. This transformation leads to higher engagement and improved user satisfaction.
Key Approaches for Enhancing Content Relevance
- Contextual Understanding: AI can analyze and understand the context of a conversation or search query to tailor content more effectively.
- Intent Recognition: By recognizing user intent, AI systems can provide content that aligns with the user’s specific goals or interests.
- Semantic Search: NLP enables search engines to prioritize meaning and context over simple keyword matching, resulting in more relevant search results.
Practical Applications
- Personalized Recommendations: AI systems can recommend articles, products, or services based on an individual’s previous interactions and preferences.
- Dynamic Content Creation: AI tools can assist in generating high-quality, contextually appropriate content, such as blog posts or social media updates.
- Content Optimization: NLP technologies help refine existing content by suggesting improvements for clarity, tone, and relevance.
Benefits of AI in Content Relevance
Benefit | Description |
---|---|
Enhanced Engagement | Users interact more with content that is relevant and aligned with their interests and needs. |
Increased Conversion Rates | Personalized content leads to higher conversion rates by appealing directly to the user’s motivations. |
Efficient Content Strategy | AI helps businesses identify trends and content gaps, enabling more targeted and effective content strategies. |
Important: AI-driven improvements in content relevance are not just about personalization, but also about adapting to evolving user preferences and emerging trends.
AI and Personalization: Creating Tailored Experiences at Scale
AI-driven systems are revolutionizing how businesses approach personalization. By leveraging machine learning and data analytics, AI can process vast amounts of consumer data to deliver individualized content and experiences. This goes beyond simple recommendations; it allows companies to understand preferences, predict behavior, and create dynamic interactions in real time. For example, e-commerce platforms are able to present unique product suggestions to each user based on their browsing history, demographic information, and even emotional responses to previous interactions.
The ability to scale this level of personalization is where AI truly stands out. With traditional methods, tailoring an experience to each user individually could be costly and time-consuming. However, AI enables businesses to offer personalized experiences on a large scale, ensuring that each interaction is relevant and engaging, without the need for extensive human intervention. This shift is particularly noticeable in sectors like retail, entertainment, and online services, where user engagement is key to retaining customers and driving revenue.
Key Techniques in AI-Powered Personalization
- Predictive Analytics: AI uses historical data to predict future behaviors and preferences.
- Natural Language Processing: NLP allows AI to understand and interpret user sentiment from text, improving communication.
- Behavioral Targeting: AI tracks user behavior to customize content delivery based on interests and past actions.
"The true power of AI lies in its ability to tailor experiences in real time, adapting to user behavior as it evolves." - Tech Analyst
Impact of AI Personalization Across Industries
Industry | Personalization Benefits |
---|---|
Retail | Customized product recommendations, personalized discounts, and dynamic pricing. |
Entertainment | Curated content, tailored show recommendations, and targeted advertisements. |
Healthcare | Personalized treatment plans, targeted health advice, and proactive care. |
Tracking Brand Sentiment Through AI-Driven Meaning Analysis
In today's highly competitive business environment, understanding customer sentiment is crucial for maintaining a strong brand presence. AI-powered tools that analyze the meaning behind customer feedback, social media posts, and reviews offer businesses a deeper understanding of their brand perception. These tools utilize advanced natural language processing (NLP) and machine learning techniques to assess emotional tones, identify key topics, and detect subtle nuances in consumer language that traditional methods might miss.
The ability to process large volumes of unstructured data enables brands to quickly identify shifts in sentiment, allowing them to respond proactively. By accurately analyzing sentiment trends, brands can tailor their marketing strategies, improve product offerings, and engage with their customers more effectively. AI tools make it possible to not only track positive or negative sentiment but also uncover underlying reasons for customer feelings.
Key Benefits of AI-Driven Sentiment Analysis
- Improved Brand Awareness: AI systems help in identifying real-time shifts in consumer sentiment, providing brands with instant feedback on their campaigns.
- Deeper Insights into Customer Preferences: AI can detect emerging trends and customer concerns that may not be evident through traditional surveys or focus groups.
- Better Crisis Management: By spotting negative sentiment early, brands can take immediate action to address potential public relations issues before they escalate.
How AI Analyzes Sentiment
- Data Collection: AI algorithms gather data from various sources, such as social media, reviews, forums, and news articles.
- Natural Language Processing: NLP tools assess the language used in the text, detecting emotions, tone, and intent.
- Sentiment Scoring: The sentiment is classified as positive, negative, or neutral, with more granular analysis revealing customer emotions such as joy, frustration, or anger.
"AI-powered sentiment analysis provides companies with a scalable way to monitor customer perceptions and react to them in real time, offering a competitive advantage in the marketplace."
Sentiment Analysis Data Breakdown
Sentiment | Percentage |
---|---|
Positive | 50% |
Negative | 30% |
Neutral | 20% |
Streamlining Customer Support with AI-Enhanced Knowledge Extraction
Modern customer support is increasingly reliant on artificial intelligence (AI) to enhance both the speed and accuracy of responses. One of the key developments is AI-powered knowledge extraction, which allows support systems to automatically retrieve relevant information from vast databases. This process not only reduces human workload but also ensures that customers receive consistent and up-to-date answers to their queries. By analyzing large volumes of text, AI can efficiently organize data, making it accessible to support agents or even directly to customers through chatbots or automated responses.
In addition to reducing response times, AI-driven systems improve the overall quality of customer support by tailoring responses to specific needs. Advanced AI algorithms can understand context, identify key information, and filter out irrelevant data. This technology empowers support teams to provide more precise solutions, fostering better customer experiences and increasing satisfaction rates. Below are some significant benefits and applications of AI-enhanced knowledge extraction:
Key Benefits of AI-Powered Knowledge Extraction in Customer Support
- Faster Resolution Times: AI accelerates the process of retrieving and delivering accurate answers, reducing wait times for customers.
- Consistency: AI ensures uniformity in responses, eliminating human errors or variations in how solutions are presented.
- Scalability: AI systems can handle an increasing number of customer interactions without the need for additional human resources.
- Contextual Understanding: Advanced AI algorithms can comprehend the context of customer queries, providing tailored solutions.
AI Tools for Knowledge Extraction
- Natural Language Processing (NLP): NLP techniques enable AI to understand and process customer inquiries, enhancing its ability to extract relevant data.
- Machine Learning Models: These models continuously improve by analyzing feedback, learning from previous interactions to refine the accuracy of the information provided.
- Data Mining Tools: AI uses data mining to sift through vast repositories of information, identifying patterns and knowledge gaps that improve the support process.
"AI-powered knowledge extraction not only optimizes the operational efficiency of customer support teams but also elevates the overall customer experience by delivering more accurate, relevant, and timely information."
AI-Driven Knowledge Extraction: Key Metrics
Metric | Impact |
---|---|
Resolution Time | Reduced by up to 40% |
Customer Satisfaction | Improved by 30% |
Support Costs | Decreased by 20% |
Maximizing ROI through AI-Driven Automated Decision Making
In today's data-rich environment, businesses are under increasing pressure to make fast, informed decisions. The use of artificial intelligence (AI) to automate data-driven decision-making processes can significantly enhance operational efficiency and deliver substantial returns on investment (ROI). By leveraging AI's ability to analyze vast amounts of data at high speed, companies can shift from reactive to proactive decision-making models. This transition not only improves decision accuracy but also speeds up the overall process, enabling faster responses to market changes.
AI-powered systems can identify patterns and trends within complex datasets that are often invisible to human analysts. With the right tools, businesses can harness these insights to optimize various operational aspects, such as marketing campaigns, inventory management, and customer service, all of which contribute to maximizing profitability. The integration of AI ensures that decisions are data-backed, reducing the risks associated with human error and intuition-based choices.
Key Benefits of AI-Driven Decision Making
- Increased Speed: AI can process and analyze large datasets in real-time, enabling quick decision-making.
- Improved Accuracy: AI identifies patterns and trends that humans may overlook, leading to more precise business decisions.
- Cost Efficiency: Automation reduces the need for manual intervention, cutting down on operational costs.
- Scalability: AI systems can handle growing data volumes, ensuring sustained performance as the business expands.
Examples of Automated Decision Making
- Customer Behavior Prediction: AI systems can analyze customer data to predict purchasing behavior, allowing businesses to tailor marketing strategies accordingly.
- Inventory Optimization: AI-driven algorithms can forecast demand and adjust stock levels, minimizing waste and ensuring products are always available when needed.
- Dynamic Pricing: AI can adjust prices in real-time based on market demand, competitor pricing, and other variables, maximizing revenue.
Impact on ROI
Automation of decision-making processes reduces the reliance on human judgment, leading to more consistent and reliable outcomes. This, in turn, enhances ROI by minimizing errors, improving efficiency, and allowing for quicker adaptation to market dynamics.
Example of ROI with AI Implementation
Business Area | Impact on ROI |
---|---|
Customer Insights | Improved targeting and personalized offers, increasing customer conversion rates. |
Operational Efficiency | Reduction in time and resources spent on decision-making processes. |
Inventory Management | Lower operational costs due to optimized stock levels and reduced waste. |