Blended Learning Data
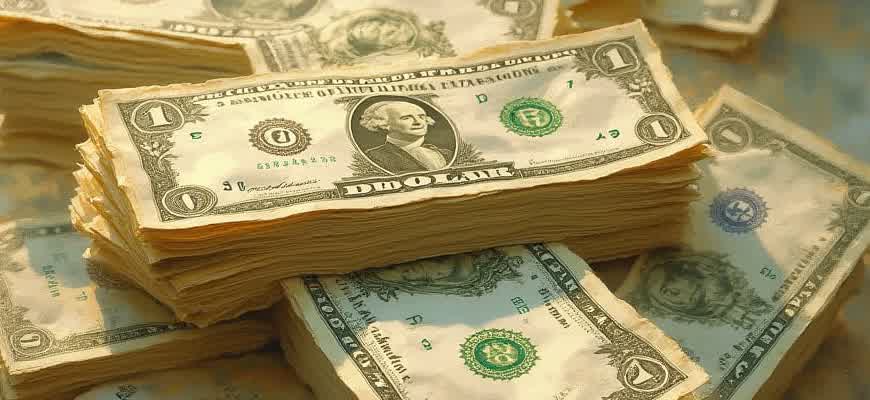
Blended learning is an educational approach that combines traditional classroom methods with online instruction. This integration of physical and virtual learning environments generates a wealth of data that can be analyzed to improve teaching and learning outcomes. Various types of data are collected, including student engagement, performance metrics, and content interaction. Understanding these data points is critical for adapting teaching strategies to meet the needs of learners.
Types of Data Collected in Blended Learning
- Student interaction with online content
- Assessment results (quizzes, exams, assignments)
- Time spent on learning materials
- Frequency and quality of participation in class discussions
Key Insights from Blended Learning Data
Data analysis in blended learning helps identify patterns in student performance, enabling educators to tailor instruction to individual needs and preferences.
Data Visualization: Performance Trends
Student Name | Average Score (%) | Online Interaction Time (hrs) |
---|---|---|
John Doe | 85 | 12 |
Jane Smith | 90 | 15 |
Tom Brown | 78 | 10 |
Analyzing Student Performance Data in Blended Learning Environments
In blended learning models, student performance data provides valuable insights into how learners engage with both online and in-person components. Analyzing this data allows educators to tailor their teaching strategies, identify areas for improvement, and make data-driven decisions that enhance learning outcomes. Blended environments, which combine traditional face-to-face instruction with digital tools, require continuous assessment of how students interact with different types of learning materials and platforms.
Data analysis in this context focuses on several key aspects, such as engagement levels, academic progress, and the effectiveness of various learning modalities. By tracking metrics like online quiz results, time spent on digital platforms, and participation in group activities, educators can create a comprehensive view of each student's performance. This allows for a more personalized approach to teaching that adapts to individual needs.
Key Metrics in Blended Learning Data Analysis
- Engagement Data: Interaction with digital content, such as videos, assignments, and discussion boards.
- Academic Performance: Quiz scores, project grades, and assignment completion rates.
- Time Management: Time spent on online activities versus traditional classroom time.
"Effective use of data analytics in blended learning environments helps instructors pinpoint struggling students and offer targeted interventions."
Approaches to Analyzing Performance Data
- Quantitative Analysis: Involves reviewing scores, completion rates, and time spent on tasks. This type of data is often presented in tables or charts for easier interpretation.
- Qualitative Analysis: Focuses on feedback, such as student surveys, course evaluations, and instructor observations. This provides context to the numerical data.
- Mixed-Methods Analysis: Combines both quantitative and qualitative data to give a more complete picture of student progress.
Performance Data Example
Student | Online Participation | Quiz Scores | In-Class Participation |
---|---|---|---|
John Doe | 75% | 85% | 90% |
Jane Smith | 60% | 70% | 80% |
Mark Lee | 90% | 95% | 85% |
Using Learning Analytics to Optimize Blended Learning Strategies
Incorporating learning analytics into blended learning environments provides actionable insights into student behavior, engagement, and performance. By continuously monitoring digital interactions and classroom activities, educators can better understand how students are interacting with content, and where additional support or adjustments may be necessary. This data-driven approach enables institutions to make informed decisions that enhance both the online and in-person components of the blended learning experience.
Learning analytics tools enable the tracking of various metrics such as time spent on digital platforms, participation rates, and assessment outcomes. These metrics can be used to refine the curriculum, personalize learning paths, and identify students who may need additional interventions. With the right analytics infrastructure, educational institutions can ensure a more adaptive and responsive learning environment.
Key Metrics for Optimizing Blended Learning
- Engagement Rates: Tracking student interaction with digital content and discussions.
- Assessment Performance: Analyzing how well students are performing in both online and face-to-face assessments.
- Time Spent: Monitoring how much time students spend on various learning activities.
Benefits of Data-Driven Adjustments
Learning analytics provide educators with concrete data that can be used to enhance course design, offering timely interventions to prevent student disengagement.
- Informed decision-making regarding content pacing and delivery.
- Personalized learning pathways for students who require additional support or more advanced content.
- Improved student retention through proactive interventions based on early warning signs.
Example of Learning Analytics Implementation
Metric | Actionable Insight |
---|---|
Low engagement with video content | Adjust video length or add interactive elements to increase student interaction. |
Declining quiz scores | Provide targeted review materials or additional practice problems for students. |
Implementing Data-Driven Decisions to Personalize Learning Experiences
In modern education, the integration of data-driven insights into the teaching process allows for more tailored and efficient learning pathways. By continuously analyzing learner performance, instructors can adjust lesson plans and teaching methods to meet the individual needs of students. This approach moves away from the one-size-fits-all model, offering a more flexible and dynamic learning experience.
The application of data not only aids in identifying areas where students may be struggling but also highlights strengths that can be further nurtured. Personalized learning paths allow educators to make informed decisions, ensuring that students receive the right support at the right time. This can be particularly effective in blended learning environments, where both digital tools and traditional teaching methods are utilized to enhance learning outcomes.
Key Steps in Implementing Data-Driven Personalization
- Gathering Data: Collect data from a variety of sources such as assessments, online interactions, and participation in classroom activities.
- Analyzing Student Performance: Use tools to analyze trends and patterns in students' learning progress, identifying both strengths and weaknesses.
- Adapting Instruction: Modify teaching strategies and learning materials based on data to address individual student needs.
Examples of Data-Driven Personalization in Action
- Tracking student progress through formative assessments and using results to adjust instructional content.
- Using learning management systems to deliver targeted lessons and exercises based on performance data.
- Offering personalized feedback through data analysis, guiding students on how to improve specific skills.
"Data-driven personalization enables educators to provide tailored instruction, fostering a more engaging and effective learning experience."
Benefits of Data-Driven Personalization
Benefit | Description |
---|---|
Improved Engagement | Students are more likely to stay engaged when learning is customized to their needs and preferences. |
Targeted Interventions | Data helps identify students who need additional support, allowing timely interventions. |
Increased Retention | Personalized learning pathways improve long-term retention by adapting to students' learning speeds. |
Ensuring Data Security and Privacy in Blended Learning Models
In blended learning environments, where both online and offline educational components are integrated, ensuring data security and privacy is critical. As the model relies on various digital platforms, large volumes of personal and academic data are generated, creating new risks that need to be effectively managed. Protecting sensitive student information from unauthorized access or breaches requires robust security measures at both the institutional and technological levels.
Educational institutions must take a proactive approach to safeguard the data that is shared, stored, and processed during blended learning activities. This includes implementing secure platforms, using encryption technologies, and establishing strict access controls. Data protection policies should be clearly outlined, and stakeholders must be educated on the importance of maintaining privacy in such learning ecosystems.
Key Strategies for Ensuring Data Protection
- Data Encryption: Ensures that all sensitive data, whether at rest or in transit, is encoded and unreadable to unauthorized parties.
- Access Control: Implements role-based access to restrict sensitive data access only to authorized personnel.
- Data Minimization: Collects only the necessary information from students, avoiding the storage of unnecessary personal data.
- Regular Audits: Conducts routine security audits and vulnerability assessments to identify and fix potential weaknesses in the system.
“Protecting student privacy in digital learning environments is not just a legal requirement, it’s a fundamental responsibility to foster trust and secure the educational process.”
Privacy Protection Measures for Blended Learning Tools
To further enhance privacy protection, blended learning tools must adhere to specific guidelines and standards. Below are the key recommendations for securing these platforms:
Security Measure | Description |
---|---|
Data Encryption | Ensure that all data transferred between devices and servers is encrypted using robust encryption protocols. |
Secure Login Protocols | Implement multi-factor authentication (MFA) to prevent unauthorized access to student accounts. |
Compliance with Regulations | Ensure adherence to global privacy regulations such as GDPR, FERPA, or CCPA, depending on geographical location. |
“Strict compliance with privacy regulations is crucial not only to avoid legal repercussions but also to maintain the trust of students and educators alike.”
Measuring the ROI of Blended Learning Solutions through Data Analysis
As organizations increasingly adopt blended learning models, evaluating the return on investment (ROI) of these solutions becomes a crucial task. The integration of both traditional classroom and digital learning experiences presents unique opportunities for data-driven assessments. By leveraging data analysis, institutions can gain valuable insights into the effectiveness and cost-efficiency of blended learning programs. This enables better decision-making, resource allocation, and overall strategy refinement.
Effective measurement of ROI requires a comprehensive approach that incorporates both quantitative and qualitative data. Organizations must analyze key performance indicators (KPIs), learner engagement metrics, and long-term business outcomes. This data-driven evaluation helps identify areas for improvement, optimize learning strategies, and ensure that the investments in blended learning yield the desired benefits.
Key Metrics for ROI Evaluation
- Cost Savings: Track reductions in travel, infrastructure, and instructor costs through virtual learning platforms.
- Learning Outcomes: Measure improvements in knowledge retention, skill acquisition, and learner performance.
- Engagement Levels: Analyze student participation rates, course completion rates, and feedback on learning experiences.
- Time Efficiency: Evaluate the reduction in time spent on training due to the flexibility of blended learning formats.
Data Analysis Tools and Techniques
To effectively measure ROI, organizations can utilize various data analysis techniques and tools, including:
- Learning Management Systems (LMS): Track student progress, engagement, and completion rates through built-in reporting features.
- Surveys and Feedback: Collect learner and instructor feedback on the perceived effectiveness of the blended learning approach.
- Performance Analytics: Use predictive analytics to assess learner performance and identify trends over time.
"Data-driven decisions enable organizations to refine their blended learning approaches, ensuring that both cost and educational outcomes are optimized for maximum impact."
Example ROI Calculation
Metric | Value |
---|---|
Cost of Traditional Training | $100,000 |
Cost of Blended Learning | $60,000 |
Learning Outcome Improvement | 20% |
ROI | 67% (calculated based on cost savings and learning outcomes) |