Ai Based Personalized E Learning Systems
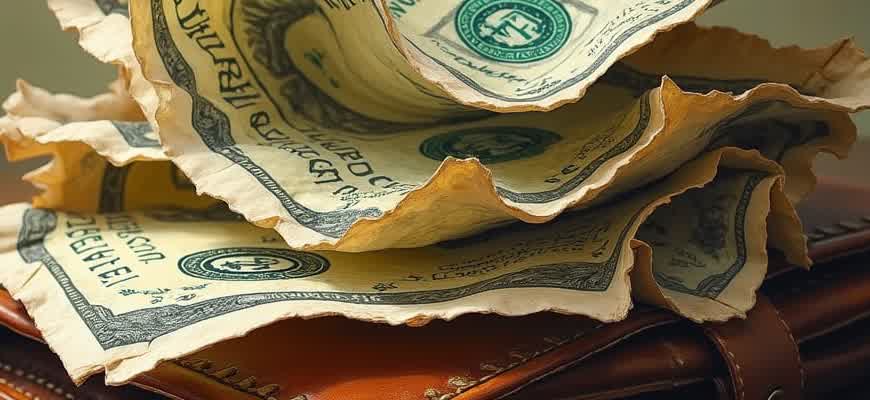
The integration of artificial intelligence in education has given rise to platforms that tailor learning experiences to individual needs. These systems collect data from user interactions to provide personalized content, enabling a more effective learning journey. By analyzing user behavior, AI can determine the pace, style, and difficulty level that best suits each learner.
Key Features of AI-Based Learning Systems:
- Adaptive content delivery based on learner progress
- Instant feedback mechanisms to guide improvement
- Dynamic course adjustments based on learner performance
- Advanced data analytics for real-time assessment
Advantages:
- Improved learner engagement through personalized experiences
- Higher retention rates due to content optimization
- Efficient resource allocation by identifying learners who need additional support
"AI-powered systems not only personalize content but also create an environment where learners can progress at their own pace, ensuring better outcomes."
Performance Evaluation:
Criteria | Before AI Implementation | After AI Implementation |
---|---|---|
Engagement | Moderate | High |
Retention Rate | 60% | 85% |
Learning Speed | Fixed | Adaptive |
AI-Driven Personalized Learning Systems
AI-based personalized learning systems leverage machine learning and data analytics to tailor educational content to individual learning styles and needs. These platforms assess the strengths and weaknesses of each learner, allowing for adaptive learning paths that maximize engagement and knowledge retention. By analyzing real-time data, AI systems can modify the difficulty level, content delivery, and types of assessments, ensuring a more customized experience.
Personalized learning powered by AI not only enhances the student’s understanding but also improves the efficiency of the learning process. The ability of these systems to provide immediate feedback and recommendations based on a learner's performance is one of their most valuable features. As AI continues to evolve, the potential for creating highly responsive and effective educational environments grows exponentially.
Key Components of AI-Powered Learning Systems
- Data Collection: AI systems gather data on learner behavior, preferences, and progress.
- Adaptive Learning Algorithms: Machine learning models adjust the learning content based on real-time performance.
- Personalized Feedback: AI provides instant, targeted feedback that helps students stay on track and improve quickly.
Benefits of AI in Education
- Increased Engagement: Content is tailored to the learner’s pace, keeping them more engaged and motivated.
- Efficiency: Learners progress at their own pace, making the learning process faster and more effective.
- Scalability: AI systems can support a large number of students without compromising the quality of instruction.
"AI-powered systems offer a scalable solution to personalized learning, transforming traditional education into a dynamic and individualized experience."
Table: Comparison of Traditional and AI-Enhanced Learning Systems
Feature | Traditional Learning | AI-Based Learning |
---|---|---|
Content Delivery | Fixed curriculum | Adaptive to learner’s needs |
Feedback | Periodic teacher assessments | Instant, real-time feedback |
Engagement | One-size-fits-all approach | Personalized learning experience |
How AI Personalizes Learning Paths for Each Student
Artificial intelligence (AI) has revolutionized personalized education by adapting learning materials to match the individual needs of students. The system continuously analyzes a learner's performance, identifying their strengths, weaknesses, and preferred learning styles. Based on this data, AI creates a tailored learning path that optimizes both the pace and depth of the content delivered. This approach ensures that each student receives a learning experience that aligns with their unique abilities and progress.
AI-driven platforms utilize a variety of techniques to customize learning. These systems adjust content dynamically, offering students the right level of difficulty and providing resources to address specific gaps in knowledge. Through real-time feedback and data-driven insights, students are guided through personalized curricula, which enhances their engagement and retention.
Key Methods of Personalization
- Adaptive Learning Systems: AI monitors a student’s interactions with the content, adjusting the difficulty level based on performance. If a student excels in a particular area, the system introduces more challenging material.
- Behavioral Analytics: AI tracks student behavior, such as time spent on tasks or responses to quizzes, to detect patterns and predict the best next steps for learning.
- Content Recommendation: Based on prior knowledge and learning progress, AI recommends supplementary resources such as articles, videos, or exercises that are most relevant to the student's needs.
Example of AI Customization Process
Step | AI Action | Outcome |
---|---|---|
1. Initial Assessment | AI tests the student’s current knowledge level | Determines areas of strength and weakness |
2. Learning Path Creation | AI designs a personalized learning trajectory | Focuses on reinforcing weak areas while challenging strong points |
3. Continuous Adjustment | AI continually adapts content based on student progress | Ensures consistent engagement and prevents frustration |
"AI allows each student to progress at their own pace, ensuring that no one is left behind or forced to move ahead too quickly."
Optimizing Content Delivery Using AI Algorithms
Artificial Intelligence plays a critical role in enhancing the effectiveness of personalized learning experiences by optimizing content delivery. By analyzing learners' data, AI systems can identify individual preferences, strengths, and weaknesses, ensuring that content is tailored to the specific needs of each student. This leads to a more efficient learning process, as students receive material that is relevant and engaging, preventing cognitive overload and disengagement.
AI algorithms use various techniques to dynamically adjust the pace and complexity of educational content. Machine learning models can track progress and make real-time adjustments, improving both engagement and retention rates. The content delivery is not only more efficient but also adapts continuously to the evolving learning journey of each individual.
Key Techniques for Content Personalization
- Adaptive Learning: AI analyzes learner data to adjust content based on performance, presenting more challenging tasks when a student demonstrates mastery or simplifying material when necessary.
- Natural Language Processing (NLP): NLP enables AI to understand and analyze students' responses, providing personalized feedback or generating relevant study resources.
- Predictive Analytics: AI uses historical data to predict learners’ future needs, helping educators plan content ahead of time that is most likely to engage the student.
Content Delivery Models
- Self-Paced Learning: Learners can move through content at their own speed, with AI adjusting difficulty levels based on their progress.
- Instructor-Led Personalization: AI assists instructors by recommending customized content for each student, allowing more targeted interventions during live sessions.
- Gamified Learning Paths: AI integrates gaming elements, providing interactive and engaging content to motivate learners.
"AI-powered systems ensure that content is always relevant, timely, and appropriately challenging, maximizing learning outcomes for each student."
Comparing Content Delivery Models
Model | Advantages | Challenges |
---|---|---|
Self-Paced Learning | Flexible, personalized learning speed | Requires self-motivation from learners |
Instructor-Led Personalization | More direct feedback and support | Relies heavily on instructor input |
Gamified Learning | Engaging and motivating for students | May distract from academic objectives |
Real-Time Adaptation to Learner’s Progress and Needs
In the realm of AI-driven personalized e-learning, real-time adaptation is crucial for delivering an experience tailored to each learner’s unique progress and requirements. These systems are designed to continuously assess a learner’s performance, dynamically adjusting content, pace, and difficulty. By analyzing responses, engagement patterns, and completion times, AI models offer a personalized learning path that aligns with the individual's evolving needs.
Real-time adaptation enhances the learning experience by responding to the learner’s actions, ensuring that they are neither overwhelmed nor under-challenged. This helps maintain motivation and fosters an efficient learning environment where students receive exactly what they need to succeed at any given moment.
Key Components of Real-Time Adaptation
- Continuous Performance Tracking: AI systems monitor how learners interact with content, including test results, task completion time, and frequency of mistakes.
- Dynamic Content Adjustments: Based on progress, the system can modify the content difficulty, offer additional resources, or provide alternative learning materials.
- Personalized Feedback: Immediate and customized feedback ensures that learners understand their mistakes and are guided toward improvement in real-time.
Example of Adaptation in Action
Stage | Action Taken by AI | Outcome |
---|---|---|
Initial Assessment | Evaluate learner’s prior knowledge and learning style | Adjust initial content to match the learner’s baseline understanding |
Mid-Learning Check | Analyze learner's engagement and progress speed | Modify pace, suggest supplemental materials or offer more challenging content |
Final Assessment | Summarize overall performance and provide detailed feedback | Offer recommendations for further learning or suggest advanced topics |
Real-time adaptation ensures that learning experiences remain relevant, keeping learners engaged and effectively progressing toward their goals.
Enhancing Learner Engagement through AI-Integrated Gamification
AI-powered gamification elements in e-learning systems offer innovative ways to increase user interaction and engagement. By utilizing adaptive learning algorithms, these systems can dynamically adjust challenges and rewards based on the learner's progress, maintaining optimal difficulty levels that keep learners motivated. Gamification strategies, such as points, badges, and leaderboards, can be tailored to each user’s unique learning style and performance, resulting in a more immersive and enjoyable experience.
The integration of AI with gamified features allows for real-time feedback and personalized incentives, making learning both fun and effective. Instead of following a rigid learning path, users can explore educational content through game-like scenarios, which encourages both critical thinking and problem-solving. The continuous adaptation to the learner’s needs creates a seamless flow, preventing frustration while promoting a sense of accomplishment.
Key AI-Driven Gamification Features
- Dynamic Difficulty Adjustment: AI adjusts challenges to match the learner’s skill level in real time, ensuring they are neither too easy nor too difficult.
- Personalized Rewards: Learners receive tailored rewards based on their performance, such as virtual badges, points, or exclusive content.
- Leaderboards: Learners can compare their performance with others, fostering healthy competition and motivation to improve.
Gamification Benefits in E-Learning
"AI-driven gamification not only enhances the learning experience but also fosters intrinsic motivation by providing personalized and adaptive challenges."
- Increased Motivation: The competitive aspect of leaderboards and achievement tracking encourages learners to engage more consistently with the material.
- Improved Retention: Gamified learning experiences are more memorable, increasing the likelihood that learners will retain information.
- Enhanced Learning Experience: Personalized challenges help learners progress at their own pace, preventing feelings of frustration or boredom.
AI-Gamification System Features
Feature | Description |
---|---|
Adaptive Learning | AI adjusts content complexity based on user performance, optimizing engagement. |
Real-Time Feedback | Immediate feedback on progress helps learners adjust and improve their performance. |
Motivational Incentives | Points, badges, and other rewards are used to recognize achievement and encourage further engagement. |
Data-Driven Insights for Educators to Monitor Student Performance
AI-powered personalized learning systems provide valuable insights that can help educators track student progress with unprecedented accuracy. These systems continuously collect and analyze vast amounts of data, offering real-time feedback on student performance, engagement, and learning patterns. By leveraging these data points, educators can identify struggling students early and provide tailored interventions to optimize learning outcomes.
Advanced analytics give educators the ability to drill down into specific areas of student performance, allowing them to target personalized learning strategies effectively. These insights can include detailed reports on areas such as comprehension rates, time spent on tasks, and overall academic trends, ensuring that teaching approaches remain adaptive and data-informed.
Key Insights Provided by AI Systems
- Real-Time Performance Tracking: AI systems provide instant reports on student engagement, assessment results, and activity completion, helping educators stay updated on student progress.
- Learning Gaps Identification: Data-driven tools pinpoint areas where students are struggling, allowing teachers to create focused, personalized remediation plans.
- Predictive Analytics: AI systems can predict future performance trends, helping educators anticipate challenges and intervene proactively before problems escalate.
These insights enable a more dynamic and responsive teaching environment, which benefits both teachers and students. Teachers can utilize the data to adjust lesson plans or provide additional support when necessary. Students, on the other hand, receive instruction that is directly aligned with their learning needs, increasing their overall engagement and success.
"Data-driven education allows for personalized interventions, ensuring that no student is left behind due to lack of attention to their unique needs."
Example of Data-Driven Monitoring
Metric | Low Performing Students | High Performing Students |
---|---|---|
Time on Task | Less than 20 minutes/day | Over 60 minutes/day |
Assessment Scores | Below 60% | Above 90% |
Completion Rate | Under 50% | Over 85% |
- By monitoring metrics such as time spent on tasks and assessment scores, educators can gain a comprehensive understanding of individual performance.
- Low-performing students may require targeted interventions or additional practice, while high-performing students can be offered advanced challenges to maintain engagement.
Integrating AI with Existing Learning Management Systems
The integration of AI-powered solutions with traditional learning management platforms offers a wide array of advantages, including personalized learning experiences and real-time data analysis. However, this integration requires careful planning and a seamless approach to ensure that both technologies complement each other effectively. By leveraging AI's ability to adapt to individual learner needs, educational institutions can enhance engagement and outcomes without overhauling their existing systems entirely.
When adding AI functionalities to established learning management systems (LMS), there are multiple strategies to consider. A smooth integration should address both the technical aspects and the user experience. It's essential to ensure that AI tools, such as chatbots, content recommendations, and learning analytics, function cohesively within the LMS's architecture to optimize the platform’s capabilities.
Steps to Integration
- Assess Compatibility: Ensure that the AI system can work with the current LMS architecture without requiring significant changes to the core platform.
- Data Integration: AI tools thrive on data. It’s critical to feed the AI system with relevant data from the LMS to create personalized learning paths for students.
- Scalability: Implement AI in a way that allows it to grow alongside the institution’s needs, providing flexibility for future updates and additions.
Challenges and Considerations
Integrating AI into existing LMS platforms might face resistance due to concerns over complexity, data privacy, and the learning curve for both educators and students. Addressing these concerns is crucial to the success of the integration.
- Technical Complexity: The integration process might require a skilled development team to ensure that the AI systems function smoothly within the LMS.
- Data Privacy: It is essential to ensure that the AI system complies with data protection regulations, safeguarding sensitive student information.
- Teacher and Student Adoption: Training instructors and students to utilize AI features effectively is key to maximizing the potential benefits.
AI-Enhanced Features for LMS
AI Feature | Benefit |
---|---|
Personalized Content Recommendations | Provides learners with tailored course materials based on individual progress and preferences. |
Real-time Learning Analytics | Offers educators insights into student performance, enabling timely interventions. |
Intelligent Tutoring Systems | Supports students with personalized, on-demand tutoring based on their learning patterns. |
How AI Personalization Improves Knowledge Retention and Skill Development
Personalized learning systems, powered by artificial intelligence, significantly enhance how learners absorb and retain information. By analyzing individual learning patterns, AI-driven platforms can adapt content to the specific needs and preferences of each student, offering a tailored experience that maximizes engagement and understanding. The ability of these systems to predict and adjust to a learner’s progress ensures that learners are continuously challenged within their zone of proximal development, leading to better retention of the material.
Furthermore, AI enhances skill acquisition by delivering content in a manner that is both dynamic and efficient. It tracks learners' performance over time, identifying areas where they may struggle and providing real-time feedback. This continuous adaptation fosters deeper learning, as learners are not forced to move on before mastering a concept. By addressing individual weaknesses and reinforcing strengths, AI-powered systems create an optimized path for skill development.
Personalized Learning Benefits for Retention
- Targeted content delivery: AI systems deliver content that aligns with the learner's proficiency, helping them engage with material at an appropriate difficulty level.
- Real-time feedback: Immediate feedback on mistakes allows learners to adjust their approach and reinforce concepts immediately.
- Adaptive pacing: AI adjusts the speed of learning based on how quickly a student grasps concepts, ensuring that they are neither bored nor overwhelmed.
Impact on Skill Development
- Customized learning paths: The AI designs a unique journey for each learner, focusing on their individual areas for improvement and skill progression.
- Continuous tracking and updates: Regular assessments help refine the learning process and align it with evolving skill sets.
- Engagement through personalization: Personalization leads to a greater sense of ownership and motivation, encouraging learners to invest in the learning process.
AI-driven learning platforms offer not just personalized content, but also a feedback loop that continuously refines the learning experience to ensure maximum knowledge retention and skill development.
Efficiency in Knowledge Retention
AI Function | Effect on Retention |
---|---|
Real-time assessments | Provides immediate correction, preventing knowledge gaps from forming. |
Personalized challenges | Helps reinforce material through targeted repetition at ideal intervals. |
Dynamic learning paths | Adapts to the learner’s progress, keeping the content fresh and engaging. |
Ensuring Data Privacy and Security in AI Learning Systems
As artificial intelligence (AI) becomes increasingly integrated into personalized learning environments, ensuring data privacy and security has become a critical concern. AI-based learning platforms collect and process vast amounts of sensitive user data, including personal information, learning behaviors, and academic performance. Protecting this data is essential not only for regulatory compliance but also to maintain trust between users and providers.
Various measures must be implemented to safeguard sensitive data in AI-powered education systems. This includes the use of robust encryption protocols, the anonymization of user data, and the implementation of access control mechanisms. Furthermore, the involvement of AI technologies such as machine learning in data handling brings additional complexities that need to be addressed to prevent unauthorized access or misuse.
Key Data Protection Practices in AI-Based Learning Systems
- Data Encryption: All sensitive information, whether stored or transmitted, should be encrypted using advanced encryption standards (AES). This ensures that even if data is intercepted, it cannot be read or tampered with.
- Anonymization: Personal data should be anonymized to prevent identification of individuals in datasets. This reduces the risk of data being linked back to a specific person, enhancing privacy.
- Access Controls: Strict access controls must be implemented, ensuring that only authorized personnel or systems can access sensitive data. This includes multi-factor authentication (MFA) for users and administrative controls for system managers.
- Data Minimization: Collect only the data necessary for the specific purpose of the AI system, reducing the risk of data over-collection and unnecessary exposure.
Important: AI systems should also include monitoring tools that detect any unusual access patterns or potential security breaches in real-time. Proactive monitoring helps prevent security incidents before they escalate.
Security Measures and Compliance Frameworks
- Regulatory Compliance: Ensure adherence to privacy laws and regulations such as the General Data Protection Regulation (GDPR) and the Family Educational Rights and Privacy Act (FERPA), which mandate strict data handling and storage guidelines.
- Security Audits: Regular security audits should be conducted to identify vulnerabilities in the system, ensuring compliance with security standards and identifying areas for improvement.
- Third-Party Data Protection: If third-party vendors are involved in the data processing or storage, ensure they also comply with the same security protocols and privacy standards to prevent data leakage or breaches.
Technology and Tools for Securing AI Learning Systems
Technology | Purpose | Benefits |
---|---|---|
Blockchain | Secure and immutable data storage | Prevents unauthorized changes to data, enhances transparency |
Federated Learning | Distributed learning without data sharing | Protects data privacy by training models locally |
Homomorphic Encryption | Encrypts data while it’s being processed | Enables secure computations without exposing sensitive data |