Best Ai Platform for Data Analysis
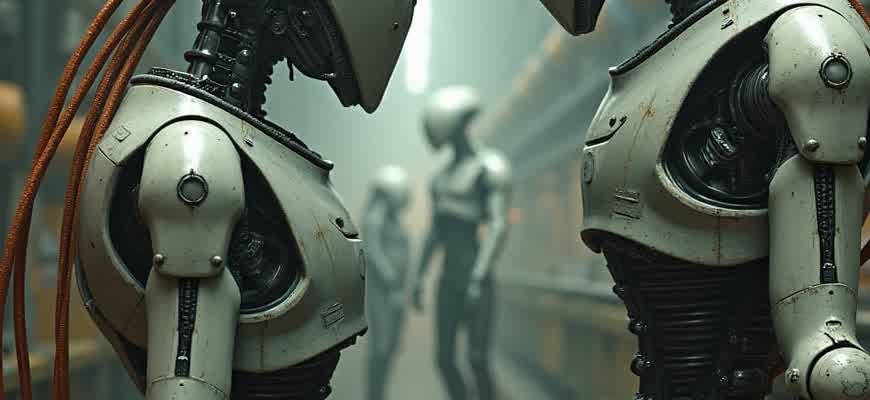
In the rapidly evolving field of data analytics, selecting the right AI-powered platform can make a significant difference in the quality of insights derived from data. The best platforms offer a blend of powerful machine learning tools, robust data visualization capabilities, and easy-to-use interfaces. Below are some of the leading options for professionals and businesses seeking to leverage AI in their data analysis tasks.
- Google Cloud AI Platform: Known for its scalability, advanced machine learning tools, and integration with other Google services.
- IBM Watson: Offers deep learning models and natural language processing capabilities that excel in extracting insights from unstructured data.
- Microsoft Azure AI: Provides a wide range of analytics and machine learning tools with easy integration into existing IT ecosystems.
The following table compares key features of each platform:
Platform | Key Features | Best For |
---|---|---|
Google Cloud AI | Scalable ML models, integration with Google BigQuery, TensorFlow support | Large-scale analytics, deep learning |
IBM Watson | Natural language processing, data-driven insights, predictive analytics | Text analysis, industry-specific solutions |
Microsoft Azure AI | Wide variety of analytics tools, seamless integration with Microsoft services | Comprehensive enterprise analytics |
When choosing an AI platform for data analysis, it’s crucial to consider the specific needs of your business, including the types of data you handle and your team’s technical expertise.
Top AI Platforms for Data Analytics
When it comes to advanced data analytics, choosing the right AI platform is critical for achieving reliable insights and making data-driven decisions. Various platforms offer distinct features tailored to specific needs, ranging from machine learning model training to data visualization and predictive analytics. These tools can significantly enhance the efficiency of data analysis and improve overall business performance.
Several AI platforms are widely recognized for their capabilities in handling complex datasets, offering intuitive interfaces and powerful algorithms. Below are some of the best AI platforms to consider for robust data analysis.
Leading AI Tools for Data Analytics
- Google Cloud AI: Known for its scalability, Google Cloud AI is a powerful platform that integrates various machine learning services, such as TensorFlow and BigQuery, to process large datasets and perform predictive analytics.
- Microsoft Azure AI: This platform provides a range of analytics services, including Azure Machine Learning and Cognitive Services, enabling data scientists and developers to build, deploy, and manage AI models with ease.
- IBM Watson: Offering robust data analysis capabilities, IBM Watson integrates AI, machine learning, and natural language processing to help businesses derive actionable insights from structured and unstructured data.
- Amazon SageMaker: With a focus on machine learning, SageMaker allows users to quickly build, train, and deploy machine learning models at scale, supporting various algorithms and frameworks.
Comparison Table of AI Platforms
Platform | Key Features | Strengths |
---|---|---|
Google Cloud AI | TensorFlow, BigQuery, AutoML | Scalable, customizable, integrated with Google services |
Microsoft Azure AI | Machine Learning, Cognitive Services | Comprehensive, supports a wide range of models |
IBM Watson | Natural Language Processing, Predictive Analytics | Advanced NLP, strong industry-specific solutions |
Amazon SageMaker | Model Building, Training, Deployment | Flexible, integrates with AWS ecosystem |
Key Insight: When selecting an AI platform for data analysis, it’s important to consider your specific needs–whether it's scalability, ease of deployment, or advanced machine learning features.
How to Choose the Right AI Platform for Your Data Needs
Selecting an AI platform for data analysis requires a comprehensive understanding of the specific needs of your project. The platform should align with your business goals, data types, and available resources. Key factors like data volume, processing speed, and the complexity of algorithms are crucial when evaluating your options. It's important to assess whether the platform can scale with your data as it grows and whether it offers the flexibility to integrate with existing tools and infrastructure.
When selecting an AI tool, consider factors such as ease of use, cost, customer support, and available training resources. An ideal platform should provide sufficient documentation and active community support. It's also vital to evaluate the security features to ensure your data is protected. Here are some factors to guide your decision-making process:
- Data compatibility: Does the platform support various data types such as structured, unstructured, or real-time data?
- Scalability: Can the platform handle increased data loads without compromising performance?
- AI capabilities: Does it provide advanced machine learning, deep learning, and analytics tools?
- Integration with existing systems: Can it seamlessly connect with your current data infrastructure?
"The right AI platform should not only address your immediate needs but also scale with your future requirements. It’s essential to test how well it integrates into your existing workflows."
Key Criteria for Selecting the Platform
To make an informed decision, use the following criteria to evaluate AI platforms:
Criterion | Importance | Considerations |
---|---|---|
Ease of Use | High | Look for user-friendly interfaces, customizable dashboards, and a low learning curve. |
Cost | Medium | Compare pricing models–subscription vs. pay-per-use–and ensure it fits your budget. |
Support | High | Access to technical support, training materials, and community forums. |
Security | Critical | Ensure the platform offers robust security protocols and data encryption. |
"Security and ease of use are paramount when selecting an AI platform, especially for organizations with sensitive data."
Key Features to Look for in AI Tools for Data Analysis
When selecting an AI tool for data analysis, it's crucial to focus on specific features that enhance data processing, visualization, and predictive capabilities. With the increasing complexity of data sets, AI solutions must offer robust tools that can handle large volumes of information, clean data efficiently, and provide actionable insights. The following key features are essential for maximizing the effectiveness of AI-driven data analysis platforms.
AI platforms should also integrate easily with existing systems, ensuring smooth workflows and compatibility with various data sources. A versatile tool will help analysts and data scientists make more informed decisions through advanced algorithms and automation features.
Essential Features for AI Data Analysis Tools
- Data Preprocessing & Cleaning: Tools for handling missing values, outliers, and ensuring data quality.
- Scalability: The ability to process large-scale datasets without compromising speed or accuracy.
- Advanced Analytics: Machine learning models for clustering, classification, and regression tasks.
- Automation: Automated data analysis pipelines for faster insights generation.
- Visualization Tools: Interactive dashboards and advanced plotting to better interpret data trends.
Automated data preprocessing is a game-changer, as it reduces the need for manual data cleaning and speeds up analysis.
Important Considerations for AI Tools
- Integration with Data Sources: Ensure the tool supports various file formats and databases (e.g., SQL, CSV, and cloud storage systems).
- Customizability: The ability to modify and create custom algorithms tailored to specific business needs.
- Security: Safeguarding sensitive data by providing encryption and compliance with regulations.
Feature | Importance |
---|---|
Data Cleaning | Ensures high-quality, reliable datasets for analysis |
Scalability | Handles large datasets without affecting performance |
Automation | Reduces manual tasks, speeding up analysis |
Integration of AI Platforms with Existing Data Infrastructure
Integrating AI platforms into an organization's existing data infrastructure is a critical step towards enabling data-driven decision-making. Such integration ensures that AI tools can leverage existing databases, pipelines, and cloud services without disrupting current workflows. Seamless integration not only optimizes the performance of the AI models but also allows businesses to extract meaningful insights from historical data while leveraging advanced machine learning techniques.
However, successful integration requires thorough planning, understanding the system architecture, and determining compatibility between AI tools and the current data environment. The process typically involves several steps, including data preparation, system configuration, and ongoing monitoring. It's essential to ensure that the data infrastructure is scalable and flexible to accommodate evolving AI needs.
Key Considerations for Integration
- Data Consistency: Ensuring that data flows consistently between systems is crucial. AI platforms must work with structured and unstructured data from multiple sources.
- Scalability: The platform should scale with the growth of data, allowing the AI models to expand and improve over time without significant infrastructure changes.
- Data Security: Implement robust security protocols to safeguard sensitive data during the integration process, ensuring compliance with data protection regulations.
Steps for Successful Integration
- Assess the current data infrastructure and AI platform requirements.
- Map out the necessary APIs and data connectors for smooth communication between platforms.
- Test integration on smaller datasets to identify potential issues.
- Scale the integration process as required, ensuring all data sources are properly connected.
- Implement monitoring and reporting tools to track the system's performance post-integration.
Example Data Integration Structure
Data Source | Integration Tool | AI Model |
---|---|---|
Cloud Storage | API Connector | Predictive Analytics |
CRM System | ETL Pipeline | Customer Segmentation |
Sales Database | SQL Query Integration | Sales Forecasting |
Note: Proper data integration enables AI systems to learn from real-time and historical data simultaneously, resulting in more accurate predictions and insights.
Cost-Benefit Evaluation: Is AI Investment Worthwhile for Data Analysis?
Investing in AI platforms for data analysis is a decision many organizations face, especially as the demand for faster and more accurate insights grows. While AI offers powerful capabilities in automating tasks and enhancing decision-making, the costs involved in adoption and implementation can be substantial. It's essential to carefully consider whether the potential benefits outweigh the investment required. In this analysis, we explore the costs and benefits of using AI for data analytics and how to determine if it’s a wise choice for your business.
Before committing to AI solutions, organizations must weigh the financial outlay, including software acquisition, training, and maintenance, against the value AI can provide. While the return on investment (ROI) may not be immediately evident, the long-term benefits in terms of efficiency, scalability, and actionable insights often justify the upfront costs. However, understanding the specific use cases and business needs is crucial to make an informed decision.
Key Factors to Consider
- Initial Investment: The cost of acquiring and implementing AI tools, including software licensing and integration, can be high, especially for small to medium-sized enterprises.
- Operational Efficiency: AI tools can significantly reduce manual data processing time, leading to faster analysis and quicker decision-making.
- Scalability: AI platforms can handle large datasets effortlessly, which becomes increasingly important as data volumes grow over time.
- Expertise Requirements: Hiring or training staff with the necessary expertise in AI and data science can add to the cost, but it's essential for maximizing the platform's potential.
- Ongoing Maintenance: Continuous updates, troubleshooting, and optimizations of AI systems can lead to additional operational costs.
Benefits of AI-Driven Data Analysis
- Speed: AI algorithms can process vast amounts of data at speeds impossible for human analysts, providing real-time insights.
- Accuracy: AI reduces human error and inconsistencies, improving the reliability of results.
- Predictive Insights: With machine learning models, AI can forecast trends, offering valuable foresight for strategic planning.
Cost-Benefit Comparison
Cost | Benefit |
---|---|
High initial investment for software and integration | Long-term savings due to reduced labor costs and time saved on data processing |
Requirement for specialized expertise and training | Improved decision-making and increased competitive advantage through data-driven insights |
Ongoing maintenance and upgrades | Scalable solution that grows with data needs, ensuring future-proofing |
Key Takeaway: For organizations handling large datasets or requiring high levels of accuracy and speed, AI is often a worthwhile investment. The key lies in aligning the technology with specific business needs and having the right expertise to maximize its potential.
Scalability of AI Platforms for Growing Data Sets
As organizations accumulate large and complex data sets, it becomes essential for AI platforms to efficiently handle and scale with these growing volumes of information. The ability of AI platforms to manage increasing data loads is crucial for ensuring timely and accurate analysis, particularly as the scope of data expands exponentially. A scalable platform can maintain performance levels while accommodating additional data without a loss in speed or precision. This is especially significant in industries like healthcare, finance, and e-commerce, where real-time insights are imperative for decision-making.
To effectively support scalability, AI platforms rely on advanced infrastructure and algorithms that can distribute workloads, optimize data processing, and ensure high availability. Several factors contribute to the scalability of AI systems, such as data storage solutions, parallel processing capabilities, and cloud-based technologies that allow dynamic resource allocation. Moreover, an effective scaling strategy is not only about handling large data volumes but also about maintaining data integrity, minimizing latency, and ensuring the system can be easily expanded as required.
Key Factors Influencing Scalability
- Data Storage: Cloud-based storage and distributed databases provide flexible solutions for managing massive amounts of data. They offer scalability through elasticity and can expand or contract resources based on demand.
- Parallel Processing: Techniques like data parallelism and model parallelism allow AI systems to break tasks into smaller chunks, enabling faster and more efficient processing of large datasets.
- Optimized Algorithms: AI platforms using optimized algorithms and machine learning models are designed to scale more efficiently by utilizing available resources and minimizing computational overhead.
Strategies for Ensuring Scalable AI Solutions
- Leverage cloud infrastructure to ensure elastic resource management and cost-effective scaling.
- Implement distributed computing to split workloads across multiple processors or servers for improved processing power.
- Use data partitioning techniques to divide large datasets into smaller, manageable parts that can be processed concurrently.
"Scalability is not just about handling more data; it’s about maintaining performance as data volume, variety, and velocity increase."
Example: Cloud vs On-Premise Solutions
Aspect | Cloud Solutions | On-Premise Solutions |
---|---|---|
Resource Scalability | Highly elastic, resources can be expanded or reduced on-demand | Limited, requires physical upgrades and hardware investments |
Cost Efficiency | Pay-as-you-go model, reducing upfront costs | High initial capital expenditure and ongoing maintenance costs |
Performance | High availability with optimized performance for large datasets | Potential for performance bottlenecks as data volumes grow |
Data Security Considerations When Leveraging AI for Data Analysis
AI-powered platforms for data analysis have become indispensable for extracting insights from vast amounts of information. However, the integration of AI tools into data analysis processes brings forth significant concerns regarding the confidentiality and integrity of the data being processed. Protecting sensitive data is crucial, as AI systems often rely on complex algorithms and massive datasets, which can be vulnerable to malicious attacks, data leaks, and breaches.
To mitigate these risks, companies must implement robust security measures to ensure that data is adequately protected throughout its lifecycle, from collection to processing and storage. The following are key considerations to ensure the security of data when using AI for analysis.
Key Security Measures to Implement
- Data Encryption: Ensure that data is encrypted both at rest and in transit to prevent unauthorized access during processing.
- Access Control: Implement strict access policies, using role-based access controls (RBAC) to limit who can view or manipulate sensitive data.
- Secure Data Storage: Store sensitive information in secure environments, using secure cloud platforms or on-premise solutions with enhanced security protocols.
- Audit Trails: Maintain detailed logs of all interactions with the AI system to track data usage, changes, and potential security breaches.
Additional Considerations
- Data Anonymization: Employ data anonymization techniques to mask personally identifiable information (PII) while maintaining the usefulness of the data for analysis.
- Model Transparency: Ensure that AI models used for data analysis are transparent and auditable to identify and address any security flaws that may arise during training or deployment.
- Regular Security Audits: Conduct regular audits and penetration tests to assess the security posture of the AI platform and address vulnerabilities.
Security Best Practices
“Adopting a security-first approach and integrating data protection strategies into the AI development lifecycle is crucial for minimizing risks and ensuring compliance with privacy regulations.”
Data Security Risk Factors Table
Risk Factor | Description | Mitigation Strategy |
---|---|---|
Data Breach | Unauthorized access to sensitive data through hacking or exploitation of vulnerabilities. | Encryption, Multi-Factor Authentication (MFA), Secure APIs |
Data Corruption | Modification or loss of data integrity during analysis or storage. | Data backups, Integrity checks, Access controls |
Model Insecurity | Exploitation of weaknesses in the AI model, leading to incorrect analysis or data leakage. | Regular model audits, Red teaming, Secure model deployment |
How AI Algorithms Enhance Data Insights and Decision-Making
AI algorithms have become instrumental in transforming raw data into actionable insights. By leveraging advanced machine learning techniques, businesses can uncover patterns and trends that would otherwise go unnoticed. These insights enable companies to make data-driven decisions, optimize processes, and ultimately improve their bottom line. The core advantage lies in the ability of AI to process large datasets quickly and accurately, allowing for more informed and timely decision-making.
Moreover, AI-powered platforms provide real-time analytics, which is crucial for businesses operating in fast-paced environments. These platforms are equipped to handle various types of data–whether structured or unstructured–and can adapt to different industry needs. By automating repetitive tasks and analyzing complex datasets, AI significantly reduces human error and enhances the precision of business decisions.
Key Benefits of AI in Decision-Making
- Faster Decision-Making: AI accelerates the decision-making process by providing real-time data analysis.
- Improved Accuracy: Algorithms eliminate biases and human error, resulting in more reliable insights.
- Predictive Capabilities: AI models forecast future trends, helping businesses stay ahead of the competition.
- Cost Efficiency: By automating complex tasks, AI reduces operational costs and frees up resources.
AI’s Impact on Data Analysis: A Comparison
Traditional Data Analysis | AI-Driven Data Analysis |
---|---|
Relies heavily on manual input and human interpretation. | Automates data processing, providing insights faster and more accurately. |
Limited scalability when handling large datasets. | Can process vast amounts of data in real-time, allowing for scalable insights. |
Subject to human biases and errors in analysis. | AI algorithms reduce biases and increase the reliability of the outcomes. |
AI is revolutionizing the way businesses approach data analysis, enabling smarter and more efficient decision-making at every level.