Learning Analytics in Higher Education
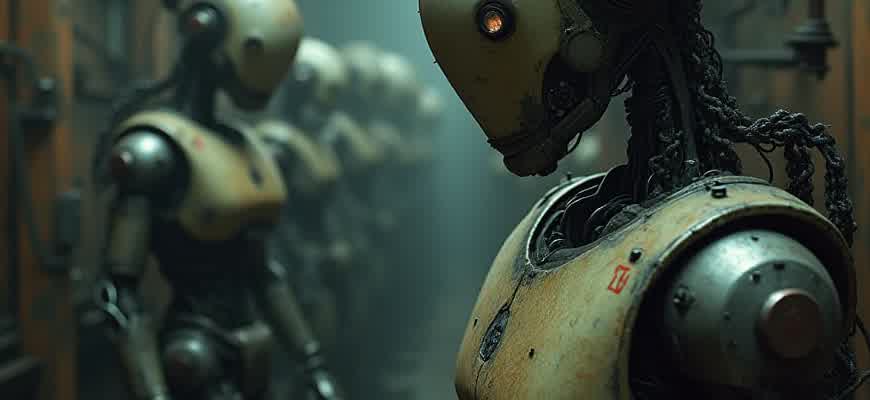
In recent years, higher education institutions have increasingly adopted data analytics tools to better understand student performance and improve educational outcomes. These tools enable universities to collect and analyze vast amounts of data, offering insights into various aspects of the learning process. By leveraging data-driven approaches, educational institutions aim to enhance teaching quality, optimize resources, and support student success.
Learning analytics involves the collection, measurement, analysis, and reporting of data related to student learning behaviors. This process helps educators identify trends, patterns, and areas where students may be struggling, ultimately providing opportunities for timely interventions. The key benefits of implementing analytics in education include:
- Improved student retention and completion rates
- Personalized learning experiences for students
- Data-driven decision-making for educators and administrators
Furthermore, the use of analytics can inform institutional policies and strategies by providing actionable insights into curriculum design and teaching methodologies.
"The effective use of data analytics in education can transform how institutions approach student success, ensuring a more tailored and responsive learning environment."
For universities to successfully implement learning analytics, they must adopt the following strategies:
- Ensure data privacy and ethical considerations are met.
- Provide training for educators to effectively interpret and apply data insights.
- Develop integrated systems that can handle and analyze large datasets efficiently.
These steps are essential for maximizing the potential of analytics and fostering an environment that prioritizes student-centered learning.
Optimizing Course Design Using Data-Driven Insights
Learning analytics provides educators with powerful tools to evaluate the effectiveness of their course designs. By analyzing student data, instructors can identify patterns of engagement, performance, and challenges, which can be used to fine-tune and optimize course structures. The process of aligning course content, assessments, and teaching strategies with real-time data helps improve student outcomes and fosters a more personalized learning experience.
One key benefit of incorporating learning analytics into course design is the ability to make data-informed adjustments. This allows instructors to intervene early when students show signs of struggle, offering tailored support or revising content delivery strategies. The continuous feedback loop created by analytics promotes an agile approach to course management, where instructors can constantly evolve their teaching practices based on actual student data.
Key Strategies for Optimizing Course Design
- Monitor student participation and identify at-risk students based on engagement metrics.
- Adjust course content delivery, such as pacing or medium (e.g., video, reading materials), based on student interaction data.
- Use assessment analytics to understand which learning objectives are being met and where students may be struggling.
Table 1: Course Optimization Metrics
Metric | Goal | Data Source |
---|---|---|
Engagement Rate | Increase student participation and interaction | Course platform analytics (e.g., logins, forum activity) |
Assessment Performance | Improve understanding of core concepts | Assignment and exam results |
Completion Rate | Ensure timely course completion | Course progress tracking |
"Learning analytics enables a proactive approach to course design, helping instructors deliver more effective and responsive learning experiences." – John Doe, Educator
Benefits of Data-Informed Adjustments
- Enhances the alignment of course content with student needs.
- Improves retention rates by addressing challenges early in the learning process.
- Allows for the efficient use of resources, optimizing both teaching time and learning materials.
Improving Student Retention Rates Through Data-Driven Insights
In higher education, student retention remains a critical concern for institutions striving to ensure academic success and institutional sustainability. By leveraging data analytics, universities can gain valuable insights into student behaviors, performance trends, and at-risk factors that contribute to student dropout rates. These insights can help develop targeted strategies to enhance retention and foster a more supportive learning environment.
Data-driven approaches allow institutions to identify patterns early, providing an opportunity to intervene proactively. With the right tools and frameworks, universities can predict student disengagement, academic struggles, and personal challenges. In this way, retention efforts can be tailored specifically to the needs of individual students, increasing the likelihood of successful academic outcomes.
Key Insights for Improving Retention Rates
- Identifying early indicators of at-risk students based on performance data.
- Analyzing learning behaviors to personalize student support services.
- Using predictive analytics to track engagement and intervene in real time.
- Enhancing communication channels between faculty, advisors, and students.
Actionable Steps for Institutions
- Implement early-warning systems to detect struggling students.
- Provide personalized academic advising based on student data.
- Offer targeted interventions, such as tutoring and counseling, for identified at-risk groups.
- Monitor and evaluate the effectiveness of retention strategies regularly.
Quote: "By applying data insights, institutions can transform how they support students, improving both their academic journey and the likelihood of graduation." - Education Data Expert
Example of Retention Data Metrics
Metric | Description | Impact on Retention |
---|---|---|
Attendance Rates | Frequency of student participation in classes | Low attendance correlates with higher dropout rates |
Assignment Submission | Timeliness and quality of assignment submission | Delays in submission may signal disengagement |
Grades and Test Scores | Academic performance metrics | Below-average performance is a strong predictor of risk |
How Learning Analytics Improves Personalized Learning Journeys
Learning analytics plays a crucial role in shaping personalized learning experiences by offering insights into student behaviors, learning patterns, and performance. By collecting data from various student interactions with digital tools, platforms, and resources, it enables educators to tailor educational content and support in real-time. This approach helps to create individualized pathways that optimize student success, ensuring that each learner receives the right resources at the right time based on their unique needs and progress.
As a result, personalized learning paths are dynamically adjusted to meet students where they are in their educational journey. By utilizing predictive models and performance tracking, learning analytics facilitates the continuous evolution of these paths, improving engagement and overall learning outcomes. Below are key ways in which learning analytics enhances this process:
- Real-Time Data Analysis: Learning analytics processes data from various sources, including assessments, assignments, and engagement metrics, to adapt the learning experience on-the-fly.
- Identifying Learning Gaps: By continuously monitoring performance, educators can quickly identify areas where students are struggling and provide targeted interventions.
- Customized Resource Allocation: Analytics allow the distribution of personalized resources and activities, ensuring each student gets what they need to succeed.
“With learning analytics, personalized learning isn’t a static goal. It’s an ongoing process, one that evolves based on student interaction, needs, and engagement.”
To understand how this works in practice, consider the following example:
Student | Performance | Suggested Resources |
---|---|---|
John | Weak in mathematics | Interactive exercises, remedial videos, practice tests |
Alice | Strong in English, needs challenge | Advanced readings, essay-writing workshops |
Through continuous feedback and adaptive strategies, learning analytics empowers educators to design more precise, student-centered learning experiences, leading to improved academic outcomes.
Identifying Students at Risk with Learning Analytics Tools
Learning analytics tools have become essential for early identification of students who might be struggling academically. These tools allow educators to track various indicators, such as engagement, participation, grades, and behavior, to detect potential risks before they become critical issues. By analyzing large volumes of student data, institutions can offer timely interventions, ensuring better retention rates and academic success.
At-risk students may exhibit several patterns, such as low engagement in online platforms, decreased attendance, or poor assignment performance. Learning analytics systems can monitor these behaviors in real time, providing educators with the necessary insights to offer support. This data-driven approach helps to personalize the learning experience and focus on areas where students need the most help.
Key Indicators for Identifying At-Risk Students
- Low interaction with course materials or classmates
- Frequent late submissions or missed deadlines
- Declining grades or lack of improvement over time
- Minimal participation in class discussions or activities
Intervention Strategies Based on Analytics
- Personalized Communication: Reaching out to students with targeted support based on their specific struggles.
- Additional Tutoring or Counseling: Offering resources like extra tutoring sessions or counseling to address non-academic challenges.
- Behavioral Analytics Alerts: Setting up automatic alerts when a student shows signs of disengagement, such as prolonged inactivity.
Learning analytics can significantly improve outcomes by enabling proactive intervention. By identifying at-risk students early, institutions can reduce drop-out rates and enhance academic performance.
Example of a Data Monitoring Table
Student | Attendance Rate | Grades | Engagement Level | Intervention Needed |
---|---|---|---|---|
John Doe | 60% | 55% | Low | Yes |
Jane Smith | 80% | 70% | Medium | No |
Mark Lee | 40% | 45% | Very Low | Yes |
Using Learning Analytics to Enhance Faculty Teaching Methods
Learning analytics offers valuable insights that can transform how instructors approach teaching in higher education. By analyzing data from student engagement, performance, and behavior, faculty members can adjust their teaching strategies to meet individual learning needs and improve overall educational outcomes. This data-driven approach allows educators to identify areas where students may struggle, providing an opportunity for targeted intervention and support.
Faculty can use the findings from learning analytics to refine their teaching techniques, optimize course materials, and personalize learning experiences. The ability to track patterns over time enables instructors to make informed decisions about how to enhance the learning environment and foster better student outcomes.
Benefits of Using Learning Analytics in Teaching
- Personalized Learning: Data helps faculty identify students’ strengths and weaknesses, enabling tailored support for diverse learning needs.
- Real-time Feedback: Instructors receive immediate insights on student progress, allowing them to adjust their teaching methods during the course.
- Informed Curriculum Design: Learning analytics provide a data-driven approach to modify the curriculum to align better with students' learning patterns.
- Increased Student Engagement: By identifying engagement trends, faculty can implement strategies to keep students motivated and involved in the learning process.
Practical Applications of Learning Analytics
- Predictive Analytics: Using historical data to predict which students are at risk of underperforming, allowing early intervention strategies.
- Adaptive Learning: Implementing technologies that adjust course materials based on the learning pace and needs of individual students.
- Assessment and Feedback Analysis: Analyzing assessment results to identify common misconceptions or areas where teaching methods may need adjustment.
Key Areas to Focus On
Area of Focus | Impact on Teaching |
---|---|
Student Engagement | Helps track how actively students are participating in the course, allowing faculty to adjust their approach to improve interaction. |
Performance Analytics | Enables instructors to identify learning gaps and provide timely interventions for students falling behind. |
Content Effectiveness | Data reveals which learning materials resonate with students and which need refinement or replacement. |
"By incorporating data from learning analytics into their teaching strategies, educators can create more adaptive, responsive, and effective learning environments that support all students."
Incorporating Data-Driven Insights into Educational Leadership
Incorporating learning analytics into decision-making processes at higher education institutions can significantly improve both the quality and efficiency of educational practices. By utilizing real-time data on student performance, behavior, and engagement, administrators can make more informed, data-backed decisions that align with institutional goals. This process includes identifying key metrics, developing actionable insights, and integrating findings into broader strategies for academic improvement, resource allocation, and policy development.
Effective integration of learning analytics requires collaboration between data specialists, faculty, and leadership teams. It’s essential that the collected data be accurate, timely, and easily interpretable to provide a clear picture of institutional needs. Properly leveraging analytics can inform decisions regarding curriculum adjustments, student support services, and staff training, ultimately leading to enhanced student success and institutional growth.
Key Areas of Decision-Making Informed by Learning Analytics
- Curriculum Design and Enhancement - Analytics can highlight which topics or teaching methods engage students most effectively, guiding curriculum development to better meet student needs.
- Student Retention and Support - Data helps identify students at risk of underperforming, allowing for timely interventions through targeted support services.
- Resource Allocation - Insights into class attendance patterns, study behaviors, and performance can inform better allocation of resources such as faculty time, learning tools, and tutoring services.
Examples of Data Utilization in Institutional Decision-Making
Learning analytics enables institutions to proactively adjust their strategies rather than reacting to issues after they arise. It allows for the identification of patterns such as student disengagement or falling grades early on, ensuring that corrective measures can be put in place swiftly.
- Using predictive analytics to forecast student performance and implement support systems before students face academic failure.
- Integrating faculty feedback with student learning data to optimize course content and teaching methods.
- Adapting institutional strategies based on the success rates of different teaching models, improving long-term academic outcomes.
Challenges and Considerations
Challenge | Consideration |
---|---|
Data Privacy | Ensure that all student data is protected and used in compliance with relevant privacy laws. |
Data Interpretation | Proper training for staff to interpret data accurately and avoid misinformed decisions. |
Integration with Existing Systems | Seamlessly integrate learning analytics tools with current academic and administrative platforms. |
Challenges of Implementing Learning Analytics in Universities
The application of learning analytics in higher education is often hindered by technical and operational issues. Universities frequently struggle with the complexity of data management. Different departments often store student data in isolated systems, making it difficult to aggregate and analyze data across the institution. This lack of centralized data structures leads to inefficiencies and can result in incomplete or misleading conclusions. Furthermore, the diverse nature of student learning pathways, including varying levels of engagement and performance metrics, makes it challenging to create analytics tools that accurately reflect the needs of all students.
Additionally, ethical concerns and data privacy issues present significant obstacles. The collection and use of personal student data can raise questions about security and consent. Universities must navigate complex regulations to ensure they are compliant with privacy laws, such as GDPR, while also addressing the concerns of students and staff about how their data is being used. Striking a balance between leveraging data for institutional benefit and maintaining individual privacy is one of the key challenges in adopting learning analytics.
Key Barriers to Effective Learning Analytics Implementation
- Fragmented Data Systems: Disparate data sources across multiple platforms can complicate efforts to gather meaningful insights from student data.
- Faculty and Staff Resistance: There may be a reluctance to adopt learning analytics due to fears of increased workload or challenges with integrating these tools into existing practices.
- Lack of Technical Expertise: Insufficient technical infrastructure or expertise can impede the development and deployment of learning analytics systems.
For instance: Schools with outdated IT infrastructure may face difficulties in handling the large volumes of data required for real-time analytics, while some faculty members may feel that learning analytics tools undermine their academic autonomy.
Successful implementation requires a collaborative approach, where stakeholders from various departments work together to ensure that technology, data management, and ethical standards are aligned.
Strategies to Address These Challenges
- Develop integrated data platforms that streamline data collection across all university systems.
- Provide professional development and training programs to help faculty and staff understand the value and use of learning analytics.
- Implement clear data governance policies to ensure the ethical handling of student information.
Challenge | Suggested Solution |
---|---|
Fragmented Data | Create a centralized data repository to unify student data across departments. |
Data Privacy Concerns | Establish robust data privacy frameworks and ensure transparent consent processes. |
Faculty Resistance | Offer incentives and training to demonstrate the benefits of analytics in teaching and learning. |
Ensuring Data Privacy and Security in Learning Analytics Systems
In the context of learning analytics, data privacy and security are critical concerns. As educational institutions increasingly adopt data-driven approaches to improve student outcomes, it is essential to ensure that sensitive student information is safeguarded against unauthorized access or misuse. This is particularly challenging as large volumes of data are collected and analyzed, which includes not only academic performance but also behavioral and personal data.
To address these concerns, educational institutions must adopt robust measures that prioritize the protection of student privacy while enabling effective data analysis. Key strategies for ensuring data security in learning analytics systems include encryption, access control mechanisms, and regular audits of data usage. By implementing these practices, institutions can mitigate the risks associated with data breaches and comply with privacy regulations, such as GDPR or FERPA.
Key Practices for Data Privacy and Security
- Data Encryption: Ensuring that sensitive data is encrypted both in transit and at rest helps protect it from unauthorized access.
- Access Control: Limiting access to data based on roles ensures that only authorized personnel can view or manipulate sensitive information.
- Regular Audits: Conducting frequent audits of data usage and access helps identify and address potential security gaps before they can be exploited.
Important Consideration: Effective data security measures must also address the following aspects:
- Data Anonymization: Anonymizing personal information in datasets reduces the risk of privacy breaches while still allowing for valuable insights to be drawn.
- Legal Compliance: Ensuring adherence to relevant privacy laws and regulations, such as the GDPR in Europe or FERPA in the U.S., is essential for protecting student data.
- Stakeholder Transparency: Informing students and faculty about how their data is being collected, used, and protected fosters trust and collaboration in the process.
Best Practice Example: The University of X implemented a comprehensive encryption system for student data, alongside access controls that ensure only authorized users can access sensitive information. This initiative has significantly improved data security and helped meet regulatory compliance requirements.
Security Measure | Description |
---|---|
Encryption | Ensures that all sensitive data is protected, even if intercepted. |
Access Control | Limits access to data based on user roles, minimizing the risk of unauthorized access. |
Audits | Regular checks to ensure that data usage complies with privacy standards. |