Ai Orchestration and Automation Platform
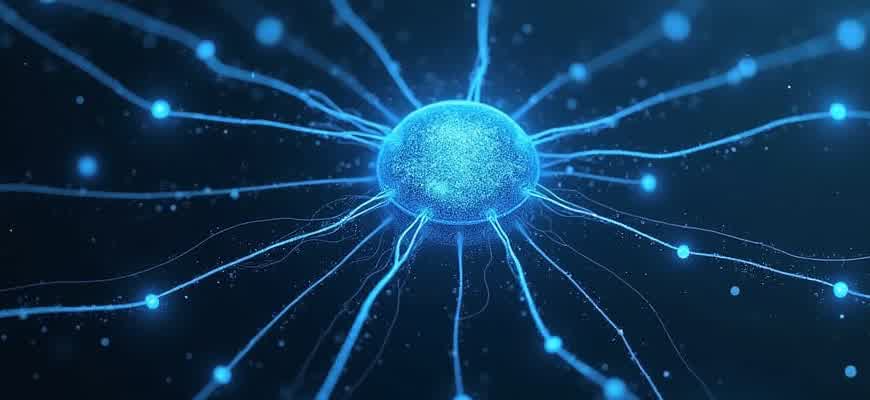
The integration of artificial intelligence (AI) in orchestration and automation platforms has revolutionized the way businesses streamline their processes. These advanced systems utilize AI to improve decision-making, optimize workflows, and reduce human intervention in complex tasks. By automating repetitive and time-consuming activities, organizations can achieve higher efficiency and scalability across various operations.
Key Benefits of AI-Enhanced Automation Platforms:
- Improved Operational Efficiency: Automating manual processes reduces errors and speeds up execution times.
- Cost Savings: By minimizing the need for human resources in routine tasks, businesses can lower operational costs.
- Data-Driven Insights: AI systems analyze data in real-time, providing actionable insights that guide business decisions.
- Scalability: AI-based automation platforms can scale with the growing demands of a business, adapting to new challenges seamlessly.
How AI Orchestration Works:
- AI algorithms monitor business processes and identify areas for improvement.
- Automated tasks are scheduled and executed based on predefined criteria.
- Data is continuously analyzed to optimize future workflows and decision-making.
- AI systems provide recommendations for improvements, helping organizations stay ahead of competition.
"AI-powered automation platforms enable organizations to not only increase efficiency but also make intelligent decisions that drive long-term growth."
Table: Comparison of Traditional vs AI-Oriented Automation
Feature | Traditional Automation | AI-Driven Automation |
---|---|---|
Flexibility | Limited adaptability | Highly adaptive to changing conditions |
Data Utilization | Static rule-based | Dynamic real-time data analysis |
Scalability | Requires manual updates | Scales effortlessly with demand |
Cost Efficiency | High operational costs | Reduces costs by eliminating redundant tasks |
AI-Driven Workflow and Automation Solutions
The evolution of AI-powered platforms has significantly impacted various industries by enabling intelligent orchestration and automated workflows. These platforms are designed to integrate multiple systems, processes, and data sources, creating seamless interactions that reduce manual intervention. Businesses can leverage these solutions to optimize complex operations, ensuring improved efficiency, reduced operational costs, and faster decision-making.
AI-based orchestration platforms use advanced algorithms to automate and manage business workflows. By orchestrating tasks across different departments and systems, these platforms facilitate a more fluid, dynamic environment where the automation of repetitive tasks becomes a key driver of productivity. AI-powered systems not only streamline operations but also provide actionable insights that help in strategic decision-making.
Core Capabilities of AI-Oriented Automation Platforms
- Automation of Routine Tasks: AI platforms can automate repetitive tasks such as data entry, scheduling, and reporting, reducing human error and time spent on manual processes.
- Advanced Process Orchestration: The platforms allow seamless coordination between different tools and departments, enhancing operational flow without constant oversight.
- Data-Driven Insights: AI algorithms process large datasets to provide insights that can predict trends, identify bottlenecks, and offer optimization strategies.
Key Benefits
- Increased Efficiency: With AI handling routine tasks, employees can focus on more strategic and creative aspects of their roles.
- Cost Reduction: Automation eliminates the need for manual labor in routine processes, cutting down operational expenses.
- Scalability: AI platforms are scalable, enabling businesses to expand their automation processes as they grow, without additional resources.
Platform Architecture
Component | Description |
---|---|
Data Integration Layer | Facilitates the connection of various data sources to enable seamless data flow across the system. |
AI Engine | Utilizes machine learning and predictive analytics to process data, identify patterns, and automate decision-making. |
Task Orchestrator | Coordinates and automates workflows between departments, systems, and processes. |
"AI-based platforms empower businesses to not only automate tasks but also gain valuable insights from their operations, leading to smarter decision-making and optimized workflows."
How AI Integration Enhances Cross-Departmental Collaboration
AI-driven orchestration platforms are revolutionizing the way departments interact, streamlining processes, and improving overall efficiency. By automating repetitive tasks and providing real-time insights, these systems create a cohesive environment that fosters seamless collaboration across different teams. The use of AI reduces the need for manual interventions and minimizes bottlenecks, leading to faster decision-making and smoother transitions between departments.
Through advanced analytics and machine learning, AI platforms can predict potential issues and optimize workflows by assigning tasks based on real-time data. This enhances cross-functional coordination, ensuring that all teams, from marketing to customer support, can work in sync without delay. By connecting disparate systems and providing an intuitive interface, AI orchestration acts as a bridge between departments, facilitating better communication and reducing friction.
Key Benefits of AI Orchestration for Departmental Workflows
- Increased Efficiency: By automating routine tasks, AI systems free up time for employees to focus on higher-value activities, improving overall productivity.
- Faster Decision-Making: Real-time data analytics allow for quicker identification of issues, enabling departments to make informed decisions faster.
- Enhanced Collaboration: AI platforms integrate various departmental tools, ensuring that teams can access the same data and communicate effectively.
How AI Improves Workflow Automation Across Teams
- Centralized Data Access: AI platforms consolidate data from different sources, ensuring that all departments have access to up-to-date and relevant information.
- Task Assignment Optimization: By analyzing employee availability and task priorities, AI can automatically assign tasks to the right individuals, reducing human error.
- Continuous Monitoring: AI tools monitor workflows in real-time, alerting teams to potential delays or issues before they become critical.
"AI orchestration creates a unified ecosystem where each department is empowered with the tools they need to collaborate effectively and deliver results faster."
Department | Primary Benefit | AI Functionality |
---|---|---|
Sales | Faster Lead Conversion | Automated lead scoring and prioritization |
Marketing | Enhanced Campaign Effectiveness | Real-time analytics and content optimization |
Support | Improved Response Times | AI-driven chatbots and issue routing |
Automating Repetitive Tasks with AI: A Step-by-Step Approach
In the fast-paced digital landscape, businesses often struggle with repetitive tasks that consume valuable time and resources. Artificial Intelligence (AI) offers a powerful solution to streamline these processes, ensuring efficiency and accuracy. By integrating AI into workflows, companies can automate routine activities, allowing employees to focus on more strategic tasks that drive growth.
This step-by-step guide outlines the key processes involved in leveraging AI for task automation. From identifying suitable processes to implementing AI-driven solutions, each phase ensures maximum productivity gains and smoother operations.
Step 1: Identify Repetitive Tasks
The first step in automation is recognizing tasks that can benefit from AI. Focus on those that are high-frequency, rule-based, and require minimal human decision-making. Common examples include data entry, report generation, and customer service inquiries.
- Data entry tasks
- Email management
- Customer support queries
- File organization
Step 2: Select the Right AI Tools
Once tasks are identified, the next step is choosing the appropriate AI tools. These tools can range from simple bots to more advanced machine learning systems, depending on the complexity of the tasks.
- Robotic Process Automation (RPA): Ideal for tasks that involve structured data and fixed processes.
- Natural Language Processing (NLP): Best for automating text-based interactions, such as customer service chats.
- Machine Learning Algorithms: Useful for tasks that require learning from data and improving over time.
Step 3: Implement and Monitor
After selecting the right tools, it’s time to implement them into existing workflows. This may involve integrating AI solutions with legacy systems or using cloud-based platforms for faster deployment.
Task | AI Solution | Expected Outcome |
---|---|---|
Data Entry | RPA | Reduced errors and time spent on manual input. |
Customer Support | NLP | Faster response times and improved customer satisfaction. |
Analytics | Machine Learning | Automated insights and data-driven decision-making. |
Tip: Monitor AI performance regularly to ensure the system adapts to new scenarios and continuously improves its efficiency.
Integrating AI-Driven Workflow Management with Existing Enterprise Infrastructure
For organizations to leverage AI-driven automation effectively, integration with existing business systems is essential. The success of AI orchestration platforms largely depends on how seamlessly they can interface with current IT ecosystems, including databases, enterprise resource planning (ERP), customer relationship management (CRM), and legacy software systems. These integrations enable AI to process and analyze data across the organization in real-time, ensuring that automated processes align with business objectives and operational needs.
Effective integration involves mapping existing workflows, identifying points of automation, and selecting appropriate AI tools that can operate in conjunction with these systems. For companies to achieve a smooth transition, they must focus on maintaining system compatibility, ensuring data flow between platforms is uninterrupted, and managing change without disrupting ongoing business processes.
Key Strategies for Integration
- Data Mapping: Identifying which data needs to be shared between AI systems and existing platforms to ensure automation accuracy.
- API Connections: Using application programming interfaces (APIs) to connect AI tools with enterprise systems without requiring full system overhauls.
- Scalability Considerations: Ensuring AI orchestration platforms can scale up as data and business requirements evolve.
Best Practices for Seamless Integration
- Start with a pilot: Test AI orchestration tools in one part of the business to identify potential challenges before a full-scale implementation.
- Use modular architectures: Implement AI automation in stages, allowing different components of the platform to be updated or replaced without disrupting other areas.
- Ensure real-time monitoring: Continuously track the performance of AI systems to address issues before they affect business operations.
Challenges to Consider
Challenge | Impact | Solution |
---|---|---|
Data Silos | Restricted flow of information between departments | Integrate data sources using centralized platforms or cloud systems |
System Compatibility | Older systems may not support modern AI tools | Gradual migration with legacy system support |
Security Risks | AI systems introducing vulnerabilities | Implement strong data protection protocols and access control |
Note: For a truly successful AI integration, it is crucial to maintain the balance between automation and human oversight to prevent errors and ensure the AI system aligns with evolving business goals.
Improving Decision-Making with Real-Time Data Automation
The integration of real-time data automation significantly enhances the decision-making process by providing up-to-the-minute insights. By streamlining data flows from various sources, organizations can eliminate delays and react faster to changing conditions. Automation tools enable businesses to track key metrics automatically, ensuring that decision-makers have immediate access to the most relevant and accurate information available.
Automating data collection and processing allows for quicker identification of patterns and trends. This leads to more precise predictions and informed decisions, which is essential for businesses aiming to maintain a competitive edge. With real-time data automation, manual data gathering becomes obsolete, reducing human error and increasing overall efficiency.
Key Benefits of Real-Time Data Automation
- Faster Response Times: Immediate access to data allows organizations to respond to market shifts or operational issues as they occur.
- Improved Accuracy: Automation reduces human intervention, minimizing errors and ensuring the consistency of data processing.
- Better Resource Allocation: With real-time insights, organizations can allocate resources more effectively, prioritizing areas that need attention.
How Automation Supports Smarter Decision-Making
"By automating the data pipeline, businesses gain the ability to process vast amounts of information instantly, which enhances strategic and operational decision-making."
Automated systems provide a continuous flow of updated data, allowing for dynamic adjustments in decision-making processes. With predictive analytics integrated into automation platforms, businesses can anticipate future trends based on real-time information, improving overall strategic planning.
Real-Time Data Processing in Action
Process Step | Action | Impact on Decision-Making |
---|---|---|
Data Collection | Automated sensors and systems gather data continuously. | Ensures no critical information is missed. |
Data Analysis | Algorithms process and analyze incoming data in real-time. | Immediate insights lead to more accurate predictions. |
Decision Support | Decision-making platforms present actionable insights to managers. | Allows quicker, more informed decisions across various departments. |
Reducing Human Error and Increasing Accuracy with AI Automation
In today’s fast-paced and data-driven environment, human errors are inevitable, especially in complex tasks that require precision. AI-driven automation platforms offer a transformative solution by minimizing these mistakes through consistent, rule-based decision-making. Unlike human operators, AI systems follow predefined algorithms without emotional or cognitive biases, leading to more accurate and reliable results. This reduction in human error not only enhances efficiency but also reduces operational costs and time spent on corrections.
AI technologies, such as machine learning and natural language processing, enable automation platforms to analyze vast amounts of data, identify patterns, and make decisions with exceptional speed and accuracy. By integrating these technologies into processes, businesses can ensure that tasks are completed with minimal errors, allowing human workers to focus on more strategic or creative activities.
Key Benefits of AI Automation in Reducing Human Error
- Consistency: AI systems operate with the same efficiency and accuracy every time, eliminating the variability introduced by human fatigue or distraction.
- Faster Decision Making: AI can process data much quicker than humans, leading to faster and more accurate decisions.
- Real-time Error Detection: Automated systems can instantly identify discrepancies or deviations from expected outcomes, reducing the chances of errors going unnoticed.
AI’s Impact on Accuracy Across Industries
AI automation platforms are being widely adopted across industries to enhance accuracy. Below is an example of how AI improves precision in key sectors:
Industry | Application of AI | Accuracy Improvement |
---|---|---|
Healthcare | Diagnosis Support | Reduced diagnostic errors through AI-based imaging analysis |
Finance | Fraud Detection | Improved fraud detection with machine learning algorithms |
Manufacturing | Predictive Maintenance | Increased machine uptime by predicting failures with high accuracy |
Important: While AI automation greatly reduces human errors, it is crucial to ensure that algorithms are properly trained and continuously updated to adapt to changing conditions and data.
AI Platform Security: Best Practices for Protecting Your Data
Ensuring the security of data within AI orchestration and automation platforms is paramount, as these platforms often handle sensitive business information and complex algorithms. To maintain the confidentiality, integrity, and availability of data, organizations must adopt a layered approach to security, focusing on both technical and operational measures. The implementation of effective security protocols can significantly mitigate the risk of data breaches and system vulnerabilities.
AI platforms can be particularly susceptible to threats due to their reliance on vast datasets and interconnected systems. To address these risks, companies should follow a combination of best practices that span across access control, encryption, monitoring, and compliance. By focusing on securing data both at rest and in transit, organizations can safeguard against a wide range of cyber threats.
Key Security Practices for AI Platforms
- Access Control: Implement strict user authentication and role-based access controls (RBAC) to restrict access to sensitive data and system components.
- Data Encryption: Ensure that all data, whether stored or transmitted, is encrypted using strong encryption algorithms such as AES-256.
- Regular Audits and Monitoring: Conduct routine security audits and continuously monitor AI systems to detect anomalies or unauthorized access attempts.
- Data Anonymization: Use anonymization techniques to reduce the risk of exposing personal or sensitive information during data processing.
- Compliance with Standards: Adhere to industry standards and regulations (e.g., GDPR, CCPA) to ensure that AI platforms meet legal and privacy requirements.
Important Security Measures to Consider
Organizations should invest in continuous training for teams managing AI systems, as human error remains one of the leading causes of data security breaches.
- Network Security: Ensure that the network infrastructure is secure by using firewalls, intrusion detection systems (IDS), and regularly updating software to patch vulnerabilities.
- Backup and Recovery Plans: Establish comprehensive backup and disaster recovery plans to protect critical data from loss or corruption.
- Zero Trust Architecture: Adopt a zero-trust model where every user, device, and network request is verified, regardless of whether it's internal or external.
Security Measures Overview
Security Measure | Purpose |
---|---|
Encryption | Protects data confidentiality during storage and transmission. |
Access Control | Restricts system access based on user roles and authentication levels. |
Auditing and Monitoring | Helps detect unauthorized access and suspicious activities. |
Compliance | Ensures adherence to relevant privacy and security regulations. |
Assessing the Impact of AI-Driven Process Automation on Business ROI
Understanding the financial returns from implementing AI automation in business workflows is crucial for organizations aiming to optimize operations. Measuring ROI in this context involves evaluating both tangible and intangible benefits that AI systems bring to the table. The process begins by identifying the initial investment, including both the development and integration costs, as well as the operational expenses associated with AI tools and platforms.
Once the costs are accounted for, businesses need to measure the improvements AI brings, such as time saved, accuracy of automated tasks, and scalability of processes. This data provides a foundation for calculating the return on investment and helps businesses determine whether AI automation justifies the resources committed to it.
Key Factors in Measuring AI Automation ROI
- Cost Reduction: AI can significantly cut down labor costs by automating repetitive tasks, reducing the need for human intervention.
- Efficiency Gains: Automation allows businesses to streamline processes, leading to faster execution of tasks and higher throughput.
- Quality and Accuracy: AI systems can improve the precision of operations, reducing errors and enhancing product or service quality.
- Scalability: AI-driven platforms can scale quickly to handle increased workload without the need for proportional increases in resources.
Measuring ROI: A Step-by-Step Approach
- Define Metrics: Establish key performance indicators (KPIs) like reduced processing time, error rates, and labor costs saved.
- Calculate Direct Financial Gains: Quantify how much money is saved through reduced labor or operational costs, and track increased revenue from enhanced productivity.
- Account for Indirect Benefits: Consider the value of improved customer satisfaction, brand reputation, and scalability potential, which can lead to long-term financial growth.
- Monitor Long-term Performance: Continuously assess the system’s effectiveness, refining AI algorithms and optimizing processes to ensure sustained ROI over time.
"AI's impact on ROI goes beyond immediate savings; it involves a long-term transformation of how businesses function and scale." - Business Automation Expert
Example of ROI Calculation for AI Automation
Metric | Before AI | After AI | Improvement |
---|---|---|---|
Labor Costs | $500,000 | $200,000 | 60% reduction |
Processing Time | 48 hours | 12 hours | 75% reduction |
Error Rate | 5% | 0.5% | 90% reduction |
Revenue Growth | $1,000,000 | $1,500,000 | 50% increase |