Opencourseware Ai
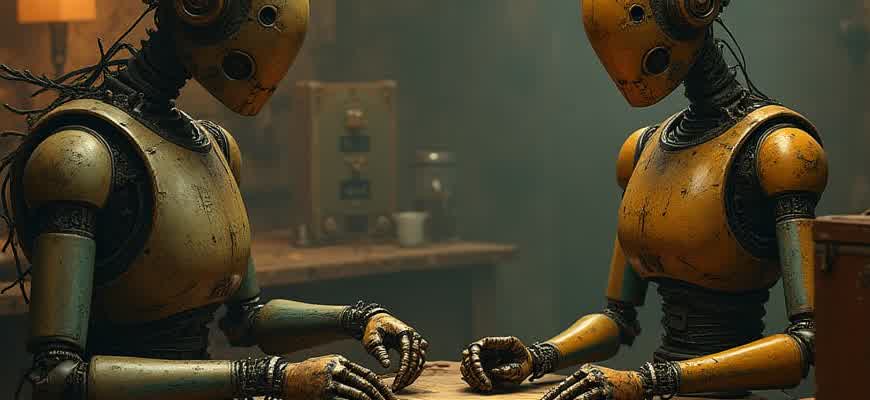
In recent years, the integration of Artificial Intelligence (AI) with OpenCourseWare (OCW) platforms has revolutionized online learning. These systems leverage machine learning algorithms to enhance the user experience, improve content personalization, and optimize educational processes. By analyzing learner data, AI technologies enable more adaptive and responsive course delivery, catering to the individual needs of students.
The impact of AI on OCW can be observed in several key areas:
- Content Personalization: AI tailors course materials based on learner progress and preferences.
- Automated Assessments: AI tools assist in grading assignments and quizzes, offering immediate feedback.
- Recommendation Systems: AI suggests courses and resources that align with a learner's previous interactions and interests.
"AI has the potential to create a more engaging, dynamic, and individualized learning experience that evolves with the student's needs."
These technologies work through continuous analysis of learner behavior, generating patterns that help adapt content, increase engagement, and track progress more efficiently. Here is a breakdown of how AI is integrated into OCW platforms:
AI Feature | Function |
---|---|
Machine Learning Algorithms | Analyze student data for personalized content recommendations and adaptive learning paths. |
Natural Language Processing | Enhance interaction with learning materials through speech recognition and text analysis. |
Automated Grading Systems | Instant feedback and evaluation for assignments, quizzes, and exams. |
AI-Driven OpenCourseWare: Maximizing the Potential of Modern Educational Tools
OpenCourseWare (OCW) has revolutionized how learners access high-quality education, offering free and open access to a wide variety of courses from top universities. With the integration of artificial intelligence (AI), OCW platforms can now provide an even more personalized and efficient learning experience. By leveraging AI technologies, educational content can be tailored to individual learner needs, enhancing engagement and improving learning outcomes.
This approach not only increases accessibility but also enriches the learning process. AI-powered systems can analyze student progress, recommend resources, and adapt to learning styles, making the educational journey smoother and more effective. Below, we explore how AI can be harnessed within OCW platforms to create smarter, more engaging learning environments.
AI Integration in OpenCourseWare Platforms
By integrating AI into OCW systems, educational platforms can automate various tasks and optimize learning pathways for users. Here are some examples of how AI can be leveraged:
- Personalized Learning Paths: AI can analyze individual progress and suggest tailored learning content, adjusting the difficulty level and types of resources based on student performance.
- Real-Time Feedback: AI-driven tools can assess student responses and provide instant feedback, helping learners identify strengths and areas for improvement.
- Enhanced Search and Recommendations: AI can refine content search capabilities, making it easier for students to find relevant material based on their specific needs.
Additionally, AI can support educators by automating administrative tasks, such as grading assignments or tracking student performance over time, allowing them to focus more on teaching and mentoring. With the implementation of AI, learning platforms can adapt more efficiently to a variety of learners' needs, fostering a more engaging and supportive environment.
Key Benefits of AI in OCW
Benefit | Description |
---|---|
Scalability | AI allows for massive scaling of personalized education, enabling platforms to serve thousands of students simultaneously with custom-tailored learning experiences. |
Efficiency | Automation of grading, feedback, and content recommendations saves time for both educators and learners, improving overall efficiency in the learning process. |
Data-Driven Insights | AI tools provide valuable insights into student behavior, enabling educators to make informed decisions about curriculum design and student support strategies. |
AI technology empowers both educators and learners by delivering a dynamic and adaptive learning environment that evolves with the needs of the student.
In conclusion, integrating AI into OpenCourseWare platforms holds immense potential for transforming how we learn. By leveraging intelligent systems that cater to individual needs and automate time-consuming tasks, education becomes more efficient, accessible, and personalized than ever before.
Understanding AI in OpenCourseWare and How It Functions
AI-integrated OpenCourseWare (OCW) refers to the application of artificial intelligence technologies in open educational resources. These resources are freely accessible, providing students with comprehensive course materials across various subjects. The incorporation of AI aims to personalize the learning experience, making educational content more dynamic and adaptable to individual needs.
AI-based OCW platforms can enhance learning by utilizing algorithms that adapt content, assess student progress, and even recommend specific resources based on learner behavior. By doing so, they offer a more tailored educational experience compared to traditional static learning methods.
How AI Enhances OpenCourseWare
AI-powered features in OpenCourseWare include:
- Personalized learning paths: AI analyzes user behavior to suggest tailored courses and resources.
- Smart assessments: Automated grading systems and quizzes provide real-time feedback to learners.
- Content recommendations: AI can suggest supplementary materials based on learning progress and interests.
Key Features and Functionalities
Feature | Functionality |
---|---|
Adaptive Content | Content that changes based on user preferences and learning pace. |
Automated Feedback | Real-time evaluation of learner performance with suggestions for improvement. |
Intelligent Tutoring | AI-driven tutors that guide students through complex concepts. |
"The future of education lies in adaptive learning technologies that make learning more engaging and effective."
How AI in OpenCourseWare Can Transform Online Learning Platforms
AI-powered OpenCourseWare (OCW) has the potential to reshape the landscape of online education by providing personalized learning experiences. By integrating advanced algorithms and machine learning models, OCW systems can analyze student data to tailor content and assessments to individual needs. This approach enhances learning outcomes, making education more accessible and efficient for diverse learner groups across the globe.
Moreover, AI can automate many administrative tasks such as grading, feedback, and course management, reducing the workload on instructors and allowing them to focus on more strategic tasks like content creation and engagement. This transformation is critical as it opens doors to greater scalability and consistency in delivering high-quality education at a fraction of the cost of traditional methods.
Key Benefits of AI Integration in OpenCourseWare
- Personalized Learning: AI adapts the course content based on each student’s progress and learning style, ensuring a tailored educational experience.
- Automation of Administrative Tasks: AI systems can handle grading, assessments, and feedback, minimizing human error and freeing up time for instructors.
- Data-Driven Insights: Advanced analytics help instructors understand student performance and adjust course content in real-time.
- 24/7 Availability: AI-based systems can be accessed at any time, providing students with continuous support outside of scheduled class hours.
AI Tools for Enhanced Interaction
- Chatbots: AI-driven chatbots provide instant answers to student queries, reducing wait times and improving engagement.
- Recommendation Systems: AI suggests relevant resources or courses based on students' past interactions and interests.
- Virtual Tutors: AI tutors offer real-time guidance and personalized learning tips for students struggling with specific topics.
"AI in OpenCourseWare can break down the barriers of traditional education, allowing for more flexible, adaptive, and scalable learning environments."
Comparison: Traditional vs AI-Enhanced Online Learning
Feature | Traditional Learning | AI-Powered OCW |
---|---|---|
Content Customization | Generic, one-size-fits-all | Personalized to student progress |
Student Support | Limited to office hours | 24/7 through AI chatbots and tutors |
Feedback Speed | Delayed, dependent on instructor | Instant feedback from AI systems |
Scalability | Limited by instructor availability | Scalable across thousands of students simultaneously |
Practical Use Cases: Incorporating AI into OpenCourseWare for Course Development
Integrating AI technologies into OpenCourseWare (OCW) platforms offers powerful opportunities for enhancing the quality and accessibility of course materials. By leveraging AI, course developers can automate content creation, streamline feedback processes, and enhance personalized learning experiences. These advancements not only save time but also improve the overall efficiency and interactivity of educational resources, benefiting both instructors and students alike.
The application of AI in course development can transform how educational content is designed and delivered. By utilizing advanced algorithms, AI tools can analyze student performance, identify knowledge gaps, and provide real-time suggestions for improving the curriculum. This allows for a more dynamic and adaptive learning environment, where courses can evolve based on actual student needs and preferences.
Key Use Cases of AI in OpenCourseWare Course Development
- Automated Content Creation: AI can generate quizzes, assignments, and even lecture materials based on existing course frameworks, reducing the time and effort needed by instructors.
- Personalized Learning Paths: AI can track individual progress and recommend customized resources, creating a tailored learning experience for each student.
- Real-time Assessment and Feedback: AI-powered tools can provide instant feedback on student submissions, identifying areas for improvement and suggesting corrective actions.
By implementing AI in course design, educators can create more engaging, flexible, and effective learning environments that cater to diverse student needs.
Examples of AI Tools in Course Development
AI Tool | Use Case |
---|---|
Natural Language Processing (NLP) | Enhancing text-based materials by analyzing and summarizing content for easier comprehension. |
Machine Learning Algorithms | Identifying learning patterns and adapting course content to suit individual student needs. |
Chatbots | Providing real-time assistance to students and answering frequently asked questions. |
Benefits for Educators and Students
- Time Efficiency: Automating repetitive tasks like grading and content generation frees up time for instructors to focus on more critical aspects of teaching.
- Improved Student Engagement: Personalized feedback and adaptive learning paths keep students motivated and involved in the course material.
- Data-Driven Insights: AI tools analyze student data, offering instructors valuable insights into student progress and areas that need more attention.
Enhancing Learner Engagement Through Adaptive Learning Algorithms
Adaptive learning algorithms have revolutionized the way educational content is delivered in online courses, particularly within the framework of OpenCourseWare. By tailoring content to individual learner needs, these algorithms ensure that each student receives a personalized learning experience. Unlike traditional methods, where all learners follow the same path, adaptive systems adjust the difficulty, pace, and type of content based on real-time analysis of the learner's performance. This creates a more engaging and effective learning environment.
The core advantage of adaptive learning is its ability to respond dynamically to learner behavior, helping to keep students motivated and actively engaged in the material. This approach not only addresses knowledge gaps but also reinforces areas where the learner excels, ensuring they remain challenged and involved. By utilizing data-driven insights, adaptive learning platforms foster deeper understanding and promote continuous improvement in the learner's journey.
Key Elements of Adaptive Learning Systems
- Real-time assessment: Constant monitoring of learner progress to adjust content delivery.
- Personalization: Customizing learning paths based on individual strengths and weaknesses.
- Data analytics: Using learner performance data to predict future learning needs and outcomes.
Important aspects of these systems include:
Adaptive algorithms allow instructors to identify patterns in learning behaviors, enabling timely interventions and more targeted support.
Benefits of Adaptive Learning
- Improved retention: By delivering content at the right level of difficulty, learners are more likely to retain information.
- Increased motivation: Adaptive learning prevents frustration and boredom by continuously adjusting to the learner's pace and progress.
- Efficient use of resources: Learners only focus on content that challenges them, making their study time more productive.
Table: Comparison of Traditional Learning vs Adaptive Learning
Aspect | Traditional Learning | Adaptive Learning |
---|---|---|
Learning Path | Fixed, same for all learners | Dynamic, personalized for each learner |
Engagement | Varies, often passive | Active, responsive to learner progress |
Feedback | Periodic, instructor-led | Real-time, algorithm-driven |
Integrating AI-Based OpenCourseWare with Learning Management Systems for a Unified Learning Experience
As the demand for personalized learning experiences grows, integrating AI-driven OpenCourseWare (OCW) into existing Learning Management Systems (LMS) is becoming a key approach to enhancing educational platforms. This synergy allows instructors and students to benefit from AI’s capabilities, including adaptive learning paths, automated grading, and personalized content recommendations. The integration can streamline workflows and provide a more engaging and efficient learning experience for both educators and learners.
To effectively integrate AI-powered OCW into an LMS, several considerations need to be made, including data compatibility, user interface adjustments, and continuous monitoring of AI performance. A smooth transition requires ensuring that AI algorithms are compatible with existing LMS structures and that they can support diverse educational tools. Below, we outline the key steps for achieving this integration.
Key Steps for Integration
- Data Synchronization: Ensure that AI models can access and process data from the LMS seamlessly. This includes user profiles, course content, and progress tracking.
- Adaptive Learning Algorithms: Integrate AI algorithms that can analyze student progress and recommend tailored learning paths.
- Automated Feedback Mechanisms: Implement AI systems that can provide real-time feedback to students based on their performance in assignments and quizzes.
- User Interface Compatibility: Adapt the LMS interface to display AI-generated insights and feedback in a user-friendly manner.
Challenges and Solutions
- Data Privacy Concerns: Ensure AI tools comply with data protection regulations such as GDPR to maintain student privacy.
- System Overload: Ensure the AI system is optimized to handle the volume of interactions within the LMS without causing performance issues.
- Instructor Training: Provide proper training for instructors on how to leverage AI tools effectively in the LMS environment.
Benefits of Integration
Benefit | Description |
---|---|
Personalized Learning | AI can offer personalized content and learning paths based on individual student needs and progress. |
Efficiency Gains | Automated grading and feedback reduce administrative burdens on instructors, allowing more focus on content delivery. |
Real-Time Analytics | AI provides real-time analytics that help instructors monitor student engagement and performance. |
"Integrating AI with OpenCourseWare not only enhances student learning but also empowers instructors with data-driven insights to improve teaching strategies."
Optimizing Content Personalization with AI-Based Recommendations
Personalizing content in digital platforms is becoming an essential tool to enhance user engagement and satisfaction. AI-driven recommendation systems have gained significant attention in recent years due to their ability to tailor content based on individual preferences and behaviors. By analyzing vast amounts of user data, these systems predict what content will be most relevant to each user, fostering a more personalized experience. This process is powered by machine learning algorithms that continuously refine their predictions based on new data inputs, ensuring that recommendations evolve over time.
The key to an effective recommendation system lies in its ability to combine various data sources, such as user behavior, historical interactions, and even contextual information, to make precise suggestions. As AI technologies advance, the precision of these systems improves, enabling better content targeting across various domains, from e-commerce platforms to educational resources. A deep dive into these systems reveals the methodologies, challenges, and benefits of AI-powered personalization techniques.
Core Methodologies in AI-Powered Content Personalization
- Collaborative Filtering: Uses user-item interactions to recommend content by identifying patterns of behavior shared by similar users.
- Content-Based Filtering: Recommends content based on the features of items a user has previously interacted with, like keywords or genres.
- Hybrid Approaches: Combines both collaborative and content-based filtering methods to increase accuracy in recommendations.
Challenges and Benefits
- Challenge: Balancing the need for personalized content without overwhelming users with excessive choices.
- Challenge: Maintaining data privacy and security while collecting and processing personal user data.
- Benefit: Increased user engagement through relevant, tailored recommendations that align with their preferences.
- Benefit: Improved user satisfaction, leading to higher retention rates and more frequent usage.
AI-powered recommendation systems have revolutionized the way users interact with content, enabling platforms to provide more engaging and relevant experiences. However, they must also navigate challenges like data privacy and algorithmic bias to maintain trust and accuracy.
Example of AI-Driven Content Personalization
Platform | Recommendation Approach | Key Feature |
---|---|---|
Netflix | Hybrid model (Collaborative + Content-Based) | Personalized movie/show recommendations based on viewing history |
Amazon | Collaborative Filtering | Product recommendations based on previous purchases and user preferences |
Coursera | Content-Based Filtering | Course suggestions based on past course enrollments and interests |
Evaluating the Influence of AI-Enhanced OpenCourseWare on Learner Outcomes
The integration of artificial intelligence (AI) into OpenCourseWare (OCW) platforms has provided significant opportunities for enhancing the educational experience. With AI-driven tools, learners can access personalized recommendations, real-time feedback, and adaptive learning paths that cater to their individual needs. These technological advancements raise questions about the actual impact of AI in OpenCourseWare on learner performance. Specifically, measuring the effectiveness of these innovations requires a deeper understanding of how they influence student engagement, knowledge retention, and overall success.
One of the key metrics for evaluating AI's role in learning environments is learner performance. Various factors such as academic achievements, engagement with the content, and time spent on tasks are essential indicators. By analyzing the data collected from AI-enhanced platforms, educators can gain insights into which features are most effective in improving learner outcomes. This data-driven approach allows for ongoing optimization of educational strategies and tools.
Impact Assessment Criteria
- Engagement: The extent to which students interact with AI-powered learning modules.
- Knowledge Retention: How well students remember and apply the information over time.
- Completion Rates: The percentage of learners who successfully finish courses or tasks.
- Performance Improvement: The measurable improvement in test scores or assessments.
Measuring Tools and Methods
- Learning Analytics: Collecting data from student interactions to monitor progress and engagement.
- Surveys and Feedback: Gathering qualitative data from learners about their experience with AI tools.
- Comparative Analysis: Comparing the performance of students using AI-enhanced OCW with those using traditional methods.
Key Findings
Study | Effect on Learner Performance | Key Observation |
---|---|---|
AI-Based Adaptive Learning | Significant improvement in knowledge retention | Personalized learning paths lead to better understanding. |
AI-Driven Feedback Systems | Higher student engagement and task completion rates | Real-time feedback boosts motivation and persistence. |
AI-powered OpenCourseWare tools are transforming learning by providing a more tailored educational experience, which is positively impacting student outcomes and overall course success.