Best Ai Platform for Literature Review
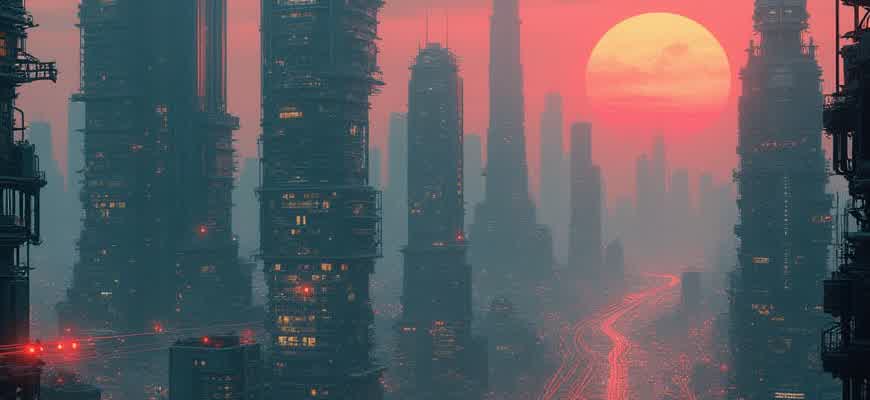
Artificial intelligence has revolutionized the way researchers approach literature reviews, enabling faster, more efficient processing of large volumes of academic papers. Whether you’re exploring the latest advancements in your field or conducting a systematic review, AI tools can help synthesize vast amounts of information, categorize research, and identify trends. Below are some of the best platforms tailored to streamline this process.
1. ResearchRabbit
- Automates the process of finding relevant papers based on initial research inputs.
- Provides citation mapping to visualize connections between studies.
- Uses AI to suggest papers with high relevance, even those outside traditional search databases.
2. Iris.ai
- Uses natural language processing to understand the content of papers at a conceptual level.
- Identifies key research themes and organizes papers by relevance.
- Enables the mapping of knowledge spaces to explore related fields.
"AI-powered platforms are transforming how we access, process, and understand academic literature, allowing researchers to focus on innovation rather than data collection."
Comparison Table: Features of AI Platforms for Literature Review
Platform | Key Feature | Unique Advantage |
---|---|---|
ResearchRabbit | Automatic paper suggestion and citation mapping | Visual representation of research connections |
Iris.ai | Concept-based literature exploration | Understanding content beyond keywords |
How to Choose the Right AI Platform for Academic Research
Choosing an AI platform for academic research requires careful consideration of several factors, including the platform's ability to process large datasets, generate meaningful insights, and support academic citation standards. Researchers should prioritize platforms that offer reliable data sources, strong analytical tools, and integration with research management systems. Since each academic field has unique requirements, it’s essential to ensure that the chosen AI platform supports the specific methodologies and citation formats relevant to your work.
In addition to these features, the ease of use and the quality of the platform’s user interface are crucial. A platform that is overly complex or difficult to navigate may hinder your research productivity. To make the right decision, researchers should assess both the technical capabilities and the usability of the platform, keeping in mind long-term goals for their research projects.
Key Factors to Consider When Choosing an AI Platform
- Data Access and Quality: Ensure the platform offers access to relevant and reliable data sources. Some platforms provide academic databases, while others may focus on publicly available datasets.
- Analytical Tools: Choose a platform that offers powerful tools for text analysis, natural language processing (NLP), and data visualization, which are essential for conducting a thorough literature review.
- Integration with Citation Management: A platform that integrates with citation management tools (e.g., Zotero, EndNote) can save significant time during the research and writing process.
- Cost: Evaluate whether the platform fits within your research budget, as many AI tools have premium features that might require a subscription.
Platform Evaluation Process
- Step 1: Define Your Needs – Understand the specific requirements of your research, such as the need for large-scale data analysis, support for specific languages, or citation compatibility.
- Step 2: Test the Platform – Use free trials or demo versions to evaluate the platform's features, interface, and overall usability.
- Step 3: Read Reviews and Recommendations – Check for feedback from other researchers in your field to understand their experiences with the platform.
- Step 4: Compare Platforms – Create a comparison table based on key features like cost, data sources, and tools offered, to choose the most suitable option.
Comparison of Popular AI Platforms for Academic Research
Platform | Features | Pricing | Integration |
---|---|---|---|
Platform A | Advanced NLP, citation integration, customizable reports | Subscription-based | Works with Zotero, EndNote |
Platform B | Data visualization, keyword extraction, API access | Free and Premium versions | Limited integration |
Platform C | Text mining, academic database access, collaboration tools | Paid plans | Full integration with citation tools |
It’s essential to choose an AI platform that not only meets your research needs but also aligns with the goals of your academic work. Consider long-term usability and potential for scaling your research projects.
Automating Literature Search with AI: Key Features to Look For
AI tools are revolutionizing the way literature reviews are conducted, allowing researchers to quickly identify relevant sources, analyze vast datasets, and stay updated with the latest publications. When evaluating AI platforms for literature search, there are several critical features to consider that can streamline the entire process and ensure efficiency. These features not only save time but also enhance the depth and accuracy of the search results, ultimately benefiting the quality of research outcomes.
When selecting an AI platform for automating literature searches, it is essential to focus on capabilities that allow for comprehensive, precise, and up-to-date results. Below are some of the most important features to look for in a literature search AI tool:
Key Features for Efficient Literature Search
- Advanced Keyword Matching: The platform should be able to match both exact and related terms to cover a broad spectrum of relevant research.
- Natural Language Processing (NLP): AI that can understand and process complex search queries in human language will yield more accurate results, even from ambiguous or incomplete search phrases.
- Automated Citation Tracking: Look for tools that not only find papers but also track citations and their relationships to other works to provide a broader view of a topic's research landscape.
- Integration with Databases: Seamless integration with academic databases (e.g., PubMed, IEEE Xplore, Google Scholar) is critical to access a wide range of sources.
- Real-time Updates: The ability to receive notifications about newly published papers in your area of interest ensures your review remains current.
Additional Functionalities to Enhance Literature Search
- AI-Powered Summarization: The platform should offer summarization tools to extract the most relevant information from papers quickly.
- Automatic Categorization: Automatically grouping research into topics or themes can save significant time when sorting through large datasets.
- AI-Powered Recommendations: Personalized suggestions based on your past searches and preferences can bring new and relevant literature to your attention.
Tip: Platforms that combine machine learning and NLP techniques can significantly reduce the time spent on manual literature searches, providing a more comprehensive and focused review.
Feature Comparison
Feature | Tool A | Tool B | Tool C |
---|---|---|---|
Keyword Matching | Advanced | Basic | Advanced |
NLP Capabilities | Yes | No | Yes |
Real-Time Updates | Yes | Yes | No |
Citation Tracking | Yes | No | Yes |
Maximizing Citation Accuracy: AI Tools That Ensure Precision
Accurate citation management is crucial in any literature review process. Researchers depend on precise referencing to ensure credibility and avoid unintentional plagiarism. Artificial intelligence tools designed for this purpose are able to streamline the referencing process, offering not only speed but also accuracy. These AI platforms utilize machine learning algorithms to parse through vast databases, recognizing and categorizing sources with exceptional precision.
By leveraging natural language processing (NLP) and other advanced technologies, AI-driven citation tools can identify potential errors, suggest corrections, and provide proper formatting across various citation styles. Researchers no longer need to manually check each reference, as AI ensures that all sources are correctly attributed and formatted according to specific guidelines.
Key AI Tools for Citation Accuracy
- Zotero: A free and open-source tool that automatically collects, organizes, cites, and shares research materials.
- Mendeley: A reference manager with AI-powered features that help researchers discover relevant papers and generate citations.
- EndNote: A robust reference management software that integrates AI algorithms to streamline the citation process.
How AI Tools Ensure Citation Precision
- Database Matching: AI tools compare sources with vast databases to ensure references match the correct publication.
- Error Detection: The AI identifies formatting errors and inconsistencies, suggesting accurate fixes in real-time.
- Cross-Platform Support: AI citation tools work across multiple citation styles (APA, MLA, Chicago, etc.), ensuring consistency in your work.
"AI-powered citation tools remove the guesswork, offering researchers confidence in the accuracy and credibility of their references."
Comparing Popular Citation AI Tools
Tool | Key Features | Best For |
---|---|---|
Zotero | Automatic citation collection, reference sharing, browser integration | Individual researchers, collaborative projects |
Mendeley | Paper discovery, citation generation, cloud syncing | Academics and researchers in need of paper organization |
EndNote | Advanced referencing, citation management, database integration | Professional researchers, large academic projects |
AI-Powered Summarization: Streamlining the Literature Review Process
The process of conducting a literature review can be time-consuming and overwhelming, especially when researchers must sift through an extensive amount of academic papers. AI-powered summarization tools have revolutionized this process by providing a faster and more efficient way to extract the key insights from a vast pool of research materials. By using sophisticated natural language processing (NLP) algorithms, these tools can quickly analyze and summarize articles, helping researchers focus on the most relevant information without having to manually read through each paper in its entirety.
These summarization platforms are equipped with capabilities that allow users to automatically condense large volumes of research into concise, meaningful summaries. They can identify the central themes, methodologies, and results of studies, offering researchers a clear overview of the literature without sacrificing critical details. The result is a more streamlined and effective literature review process, saving valuable time while maintaining the quality of research analysis.
Key Benefits of AI Summarization in Literature Reviews
- Time Efficiency: AI tools significantly reduce the time required to go through multiple sources, helping researchers extract the most pertinent information in minutes rather than hours.
- Improved Accuracy: By leveraging AI’s ability to identify patterns and important details, these tools can generate summaries that focus on key findings without omitting crucial data.
- Enhanced Focus: AI can prioritize specific areas of interest in a literature review, allowing researchers to narrow down their focus and explore specific themes in more depth.
How AI Summarization Works
- Text Extraction: AI tools extract relevant text from full-text research papers.
- Content Analysis: NLP algorithms analyze the extracted content to identify the most important themes and insights.
- Summary Generation: A concise summary is generated, highlighting the essential information such as the research’s objectives, methodology, findings, and conclusions.
Example of AI Summarization
Original Text | AI Summary |
---|---|
Researchers explored the impact of AI on healthcare delivery, finding that machine learning algorithms improved patient diagnosis and treatment planning, leading to better outcomes in a range of medical fields. | AI in healthcare has improved diagnosis and treatment planning, enhancing patient outcomes. |
AI-powered summarization offers an unprecedented level of efficiency and precision in the literature review process, ensuring that critical insights are not overlooked.
Integrating AI with Reference Management Software for a Smooth Workflow
In the context of academic writing and literature reviews, reference management software plays a crucial role in organizing sources and ensuring proper citation formatting. However, as research grows increasingly complex, integrating AI into these systems can enhance efficiency by automating tasks that traditionally require manual effort. AI algorithms can assist with source identification, content summarization, and citation formatting, significantly reducing the workload for researchers. This seamless integration can provide a unified platform for both managing and reviewing sources with minimal human intervention.
By merging AI with reference management tools, researchers can create an intelligent environment where data from multiple sources is not only organized but also analyzed for relevance. Such integration helps in ensuring accuracy, saving time, and increasing the overall productivity of research workflows. The following sections explore how this integration works and the benefits it brings to researchers.
How AI Enhances Reference Management
AI-powered reference management tools can streamline the entire research process, from data collection to citation generation. Below are some key ways AI enhances the functionality of these tools:
- Automated Citation Generation: AI can automatically generate citations in various formats such as APA, MLA, or Chicago by analyzing metadata from scholarly articles.
- Smart Search and Sorting: AI can improve the search capabilities of reference managers, allowing users to locate articles based on specific themes, keywords, or relevance.
- Content Summarization: AI can provide concise summaries of research papers, making it easier to assess the relevance of each source without reading through the entire text.
Benefits of Integrating AI and Reference Management Systems
The combination of AI with reference management software offers several advantages that improve the quality and speed of literature reviews:
- Increased Efficiency: AI automates repetitive tasks such as citation formatting, allowing researchers to focus more on analysis and interpretation.
- Improved Accuracy: AI reduces the likelihood of human errors in citation generation and source management.
- Enhanced Research Quality: AI-powered tools provide intelligent recommendations, suggesting relevant sources based on the researcher's interests and needs.
Example of AI Integration in a Reference Management Tool
Feature | AI Integration Benefit |
---|---|
Automatic Citation | Generates citations based on extracted metadata, reducing time spent on formatting. |
Smart Source Filtering | Uses AI algorithms to identify the most relevant sources based on research queries. |
Content Summarization | AI reads and summarizes articles, allowing researchers to quickly assess relevance without reading the full text. |
Integrating AI with reference management tools enables seamless workflows by automating routine tasks, ensuring researchers can focus on higher-level analysis and decision-making.
AI-Driven Keyword Extraction for Faster Topic Identification
AI-powered tools can significantly enhance the process of identifying relevant topics by extracting key terms from large volumes of literature. Traditional methods of manually scanning papers are time-consuming, but AI can automate the identification of core concepts, making the process faster and more efficient. By leveraging natural language processing (NLP) algorithms, these platforms analyze text data to pull out the most pertinent keywords, helping researchers quickly pinpoint relevant themes and areas of interest.
Furthermore, AI-driven keyword extraction can reduce human bias in selecting terms, ensuring a more comprehensive and objective approach. By utilizing advanced techniques such as machine learning models, AI tools can continually improve their ability to recognize patterns and identify emerging trends within research literature, making them invaluable for systematic literature reviews and meta-analyses.
Key Advantages of AI-Driven Keyword Extraction
- Efficiency: AI tools automate the process, saving time compared to manual keyword extraction.
- Accuracy: Advanced algorithms reduce human error and ensure that the most relevant terms are identified.
- Scalability: AI platforms can handle large volumes of research papers without losing effectiveness.
How AI Improves Topic Identification
- Text Analysis: AI algorithms process the full text of documents, extracting semantic meaning from context, rather than relying on isolated keywords.
- Contextual Understanding: AI systems can understand the relationship between terms and concepts, ensuring that the keywords selected are contextually relevant.
- Continuous Learning: Machine learning models evolve over time, becoming increasingly adept at recognizing the most significant keywords and topics as new research trends emerge.
Examples of AI-Driven Keyword Extraction Tools
Tool | Features |
---|---|
TextRazor | Offers advanced NLP for keyword extraction and topic detection from scientific literature. |
IBM Watson Natural Language Understanding | Provides semantic analysis to identify key terms and relationships in text. |
Google Cloud Natural Language | Analyzes documents to extract key phrases and identify trends in research topics. |
AI-driven keyword extraction tools are revolutionizing the way researchers conduct literature reviews, offering faster and more accurate topic identification compared to traditional manual methods.
Evaluating AI Platforms: Metrics and Benchmarks for Quality Assessment
When assessing AI platforms for literature review, it is critical to establish a set of standards that ensures the reliability, effectiveness, and precision of the platform's capabilities. The evaluation process involves various metrics that measure the platform's performance in different areas, such as accuracy, speed, and relevance. The quality assessment of these platforms is fundamental for users aiming to make informed decisions based on the platform’s output.
To evaluate an AI system thoroughly, metrics and benchmarks play a vital role. These allow users to quantify the AI's performance, enabling comparisons across different platforms. Key factors like data processing capabilities, contextual understanding, and the ability to generate comprehensive literature reviews based on diverse academic sources must be taken into account.
Key Metrics for Evaluation
- Accuracy: Measures how well the AI platform extracts and synthesizes relevant data.
- Processing Speed: Indicates the time taken for the AI to generate insights from large datasets.
- Contextual Relevance: Evaluates the platform’s ability to maintain the relevance of sources within the context of the literature review.
- Comprehensiveness: Assesses the platform’s ability to cover a wide array of sources without omitting crucial information.
Benchmarking AI Platforms
Effective benchmarking involves comparing AI platforms based on standardized datasets and predefined criteria. The comparison can be facilitated by the use of various performance benchmarks such as:
- Standardized Datasets: Using consistent datasets across platforms to compare how each system handles literature extraction.
- Precision and Recall: Quantifying the AI’s ability to identify relevant studies (precision) and cover all necessary references (recall).
- User Feedback: Collecting reviews and feedback from researchers who have used the platforms to gain insights into real-world usability.
Performance Table
Platform | Accuracy | Speed | Contextual Relevance | Comprehensiveness |
---|---|---|---|---|
AI Platform A | 95% | Fast | High | Comprehensive |
AI Platform B | 89% | Moderate | Medium | Moderate |
AI Platform C | 98% | Fast | High | High |
Important Note: Consistent monitoring and re-evaluation of AI platform performance are necessary as technological advancements can lead to significant improvements in processing abilities and quality outputs.