Learning Analytics Courses
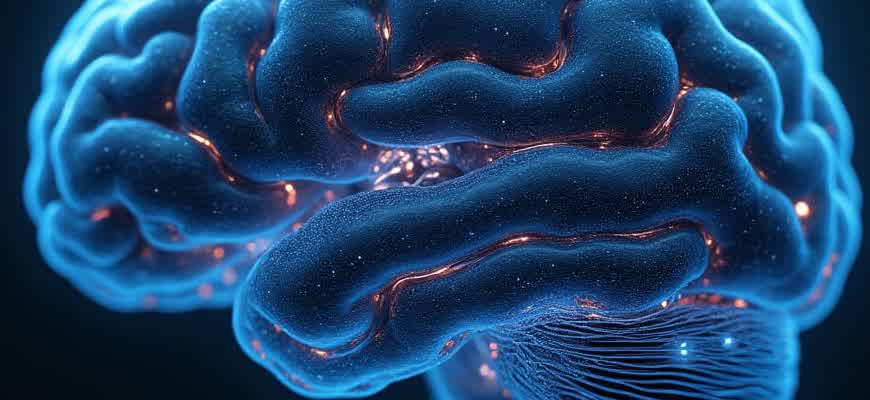
Learning analytics is a rapidly growing field that applies data analysis techniques to improve educational outcomes. Many institutions are now offering specialized programs that provide learners with the skills to analyze educational data and implement strategies for better learning experiences.
These courses generally focus on key areas such as:
- Data collection and interpretation in education
- Predictive modeling and algorithms
- Visualization tools for educational data
Some courses emphasize a hands-on approach with real-world data sets, while others provide theoretical foundations for understanding how data can influence decision-making processes in education.
Courses in learning analytics equip students with essential tools to not only analyze student performance but also to foster personalized learning experiences.
Here is a table summarizing some common topics covered in learning analytics programs:
Topic | Key Skills | Duration |
---|---|---|
Introduction to Learning Analytics | Basic data analysis, education data interpretation | 4 weeks |
Advanced Predictive Modeling | Machine learning, regression analysis | 8 weeks |
Visualization and Reporting | Data visualization tools, dashboard creation | 6 weeks |
Key Competencies Developed Through Learning Analytics Training for Educators
Training in learning analytics equips educators with a wide array of valuable skills that can enhance their teaching effectiveness and student outcomes. By utilizing data-driven approaches, educators can better understand student behaviors, performance trends, and learning patterns. These insights enable them to make informed decisions that improve learning environments, interventions, and overall academic success.
One of the core strengths of learning analytics is its ability to transform raw data into actionable insights. Educators who undergo this training gain competencies that are directly applicable to real-world classroom settings, allowing them to track student progress and predict potential challenges with greater accuracy.
Essential Skills Gained
- Data Interpretation: Educators learn how to analyze various forms of student data, such as assessment scores, participation metrics, and engagement levels, to uncover trends and identify areas for improvement.
- Predictive Analytics: Training helps educators understand how to forecast student performance and identify at-risk students early on, allowing for timely interventions.
- Personalized Learning: Educators can use learning analytics to customize educational content and experiences based on individual student needs, ensuring a more tailored approach to teaching.
- Learning Outcome Evaluation: Educators are trained to assess the effectiveness of teaching strategies and curricula, refining them based on student feedback and performance data.
Practical Applications in Teaching
"Learning analytics training empowers educators to make data-informed decisions, which lead to improved student engagement and academic success."
The implementation of these skills leads to more responsive teaching methods. By integrating analytics into day-to-day practices, educators can design interventions that are specifically suited to address student needs, whether it’s through adjusting content delivery or providing additional support to struggling students.
Tools and Techniques Learned
Tool/Technique | Description |
---|---|
Learning Management Systems (LMS) | Used for collecting and analyzing data on student interactions, assignments, and grades. |
Dashboards and Visualizations | Allow educators to quickly assess student performance and engagement through visual representations of data. |
Advanced Analytics Software | Helps in conducting deeper analysis to predict outcomes and identify patterns within large datasets. |
Understanding the Role of Predictive Analytics in Student Performance
Predictive analytics is rapidly transforming educational settings by offering insights into student performance. By analyzing historical data, these tools can forecast how individual students or entire cohorts might perform in future assessments or courses. This can significantly influence how educators allocate resources, design interventions, and personalize learning experiences. The insights derived from predictive models help institutions respond proactively to student needs, optimizing learning outcomes.
The potential of predictive analytics extends beyond performance prediction. By identifying patterns in behavior, engagement, and academic history, institutions can predict not just grades, but also student retention, dropout risks, and the likelihood of success in specific fields of study. This allows for more precise decision-making and tailored support systems that can make a substantial difference in academic success.
Key Components of Predictive Analytics in Education
- Data Collection - Gathering data from various sources, such as past grades, attendance, participation, and even social engagement, is fundamental to building effective predictive models.
- Algorithm Development - Statistical models and machine learning algorithms are employed to analyze the collected data and identify meaningful patterns that can predict student success or failure.
- Decision Support - The predictions provided by these models assist educators in making data-driven decisions to improve learning environments and interventions.
How Predictive Analytics Impacts Student Support Systems
“By identifying at-risk students early on, predictive analytics allows educators to intervene before challenges become insurmountable, ensuring timely academic and emotional support.”
Predictive analytics systems provide actionable insights that can shape student support strategies. This might include early alerts about students who may struggle in specific subjects or might be at risk of dropping out. These insights help institutions implement preventative measures to improve retention rates and overall academic performance.
Examples of Predictive Analytics Use in Education
- Predicting which students are most likely to fail based on past academic data.
- Assessing the likelihood of a student completing a program based on attendance, engagement, and coursework submission rates.
- Personalizing learning experiences by identifying strengths and weaknesses to offer tailored study paths.
Data-Driven Insights into Student Success
Indicator | Impact on Student Performance |
---|---|
High engagement in class discussions | Predicts higher academic achievement and retention |
Frequent absenteeism | Indicates potential risk of lower grades and dropout |
Timely completion of assignments | Correlates with better exam performance and course completion |
Choosing the Right Learning Analytics Tools for Your Educational Institution
Selecting the appropriate learning analytics platform is crucial for enhancing the educational experience and making data-driven decisions. Educational institutions must carefully consider a range of factors, such as ease of integration, scalability, and the type of data they intend to collect and analyze. With numerous tools available, it is essential to prioritize those that align with both your institution's goals and the needs of the learners.
Before adopting a learning analytics tool, it's important to evaluate the types of insights it provides, such as learner performance, engagement, and progress. These insights should be actionable and directly relevant to improving both teaching practices and student outcomes. Below are some factors to consider when selecting a tool that best fits your educational environment.
Key Considerations for Tool Selection
- Data Integration: Ensure the tool can seamlessly integrate with your existing Learning Management System (LMS) and other educational technologies.
- Customization: The tool should allow you to tailor dashboards and reports to meet specific institutional needs.
- Ease of Use: Prioritize user-friendly interfaces that require minimal training for both educators and administrators.
- Actionable Insights: Look for platforms that provide not just raw data but also interpretative analysis that can lead to informed decisions.
- Scalability: The tool should accommodate the growing demands of your institution as it evolves.
Evaluating Available Tools
When evaluating learning analytics tools, create a shortlist based on the criteria outlined above. Below is a comparison table of some popular platforms that cater to different needs:
Tool | Features | Best For |
---|---|---|
Tool A | Advanced data visualization, integration with LMS | Large institutions with complex data needs |
Tool B | Real-time student tracking, engagement analytics | Smaller institutions focused on student engagement |
Tool C | Predictive analytics, personalized learning insights | Institutions seeking to improve student retention |
"Choosing the right tool can make a significant difference in how effectively you can monitor, interpret, and act on student data."
Practical Applications of Learning Analytics in Personalized Learning
Learning analytics plays a crucial role in transforming the way personalized learning is approached. By collecting and analyzing student data, instructors can gain insights into individual learning patterns, preferences, and progress. This allows educators to create tailored learning experiences that meet the specific needs of each student, fostering a more effective and engaging learning environment.
Personalized learning powered by learning analytics can enhance student outcomes by addressing their unique strengths and weaknesses. By analyzing real-time data, educators can adjust learning paths, provide targeted interventions, and create individualized support structures that promote deeper understanding and mastery of the material.
Key Applications in Personalized Learning
- Adaptive Learning Systems: Learning analytics enables adaptive learning platforms to adjust the content and pace based on the learner's performance. This ensures that students receive the right level of challenge, avoiding frustration or disengagement.
- Targeted Interventions: Through continuous monitoring, teachers can identify students who may need additional support or those excelling in certain areas. This allows for timely interventions, helping students overcome challenges or further enhance their skills.
- Personalized Feedback: By tracking individual performance metrics, educators can provide personalized, actionable feedback, helping students understand their progress and areas needing improvement.
Tools and Techniques Used
- Learning Management Systems (LMS): Platforms like Moodle and Canvas integrate learning analytics to track student engagement and performance, providing insights for customized learning paths.
- Predictive Analytics: Tools that predict student success or failure based on historical data, allowing for early intervention and support.
- Real-time Data Dashboards: Dashboards that display real-time analytics allow both educators and students to monitor progress and adapt their learning strategies accordingly.
Impact on Student Engagement and Performance
Application | Impact on Learning |
---|---|
Adaptive Content Delivery | Ensures students engage with appropriately challenging material, improving retention and satisfaction. |
Targeted Interventions | Helps prevent students from falling behind by providing timely academic support. |
Personalized Learning Paths | Allows students to progress at their own pace, leading to a deeper understanding of the material. |
"By leveraging learning analytics, educators can move away from a one-size-fits-all approach, creating a more student-centered, adaptive learning environment that enhances both engagement and achievement."
Integrating Learning Analytics into Existing Curriculum Design
Integrating learning analytics into an established curriculum requires a strategic approach that blends data insights with instructional goals. This process involves using data collected from students' performance, behaviors, and interactions to optimize teaching methods and course materials. By carefully analyzing these metrics, educators can identify strengths and weaknesses within the curriculum and make informed decisions about improvements.
The primary goal is to enhance the learning experience by adapting the curriculum to meet students' needs more effectively. This requires collaboration between curriculum designers, educators, and data analysts to ensure that insights from learning analytics are applied in a meaningful way without disrupting the existing pedagogical framework.
Steps to Integrate Learning Analytics
- Identify Key Learning Objectives - Define the most important goals of the course and determine how analytics can support these objectives.
- Collect and Analyze Data - Use tools such as Learning Management Systems (LMS) to gather data on student performance and engagement.
- Align Data Insights with Instruction - Review collected data to identify patterns or gaps, then adjust teaching methods and content accordingly.
- Monitor Progress Continuously - Regularly evaluate data throughout the course to ensure ongoing improvements and timely interventions.
- Incorporate Feedback Loops - Create a system where students can provide feedback on the changes made, ensuring that adjustments are effective.
"The successful integration of learning analytics is not about collecting more data, but about using it to make informed decisions that enhance the learning process." - Curriculum Design Expert
Challenges to Consider
- Data Overload - Managing large volumes of data without overcomplicating the process is crucial.
- Student Privacy Concerns - Ensure that data collection respects student privacy and follows relevant regulations.
- Teacher Training - Educators must be adequately trained in both the technology and pedagogical aspects of learning analytics.
Example of Analytics Integration in a Course
Phase | Action | Analytics Utilized |
---|---|---|
Pre-course | Define learning outcomes | Historical performance data |
Mid-course | Adjust instructional methods | Engagement tracking, quiz results |
Post-course | Analyze effectiveness | Final grades, student feedback |
Evaluating the Influence of Learning Analytics on Educational Performance
Learning analytics has become a cornerstone of modern education, enabling institutions to track and assess various factors that impact student success. By collecting data on student behavior, engagement, and progress, educators can make informed decisions aimed at improving outcomes. However, evaluating the specific effects of learning analytics on student performance requires careful measurement and analysis. Institutions are increasingly looking for ways to determine whether these tools lead to tangible improvements in academic achievement, retention rates, and overall student satisfaction.
One of the key challenges in measuring the impact of learning analytics is the diversity of educational contexts and the range of factors influencing student performance. To gain a clearer understanding, data must be gathered systematically and analyzed with attention to the context in which the learning occurs. A variety of metrics can be used, ranging from course completion rates to more granular data on individual learning behaviors. This enables educators to pinpoint areas for intervention and refine their approaches accordingly.
Methods for Assessing Learning Analytics Impact
The impact of learning analytics can be measured through a combination of qualitative and quantitative methods. Some common approaches include:
- Pre- and Post-Implementation Comparisons: Comparing student performance and engagement metrics before and after the introduction of analytics tools.
- Student Feedback: Surveys and interviews with students to assess their perception of how analytics have influenced their learning experiences.
- Longitudinal Studies: Tracking student progress over an extended period to assess the long-term effects of learning analytics interventions.
In addition to these methods, specific metrics often used to evaluate impact include:
Metric | Measure |
---|---|
Completion Rates | Percentage of students successfully completing courses after implementing learning analytics tools. |
Engagement Levels | Tracking how frequently students interact with course materials and their consistency in participating in learning activities. |
Retention Rates | Changes in student retention from one term to the next, correlated with analytics usage. |
"By systematically tracking students' interactions and analyzing performance patterns, educators can not only predict outcomes but also create personalized learning experiences that improve retention and success rates."
Top Obstacles in Learning Analytics and Approaches to Address Them
Learning analytics offers valuable insights into the educational process, enabling institutions to improve learning outcomes. However, the implementation and effective use of analytics come with a series of challenges. Understanding these challenges and devising strategies to address them is crucial for maximizing the benefits of data-driven education.
One of the primary issues in learning analytics is data quality. Without reliable and accurate data, any analysis or decision-making process becomes questionable. Furthermore, the sheer volume of data can be overwhelming, making it difficult to extract meaningful insights. Institutions must find ways to ensure the data is both accurate and manageable for effective use in educational strategies.
Challenges in Learning Analytics
- Data Privacy and Ethics: The collection and use of personal and academic data raise concerns regarding privacy and consent.
- Data Integration: The difficulty of integrating data from diverse sources such as LMS, student information systems, and external platforms.
- Technological Barriers: The need for advanced tools and expertise to analyze and interpret complex data sets.
- Over-Reliance on Quantitative Data: Relying solely on numerical data may overlook qualitative factors that are crucial to student success.
Approaches to Overcome These Challenges
- Enhancing Data Privacy Measures: Institutions should prioritize transparent data governance policies and obtain informed consent to protect student information.
- Streamlining Data Integration: Developing standardized protocols and using interoperable systems can help consolidate data from various sources.
- Investing in Technology and Training: Providing the necessary tools and skills to staff will ensure that data can be analyzed accurately and meaningfully.
- Incorporating Qualitative Data: Supplementing quantitative analysis with qualitative insights, such as student feedback, can provide a more holistic view of learner performance.
"To truly unlock the potential of learning analytics, institutions must address both the technical and ethical challenges that come with data collection and analysis."
Data Challenges in Detail
Challenge | Impact | Solution |
---|---|---|
Data Privacy Concerns | Risk of violating students' privacy rights, leading to legal and ethical issues. | Implement robust data protection policies and ensure clear consent protocols. |
Data Integration | Inability to combine data from different systems, resulting in incomplete analyses. | Develop common data standards and invest in integration tools. |
Technological Limitations | Inadequate tools for data processing, leading to poor decision-making. | Upgrade systems and provide staff training on analytics software. |