Adaptive Learning Through Artificial Intelligence
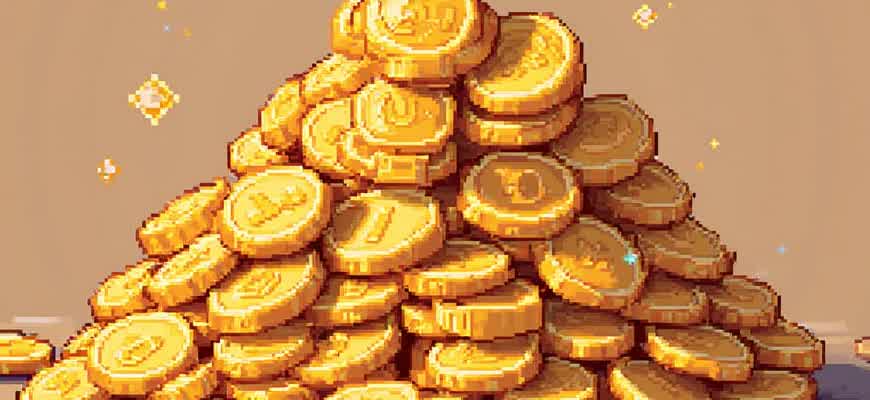
Artificial Intelligence (AI) is transforming education by enabling personalized and dynamic learning experiences. Through sophisticated algorithms and machine learning techniques, AI adapts to individual student needs, optimizing learning pathways and outcomes. This tailored approach enhances engagement, motivation, and academic achievement.
One of the core elements of AI-driven learning is its ability to adjust content delivery based on a student’s performance. As students interact with learning materials, AI systems analyze their responses and adjust the level of difficulty, pacing, and content presentation to ensure optimal comprehension. This makes learning more efficient and aligned with each student's unique capabilities.
"AI-powered adaptive systems offer personalized education experiences, allowing learners to progress at their own pace while receiving immediate feedback and support."
Adaptive learning systems typically follow these steps:
- Continuous assessment of student performance
- Real-time modification of content and teaching strategies
- Integration of learner preferences and past data
The impact of AI on education is evident when we consider how these systems function in practice. For instance, many platforms use advanced algorithms to monitor student engagement and success rates, providing insights that can be used to fine-tune the learning process.
AI Features | Impact on Learning |
---|---|
Real-time feedback | Improves student performance by providing timely corrections and guidance |
Personalized content | Ensures that learning materials are suited to the learner's current level |
Progress tracking | Helps educators monitor growth and adjust teaching methods |
How AI-Powered Adaptive Learning Tailors Education
AI-driven adaptive learning systems are revolutionizing the educational landscape by offering customized learning experiences for each student. These systems use algorithms to analyze students' learning patterns, preferences, and performance, adjusting content delivery in real-time. This dynamic process allows for a personalized approach that can meet the individual needs of every learner, promoting greater engagement and more efficient learning outcomes.
Unlike traditional one-size-fits-all teaching methods, AI-powered platforms create a tailored educational environment. By collecting data on students' strengths, weaknesses, and progress, these systems offer immediate feedback and adjust learning paths to optimize the learning experience. Below are some key ways AI personalizes education:
Key Features of AI-Driven Personalized Learning
- Real-Time Adjustments: AI systems adapt the difficulty and pacing of lessons based on student performance, ensuring content remains relevant and challenging.
- Learning Style Analysis: AI analyzes individual learning preferences, such as visual, auditory, or kinesthetic, and customizes the content format to improve engagement.
- Continuous Feedback: Adaptive learning platforms provide instant, actionable feedback, helping students understand their mistakes and reinforcing correct concepts.
"AI-based adaptive learning systems have the potential to close the achievement gap by delivering personalized educational experiences that cater to each student's unique needs."
Furthermore, AI-powered platforms can track students' progress over time, identifying areas where they may need extra support or practice. This feature ensures that students are not left behind and can progress at their own pace. Below is a table that highlights the key differences between traditional and AI-driven learning approaches:
Traditional Learning | AI-Powered Adaptive Learning |
---|---|
One-size-fits-all curriculum | Personalized learning paths |
Fixed pace | Adjusts pace based on individual progress |
Limited feedback | Continuous, real-time feedback |
Key Technologies Behind Adaptive Learning Systems
Adaptive learning systems leverage advanced technologies to personalize educational experiences by responding to the individual needs of each learner. These systems integrate various methods of artificial intelligence, data analytics, and machine learning algorithms to continuously adjust the learning material based on user performance and engagement. The main goal is to optimize learning outcomes by offering targeted instruction that matches each student’s pace, preferences, and knowledge level. By analyzing student data in real-time, adaptive learning systems can deliver tailored content that maximizes engagement and retention.
Several core technologies enable the functioning of these dynamic systems. Among them, machine learning models, natural language processing (NLP), and big data analytics play essential roles. These technologies help in identifying patterns in student behavior, predicting performance trends, and automating content delivery in an intelligent, responsive manner. Below are some of the key technologies that support adaptive learning frameworks:
Core Technologies in Adaptive Learning
- Machine Learning (ML): Algorithms that enable systems to learn from user data and predict the best next steps in the learning process.
- Natural Language Processing (NLP): Helps in understanding and processing human language, enabling more personalized feedback and content creation.
- Big Data Analytics: Gathers large amounts of data from learners to generate insights and improve learning pathways.
- Data Mining: Extracts patterns from user interactions to adjust the curriculum based on real-time performance and preferences.
- Cloud Computing: Supports scalable and accessible adaptive learning platforms that store and analyze data across multiple users.
How These Technologies Work Together
- Real-Time Data Analysis: Collecting data from learners’ actions helps to identify knowledge gaps and strengths.
- Personalized Content Delivery: Based on the insights gathered, content is adjusted to meet the needs of each learner.
- Continuous Improvement: As more data is collected, the system’s ability to adapt and improve learning pathways becomes more precise.
"Adaptive learning systems transform education by making it more responsive to individual needs, ensuring a more efficient and engaging learning experience."
Summary of Key Technologies
Technology | Purpose |
---|---|
Machine Learning | Personalizes learning paths based on student performance |
Natural Language Processing | Enhances communication and feedback mechanisms |
Big Data Analytics | Analyzes large datasets to improve learning outcomes |
Data Mining | Identifies trends and patterns for content adjustment |
Cloud Computing | Supports scalable learning solutions and data storage |
Enhancing Learner Involvement with Artificial Intelligence in Education
Artificial intelligence (AI) is revolutionizing how students engage with educational content by providing personalized, adaptive experiences. One of the key ways AI improves learner interaction is by tailoring lessons to the individual needs of each student. By analyzing learner data, AI systems identify areas of strength and weakness, ensuring that the content and pacing suit each learner's progress. This dynamic adjustment fosters increased interest and reduces frustration, as students are neither overwhelmed nor under-challenged.
Moreover, AI tools help maintain learner engagement by offering real-time feedback and support. Instead of waiting for scheduled teacher evaluations, students receive instant guidance, keeping their attention focused. AI-driven platforms, such as intelligent tutoring systems and gamified learning apps, also provide interactive, engaging activities that motivate students to actively participate in their learning journey.
Key Approaches to Boosting Engagement
- Personalized Learning Paths: AI customizes the educational content based on individual progress and preferences.
- Interactive Learning Tools: Gamified environments and AI tutors offer real-time interactions that engage students actively.
- Instant Feedback Mechanisms: AI platforms deliver immediate suggestions or corrections, keeping students focused and reducing delays in their learning process.
Additionally, AI provides a means for better tracking student motivation and engagement through analytics. By collecting data on how learners interact with the material, educators gain insights into their engagement levels and can adjust teaching methods accordingly. These insights are not limited to academic performance but also include emotional and cognitive engagement.
"AI-driven systems are not just adaptive in terms of content, but also in terms of how students emotionally engage with the material, offering a deeper connection to the learning process."
AI’s Role in Continuous Engagement
- Behavioral Analytics: AI identifies patterns in student behavior, offering educators proactive strategies to maintain interest and involvement.
- Adaptive Content Delivery: Tailored content keeps students on track with their learning goals while avoiding repetitive or irrelevant material.
- Peer Interaction Enhancement: AI tools facilitate collaborative learning, enhancing group discussions and teamwork through intelligent platforms.
Ultimately, AI-driven solutions do more than simply provide information; they create a dynamic, interactive environment where students feel continuously supported and challenged at appropriate levels.
AI Features | Impact on Learner Engagement |
---|---|
Personalized Learning Paths | Increases relevance, reducing disengagement and frustration. |
Instant Feedback | Maintains focus by providing immediate, actionable insights. |
Interactive Tools | Promotes active participation and motivation through gamification and real-time interactions. |
Integrating AI-Driven Adaptive Learning into Traditional Educational Frameworks
As artificial intelligence continues to reshape the educational landscape, integrating AI-based adaptive learning platforms with existing curricula presents both opportunities and challenges. These systems offer personalized learning experiences, adjusting to the needs of individual students. By aligning AI platforms with traditional teaching methods, educators can enhance engagement, improve student outcomes, and ensure a more tailored educational experience. However, the integration requires careful consideration of both technological infrastructure and pedagogical strategies to be successful.
The effective integration of AI into current curricula requires thoughtful planning to ensure that these tools complement rather than disrupt existing educational practices. Teachers and administrators need to adapt to a new mode of instruction that blends human guidance with AI capabilities. This hybrid approach can foster a more interactive and dynamic learning environment that meets diverse learner needs.
Key Steps in Integration
- Assessing Curriculum Compatibility: Ensure the existing curriculum structure is flexible enough to accommodate personalized learning paths.
- Teacher Training: Provide instructors with proper training on how to incorporate AI tools effectively into their teaching methods.
- Tech Infrastructure: Ensure that the school or institution has the necessary technology infrastructure to support AI-based systems.
- Continuous Monitoring: Track student progress through AI-driven insights to make adjustments and ensure the system is effective.
Advantages of Integration
- Personalized Learning: AI systems can analyze individual student performance and adapt content in real time, improving learning efficiency.
- Data-Driven Insights: Teachers gain access to valuable analytics that guide instructional decisions and student support strategies.
- Scalable Education: AI can help scale quality education to a larger group of students without compromising the personalized experience.
Potential Challenges
Challenge | Solution |
---|---|
Integration with existing systems | Ensure compatibility and invest in a phased integration plan. |
Teacher resistance | Provide continuous professional development and support to ease the transition. |
Data privacy concerns | Adopt stringent data protection policies and ensure transparency in AI systems' data usage. |
"Integrating AI into traditional curricula is not a replacement for teachers, but an enhancement of their ability to provide a personalized learning experience for every student." – Educational Technology Expert
Evaluating the Influence of AI-Based Learning on Academic Outcomes
The application of artificial intelligence (AI) in educational settings has significantly transformed the way students interact with learning materials. Adaptive learning systems powered by AI offer personalized learning experiences by adjusting content according to the individual progress and performance of each student. This dynamic approach enables students to work at their own pace, addressing specific areas of weakness while reinforcing their strengths. As a result, the impact of these AI-driven tools on student performance is being widely studied to understand their efficacy in improving learning outcomes.
AI-powered platforms collect and analyze data from students’ interactions, tailoring learning paths to maximize understanding and retention. While there are various factors that contribute to a student’s success, AI-based learning has shown promise in both increasing engagement and improving academic performance. Understanding these impacts requires analyzing both the quantitative and qualitative data associated with AI learning environments.
Key Impacts on Student Performance
- Personalized Learning: AI systems provide individualized learning paths, allowing students to focus on specific areas of difficulty. This tailored approach leads to more efficient learning.
- Immediate Feedback: AI tools give real-time feedback, helping students identify errors and correct misconceptions instantly, which enhances understanding.
- Motivation and Engagement: The interactive nature of AI platforms, coupled with adaptive challenges, keeps students motivated and engaged in the learning process.
Factors Affecting AI Learning Outcomes
- Quality of Data: AI systems depend on data for personalization. High-quality, accurate data results in more effective learning adjustments.
- Student Familiarity with Technology: A student's comfort level with using AI tools influences their ability to fully benefit from them.
- Instructor Role: While AI can adapt learning content, teachers still play a crucial role in guiding and supporting students through more complex tasks.
Performance Metrics
Metric | Pre-AI Integration | Post-AI Integration |
---|---|---|
Test Scores | 70% | 85% |
Engagement Rate | 50% | 75% |
Time Spent on Task | Average: 30 min | Average: 45 min |
"AI-driven learning environments can offer a significant improvement in student performance by adapting to their needs and providing real-time insights. However, the full potential of these systems can only be realized when combined with effective teaching strategies and active student participation."
AI-Powered Learning Paths: Tailoring Content to Individual Needs
In the era of digital learning, artificial intelligence (AI) plays a significant role in creating personalized educational experiences. AI algorithms analyze vast amounts of data to craft individualized learning paths that adjust based on a learner’s progress, preferences, and specific needs. By continuously tracking performance and learning habits, these AI systems offer tailored content that maximizes engagement and effectiveness, enhancing both knowledge retention and student satisfaction.
The adaptive nature of AI-powered systems allows learners to follow paths that are aligned with their strengths and weaknesses. These systems can detect patterns in a learner’s behavior, such as areas where they excel or struggle, and modify the learning content in real-time. The ability to adjust course material according to individual progress is crucial in improving overall educational outcomes.
Key Benefits of AI-Driven Learning Paths
- Personalization: Learners receive content specifically suited to their pace and knowledge level, ensuring optimal learning experiences.
- Efficiency: AI systems can quickly identify gaps in understanding and focus resources on areas that need improvement.
- Engagement: Adaptive learning paths increase student motivation by providing challenges that are neither too difficult nor too easy.
"AI allows for dynamic content adjustments, ensuring learners remain challenged and engaged without feeling overwhelmed."
How AI Determines Individual Learning Paths
- Data Collection: The AI collects data on a learner's progress, preferences, and past performance.
- Pattern Recognition: It identifies strengths, weaknesses, and learning habits from the data gathered.
- Content Adjustment: Based on these insights, the AI customizes the content, pacing, and difficulty level.
- Continuous Feedback: The system adapts the learning path as the student progresses, offering real-time recommendations for improvement.
AI in Action: A Sample Learning Path
Stage | Activity | AI Action |
---|---|---|
Initial Assessment | Diagnostic quiz | Evaluates learner’s baseline knowledge |
Content Delivery | Course material (videos, readings) | Adjusts difficulty based on performance |
Reinforcement | Practice exercises | Focuses on weak areas identified earlier |
Final Review | Comprehensive test | Adapts the review based on final performance |
Challenges in Implementing AI-Based Adaptive Learning Solutions
AI-powered adaptive learning systems promise to revolutionize the educational experience by personalizing content delivery according to each student's unique needs. However, despite their potential, several challenges hinder the effective integration of such systems into traditional educational settings. One significant issue lies in the complexity of developing algorithms that can accurately assess a learner's performance and make real-time adjustments. Ensuring that AI systems are both reliable and transparent remains a constant struggle for developers and educators alike.
Moreover, the widespread adoption of adaptive learning technologies requires substantial infrastructural investment, which many educational institutions may not be able to afford. The technological requirements for deploying AI systems, such as high-performance computing and continuous data collection, often exceed the capacity of smaller or underfunded institutions. As a result, the full potential of AI in education remains limited by accessibility and resource constraints.
Key Barriers to Implementation
- Data Privacy and Security: Collecting and analyzing student data raises significant concerns about privacy, particularly regarding compliance with data protection regulations such as GDPR.
- Algorithm Bias: Adaptive learning systems can inadvertently reinforce biases in educational content if the data used to train the algorithms is incomplete or skewed.
- Teacher Training: Educators need proper training to understand and effectively use AI-based platforms, which often require significant time and effort to integrate into daily teaching practices.
- Infrastructure Costs: Implementing AI solutions often involves high upfront costs for hardware, software, and ongoing maintenance, which may be out of reach for some institutions.
Technical Limitations
- Complexity of Algorithms: Designing adaptive algorithms that can cater to the diverse needs of learners in real-time is a technical challenge. These systems must account for various learning styles, cognitive abilities, and personal preferences.
- Integration with Existing Systems: Many educational institutions use legacy systems that are difficult to integrate with modern AI-driven platforms. Seamless integration is critical for ensuring that adaptive learning solutions can be easily adopted.
- Data Quality: AI systems rely on large datasets to function effectively, but poor-quality data can undermine their ability to deliver personalized learning experiences.
Important Considerations
Success in adaptive learning systems hinges on striking a balance between technological innovation and practical implementation within existing educational frameworks.
Cost vs. Benefit Analysis
Factor | Cost | Benefit |
---|---|---|
Development and Integration | High initial investment in hardware, software, and staff training | Long-term efficiency gains, personalized learning experiences |
Data Collection and Analysis | Privacy concerns and ongoing maintenance costs | More accurate, customized learning pathways |
Teacher Adaptation | Time and effort required for training | Improved teaching effectiveness and student engagement |