Disadvantages of Adaptive Learning
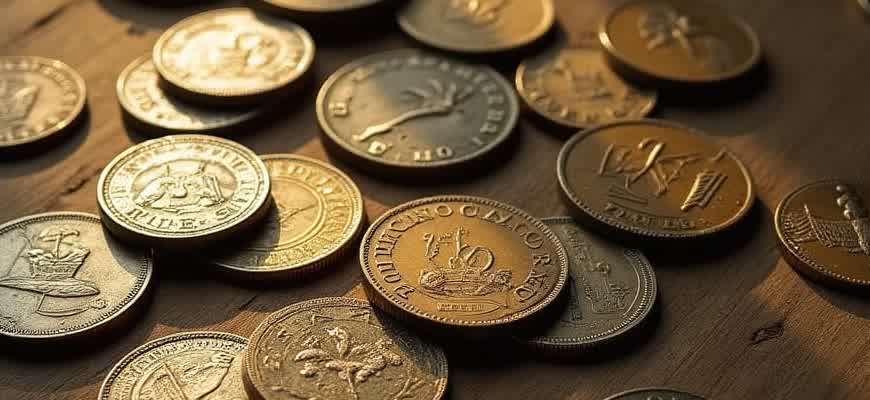
While adaptive learning offers several advantages, it also presents notable challenges that may hinder its effectiveness. One of the key issues is the reliance on technology, which can result in accessibility problems for some users. Not all students have equal access to the devices or stable internet connections necessary to engage with adaptive learning platforms effectively. This digital divide can exacerbate existing inequalities in education.
Another disadvantage is the potential for oversimplification in learning. Adaptive systems often focus on delivering personalized content based on predefined algorithms, which can sometimes lead to a narrow scope of learning materials. This may limit students' exposure to diverse perspectives and critical thinking opportunities.
“Adaptive learning systems can inadvertently narrow a student's learning experience, reinforcing only the content that the algorithm determines to be relevant, which may not always foster a deeper understanding of complex subjects.”
Moreover, while these platforms aim to provide a customized learning path, they may fail to accurately assess the full range of a student's abilities. Errors in the system's analysis could lead to students receiving inappropriate content levels, either too easy or too difficult, undermining the learning process.
- Access issues: Not every student has the required technology or internet access.
- Content limitations: Narrow focus can reduce the diversity of learning materials.
- Incorrect assessments: Algorithmic errors may result in unsuitable learning content.
The following table highlights these challenges:
Disadvantage | Impact |
---|---|
Access issues | Limited engagement due to lack of necessary technology or connectivity. |
Content limitations | Reduced exposure to diverse materials and perspectives. |
Incorrect assessments | Inaccurate difficulty levels can hinder progress or cause frustration. |
High Initial Setup Costs for Adaptive Learning Systems
Implementing adaptive learning technologies requires a significant upfront investment. Schools, universities, and companies must often pay high costs for the necessary infrastructure, software, and training. These expenses can make the transition to adaptive learning systems challenging for organizations with limited budgets. In addition to purchasing the software and hardware, organizations may also need to hire specialists to customize and integrate the system into existing educational frameworks.
Moreover, the complexity of these systems adds to the costs. Adaptive learning platforms typically require advanced algorithms, data analytics, and continuous updates to remain effective. This can result in substantial ongoing expenses for software maintenance and content development. In the long run, the total cost of ownership may surpass initial expectations, especially if the system is not fully optimized from the outset.
Key Factors Contributing to High Setup Costs
- Technology Infrastructure: High-performance servers, storage solutions, and cloud-based systems are often required to handle the extensive data processing.
- Software Development and Licensing: Custom software solutions, or licenses for existing platforms, can be costly. Many adaptive systems are tailored to the specific needs of the institution.
- Training and Support: Employees must be trained to use the system effectively, and ongoing technical support may be necessary for smooth implementation.
Example of Typical Initial Expenses
Item | Estimated Cost |
---|---|
Software Licensing | $20,000 - $100,000 |
Hardware Infrastructure | $50,000 - $200,000 |
Training Programs | $5,000 - $25,000 |
"While adaptive learning systems offer many long-term benefits, the initial investment is often prohibitive for smaller institutions or businesses without dedicated funding."
Challenges in Data Privacy and Security in Adaptive Platforms
Adaptive learning platforms rely on gathering large amounts of personal and educational data to tailor content and optimize learning experiences. However, this dependency raises significant concerns regarding the protection of sensitive user information. The data generated from user interactions, such as behavioral patterns and performance metrics, can be exploited if not properly secured, leading to privacy breaches or misuse by unauthorized entities.
Ensuring data privacy and security within adaptive learning systems is particularly complex due to the vast scope of data collected, its continuous evolution, and the various stakeholders involved. As these platforms often involve third-party service providers for storage and analysis, maintaining control over sensitive data becomes even more challenging. Furthermore, the integration of AI and machine learning algorithms introduces potential vulnerabilities that can be exploited if not carefully managed.
Potential Risks and Security Gaps
- Unauthorized data access by third parties or hackers.
- Inadequate data anonymization, leading to the exposure of personal details.
- Security lapses in the use of cloud-based storage and data processing systems.
- Difficulty in ensuring compliance with privacy regulations (e.g., GDPR, CCPA).
Mitigation Strategies
- Implementing end-to-end encryption to safeguard data in transit and at rest.
- Adopting strong authentication methods to restrict access to sensitive information.
- Regular security audits and vulnerability assessments of the platform.
- Ensuring transparency with users about data usage and obtaining informed consent.
Important: While adaptive platforms offer personalized learning experiences, their success hinges on the ability to secure and protect user data. Any lapses in data security could significantly damage trust in these technologies.
Data Privacy Concerns in Adaptive Learning
Data Type | Privacy Risks | Potential Impact |
---|---|---|
Personal Identifiable Information (PII) | Exposure due to insufficient encryption | Identity theft, unauthorized access to personal accounts |
Learning Behavior Data | Misuse or sale of behavioral data without consent | Exploitation for targeted advertising or manipulative practices |
Performance Data | Leakage of grades or test results | Damaged academic reputation or discrimination in future opportunities |
Overreliance on Algorithms: Can Machines Replace Human Judgment?
In recent years, the increasing integration of algorithms in adaptive learning systems has sparked concerns regarding their ability to replicate human judgment. While these systems are capable of processing vast amounts of data and providing personalized recommendations, they are limited by the boundaries of their programming and underlying models. This raises a fundamental question: Can machines truly replace the nuances of human decision-making, particularly in complex learning environments?
Algorithms in adaptive learning rely heavily on patterns derived from data to make decisions. However, unlike humans, machines lack the ability to understand context, emotions, or ethical considerations that often guide human choices. This can lead to overgeneralizations or inappropriate recommendations, especially when the data input is incomplete or biased.
Limitations of Algorithms in Adaptive Learning
- Contextual understanding: Algorithms are not capable of fully grasping the subtleties of each learner's unique circumstances, such as emotional state or specific educational needs.
- Ethical considerations: Machines cannot assess ethical implications or make morally grounded decisions, something human judgment excels at.
- Bias in data: If the input data is skewed or incomplete, the machine’s decision-making may reinforce stereotypes or perpetuate inequality.
While algorithms excel at processing large datasets, they are incapable of replicating the depth of human empathy and ethical reasoning that are essential in educational settings.
Potential Risks of Algorithm Overreliance
- Reduced critical thinking: Excessive dependence on algorithmic recommendations may discourage learners from developing independent problem-solving and critical thinking skills.
- Loss of human oversight: Without human involvement, machines may make decisions that overlook important contextual factors, potentially leading to negative educational outcomes.
- Data privacy concerns: The extensive use of algorithms in adaptive learning systems requires large amounts of personal data, raising issues related to privacy and security.
Comparison of Human Judgment vs. Algorithmic Decision-Making
Aspect | Human Judgment | Algorithmic Decision-Making |
---|---|---|
Context Awareness | Highly contextual, considers emotions, experiences, and ethical implications | Lacks depth in understanding complex, emotional, or ethical contexts |
Adaptability | Highly adaptable, capable of adjusting to new information and changing conditions | Limited to pre-programmed algorithms and existing data sets |
Bias | Prone to personal biases but capable of critical reflection | Can perpetuate biases if data is flawed or skewed |
Limited Content Availability for Personalized Learning Paths
One of the primary drawbacks of adaptive learning systems is the limited availability of content tailored to specific learning needs. While adaptive learning platforms aim to adjust the learning experience based on individual progress, the effectiveness of this approach is often constrained by the resources available. When content is not sufficiently diverse or comprehensive, it can limit the adaptability of the learning path, leaving students without adequate materials to fully engage with the topic.
For personalized learning to be truly effective, the content must not only cover a broad range of subjects but also be tailored to the student's learning pace and style. However, in many cases, the content available on adaptive learning platforms is limited, reducing the potential for a fully customized educational experience.
The lack of diverse content options prevents adaptive learning systems from reaching their full potential in personalization.
- Content is often restricted to certain topics or formats, limiting personalization.
- Insufficient variety in learning materials can hinder the development of a truly individualized path.
- Adaptive learning systems may not always offer content that aligns well with the specific needs or preferences of every learner.
In some cases, the constraints of content availability can also affect the accuracy of adaptive learning systems in assessing a learner’s needs. As the system is only able to work with the available content, it may overlook certain knowledge gaps or fail to provide alternative resources to better suit the learner's profile.
Advantages of Adaptive Learning | Disadvantages due to Limited Content |
---|---|
Customization of learning experience based on individual progress. | Limited scope of personalized learning paths due to content restrictions. |
Improved learning efficiency through tailored materials. | Risk of content redundancy and gaps in knowledge. |
Dynamic adjustments to teaching methods and content. | Missed opportunities for deeper engagement and exploration of topics. |
Potential Bias in Adaptive Learning Algorithms
Adaptive learning systems rely heavily on algorithms that adjust content and pacing according to a learner's performance. While these systems are designed to offer personalized educational experiences, they may inadvertently introduce bias, leading to skewed learning outcomes. These biases typically arise from the data used to train the algorithms, the design choices made by developers, and the inherent limitations in how these systems assess learner performance. Over time, this can perpetuate or even amplify existing inequalities in educational contexts.
The risk of bias in adaptive learning algorithms is particularly concerning because it can affect different groups of students in varying ways. For example, if the data used to train the system is not diverse enough, the algorithm may favor the learning patterns of certain demographic groups, such as those with more access to resources or specific learning styles. This can lead to unequal learning opportunities for students from underrepresented or disadvantaged backgrounds.
Types of Bias in Adaptive Learning Systems
- Data Bias: The algorithms are often trained on historical data, which may reflect past biases. For instance, if the data predominantly comes from students in affluent areas, the system may favor their learning behaviors, overlooking the needs of students from different socioeconomic backgrounds.
- Model Bias: The choices made by developers in how algorithms process data and make decisions can introduce bias. If the model is designed to prioritize certain types of learning outcomes over others, it could disadvantage learners with different educational needs.
- Feedback Loop Bias: Adaptive systems adjust their recommendations based on past performance. If the system gives biased feedback initially, it may create a cycle where certain students are continually assessed based on skewed criteria, leading to reduced learning effectiveness.
Examples of Potential Bias in Adaptive Learning Algorithms
- Students who do not perform well in initial assessments may receive less challenging material, limiting their potential to improve.
- Underrepresented groups might receive less personalized learning experiences if their patterns of engagement differ from the majority group used to train the system.
- The system may unintentionally reinforce stereotypes by making assumptions about students' abilities based on biased data inputs.
"Bias in adaptive learning algorithms can create unequal learning environments, where certain students are unintentionally disadvantaged by the very system designed to support their growth."
Impact of Bias in Educational Outcomes
Bias Type | Effect on Learner |
---|---|
Data Bias | Students from underrepresented backgrounds may receive less effective or relevant content. |
Model Bias | Certain learning preferences may be prioritized, leaving others underserved. |
Feedback Loop Bias | Students may struggle to break free from negative performance patterns, hindering progress. |
Difficulties in Implementing Adaptive Learning in Traditional Classrooms
Adaptive learning systems, while promising, face significant challenges when integrated into traditional educational settings. These systems rely heavily on technology and data to personalize the learning experience for each student, yet in many classrooms, there are structural and logistical barriers that hinder their successful implementation. The transition from conventional teaching methods to adaptive platforms can be complicated due to factors such as limited access to resources, resistance to change, and the need for specialized training for educators.
In traditional classrooms, teachers often struggle to balance the use of technology with established teaching methods. The lack of sufficient infrastructure and training can prevent adaptive learning from being fully effective. Moreover, the individualization of learning, a core benefit of adaptive systems, can be difficult to implement when teachers have limited time and resources to manage a diverse range of student needs.
Key Challenges in Traditional Classrooms
- Lack of Technological Infrastructure: Many schools lack the necessary hardware, software, or internet connectivity to fully support adaptive learning systems.
- Teacher Readiness: Educators may be unfamiliar with the tools and methodologies required to implement adaptive learning effectively.
- Time Constraints: Teachers often have limited time to focus on each student’s specific needs, making it difficult to provide tailored instruction in real time.
- Student Resistance: Some students may resist using technology in the classroom, especially if they are accustomed to traditional methods of learning.
Challenges in Classroom Dynamics
- Increased Teacher Workload: Teachers may feel overwhelmed by the need to monitor and adjust adaptive systems, in addition to their other duties.
- Disruption to Teaching Flow: The integration of adaptive learning tools can disrupt the natural flow of classroom instruction, especially in well-established educational environments.
- Limited Access for Some Students: Adaptive learning systems may not address the needs of students with disabilities or those who require additional support.
"Adapting to new educational tools and methods can be a significant hurdle for both educators and students. It requires substantial effort, time, and resources to effectively implement adaptive learning in traditional classrooms."
Comparison of Traditional vs. Adaptive Learning Implementation
Traditional Learning | Adaptive Learning |
---|---|
Focuses on a one-size-fits-all approach. | Personalizes learning based on individual student needs. |
Limited use of technology. | Relies heavily on technology and data analytics. |
Teachers lead the classroom with fixed curriculum. | Teachers act as facilitators, with students progressing at their own pace. |
Challenges for Learners with Special Educational Needs in Adaptive Learning Systems
Adaptive learning systems are designed to provide personalized learning experiences, but they often fall short when it comes to meeting the needs of students with special educational requirements. These learners may struggle to navigate rigid, one-size-fits-all approaches that do not account for their unique challenges, such as cognitive disabilities or sensory impairments. As these systems typically adjust content based on general learning performance, they can inadvertently exclude or overwhelm students who need a different type of support or pacing.
Moreover, the lack of flexibility within these systems means that students with special needs may not receive the individualized assistance they require. While adaptive learning systems often rely on algorithms to track progress and make adjustments, they fail to account for a variety of learning difficulties, leaving behind students who need more direct guidance or alternative instructional methods.
Limited Adaptation for Diverse Learning Needs
Adaptive learning environments are not always equipped to modify their content in ways that cater to students with special needs. The adjustments they offer might be insufficient or not tailored to specific learning disabilities. Key areas of concern include:
- Cognitive Disabilities: Many adaptive learning platforms prioritize speed and efficiency over the depth of explanation or support, making it difficult for students who require more time or simplified content.
- Sensory Impairments: Students with hearing or vision impairments might not be able to access the system’s content effectively, as some platforms may not provide necessary adjustments, such as captions or audio descriptions.
- Behavioral Disorders: Adaptive learning may not consider the behavioral aspects of learning, such as students who struggle with attention or motivation, thus limiting their chances of engaging with the material meaningfully.
Failure to Provide Sufficient Support
In adaptive learning environments, systems typically adjust based on pre-set data, which may not account for the complexities of special educational needs. This leads to a lack of proper support for students who require non-standard methods of learning.
"Adaptive systems are limited in their ability to provide human-like understanding and intervention, which is crucial for learners with specific educational challenges."
For instance, while the system may adjust the difficulty of questions for a student, it might not offer the right kind of scaffolding, such as visual aids or more frequent breaks, that would help learners with special educational needs to succeed.
Summary Table: Key Challenges in Adaptive Learning for Special Needs Learners
Challenge | Description |
---|---|
Lack of Customization | Adaptive systems may not account for the specific requirements of students with cognitive or sensory impairments. |
Insufficient Support | Students may not receive the necessary level of guidance, such as emotional or behavioral support, which is critical for their success. |
Limited Flexibility | Systems may not be flexible enough to adjust to a variety of learning styles and needs, hindering students with special requirements. |