Ai for Personalized Shopping Experience
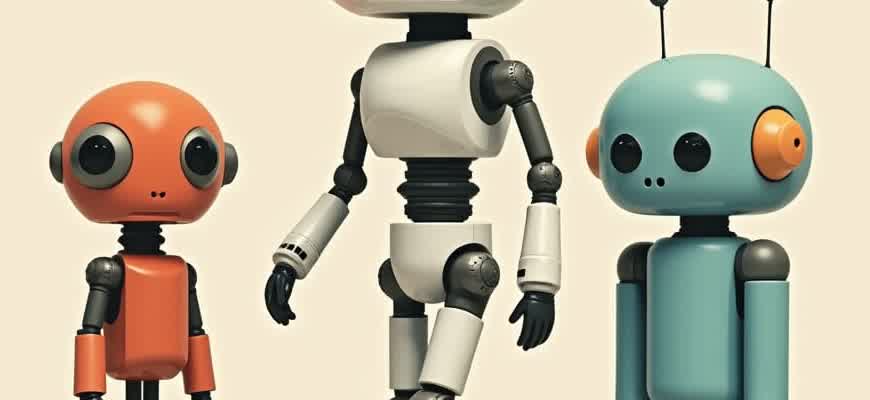
Artificial intelligence is revolutionizing the way consumers interact with online retail. By leveraging machine learning algorithms, retailers can tailor product recommendations and shopping experiences to individual preferences and behaviors. This approach not only enhances customer satisfaction but also drives higher conversion rates and brand loyalty.
AI analyzes vast amounts of data from various sources, including browsing history, purchase patterns, and even social media activity. Based on this information, it can predict what products a customer is most likely to buy, personalize offers, and create a more engaging shopping environment.
"AI allows for a level of personalization that was previously impossible, making the shopping experience feel uniquely tailored to each individual."
Key benefits of AI in personalized shopping:
- Improved product recommendations
- Dynamic pricing based on customer behavior
- Enhanced customer engagement through personalized content
Common AI-powered technologies used in this context include:
- Recommendation engines
- Chatbots for real-time support
- Visual search tools
Example of AI integration in e-commerce:
Technology | Use Case | Benefit |
---|---|---|
Recommendation Engines | Suggest products based on user history | Increased conversion rates and average order value |
Chatbots | Provide personalized customer service | Higher customer satisfaction and retention |
Visual Search | Allow users to search for items by uploading images | Faster, more accurate product discovery |
AI in Enhancing Tailored Shopping Experiences
Artificial intelligence has become a transformative force in the retail sector, reshaping how brands engage with customers. By leveraging data analytics, machine learning, and customer behavior prediction, AI enables a highly customized shopping journey. From personalized product recommendations to targeted promotions, the AI-driven shopping experience is designed to optimize both customer satisfaction and sales conversion.
Through continuous learning algorithms, AI systems can analyze vast amounts of consumer data, including browsing history, purchase patterns, and preferences. This allows for the creation of dynamic, individualized shopping experiences that adapt in real-time, making interactions more relevant and engaging for each user.
Key AI Applications in Personalized Shopping
- Product Recommendations: AI uses algorithms to suggest products based on past purchases and browsing history.
- Dynamic Pricing: Prices are adjusted in real-time based on user behavior, demand, and external market factors.
- Chatbots and Virtual Assistants: AI-powered bots provide immediate support, guiding customers through their shopping journey.
- Visual Search: AI enables customers to search for products using images instead of keywords, increasing convenience and accuracy.
Impact on Customer Experience
By offering real-time personalization, AI significantly improves user engagement. Customers feel understood, leading to higher satisfaction and repeat business. Below are key benefits:
Benefit | Description |
---|---|
Increased Conversion Rates | Personalized experiences encourage more purchases by showing relevant products. |
Customer Retention | AI builds trust by consistently offering tailored recommendations, keeping customers loyal. |
Enhanced User Engagement | Real-time interactions create a sense of connection, fostering deeper customer engagement. |
"AI in retail isn't just about automation; it's about creating meaningful, individualized experiences that resonate with customers at a personal level."
How AI Analyzes Customer Data to Predict Shopping Preferences
Artificial intelligence plays a crucial role in shaping the future of personalized shopping experiences by utilizing vast amounts of consumer data. By analyzing individual behaviors, purchase history, and online interactions, AI systems are able to identify patterns and preferences that enable businesses to create highly tailored offerings. This process relies heavily on machine learning algorithms that continuously improve their predictions by learning from new data points in real-time.
AI-driven platforms track and analyze various types of consumer data, including browsing history, social media activity, and past purchase behavior, to build a comprehensive profile of each customer. This profile is then used to predict what the customer might be interested in next. Over time, as the AI learns from new interactions, its predictions become increasingly accurate, allowing for a hyper-personalized shopping experience.
Key Elements in Data Analysis
- Behavioral Patterns: AI tracks how customers interact with websites, such as the types of products viewed or the frequency of purchases.
- Purchase History: Analyzing past buying habits helps AI understand the customer’s preferences and anticipated future purchases.
- Browsing Activity: Monitoring what products are browsed and for how long provides insights into potential interests.
Steps in Predicting Preferences
- Data Collection: Customer data is collected through various touchpoints, including websites, apps, and social media.
- Pattern Recognition: AI models analyze collected data to identify trends and behavior patterns.
- Personalized Recommendations: Based on learned preferences, the AI suggests products tailored to the individual.
- Continuous Learning: The AI continues to update its predictions as more data becomes available, refining the recommendations.
"AI's ability to process large volumes of data and predict customer preferences with high accuracy is revolutionizing the shopping experience, making it more intuitive and relevant for each consumer."
Data Analysis in Action: Example
Data Point | Impact on Recommendations |
---|---|
Browsing History | AI identifies product categories that customers are most likely to explore and recommends similar items. |
Purchase Frequency | Frequent buyers of a particular product are shown related or upgraded versions of the same product. |
Social Media Interactions | AI tracks likes and shares to recommend trending products aligned with the customer's interests. |
Harnessing AI to Suggest Products Based on Customer Behavior
AI-driven product recommendations have become an essential component of modern e-commerce platforms. By analyzing consumer interactions, purchase history, and browsing habits, AI models can offer personalized product suggestions that cater to each individual's preferences. This not only enhances user experience but also boosts conversion rates and customer satisfaction.
Through advanced machine learning algorithms, AI is capable of identifying patterns and predicting future buying behavior. This enables platforms to recommend products that align with the customer's unique needs and interests, increasing the likelihood of a sale and fostering brand loyalty.
Key Approaches for Personalized Product Suggestions
- Behavioral Tracking: AI systems track user actions, such as clicks, time spent on pages, and items added to carts, to understand interests and preferences.
- Purchase History Analysis: By reviewing past purchases, AI can recommend similar or complementary items that the customer is likely to buy.
- Real-Time Adaptation: AI adapts in real-time to changes in a customer's behavior, offering fresh recommendations based on their latest activity.
Types of AI-Driven Recommendations
- Collaborative Filtering: Suggests products based on the preferences of similar users.
- Content-Based Filtering: Recommends items similar to those the customer has shown interest in, based on product features.
- Hybrid Models: Combines both collaborative and content-based methods for more accurate predictions.
"AI recommendations are not only about predicting what the user might want, but also about enhancing the overall shopping journey by offering the right product at the right moment."
Impact of Personalized Recommendations
The personalized shopping experience not only increases user engagement but also drives business success. According to recent data, users are 50% more likely to purchase products that were recommended based on their previous activity. Additionally, tailored suggestions can reduce the time spent searching for products, making the shopping process more efficient.
Metric | Impact of AI Recommendations |
---|---|
Conversion Rate | Increased by 30-50% |
Customer Retention | Improved by 15-20% |
Average Order Value | Boosted by 10-25% |
Real-Time Personalization: Tailoring Offers Based on User Engagement
In today's competitive e-commerce landscape, delivering a personalized shopping experience in real time is crucial for maximizing customer satisfaction and conversion rates. By closely monitoring user actions and behaviors, companies can instantly modify offers, product recommendations, and content to better suit individual preferences. This immediate response not only increases the relevance of the shopping journey but also strengthens the relationship between the retailer and the customer.
Real-time personalization leverages advanced machine learning algorithms to analyze user interactions, such as browsing history, time spent on specific items, and cart activity. By doing so, retailers can dynamically adapt their offers, ensuring that each shopper sees the most relevant promotions and suggestions at the moment when they're most likely to convert.
Key Benefits of Real-Time Personalization
- Increased Engagement: Customizing content to fit a user’s needs keeps them engaged longer, boosting retention rates.
- Improved Conversion Rates: Relevant offers and recommendations lead to higher chances of purchase.
- Enhanced Customer Satisfaction: Personalization fosters a more tailored experience, making customers feel valued.
How It Works
- Data Collection: User actions such as clicks, searches, and page views are tracked in real-time.
- Behavioral Analysis: Machine learning models analyze patterns from collected data to predict preferences.
- Offer Adjustment: The system adjusts product recommendations, discounts, or promotions based on predicted preferences.
"Personalizing the shopping experience in real time transforms passive visitors into active buyers, creating a more efficient and enjoyable path to purchase."
Example of Dynamic Offer Adjustments
User Behavior | Offer Adjustment |
---|---|
Viewed multiple laptops | Show personalized laptop discounts and accessories |
Abandoned cart with shoes | Send an offer with a discount code for shoes and related products |
Browsed a specific brand | Display similar products from the same brand with personalized messaging |
Enhancing Customer Engagement with AI-Driven Chatbots
AI-powered chatbots have revolutionized how businesses interact with customers by providing real-time, personalized assistance. These intelligent systems allow brands to offer tailored shopping experiences, improving customer satisfaction and driving conversion rates. By analyzing user behavior, preferences, and past interactions, AI chatbots can recommend products, answer questions, and guide customers through the buying process in a seamless, efficient manner.
The key to enhancing customer engagement lies in the chatbot's ability to adapt to individual needs. Whether it's suggesting new arrivals based on previous purchases or addressing specific customer inquiries, chatbots can create a dynamic and interactive experience that traditional customer service cannot match. This continuous, personalized interaction encourages deeper engagement, leading to higher customer loyalty and retention.
How AI Chatbots Improve Engagement
- 24/7 Availability: AI chatbots provide constant support, ensuring customers can engage at any time of day.
- Instant Response: Customers receive immediate answers, reducing wait times and increasing satisfaction.
- Personalized Recommendations: By analyzing customer preferences and behavior, chatbots offer tailored product suggestions, improving the shopping experience.
- Proactive Assistance: Chatbots can anticipate customer needs and proactively offer help, enhancing user satisfaction.
Benefits of AI-Driven Chatbots
Feature | Benefit |
---|---|
Real-Time Interaction | Instant answers to queries, reducing friction during the shopping process. |
Data-Driven Insights | Chatbots can analyze customer data to offer better product recommendations and predict future needs. |
Scalability | AI chatbots can handle thousands of customers simultaneously without sacrificing quality of service. |
"AI chatbots are not just tools for customer service; they are integral to the creation of personalized shopping experiences that make customers feel valued and understood."
Leveraging AI for Optimized Inventory Control in Personalized Shopping
Artificial intelligence (AI) plays a crucial role in refining the way businesses manage their inventories, particularly in the context of delivering customized shopping experiences. By analyzing real-time data, AI can help retailers predict demand patterns more accurately, ensuring that the right products are available for specific customer segments. This level of precision can lead to higher sales, reduced waste, and an overall more efficient operation. Additionally, AI enhances the ability to tailor product suggestions by analyzing individual shopping behavior and aligning stock levels to meet the unique preferences of each customer.
Furthermore, AI-driven inventory management offers a dynamic approach to optimize product availability based on demand forecasting, ensuring customers have access to items they are most likely to purchase. This approach helps companies offer relevant recommendations while maintaining a balance between supply and demand. By continuously learning from interactions, AI can also adjust suggestions in real-time based on shifts in consumer behavior, enabling highly personalized shopping experiences.
Key Benefits of AI-Driven Inventory Management
- Demand Forecasting: Predicts future demand more accurately based on historical data and trends.
- Stock Optimization: Ensures that products are stocked according to customer preferences, reducing overstock and stockouts.
- Real-Time Adjustments: Reacts to market changes or customer behavior in real time to make inventory decisions.
How AI Improves Personalization Through Inventory Management
- Behavioral Analysis: AI analyzes customer browsing history and purchase patterns to understand preferences and recommend products accordingly.
- Dynamic Inventory Adjustments: When AI detects a customer’s interest in a specific category or item, it triggers inventory adjustments to align with these interests, improving availability and customer satisfaction.
- Supply Chain Coordination: AI optimizes stock replenishment by predicting which items need restocking based on demand fluctuations from different customer segments.
"AI-driven inventory management not only enhances operational efficiency but also transforms the customer shopping experience by making it more personalized and relevant."
Example of AI-Based Inventory Management in Action
AI Feature | Benefit | Example |
---|---|---|
Demand Forecasting | Ensures products are available during peak demand periods | Retailer predicts high demand for winter jackets in a particular region and adjusts inventory accordingly. |
Personalized Recommendations | Improves customer satisfaction with relevant suggestions | AI suggests shoes based on past purchase data, increasing conversion rates for the category. |
Dynamic Stock Adjustments | Reduces the risk of overstocking or stockouts | AI identifies a sudden rise in demand for a specific item and triggers restocking alerts automatically. |
AI-Driven Visual Search: Enhancing Product Discovery
The advent of AI-powered image recognition has revolutionized the way consumers discover products online. By utilizing advanced algorithms, visual search technology allows users to search for products based on images rather than keywords. This process helps bridge the gap between what shoppers see and what they want to buy, offering a more intuitive shopping experience. The ability to search with a picture, whether from a website or from their own photos, enables users to find similar products quickly and efficiently.
This technology is especially beneficial in the retail sector, where shoppers may not have the right words to describe a specific item they’ve seen or liked. AI systems analyze visual features like color, shape, and texture to identify products, providing highly accurate results. As a result, shoppers can easily match their interests with available inventory, enhancing both speed and satisfaction in the purchasing journey.
How It Works
AI-powered visual search operates through a multi-step process that combines computer vision and machine learning. Here's how it typically functions:
- Image Input: The shopper provides an image, either taken from their own device or captured on a website.
- Feature Extraction: The AI analyzes key features of the image, such as color, pattern, and shape.
- Matching Process: The system compares these features to a vast product database to identify similar items.
- Result Presentation: Relevant products are displayed with details, making it easy for the shopper to make a choice.
Key Benefits
Benefit | Description |
---|---|
Speed and Efficiency | Shoppers can find products in seconds, without having to type out detailed descriptions. |
Enhanced User Experience | Visual search provides a more intuitive and engaging method of shopping. |
Broader Selection | Consumers can discover similar items they may not have otherwise found through traditional search methods. |
"AI visual search transforms the way customers interact with e-commerce platforms, helping them find products with greater ease and accuracy."
Integrating AI with Loyalty Programs to Boost Customer Retention
Incorporating artificial intelligence into loyalty programs offers a unique opportunity to enhance customer retention by providing personalized rewards, targeted offers, and improved shopping experiences. By analyzing customer behavior, AI systems can predict future preferences and suggest tailored incentives that resonate with individual customers. This level of personalization strengthens emotional ties to the brand and increases the likelihood of repeat purchases.
AI-powered loyalty programs leverage customer data, such as purchase history and browsing patterns, to create dynamic reward systems. These systems adapt in real-time, ensuring that rewards stay relevant and enticing. As a result, businesses can drive higher engagement and customer loyalty, ultimately leading to long-term revenue growth.
How AI Enhances Loyalty Programs
- Personalized Offers: AI analyzes customer data to recommend rewards that match the customer’s preferences and buying habits.
- Dynamic Reward Tiers: Loyalty programs can automatically adjust reward levels based on customer engagement, incentivizing continued participation.
- Real-Time Adaptation: AI allows businesses to adapt rewards quickly based on changes in customer behavior or market trends.
- Optimized Communication: AI ensures the right message reaches the right customer at the optimal time, increasing the chance of conversion.
Benefits of AI-Enhanced Loyalty Programs
Benefit | Description |
---|---|
Increased Engagement | AI personalizes interactions, making customers feel valued and more likely to engage with the brand. |
Improved Customer Retention | Personalized rewards foster long-term relationships by catering to customers' individual preferences. |
Higher Revenue Potential | Engaged and loyal customers tend to spend more, contributing to increased lifetime value. |
"By implementing AI-driven insights, businesses can shift from one-size-fits-all loyalty programs to highly personalized experiences that keep customers coming back."
Ethical Considerations: Balancing Personalization with Privacy Concerns
As AI continues to reshape personalized shopping experiences, businesses must carefully consider the ethical implications of collecting and utilizing consumer data. On one hand, personalized recommendations improve the shopping journey by offering products that align with individual preferences. On the other hand, excessive data collection raises significant concerns about privacy, data security, and consumer autonomy.
While the potential for AI to enhance shopping experiences is vast, companies face the challenge of ensuring they respect consumer privacy while delivering tailored services. Striking the right balance between customization and ethical responsibility is crucial for maintaining trust and fostering long-term relationships with customers.
Privacy Risks in Personalized Shopping
Personalization relies on extensive data gathering, which can inadvertently expose sensitive customer information. Ethical risks can arise from:
- Inadequate data protection measures, leading to potential breaches.
- Over-collection of data, going beyond what is necessary for personalization.
- Lack of transparency about how data is used and shared.
To mitigate these risks, companies must prioritize data privacy and limit the use of personal information to what is strictly necessary. They should also ensure that users are aware of and in control of their data choices.
Ensuring Ethical AI in Shopping
Companies can adopt several strategies to maintain ethical AI practices:
- Transparency: Clearly communicate data collection methods and their purposes.
- Consumer Control: Offer customers the ability to opt-in or opt-out of personalized services.
- Data Security: Implement robust encryption and security protocols to protect customer information.
These steps help safeguard consumer rights while allowing businesses to use AI responsibly. It's essential to avoid exploiting personal data in ways that could erode customer trust.
"Maintaining a fair balance between offering customized experiences and ensuring user privacy is the cornerstone of building trust in AI-driven retail."
Best Practices for Data Handling in Personalized Shopping
Best Practice | Description |
---|---|
Data Minimization | Collect only the data necessary for delivering personalized experiences, avoiding excessive or intrusive data gathering. |
Clear Consent | Ensure that consumers provide informed consent before any data is collected or used for personalized recommendations. |
Regular Audits | Conduct regular audits to verify compliance with privacy standards and identify potential areas of concern. |