Diy Ai Courses Ireland
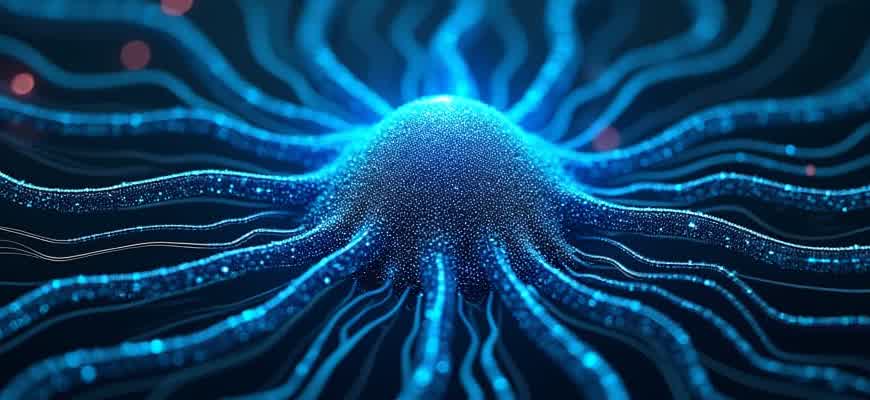
In Ireland, there is an increasing demand for Artificial Intelligence skills, and various educational platforms are offering DIY (Do-It-Yourself) courses for individuals keen to learn AI at their own pace. These self-guided programs are designed to help learners build a strong foundation in AI concepts, algorithms, and applications without the need for formal classroom settings.
Here are some key benefits of opting for a DIY AI course in Ireland:
- Flexible Learning - Take control of your learning schedule and progress at your own pace.
- Cost-Effective - Many platforms offer affordable courses compared to traditional universities or bootcamps.
- Hands-On Projects - Most courses include practical assignments and projects to develop real-world AI solutions.
"AI education is moving towards accessibility, making it easier for anyone in Ireland to dive into this high-demand field from the comfort of their own home."
For those looking to explore these options further, here is a quick overview of some popular DIY AI training platforms available in Ireland:
Platform | Course Type | Level | Price Range |
---|---|---|---|
Coursera | AI for Everyone | Beginner | $39/month |
Udacity | AI Programming with Python | Intermediate | $399/month |
edX | Machine Learning Fundamentals | Beginner to Advanced | Free (Certificate Available for $199) |
DIY AI Courses in Ireland: A Practical Guide
For those interested in learning artificial intelligence (AI) independently in Ireland, a wealth of resources is available to guide you through the process. DIY AI courses offer flexibility, allowing learners to pace their studies and choose areas of focus that match their personal interests or career goals. These courses can range from basic introductions to AI to more specialized topics such as machine learning, neural networks, and deep learning.
While some of the best resources are free or low-cost, it’s important to select the right learning paths and platforms. In this guide, we will look at essential elements of starting an AI journey independently, including how to structure your learning, the top platforms to use, and additional tips for success.
Choosing the Right Learning Path
When starting your AI journey, it’s crucial to identify your goals. AI is a broad field, so understanding the types of AI you want to explore will help focus your learning efforts. Here are some common starting points:
- Basic AI concepts: Start with foundational knowledge such as programming (Python), algorithms, and data structures.
- Machine Learning: Focus on supervised and unsupervised learning, data preprocessing, and model evaluation.
- Deep Learning: Delve into neural networks, convolutional networks, and reinforcement learning.
For many, machine learning and deep learning are the most popular areas of interest. However, understanding the underlying mathematical concepts, such as statistics and linear algebra, is crucial for success in these areas.
Top Platforms for DIY AI Courses
Here are some platforms offering DIY AI courses suitable for learners in Ireland:
- Coursera: Offers a variety of AI courses from institutions like Stanford University and the University of London.
- edX: Provides free and paid courses from top universities such as MIT and Harvard.
- Udemy: A budget-friendly option with courses focusing on practical AI applications and coding in Python.
- Fast.ai: Known for its hands-on approach to deep learning, providing free resources with a focus on practical implementation.
These platforms allow for flexible learning at your own pace, but they also provide structured learning paths that can help you stay on track. The key is to stay disciplined and consistent with your learning schedule.
Important Considerations
To succeed in DIY AI courses, it’s important to engage with the community. Join online forums, participate in Kaggle competitions, and contribute to open-source AI projects to gain practical experience.
For learners in Ireland, joining local meetups or online communities can provide valuable networking opportunities and real-world project experience. This connection can also help you find mentorship and collaborative projects that boost your understanding of AI.
Summary of Key Resources
Platform | Key Features | Cost |
---|---|---|
Coursera | University-led, comprehensive courses | Free courses, certificates for a fee |
edX | University-level content, practical skills | Free courses, certificates for a fee |
Udemy | Affordable, project-based courses | Affordable one-time fees |
Fast.ai | Hands-on deep learning courses | Free |
With the right tools, dedication, and approach, DIY AI learning can open doors to a variety of career opportunities in tech fields. Start small, focus on your interests, and leverage online resources to accelerate your learning.
Choosing the Right AI Course for Beginners in Ireland
With the rapid growth of artificial intelligence, it's no surprise that many individuals in Ireland are looking to break into this exciting field. However, with a multitude of courses available, finding the right one for beginners can be overwhelming. Choosing a course that suits your background, learning style, and goals is essential to ensure you gain a solid foundation in AI.
When selecting a beginner-friendly AI course in Ireland, consider factors such as course content, instructors' expertise, and the flexibility of the course delivery. Many institutions offer both in-person and online options, catering to various learning preferences. Additionally, it's important to evaluate whether the course provides practical hands-on experience or focuses solely on theoretical knowledge.
Key Factors to Consider
- Course Content: Look for courses that cover essential AI concepts, such as machine learning, neural networks, and natural language processing.
- Instructor Expertise: Ensure that instructors have real-world experience and relevant academic credentials in the field of AI.
- Delivery Format: Choose between online, in-person, or hybrid learning depending on your preference and availability.
- Practical Experience: It's crucial to select courses that provide opportunities to work on AI projects or case studies.
Recommended Course Structure
- Introduction to AI Concepts
- Fundamentals of Machine Learning
- Introduction to Deep Learning and Neural Networks
- Data Preprocessing and Visualization
- Hands-on AI Projects
"Practical experience in AI projects allows beginners to not only grasp theoretical knowledge but also gain confidence in applying AI techniques to real-world scenarios."
Course Comparison Table
Course Provider | Delivery Mode | Duration | Cost |
---|---|---|---|
University of Dublin | Online | 12 weeks | €500 |
Tech Academy | In-person | 6 weeks | €350 |
AI Learning Hub | Hybrid | 8 weeks | €400 |
How to Structure Your Self-Learning Plan for AI Mastery
Mastering Artificial Intelligence requires a disciplined approach, especially if you are learning on your own. To effectively navigate through the vast landscape of AI concepts, tools, and techniques, you need a structured learning plan. A well-defined plan helps you stay focused, track progress, and ensures that you cover the necessary areas without feeling overwhelmed.
The key to a successful AI learning journey is to break down the process into manageable steps. By organizing your study into clear phases, you can methodically build up your knowledge and skills. Here’s how you can approach your self-learning plan.
1. Define Your Learning Goals
Before diving into the technicalities of AI, it's essential to identify your ultimate learning objectives. What do you want to achieve? Do you aim to become proficient in machine learning, or do you want to understand the theoretical foundations of AI? Clarifying these goals will shape your learning strategy.
Set SMART goals: Specific, Measurable, Achievable, Relevant, and Time-bound. This will help you stay on track and avoid feeling lost.
2. Break Down the AI Learning Process
AI is a vast field, so it's important to segment your studies into clear stages. Here’s a step-by-step guide on how you can approach the learning process:
- Foundational Knowledge – Start with learning the basics of programming and mathematics (linear algebra, probability, and statistics). These are essential for understanding more advanced AI concepts.
- Core AI Topics – Once the foundations are in place, focus on core areas such as machine learning, deep learning, and natural language processing (NLP).
- Practical Applications – Work on real-world AI projects or participate in Kaggle competitions. Apply your knowledge to solve practical problems and gain hands-on experience.
- Advanced Concepts – Dive into advanced topics like reinforcement learning, computer vision, and AI ethics once you have mastered the core material.
3. Build a Consistent Routine
Consistency is key when learning any new subject. Set aside dedicated time each day or week to focus on AI. A structured routine will help reinforce learning and keep you progressing towards your goals.
4. Utilize Online Resources and Tools
AI is a rapidly evolving field, and there are many high-quality resources available online. Here are some valuable resources:
- Online Courses – Platforms like Coursera, edX, and Udacity offer comprehensive AI courses.
- Textbooks – Some popular books include "Deep Learning" by Ian Goodfellow and "Hands-On Machine Learning with Scikit-Learn, Keras, and TensorFlow" by Aurélien Géron.
- Open-Source Libraries – Familiarize yourself with tools like TensorFlow, PyTorch, and Scikit-learn to implement AI algorithms.
5. Track Your Progress
Regularly assess your progress to stay motivated. You can create a tracking system to monitor milestones and identify areas where you need to improve. This might include completing coding exercises, building mini-projects, or reading specific chapters from AI textbooks.
Learning Phase | Key Resources | Expected Timeframe |
---|---|---|
Foundations | Books, online tutorials | 2-3 months |
Core AI Topics | Online courses, hands-on practice | 3-4 months |
Practical Projects | Kaggle, GitHub repositories | 2-3 months |
Advanced Concepts | Research papers, specialized courses | Ongoing |
Key Tools and Platforms for Building AI Projects on Your Own
When venturing into AI development independently, it is crucial to have access to reliable tools and platforms. These resources enable you to create, train, and deploy AI models effectively. The landscape is diverse, offering both beginner-friendly platforms and advanced tools for those with experience in coding and machine learning.
Choosing the right tools depends on the scope of your project and the technologies you're comfortable working with. Here’s an overview of the essential tools and platforms to help you get started with AI development.
Popular Tools and Platforms
- TensorFlow: A powerful open-source framework for building and training machine learning models, ideal for deep learning projects.
- PyTorch: Known for its dynamic computation graph, PyTorch is a great choice for researchers and developers looking for flexibility in model building.
- Keras: A high-level neural networks API that simplifies the process of building and experimenting with deep learning models.
- Google Colab: A cloud-based platform that provides free access to GPUs for running and training AI models with Python.
- Azure AI: A comprehensive platform from Microsoft offering tools for developing, deploying, and managing AI solutions.
Key Resources for AI Development
- Jupyter Notebooks: Ideal for experimenting with code in an interactive environment, making it easier to visualize results.
- Scikit-learn: A simple and efficient tool for data mining and machine learning tasks like classification, regression, and clustering.
- Fast.ai: A deep learning library designed to make it easier for developers to create AI models with minimal effort.
- OpenCV: A library that provides tools for computer vision tasks, such as image processing and object detection.
Important Notes to Consider
When choosing the right tools, make sure to consider the specific needs of your project. If your focus is on computer vision, OpenCV and TensorFlow are excellent choices, while natural language processing tasks might benefit from using libraries like NLTK or Hugging Face.
Comparing Key Platforms for AI Projects
Platform | Features | Best For |
---|---|---|
Google Colab | Free access to GPUs, cloud-based, Python environment | Collaborative projects and small-scale AI experimentation |
Azure AI | Comprehensive cloud AI services, scalable infrastructure | Enterprise-level AI deployment and management |
TensorFlow | Extensive library for deep learning, cross-platform | Building complex machine learning models and AI applications |
Overcoming Common Challenges When Learning AI Independently
Learning Artificial Intelligence (AI) on your own can be an exciting yet challenging journey. While the abundance of online resources has made it easier to dive into AI, there are still significant hurdles that many learners face. These obstacles can slow progress and sometimes even discourage students from continuing. Understanding these common challenges and how to overcome them is essential for anyone pursuing a self-guided learning path in AI.
One of the main challenges faced by independent learners is dealing with complex and rapidly changing material. AI is an interdisciplinary field, requiring knowledge in mathematics, programming, and domain-specific knowledge. Additionally, with constant advancements in research and tools, it’s difficult to keep up. Here are some practical strategies to help overcome these issues:
1. Building a Strong Foundation
Before diving deep into AI-specific topics, it is crucial to solidify your understanding of foundational subjects. Focus on:
- Linear algebra
- Calculus
- Probability and statistics
- Programming skills (Python is most commonly used in AI)
Without a solid grasp of these fundamental areas, learning advanced AI concepts will be far more difficult. Take the time to go through relevant courses or tutorials before moving to more complex subjects.
2. Managing the Overwhelming Amount of Information
The AI field is vast, and it can be overwhelming to determine where to start. A clear roadmap or structured curriculum can help guide your progress. Breaking down your learning journey into manageable milestones can make the process more organized and less stressful:
- Start with basic machine learning concepts (e.g., supervised vs unsupervised learning).
- Then move to more advanced topics like neural networks and deep learning.
- Finally, explore specialized areas such as reinforcement learning or natural language processing.
Tip: Set small, achievable goals for yourself and track your progress. It will help keep you motivated and reduce feelings of being overwhelmed.
3. Finding Reliable Learning Resources
One of the most difficult aspects of learning AI on your own is finding trustworthy and high-quality materials. The AI community offers a variety of free and paid resources, but not all are equal in quality. Here’s a quick comparison of popular resources:
Resource Type | Example | Strength | Weakness |
---|---|---|---|
Online Courses | Coursera, edX | Structured curriculum, expert instructors | May require a subscription or fee |
Books | "Deep Learning" by Ian Goodfellow | In-depth coverage, authoritative | Can be dense and difficult to follow without prior knowledge |
Research Papers | arXiv.org | Cutting-edge information | Requires familiarity with advanced topics |
Leverage a mix of these resources to get the most comprehensive learning experience.
Finding Community Support and Networking in AI Learning
Building a strong network of support is essential when diving into the complexities of AI education. It can be challenging to master AI concepts in isolation, so connecting with other learners, experts, and local communities can significantly enhance the learning experience. Engaging with peers and mentors provides not only academic guidance but also motivation and inspiration to keep progressing through the course material.
Many learners benefit from joining online platforms and local meetups where AI topics are discussed, and collaborations are encouraged. Whether through social media, forums, or dedicated AI hubs, the opportunities to connect with others in similar learning stages or more experienced professionals are abundant. Networking within the AI community helps in exchanging ideas, troubleshooting problems, and receiving feedback on projects.
Benefits of Community Support in AI Learning
- Collaboration Opportunities: Working together with others can lead to innovative solutions and a deeper understanding of complex AI topics.
- Peer Feedback: Receiving feedback from fellow learners or experts helps refine your skills and avoid common pitfalls.
- Mentorship: Experienced mentors can provide valuable insights and guidance on career paths, research, and advanced AI topics.
- Shared Resources: Community members often share resources like courses, tools, and datasets that can help you further your learning.
"Networking within the AI community is not just about finding answers to questions, but also about creating opportunities for collaborative growth."
How to Network in AI Learning
- Join AI-related forums such as Reddit’s Machine Learning or AI Alignment Forum to discuss ideas and problems.
- Attend local meetups or conferences like AI Expo Ireland, which focus on AI development and learning.
- Participate in online coding challenges and competitions, such as those on Kaggle, to meet others in the field.
- Follow and engage with thought leaders and AI professionals on platforms like Twitter or LinkedIn to stay updated with the latest trends.
Table of Local AI Events in Ireland
Event | Date | Location |
---|---|---|
AI Expo Ireland | June 2025 | Dublin |
Machine Learning Meetup | Monthly | Cork |
Data Science and AI Workshop | October 2025 | Limerick |
How to Stay Updated with the Latest Trends in AI and Machine Learning
In the rapidly evolving fields of artificial intelligence (AI) and machine learning (ML), staying informed about the latest advancements and trends is crucial for professionals, enthusiasts, and learners. The fast pace of innovation means new techniques, algorithms, and tools are being introduced constantly, making it challenging to keep up. However, by leveraging the right resources, individuals can stay ahead of the curve and continue to develop their skills effectively.
Here are several practical strategies to remain current with developments in AI and ML:
1. Engage with Online Communities and Forums
Joining online communities focused on AI and ML can provide real-time updates on research, industry shifts, and practical applications. These platforms allow you to exchange knowledge, ask questions, and collaborate with others.
- Reddit: Subreddits like r/MachineLearning and r/artificial offer discussions on the latest topics.
- Stack Overflow: Participate in Q&A sessions related to specific machine learning problems and challenges.
- Medium: Follow popular AI and ML writers who regularly post articles on recent trends and breakthroughs.
2. Follow Industry Leaders and Research Institutions
Tracking the work of influential figures and reputable research organizations can provide invaluable insights into cutting-edge technologies.
- Andrew Ng – Co-founder of Google Brain and Coursera, known for his contributions to machine learning.
- OpenAI – A leading AI research institute focused on creating general artificial intelligence for the benefit of humanity.
- MIT CSAIL – The Computer Science and Artificial Intelligence Laboratory, a hub for AI research.
"The world of AI is ever-changing. Staying updated means engaging in continuous learning and collaboration."
3. Participate in Workshops, Webinars, and Conferences
Attending industry events is another excellent way to keep up with the latest trends. These events often feature talks, hands-on sessions, and networking opportunities with experts in the field.
- Join workshops hosted by tech companies like Google, Microsoft, or IBM.
- Attend AI-focused conferences such as NeurIPS, ICML, or CVPR to explore new research and network with professionals.
4. Use Educational Platforms and Resources
Several platforms provide courses and tutorials tailored to the latest developments in AI and machine learning. These resources allow learners to gain new skills and stay updated in a structured environment.
Platform | Course Focus | Frequency of Updates |
---|---|---|
Coursera | AI, ML, Deep Learning | Regular updates with new courses |
edX | AI, Data Science, Robotics | New content added quarterly |
Udacity | AI, Data Engineering, Robotics | Updated frequently with industry trends |
By implementing these strategies, professionals can ensure they remain knowledgeable and competitive in the ever-evolving AI and ML landscapes.
Building a Portfolio: Showcasing Your DIY AI Projects to Potential Employers
Creating a strong portfolio is an essential part of demonstrating your AI skills to potential employers. As you work through personal AI projects, you should document your process, results, and challenges in a professional manner. By showcasing these projects in a clear and organized way, you allow employers to see your problem-solving skills, technical expertise, and creativity in action.
To make your portfolio stand out, focus on showcasing a variety of AI applications. Whether you’re working on machine learning models, neural networks, or natural language processing projects, each project should highlight a different skill set. It's important to include detailed explanations, code snippets, and visualizations of the results, so that potential employers can fully understand your abilities.
Key Elements of a Strong AI Portfolio
- Project Descriptions: Provide a brief overview of the project goals, your methodology, and the results achieved.
- Code and Repositories: Link to GitHub or similar repositories where your code is publicly available. This gives employers insight into your coding style and problem-solving approach.
- Visualization of Results: Include charts, graphs, or visualizations that help explain the outcomes of your models. This makes your projects more accessible and easier to understand.
- Challenges and Solutions: Describe any obstacles you faced during the project and how you overcame them. This demonstrates your ability to solve complex problems.
"A well-organized portfolio not only shows your technical expertise, but also demonstrates your ability to communicate complex ideas effectively."
Portfolio Structure: Essential Sections
Section | Details |
---|---|
Introduction | Brief background, including your learning journey and motivation behind pursuing AI. |
Project Showcase | A collection of your most relevant and impressive AI projects, with descriptions and links to code. |
Skills and Tools | List of AI-related skills and tools you have mastered, such as Python, TensorFlow, and specific algorithms. |
Contact Information | Provide an easy way for potential employers to reach out to you, such as LinkedIn or email. |
Tips for Refining Your AI Portfolio
- Consistency: Ensure that all project descriptions and code follow a consistent format, making it easy for recruiters to navigate.
- Highlight Results: Focus on how your projects led to measurable outcomes, such as improved model accuracy or successful implementation.
- Update Regularly: As you learn new techniques or complete additional projects, keep your portfolio up-to-date to reflect your growing expertise.