Ai Analytics Tools
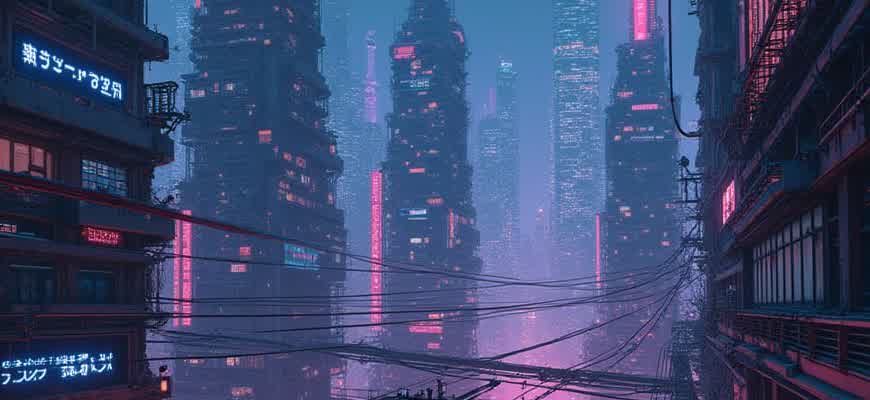
Artificial intelligence has revolutionized data analysis by providing powerful tools that enhance decision-making capabilities. These advanced systems are designed to process large volumes of data, uncovering insights that would be difficult or impossible for traditional methods to identify. With the ability to automate complex tasks, AI analytics tools are increasingly utilized across various industries.
Key benefits of AI-driven analysis include:
- Automation: AI can quickly process and interpret vast datasets without human intervention.
- Scalability: As data volumes grow, AI tools scale effortlessly to handle increased workloads.
- Predictive Insights: AI algorithms can forecast trends based on historical data, offering a competitive edge.
- Data Visualization: Advanced tools can present data in intuitive and interactive formats, making insights more accessible.
Among the tools available, some stand out for their unique capabilities:
- Machine Learning Platforms: These tools employ algorithms to detect patterns and predict outcomes.
- Natural Language Processing (NLP) Tools: These allow users to analyze unstructured data such as text and speech.
- AI-Enhanced Data Visualization Tools: Provide interactive dashboards and real-time analytics for quick decision-making.
"AI analytics tools are not just about improving efficiency but also about enabling smarter, data-driven decisions that lead to innovation and competitive advantage."
When evaluating AI analytics tools, it is crucial to consider their key features, such as:
Feature | Description |
---|---|
Data Integration | Ability to integrate various data sources into a unified platform for analysis. |
Real-Time Analytics | Providing instant insights from live data streams. |
Automation | Automating repetitive tasks to save time and improve accuracy. |
AI Tools for Data-Driven Decision Making
Artificial intelligence (AI) is transforming how businesses handle and analyze large volumes of data. By leveraging machine learning algorithms and data mining techniques, AI analytics tools provide actionable insights that help organizations make informed decisions quickly. These tools can automate data processing, detect patterns, and predict trends with remarkable accuracy. As a result, businesses gain a competitive edge in a data-driven world.
AI-powered platforms offer a range of features designed to enhance decision-making processes. From predictive analytics to real-time dashboards, these tools enable organizations to uncover hidden opportunities, identify risks, and optimize their strategies. By integrating AI into the analytics workflow, businesses can make faster, more accurate decisions based on data rather than intuition or guesswork.
Key Benefits of AI Analytics Tools
- Faster Decision Making: AI tools can process and analyze large datasets in seconds, enabling quicker responses to market changes.
- Predictive Insights: With machine learning algorithms, businesses can forecast trends and make proactive decisions before issues arise.
- Data-Driven Strategies: AI enhances the ability to base decisions on concrete data, minimizing the reliance on subjective judgment.
- Personalized Recommendations: AI tools can tailor suggestions and strategies based on individual business needs and customer behavior.
How AI Analytics Tools Improve Business Operations
- Data Collection and Cleaning: AI can automate the process of data aggregation and cleaning, ensuring that the datasets used for analysis are accurate and up-to-date.
- Trend Analysis: AI models can analyze historical data to identify patterns and trends, helping businesses understand past behavior and predict future outcomes.
- Real-Time Analytics: These tools provide instant analysis, allowing decision-makers to act quickly in a fast-paced environment.
AI analytics tools enable businesses to make data-driven decisions with high accuracy, reducing the risk of human error and bias.
Comparison Table: Popular AI Analytics Tools
Tool | Features | Best For |
---|---|---|
Google Cloud AI | Machine learning, data visualization, predictive analytics | Businesses looking for an integrated cloud-based solution |
IBM Watson | Natural language processing, AI-driven insights, automation | Enterprises requiring advanced AI capabilities for customer service |
Microsoft Azure AI | Custom AI models, deep learning, real-time data processing | Companies focused on scalable AI solutions for various industries |
Choosing the Right AI Analytics Tool for Your Business Needs
When selecting an AI-driven analytics platform, understanding your business goals and data complexity is crucial. The right tool will enable you to streamline processes, gain valuable insights, and make data-driven decisions. With a variety of options available, it's essential to assess each tool's capabilities, scalability, and alignment with your specific needs.
First, determine the primary use case for AI analytics in your business. Whether you're looking for real-time insights, predictive analytics, or automated reporting, the tool you choose should support those objectives efficiently. In addition, consider the level of expertise your team has, as some platforms may require advanced knowledge to implement and maintain.
Key Factors to Consider
- Scalability: Can the platform handle growing amounts of data as your business expands?
- Ease of Use: Does the tool offer a user-friendly interface or require extensive technical skills?
- Integration: How well does the tool integrate with your existing data sources and business systems?
- Cost: Does the pricing structure align with your budget and expected ROI?
Steps to Select the Ideal Tool
- Assess Your Data Needs: Identify the type and volume of data you need to analyze, and determine if the tool can handle it.
- Evaluate Features: Compare the features that matter most to your business, such as machine learning capabilities, reporting tools, or forecasting accuracy.
- Test the Platform: Many AI analytics platforms offer trials. Take advantage of these to test if the tool meets your expectations.
- Seek Feedback: Check reviews and case studies from other businesses in your industry to gauge the tool’s effectiveness.
Important: Choosing the right tool is a strategic decision that can impact your business growth. Prioritize features that support long-term goals, not just immediate needs.
Comparison of Popular Tools
Tool | Key Features | Ideal Use Case |
---|---|---|
Tool A | Real-time analytics, predictive modeling | Large enterprises with complex data streams |
Tool B | Automated reporting, easy integration | Small to medium-sized businesses |
Tool C | Advanced machine learning, customizable dashboards | Tech-focused businesses with deep data science needs |
How AI-Powered Tools Enhance Customer Segmentation and Targeting
Artificial intelligence (AI) plays a crucial role in revolutionizing customer segmentation and targeting by processing vast amounts of data quickly and accurately. Traditional methods often involve manual analysis of customer profiles, which can be time-consuming and prone to errors. AI, however, automates the process, providing businesses with a more efficient way to divide their audience into distinct groups based on specific attributes.
AI-powered analytics tools use advanced algorithms to identify patterns and trends in customer behavior, preferences, and demographics. This data-driven approach allows businesses to refine their marketing strategies and create more personalized experiences for their target audiences. The benefits of using AI in customer segmentation are clear: improved targeting, higher engagement rates, and increased conversion rates.
How AI Improves Customer Segmentation
AI enhances segmentation by analyzing large datasets and creating dynamic, accurate customer profiles. This goes beyond the traditional approach of grouping customers by age or location. AI tools consider a range of factors, such as purchasing behavior, online activity, and interactions with the brand. The result is highly granular segmentation that can target specific needs and preferences.
- Behavioral Segmentation: AI analyzes purchasing history, browsing behavior, and engagement patterns to group customers based on actions and interests.
- Predictive Segmentation: Machine learning algorithms predict future behavior, allowing businesses to segment customers by potential lifetime value or likelihood to purchase.
- Dynamic Segmentation: Unlike traditional methods, AI enables real-time adjustments to segments, reflecting changing behaviors and market conditions.
Effective Targeting with AI
Once customers are segmented, AI tools help to refine targeting efforts by ensuring that marketing messages are tailored to the right audience. AI enables businesses to optimize ad placement, email campaigns, and content delivery for each segment, increasing the likelihood of positive responses and higher conversion rates.
- Personalized Recommendations: AI systems recommend products or services based on customer profiles, enhancing personalization in marketing efforts.
- Real-Time Adjustments: AI allows for dynamic adjustments to marketing campaigns based on real-time data and customer responses.
- Cross-Channel Optimization: AI ensures consistent targeting across different marketing channels, including email, social media, and display ads.
Key Benefits of AI in Customer Segmentation and Targeting
Benefit | Description |
---|---|
Enhanced Accuracy | AI tools provide more precise segmentation, reducing the risk of misclassifying customers. |
Real-Time Insights | AI allows businesses to adapt their marketing strategies in real-time based on current data. |
Scalability | AI-driven systems can handle vast amounts of data, making segmentation scalable even for large customer bases. |
AI analytics tools provide a level of precision and adaptability that traditional segmentation methods simply cannot match.
Integrating AI-Powered Insights into Legacy Data Systems
Integrating AI-based analytics tools into existing data infrastructures allows organizations to harness the power of advanced machine learning models without having to overhaul their entire IT architecture. This integration aims to enhance decision-making by providing predictive insights, automated reporting, and deeper analysis of complex data sets. As companies increasingly rely on data-driven strategies, embedding AI capabilities within traditional systems is becoming a priority for maintaining competitive advantage.
To achieve a seamless integration, several key steps are required, including identifying suitable AI tools, ensuring data compatibility, and setting up a feedback loop for continuous improvement. Below are important considerations when integrating AI into current data systems:
Steps for Successful AI Integration
- Assessment of Current Infrastructure: Evaluate the readiness of your existing systems, including hardware, data storage, and processing capacity.
- Selection of AI Tools: Choose AI tools that align with the company's specific analytical needs, such as predictive analytics, natural language processing, or anomaly detection.
- Data Preparation: Clean, transform, and normalize data to ensure compatibility with AI models.
- Integration and Testing: Implement AI solutions and conduct rigorous testing to identify potential issues, such as data inconsistencies or performance bottlenecks.
- Ongoing Monitoring and Optimization: Continuously track performance metrics and fine-tune AI models for better accuracy and efficiency.
Challenges and Considerations
Integrating AI into legacy systems can be challenging due to issues like data silos, lack of interoperability between old and new technologies, and the need for specialized skills in AI implementation.
Despite the advantages, companies may face several challenges during the integration process, such as maintaining data consistency across various platforms, managing the complexity of merging AI tools with existing applications, and addressing concerns regarding data privacy and security. To mitigate these risks, it's essential to invest in staff training, ensure robust data governance policies, and prioritize scalability in AI tool selection.
Key Metrics for Evaluating AI Integration Success
Metric | Importance |
---|---|
Data Accuracy | Ensures the AI models provide reliable insights based on clean and structured data. |
Integration Speed | Measures how quickly AI tools can be incorporated into existing systems without significant downtime. |
ROI of AI Implementation | Tracks the financial impact of AI on decision-making efficiency, cost savings, and new revenue generation. |
Automating Data Collection and Analysis with AI
Automation of data gathering and analysis has become an essential aspect of modern business operations. AI-driven tools allow organizations to streamline these processes, reducing the time and resources needed to collect and interpret large datasets. Machine learning models, for example, can extract insights from complex data sets that would be too time-consuming or costly for humans to analyze manually.
By integrating artificial intelligence into data workflows, companies can enhance the accuracy and speed of decision-making. AI enables continuous, real-time monitoring of data, ensuring that important insights are not missed and that businesses can respond quickly to changing conditions. Moreover, AI tools are capable of identifying patterns and trends that might otherwise remain hidden in large volumes of unstructured data.
Key Benefits of Automating Data Collection and Analysis
- Speed: AI can process and analyze data much faster than manual methods, allowing businesses to make timely decisions.
- Accuracy: Machine learning models can reduce human error, providing more reliable insights.
- Scalability: AI tools can handle vast amounts of data, scaling effortlessly as businesses grow.
How AI Tools Enhance Data Analysis
- Data Cleansing: AI algorithms automatically detect and correct inconsistencies or errors in the data, ensuring that analysis is based on high-quality information.
- Predictive Analytics: By applying machine learning models, AI can predict future trends and behaviors, enabling proactive strategies.
- Real-time Insights: AI-powered systems can deliver live data analysis, providing immediate feedback for faster decision-making.
"AI tools don't just automate repetitive tasks, they transform the way businesses interact with data, driving innovation and enabling smarter, faster decisions."
Example of AI-Powered Data Analysis Workflow
Step | AI Role |
---|---|
Data Collection | AI tools gather data from multiple sources (web, IoT devices, social media). |
Data Cleaning | AI algorithms remove noise and correct inconsistencies. |
Data Analysis | AI models analyze data and extract actionable insights. |
Prediction | AI forecasts trends and outcomes based on historical data. |
Real-Time Analytics: Unlocking Instant Insights for Your Business
In today’s fast-paced market, the ability to act on insights immediately can be the key to staying competitive. Real-time analytics enables businesses to make data-driven decisions on the fly, optimizing processes, improving customer experiences, and responding swiftly to market changes. By continuously analyzing data as it streams in, companies can identify trends and opportunities faster than ever before.
Adopting real-time data analytics tools is becoming increasingly vital across industries. These solutions allow businesses to monitor key metrics instantly, track operational performance, and respond proactively. Real-time insights eliminate the need for delayed reporting and provide a competitive edge by ensuring that data-driven actions are taken without the waiting period often associated with traditional reporting tools.
Key Benefits of Real-Time Analytics
- Enhanced Decision Making: With live data at your fingertips, decisions are based on the most current and relevant information.
- Operational Efficiency: Monitor processes and detect inefficiencies in real-time, allowing for immediate adjustments.
- Improved Customer Experience: Address customer needs or issues as they arise, leading to better satisfaction and loyalty.
- Market Adaptability: Quickly react to market trends or competitor actions, staying ahead of the curve.
How Real-Time Analytics Works
Real-time analytics tools rely on advanced technologies like stream processing, machine learning, and AI to collect, analyze, and visualize data as it’s generated. Data from various sources, such as sensors, social media, and transactions, is processed instantly, providing businesses with actionable insights in seconds. These insights can then be delivered through dashboards, reports, or even integrated directly into business workflows.
"Real-time data empowers businesses to make smarter decisions quickly and adapt to changing conditions with agility."
Example of Real-Time Analytics in Action
Industry | Use Case | Benefit |
---|---|---|
E-Commerce | Tracking customer behavior on the website | Personalized recommendations and offers in real-time |
Healthcare | Monitoring patient vitals remotely | Immediate response to any critical changes in health |
Manufacturing | Monitoring equipment performance on the factory floor | Preventive maintenance and reduced downtime |
Implementation Considerations
- Data Quality: Real-time analytics is only as good as the data it processes. Ensure data is clean, accurate, and reliable.
- Integration: The system must seamlessly integrate with your existing data infrastructure and workflows for maximum effectiveness.
- Scalability: As your business grows, your analytics tools should be able to scale to handle larger volumes of data.
Predictive Analytics: Harnessing AI for Long-Term Business Success
Predictive analytics has emerged as a transformative tool for businesses seeking to stay ahead of the curve. By leveraging advanced AI algorithms, companies can predict future trends, customer behaviors, and potential challenges with remarkable accuracy. This approach not only allows for more informed decision-making but also enables businesses to align their strategies with market demands and anticipate shifts before they occur.
The integration of AI in predictive analytics can create future-proof strategies by providing data-driven insights into business operations. By analyzing historical data, AI models can forecast outcomes, making it easier for companies to optimize resources, manage risks, and make proactive adjustments. The predictive power of AI offers a competitive advantage, allowing businesses to adapt quickly to changes in consumer preferences, technological advancements, and economic conditions.
Key Benefits of Predictive Analytics for Strategy Development
- Improved Decision-Making: AI enables businesses to make decisions based on data-driven forecasts rather than intuition alone.
- Risk Mitigation: Identifying potential risks before they materialize allows companies to develop strategies to mitigate negative impacts.
- Operational Efficiency: Predictive insights optimize business operations by forecasting demand, inventory needs, and workforce allocation.
How Predictive Models Drive Business Strategy
- Data Collection: Gather and analyze historical data to identify patterns and correlations.
- Model Development: Develop AI models to forecast trends, customer behavior, and potential risks.
- Continuous Monitoring: Regularly update predictive models with fresh data to maintain their accuracy and relevance.
- Strategy Formulation: Use insights from the models to inform long-term strategic decisions and prepare for future market dynamics.
"By adopting predictive analytics, businesses can not only forecast future trends but also create agile strategies that evolve with changing market conditions."
AI-Driven Predictive Analytics in Action
Area of Application | Impact of AI |
---|---|
Customer Insights | AI predicts customer preferences, improving targeting and personalization. |
Supply Chain Optimization | AI forecasts demand and supply fluctuations, reducing waste and improving efficiency. |
Market Forecasting | AI analyzes economic indicators and market trends, helping businesses stay ahead of market changes. |
Scaling AI-Driven Analytics Across Various Organizational Units
Integrating AI-powered analytics tools into an organization requires careful planning, especially when extending their use across different departments. Each department may have unique goals and operational needs, making it crucial to customize the deployment of AI solutions. By tailoring these tools, organizations can ensure more efficient data-driven decision-making across all areas, from marketing to human resources, finance, and beyond.
For successful scaling, it is essential to establish a strong infrastructure that supports AI integration at every level. This involves aligning tools with specific departmental KPIs, ensuring seamless data flow, and enabling collaboration between departments. Additionally, understanding the capabilities of AI in each context allows departments to leverage the technology for the best outcomes.
Key Steps to Scale AI Analytics Tools
- Identify Departmental Needs: Understand the unique requirements of each department, from customer insights in marketing to inventory management in logistics.
- Develop a Centralized Data Strategy: Establish a unified data framework that ensures consistent data flow and quality across all departments.
- Customize AI Solutions: Tailor AI tools to address specific departmental challenges, ensuring they add value in each context.
- Ensure Cross-Departmental Collaboration: Foster communication between teams to share insights and optimize the AI solution’s effectiveness.
Implementation Best Practices
- Start with a Pilot Program: Implement AI tools in a single department or a limited scope, evaluate performance, and scale gradually.
- Invest in Training and Support: Equip staff with the necessary skills to use AI tools effectively through training and ongoing support.
- Monitor and Optimize Performance: Continuously track the performance of AI solutions and refine them based on feedback and evolving departmental goals.
Scaling AI tools successfully requires both technical infrastructure and cultural alignment across departments. Collaboration and consistent monitoring are essential to maximize the value of AI analytics.
Example of AI Analytics Tools in Different Departments
Department | AI Analytics Use Case | Benefit |
---|---|---|
Marketing | Customer segmentation and targeting | Improved campaign ROI through personalized messaging |
HR | Employee sentiment analysis | Enhanced employee retention and engagement |
Finance | Predictive financial modeling | Better financial forecasting and risk management |