Ai in Xray
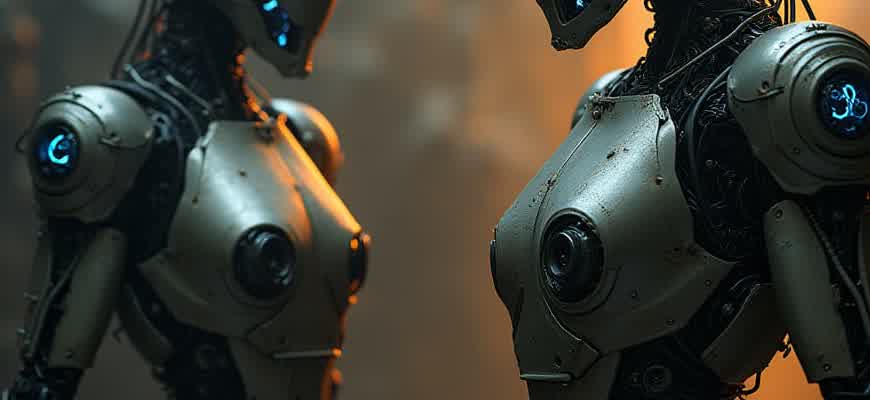
In recent years, the integration of machine learning algorithms into radiology has revolutionized the way medical professionals interpret X-ray images. AI technologies are now able to assist in the detection of abnormalities, improving diagnostic accuracy and reducing human error.
These advancements have led to several key benefits:
- Enhanced detection of early-stage diseases, such as cancer or fractures.
- Faster analysis of large volumes of X-ray images.
- Support for radiologists in decision-making, particularly in busy environments.
"AI can reduce the time needed for diagnosis and potentially improve the outcomes of treatment by identifying issues that may be overlooked by the human eye."
One example of AI in X-ray imaging is the development of deep learning models, which can be trained to recognize patterns in images with a high degree of precision. These models typically rely on convolutional neural networks (CNNs) to process and analyze X-ray scans.
Key aspects of AI-powered X-ray analysis include:
- Image preprocessing to enhance clarity and highlight areas of concern.
- Automated detection of specific conditions such as pneumonia, fractures, or tumors.
- Real-time decision support for medical professionals.
The following table highlights some common AI applications in X-ray analysis:
Condition | AI Solution | Impact |
---|---|---|
Pneumonia | AI algorithms detect signs of infection in lung tissue. | Improves speed and accuracy of pneumonia detection. |
Fractures | AI detects fractures in bones, particularly in difficult-to-spot areas. | Reduces misdiagnosis and enhances treatment planning. |
Lung Cancer | AI analyzes patterns in lung X-rays to identify potential tumors. | Increases early detection, improving patient outcomes. |
AI in X-ray: Practical Applications and Innovations
In recent years, artificial intelligence has revolutionized the way X-ray imaging is utilized in the medical field. By applying advanced machine learning algorithms, AI systems are able to assist radiologists in analyzing X-ray images with unprecedented accuracy and speed. These AI-driven systems can detect abnormalities, enhance image quality, and provide valuable insights that were previously time-consuming or difficult to identify manually.
AI is now an integral part of diagnostic procedures, helping to streamline workflows and reduce human error. By automating routine tasks, AI applications free up valuable time for healthcare professionals to focus on more complex cases. The impact of AI on X-ray technology extends beyond diagnostics, contributing to innovations in imaging techniques and patient care strategies.
Key Applications of AI in X-ray Imaging
- Automated Abnormality Detection: AI models can detect and highlight various conditions, such as tumors, fractures, or infections, with higher precision than traditional methods.
- Image Enhancement: AI algorithms improve the quality of X-ray images by reducing noise and increasing contrast, aiding in better visibility of underlying issues.
- Personalized Diagnosis: AI systems adapt to individual patient characteristics, ensuring that diagnoses are tailored to each case, resulting in more accurate and effective treatments.
Innovative Uses of AI in X-ray Technology
- Real-time Diagnostics: AI-powered X-ray systems enable immediate results, allowing healthcare providers to make faster decisions in emergency situations.
- Predictive Analytics: AI can predict potential health issues based on X-ray scans, providing early warnings for conditions like osteoporosis or lung diseases.
- Telemedicine: AI-driven systems facilitate remote X-ray readings, expanding access to expert diagnoses in underserved areas.
AI in X-ray technology is not only transforming diagnosis but also improving patient outcomes by allowing for earlier detection and intervention.
AI Tools for X-ray Imaging
Tool | Function | Use Case |
---|---|---|
Deep Learning Algorithms | Pattern recognition, classification of abnormalities | Detection of lung cancer, bone fractures |
Image Enhancement Software | Noise reduction, image contrast enhancement | Improved quality of chest X-rays |
AI-based Predictive Models | Risk assessment and prognosis prediction | Predicting progression of heart disease, osteoporosis |
Optimizing Image Quality with AI Algorithms in X-ray Scanning
AI-driven technologies have revolutionized various aspects of medical imaging, and X-ray scanning is no exception. By leveraging advanced machine learning techniques, AI algorithms can significantly enhance the clarity and diagnostic value of X-ray images. These algorithms can improve image resolution, reduce noise, and optimize contrast, which directly contributes to more accurate diagnostics and better patient outcomes.
One of the key benefits of using AI in X-ray imaging is its ability to automatically adjust the parameters of the scan to ensure optimal image quality. Traditional methods often require manual calibration or intervention, but AI can intelligently make adjustments in real-time based on the specific characteristics of the subject being scanned.
AI Techniques for Image Quality Enhancement
- Noise Reduction: AI models can identify and eliminate low-level noise in images, leading to clearer scans that are easier to interpret.
- Resolution Improvement: By analyzing multiple frames, AI algorithms can reconstruct higher-resolution images, even from low-quality X-rays.
- Contrast Optimization: AI can automatically adjust the contrast of images to highlight areas of interest, improving visibility of fine details.
Key Advantages of AI in Image Quality Optimization:
- Consistency: AI ensures consistent image quality across different scanners and settings, eliminating human error in calibration.
- Efficiency: Automating image enhancement reduces the time needed for post-processing, speeding up diagnostic workflows.
- Accuracy: Enhanced image quality directly leads to more accurate diagnosis and treatment planning.
AI-based systems can learn from vast amounts of data, continuously improving their ability to predict the optimal image parameters for various clinical scenarios.
Technique | Benefit |
---|---|
Noise Reduction | Improved image clarity and easier interpretation |
Resolution Enhancement | Sharper images with more detail |
Contrast Optimization | Better visibility of critical areas |
Reducing Diagnostic Time with AI-Powered X-ray Analysis
AI technology has revolutionized the way medical imaging is processed, especially in the field of radiology. With the increasing volume of X-ray scans being performed, manual interpretation by radiologists can be time-consuming and prone to human error. AI-driven tools now offer an efficient alternative to traditional methods, significantly speeding up the diagnostic process without compromising accuracy.
The integration of AI into X-ray analysis enables automated detection of abnormalities, pattern recognition, and faster reporting. By processing large datasets in real-time, AI can prioritize critical cases, assisting radiologists in focusing on high-priority scans. This reduces overall diagnostic time and accelerates decision-making for patient treatment.
Key Benefits of AI in X-ray Analysis
- Increased efficiency: AI systems can analyze X-ray images almost instantly, reducing the time spent on image interpretation and enabling faster reporting.
- Accurate detection: AI algorithms, trained on vast amounts of data, can detect even subtle abnormalities, improving diagnostic accuracy and helping to avoid missed diagnoses.
- Real-time results: Immediate feedback from AI tools provides radiologists with quick insights, facilitating prompt treatment and care decisions.
- Reduced workload: AI can handle routine or less complex cases, allowing radiologists to concentrate on more complicated diagnostic tasks.
Example of AI Application in X-ray
Feature | Manual Interpretation | AI-Powered Interpretation |
---|---|---|
Time to Analyze | Several minutes per image | Less than 30 seconds per image |
Accuracy | Dependent on radiologist experience | High accuracy, with minimal errors |
Workload | Radiologists must review every image | AI handles routine cases, reducing workload |
"AI's role in enhancing radiological workflows is not only about speed but also precision, allowing radiologists to spend more time on complex cases that require human expertise."
AI-Powered Detection of Common and Rare Conditions in X-ray Images
Artificial intelligence (AI) has significantly advanced the diagnostic capabilities of radiology, especially in detecting both common and rare medical conditions from X-ray scans. By training algorithms on large datasets, AI systems can quickly analyze images, identifying patterns and anomalies that may go unnoticed by human eyes. These systems have been shown to reduce diagnostic errors and improve the speed and accuracy of medical assessments, making them an essential tool for healthcare professionals.
AI applications in radiology focus on automating the process of recognizing abnormalities in X-ray images, whether they are frequent conditions like pneumonia or rare disorders such as pulmonary fibrosis. With AI models learning from vast collections of annotated data, they have the potential to recognize subtle features, enhancing the early detection of diseases and supporting timely intervention.
Key Benefits of AI in X-ray Diagnosis
- Faster Diagnosis: AI can analyze X-ray images in seconds, reducing the time needed for diagnosis and allowing for quicker decision-making in emergency and routine care settings.
- Increased Accuracy: By identifying patterns invisible to the human eye, AI can minimize the risk of misdiagnoses and false negatives.
- Improved Access: AI-based tools enable healthcare providers, especially in underserved regions, to offer expert-level diagnostics without requiring a radiologist on-site.
AI's Application to Common and Rare Conditions
AI-based systems excel in detecting both prevalent and rare diseases in chest X-rays. For instance, the detection of pneumonia and tuberculosis can be enhanced by deep learning models, which quickly pinpoint areas of concern. In contrast, for rarer diseases like sarcoidosis or idiopathic pulmonary fibrosis, AI can be trained to identify nuanced patterns, thus assisting clinicians in identifying these conditions before they reach advanced stages.
"AI systems are capable of identifying both common and rare conditions, providing a valuable tool in early diagnosis and preventive healthcare."
Comparison of AI Performance for Various Conditions
Condition | AI Accuracy (%) | Clinical Relevance |
---|---|---|
Pneumonia | 95% | Common infection, critical in emergency care |
Pulmonary Fibrosis | 87% | Rare, but critical for early intervention |
Tuberculosis | 90% | Common in specific regions, requires rapid detection |
Sarcoidosis | 80% | Rare, requires careful differentiation from other lung diseases |
Integrating AI with Existing X-ray Equipment for Seamless Workflow
Integrating artificial intelligence into traditional X-ray machines offers a significant advantage in terms of workflow optimization. By embedding AI algorithms directly into existing imaging systems, radiologists can reduce diagnostic time and improve accuracy. AI tools can automatically analyze X-ray images and highlight potential areas of concern, freeing up clinicians to focus on complex cases. The integration is designed to ensure minimal disruption to current practices, enhancing productivity without requiring complete overhauls of existing equipment.
To effectively incorporate AI into current X-ray infrastructure, seamless compatibility is key. AI systems must be designed to work with the specific hardware and software configurations of the X-ray equipment already in use. This integration should prioritize user-friendly interfaces and automated processes, enabling radiologists to access AI-driven insights with little effort. Proper training and ongoing support are crucial to maximize the benefits of AI-enhanced diagnostics.
Key Benefits of AI Integration
- Enhanced Detection: AI algorithms are capable of detecting subtle abnormalities that might be overlooked by human eyes.
- Faster Diagnosis: AI can provide preliminary analysis, reducing the time needed for radiologists to review and interpret X-ray images.
- Improved Workflow: Seamless integration with existing equipment allows for a more efficient diagnostic process, avoiding disruptions in clinical routines.
Steps for AI Integration
- Assess compatibility of AI software with current X-ray machine models.
- Install necessary hardware and software updates for AI integration.
- Train radiologists and technicians on using AI-enhanced features.
- Establish a feedback loop for continuous improvement of AI algorithms based on user experience.
"AI integration must complement and enhance existing systems, not replace them. The goal is to assist, not automate the entire diagnostic process."
Considerations for Successful Implementation
Aspect | Consideration |
---|---|
Compatibility | Ensure AI software can seamlessly operate with various X-ray models. |
Data Security | Implement robust security protocols to protect patient data during AI processing. |
User Training | Provide comprehensive training for all users to ensure effective use of AI tools. |
Enhancing Accuracy in X-ray Interpretation Using AI-Driven Insights
AI-driven technologies are revolutionizing the way medical professionals analyze X-ray images. By leveraging deep learning algorithms, AI can detect subtle patterns and anomalies that may be missed by the human eye. This increased sensitivity enhances the precision of diagnoses, especially in complex cases where traditional methods might struggle. AI tools assist radiologists by providing real-time, data-driven insights, which help in identifying conditions like fractures, tumors, or infections at early stages, improving patient outcomes significantly.
The integration of AI into X-ray diagnostics offers several advantages, including reduced human error and faster results. AI systems continuously learn from large datasets, improving their performance over time and becoming more adept at recognizing specific diseases and conditions. As a result, the role of the radiologist evolves into one that focuses more on decision-making and patient care, while AI assists in improving diagnostic accuracy and workflow efficiency.
Key Benefits of AI in X-ray Analysis
- Increased Detection Accuracy: AI systems can detect abnormalities with higher sensitivity, reducing the chances of false negatives or missed diagnoses.
- Faster Diagnosis: AI tools provide immediate results, helping healthcare professionals to make timely decisions.
- Reduced Workload for Radiologists: By automating routine tasks, AI frees up radiologists to focus on more complex cases.
Challenges and Considerations
- Data Quality: AI models rely heavily on high-quality data. Inaccurate or incomplete datasets can lead to suboptimal results.
- Training and Integration: Proper training is required for medical personnel to interpret AI-driven insights effectively and integrate these systems into existing workflows.
- Ethical and Privacy Concerns: The use of AI in medical diagnostics raises questions about data security and patient confidentiality.
"AI can significantly reduce the time required for diagnosis, enabling quicker interventions and better patient outcomes."
Examples of AI in X-ray Diagnostics
Condition | AI Application | Impact |
---|---|---|
Fractures | Detection of small, complex fractures | Improved fracture detection rates, reducing misdiagnosis |
Breast Cancer | AI-enhanced mammography analysis | Higher accuracy in identifying early-stage breast cancer |
Pneumonia | Automated lung infection identification | Quicker and more accurate pneumonia detection |
AI for Automating X-ray Image Labeling and Reporting
Automated labeling and reporting of X-ray images using AI technologies is transforming radiology workflows. Traditional manual methods of labeling images and generating reports are time-consuming and prone to human error. By implementing AI-driven systems, medical professionals can save time and improve diagnostic accuracy. AI models can quickly analyze images, identify key features, and generate descriptive labels based on the findings, reducing the burden on radiologists.
AI-based systems are trained to recognize patterns in X-ray images, which allows them to automatically categorize abnormalities and generate preliminary reports. This process not only accelerates the diagnostic process but also improves the consistency of reports. As these systems evolve, they have the potential to become more sophisticated, assisting in early detection and offering decision support for radiologists.
Key Benefits of AI in Image Labeling and Reporting
- Speed: AI can quickly label X-ray images, reducing the time required for manual annotation.
- Accuracy: AI models are trained on vast datasets, allowing them to identify abnormalities with high precision.
- Consistency: AI eliminates human subjectivity, ensuring uniformity in reports across different cases and radiologists.
- Scalability: AI systems can handle large volumes of images, making them ideal for high-throughput environments.
How AI Systems Work in X-ray Image Labeling
- Image Preprocessing: Raw X-ray images are cleaned and optimized for analysis.
- Feature Extraction: AI models analyze the image to detect patterns, edges, and anomalies.
- Label Generation: The system assigns labels based on predefined categories such as fractures, tumors, or infections.
- Report Generation: A comprehensive report is created, summarizing findings and offering recommendations.
Example Workflow
Step | Action |
---|---|
Step 1 | Preprocess the X-ray image for optimal AI analysis. |
Step 2 | AI detects abnormalities and extracts relevant features. |
Step 3 | AI generates labels for identified conditions, such as fractures or tumors. |
Step 4 | A detailed report is automatically generated and ready for review. |
"AI's ability to enhance diagnostic processes in radiology is transformative, providing faster and more accurate results while alleviating the workload of medical professionals."
Enhancing Patient Outcomes with AI-Enhanced X-ray Analysis
AI-assisted X-ray diagnostics offer a significant opportunity to transform medical imaging and improve patient care. By integrating artificial intelligence into radiology, healthcare professionals can achieve faster, more accurate interpretations, enabling early detection and more precise treatment plans. This approach minimizes the risk of human error, ensures that subtle abnormalities are not overlooked, and provides clinicians with better tools for decision-making.
Furthermore, AI systems can continuously learn and evolve from a vast array of medical images, improving their diagnostic capabilities over time. This leads to more reliable assessments, particularly in complex cases where traditional methods might struggle. By providing a second layer of analysis, AI can prioritize cases based on severity, allowing for more efficient allocation of healthcare resources and faster intervention for critical patients.
Benefits of AI-Assisted X-ray Diagnostics
- Faster Diagnosis: AI algorithms process X-ray images quickly, reducing waiting times for both patients and doctors.
- Improved Accuracy: AI reduces the likelihood of missed diagnoses by identifying patterns that may be difficult for human eyes to detect.
- Enhanced Consistency: AI systems ensure that interpretations are standardized, minimizing variations between different radiologists.
- Early Detection: AI can identify signs of conditions such as cancer or fractures at an early stage, improving patient outcomes.
"AI-assisted X-ray technology is a game-changer in radiology, offering the potential to save lives through earlier diagnosis and more precise treatment planning." – Medical Expert
Key Features of AI in X-ray Diagnostics
- Automated Image Analysis: AI tools analyze X-ray images automatically, flagging potential concerns and assisting clinicians in identifying abnormalities.
- Predictive Analytics: AI models can predict the progression of conditions based on current imaging data, helping doctors plan long-term care.
- Real-Time Feedback: AI provides instant results, reducing the time between diagnosis and treatment, which is critical for conditions like pneumonia or fractures.
AI-Assisted X-ray Diagnostic Accuracy vs Traditional Methods
Method | Accuracy | Speed |
---|---|---|
Traditional Radiology | 85-90% | Varies based on radiologist availability |
AI-Assisted X-ray | 95-99% | Immediate feedback |
AI in X-ray Radiation Dose Reduction: How It Works
Artificial intelligence has become an essential tool in optimizing X-ray imaging, particularly in reducing radiation exposure for patients. AI-based algorithms analyze images in real-time to enhance image quality while minimizing the required radiation dose. These systems use deep learning models to process data and identify patterns that would typically be missed, enabling better image clarity with less radiation.
AI in radiology can intelligently adjust scanning parameters, analyze patient data, and automatically optimize the imaging process, all of which contribute to reducing exposure. The integration of AI into X-ray systems plays a significant role in making diagnostic imaging safer without compromising diagnostic accuracy.
How AI Contributes to Radiation Dose Reduction
AI assists in several ways to reduce the amount of radiation used during X-ray imaging procedures:
- Real-time Image Enhancement: AI-based algorithms refine image quality by reducing noise and improving resolution without increasing radiation levels.
- Optimized Exposure Settings: AI systems automatically adjust the X-ray machine's exposure settings based on the patient's size, anatomy, and the clinical question, lowering unnecessary radiation.
- Post-processing Image Reconstruction: AI can enhance or reconstruct images after the scan, reducing the need for higher radiation doses during the actual scan.
AI can lower radiation exposure significantly, ensuring that the diagnostic quality is maintained while keeping patient safety a top priority.
Benefits of AI-Driven Dose Reduction
Benefit | Explanation |
---|---|
Reduced Radiation Risks | By optimizing exposure settings, AI reduces unnecessary radiation, lowering the risk of radiation-related health issues. |
Improved Diagnostic Confidence | AI enhances image quality, allowing for better diagnostics without the need for higher doses of radiation. |
Increased Patient Comfort | Lower radiation doses mean faster and more comfortable imaging procedures for patients. |