Ai in Adaptive Learning Challenges and Opportunities
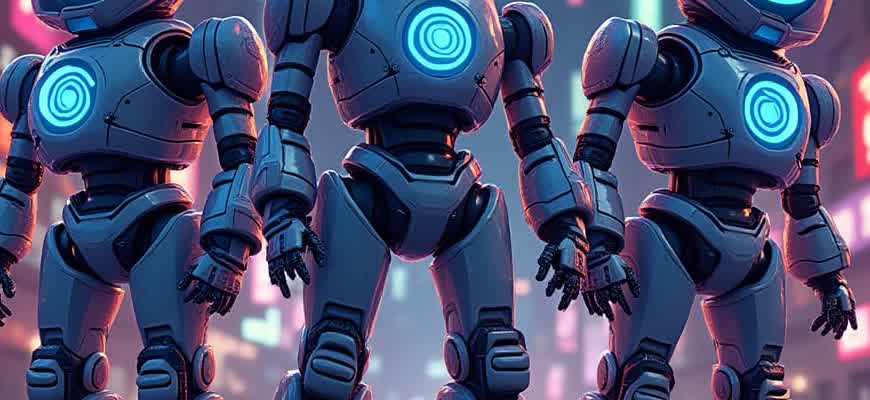
Artificial intelligence has the potential to revolutionize the field of personalized education by adapting learning paths to individual student needs. However, integrating AI into adaptive learning systems presents both challenges and opportunities. Below, we explore the key aspects of AI's role in shaping education.
Challenges in Implementing AI for Adaptive Learning:
- Data Privacy Concerns: The collection and use of personal data raise significant privacy issues. AI-driven systems rely on large datasets to provide personalized experiences, but safeguarding student data is critical.
- Bias in Algorithms: If not carefully designed, AI algorithms can perpetuate biases, leading to unequal learning opportunities for diverse student groups.
- Resource Requirements: Developing and maintaining AI systems requires substantial investment in technology, infrastructure, and expertise.
Opportunities Offered by AI in Adaptive Learning:
- Personalized Learning Paths: AI can tailor educational content to each student's pace, learning style, and skill level, enhancing engagement and retention.
- Real-time Feedback: AI provides instant feedback, enabling students to correct errors and adjust their learning strategies quickly.
- Scalable Education Solutions: With AI, educational institutions can provide personalized learning at scale, reaching a broader audience with diverse needs.
"Artificial intelligence enables us to create learning environments that adjust dynamically to the learner's needs, ensuring an individualized educational experience."
As AI continues to advance, its integration into adaptive learning will require careful consideration of both the challenges and the tremendous potential it offers to educators and students alike.
AI in Adaptive Learning: Challenges and Opportunities
Artificial Intelligence (AI) is transforming the landscape of adaptive learning by enabling personalized educational experiences that adjust to individual learner needs. However, the integration of AI into this domain presents a variety of challenges that must be addressed to fully leverage its potential. Among the significant hurdles are data privacy concerns, the need for robust algorithms, and the potential for bias in AI-driven recommendations. Nevertheless, the opportunities for enhancing educational outcomes through adaptive learning are vast, ranging from improved student engagement to more efficient learning processes tailored to diverse learning styles.
In the context of adaptive learning, AI systems have the capacity to continuously monitor a learner’s progress and adjust educational content in real-time. This allows for a more individualized learning journey, as the system can provide personalized feedback, identify areas for improvement, and modify difficulty levels based on the learner’s pace. However, the effectiveness of these systems depends on the quality of data they process, the sophistication of underlying algorithms, and the seamless integration of AI tools into traditional educational environments.
Challenges in AI-Driven Adaptive Learning
- Data Privacy and Security: AI systems require large amounts of data to make accurate predictions, raising concerns about the security of sensitive learner information.
- Bias and Fairness: If the data used to train AI algorithms is biased, it can lead to unfair recommendations, potentially disadvantaging certain groups of learners.
- Complexity of Algorithms: Developing algorithms that can accurately adapt to diverse learner needs is a complex and ongoing process, requiring continual refinement and testing.
Opportunities for AI in Adaptive Learning
- Personalized Learning Paths: AI can tailor educational content to match the learning speed, style, and preferences of individual students.
- Improved Student Engagement: By offering dynamic learning experiences, AI can keep students more engaged and motivated, enhancing their overall educational experience.
- Efficiency in Learning: Adaptive systems can identify knowledge gaps more quickly and accurately, helping students to focus on areas that require more attention.
"AI-powered adaptive learning systems hold the potential to revolutionize education by providing personalized learning experiences that are scalable and effective for all students."
Key Areas for Improvement
Area | Challenge | Opportunity |
---|---|---|
Data Collection | Ensuring the accuracy and diversity of collected data is crucial for AI models to function effectively. | Access to a broad range of learner data enables more personalized, adaptive learning experiences. |
Algorithm Development | Creating adaptable algorithms that respond to a wide variety of learning needs and environments is complex. | AI can help build systems that adapt to diverse educational contexts, improving efficiency and outcomes. |
Understanding the Role of AI in Personalizing Learning Paths
Artificial Intelligence (AI) plays a critical role in shaping individualized learning experiences by tailoring content and pacing to meet the unique needs of each learner. By analyzing data from student interactions, AI systems can create dynamic learning paths that adapt as the student progresses. This personalized approach goes beyond traditional one-size-fits-all models, ensuring that learners receive the right level of challenge and support at every stage of their educational journey.
AI-powered tools utilize a combination of algorithms, predictive analytics, and natural language processing to understand the strengths, weaknesses, preferences, and learning styles of individual students. This data is then leveraged to generate customized content and assessments, allowing for a more effective learning experience. With AI, students can explore subjects at their own pace, receiving immediate feedback and guidance based on their progress and engagement.
Key Benefits of AI in Personalized Learning Paths
- Improved Engagement: AI can present material in formats that resonate with each learner, such as interactive simulations, visual aids, or personalized readings, increasing overall engagement and motivation.
- Real-Time Adaptation: AI systems continuously monitor students' performance and adapt the learning path in real time, ensuring that learners stay on track and are never overwhelmed.
- Efficiency: By automating the adaptation process, AI reduces the time teachers need to spend on administrative tasks, allowing them to focus on more complex aspects of teaching.
How AI Customizes Learning Experiences
- Data Collection: AI gathers data from assessments, quizzes, and learner interactions to evaluate proficiency and learning patterns.
- Personalized Content Generation: Based on collected data, AI curates materials suited to the student's current knowledge level and learning style.
- Dynamic Assessment: Instead of static exams, AI adjusts the difficulty of questions in real time to challenge the student appropriately.
"AI in education is not about replacing teachers but empowering them to better understand and meet the needs of their students."
AI’s Impact on Learner Outcomes
Feature | Impact on Learner Outcomes |
---|---|
Instant Feedback | Accelerates learning by providing timely, actionable insights on student performance. |
Adaptive Pacing | Ensures that learners progress at their own pace, improving retention and mastery of concepts. |
Learning Analytics | Helps track and predict learner progress, identifying areas that need attention. |
How AI-Driven Analytics Can Identify Learning Gaps in Real-Time
AI-powered analytics tools offer unprecedented opportunities for real-time tracking of students' learning progress. By leveraging advanced algorithms, these systems can analyze vast amounts of data generated during the learning process, identifying areas where students are struggling or excelling. This allows educators to respond more quickly and effectively to learning challenges, optimizing the learning experience for each individual.
Through continuous monitoring of students' interactions with learning materials, AI can pinpoint specific knowledge gaps, providing actionable insights for both teachers and learners. By focusing on individual performance patterns, AI-driven systems can highlight discrepancies in understanding, offering targeted solutions for improvement.
Key Advantages of Real-Time AI Analytics
- Instant Feedback: AI systems can provide immediate insights into a student's strengths and weaknesses.
- Personalized Learning Paths: By analyzing performance, AI can recommend personalized learning resources tailored to individual needs.
- Predictive Capabilities: AI can predict potential future learning difficulties, allowing educators to intervene before gaps widen.
How AI Identifies Learning Gaps
- Data Collection: AI gathers detailed data from various sources such as quizzes, assignments, and student interactions with course content.
- Pattern Recognition: The system identifies patterns in responses, recognizing areas where students repeatedly struggle.
- Gap Detection: AI flags areas where a student’s understanding deviates from expected progress, identifying specific topics or skills that need improvement.
Example: An AI system may detect that a student repeatedly struggles with algebraic concepts, despite performing well in other subjects, indicating a specific knowledge gap that can be addressed with targeted exercises.
Visualization of Learning Gaps
Student | Strengths | Weaknesses |
---|---|---|
Student A | Excellent in reading comprehension | Poor in mathematics |
Student B | Strong in problem-solving tasks | Difficulty understanding grammar |
Overcoming Data Privacy Challenges in AI-Powered Adaptive Learning Systems
AI-powered adaptive learning systems can offer personalized educational experiences by analyzing vast amounts of student data. However, these systems raise significant concerns regarding data privacy and security. Given the sensitive nature of student information, such as learning behavior, personal preferences, and performance metrics, ensuring that this data is handled securely is paramount. The need to balance personalization with privacy protections is one of the key challenges in implementing AI-driven learning solutions effectively.
To address these concerns, several strategies can be implemented. These strategies focus on safeguarding data while maintaining the efficacy of adaptive learning systems. Effective data anonymization, encryption, and transparent consent processes are essential to protect sensitive information. Below are some important practices and considerations for overcoming data privacy challenges in AI-based adaptive learning systems:
Key Strategies for Addressing Data Privacy in AI Learning Systems
- Data Anonymization: One of the most effective methods to protect privacy is to anonymize personal information, ensuring that individual identities cannot be traced back from the data.
- End-to-End Encryption: Encrypting all data, both in transit and at rest, helps safeguard against unauthorized access and reduces the risk of data breaches.
- Transparent Consent Processes: Clear and informed consent must be obtained from users before any data is collected. This ensures that learners are aware of how their data will be used and have control over their participation.
Practical Measures for Implementation
- Privacy by Design: Incorporating privacy measures into the design of AI systems from the outset ensures that data protection is an integral part of the technology.
- Regular Audits and Monitoring: Continuous monitoring of data usage and regular privacy audits help identify potential vulnerabilities and improve the system's security protocols over time.
- Data Minimization: Collecting only the data necessary for the system’s operation and analysis reduces the risk of overexposure of sensitive information.
"Privacy is not just about protecting data; it is about empowering users to make informed choices about their personal information." – Privacy Advocate
Comparison of Data Protection Approaches
Approach | Advantages | Challenges |
---|---|---|
Data Anonymization | Protects user identities, ensuring that data cannot be traced back to individuals. | May reduce the accuracy of AI models by eliminating identifiable data. |
End-to-End Encryption | Secures data from unauthorized access during transmission and storage. | Increased computational costs and system complexity. |
Transparent Consent | Builds trust and compliance with legal regulations such as GDPR. | May reduce user participation if consent processes are too cumbersome. |
Integrating AI with Existing Learning Management Systems
Integrating artificial intelligence (AI) into current Learning Management Systems (LMS) presents significant opportunities to enhance personalized learning experiences, streamline content delivery, and improve learner engagement. By embedding AI technologies, such as machine learning algorithms, into LMS platforms, educational institutions can create dynamic learning environments that adapt to individual student needs. However, this integration is not without its challenges, such as system compatibility, data privacy concerns, and the necessity of continual updates to both AI models and the LMS itself.
The process of AI integration requires careful planning and a comprehensive understanding of both the technological capabilities of the LMS and the specific needs of learners. AI can be used to automate administrative tasks, such as grading and feedback, and provide insights into student performance trends. Additionally, AI-powered analytics can recommend tailored content, track progress, and identify students at risk of falling behind. For this, it is crucial that AI solutions are compatible with existing infrastructure, allowing for seamless interaction between the two systems.
Challenges and Key Considerations
- System Compatibility: AI tools must be integrated without disrupting the existing architecture of the LMS, requiring robust API support and standardized data formats.
- Data Privacy: The handling of sensitive student data must comply with regulations like GDPR or FERPA, ensuring transparency and security.
- Scalability: AI applications must scale effectively to accommodate growing numbers of users and diverse educational content.
- Continuous Maintenance: As AI technologies evolve, ongoing updates and model retraining are essential to ensure effectiveness and relevance.
Opportunities and Benefits
- Personalized Learning: AI can adapt learning paths based on individual student performance, preferences, and engagement, leading to more effective and targeted learning.
- Automation of Administrative Tasks: Routine tasks such as grading, feedback generation, and tracking student progress can be automated, saving time for instructors and enhancing the learning experience.
- Enhanced Analytics: AI-driven insights enable instructors to identify at-risk students early, providing opportunities for timely intervention.
Key Data Points
AI Feature | Benefit |
---|---|
Adaptive Learning Algorithms | Personalized educational experience for each student |
Automated Grading | Frees up instructors' time for more personalized interaction |
Predictive Analytics | Helps identify students who may need additional support |
"The integration of AI into LMS systems transforms traditional educational methods, allowing for more efficient and tailored learning experiences that can drive student success."
Challenges in Training AI Models for Diverse Student Needs
One of the main difficulties in developing AI systems for adaptive learning lies in addressing the vast range of individual student requirements. Every student has unique learning styles, backgrounds, and challenges, which can be hard for AI models to account for effectively. Models trained on data from homogeneous groups often fail to recognize the variability found in more diverse student populations. As a result, AI systems might not adapt well to all students, especially those who deviate from the "norm" in terms of learning pace, ability, or cultural context.
Moreover, gathering the necessary data for training such systems can be a logistical challenge. Diverse data sets that truly represent a wide range of learning experiences are often hard to come by. Without sufficient representation, AI models may become biased, perpetuating inequalities or offering suboptimal learning experiences for certain groups. This becomes a significant barrier to ensuring that AI-driven educational tools provide meaningful support to all learners.
Key Challenges:
- Bias in Data Collection: AI systems often depend on large datasets, but these data sets may underrepresent certain groups, leading to less effective support for those students.
- Difficulty in Capturing Learning Styles: AI models struggle to accurately model diverse learning preferences, such as visual vs. auditory learners, or those with specific disabilities.
- Complexity of Personalization: The sheer number of potential variables in student performance makes it difficult for AI to deliver consistently tailored learning experiences.
- Ethical Concerns: Ensuring fairness and privacy while using student data is crucial but difficult, particularly when training AI systems on sensitive information.
Potential Solutions:
- Collecting diverse and representative data sets to account for a wider variety of learning needs.
- Integrating feedback loops where students can inform the AI system of their learning preferences and progress.
- Utilizing multi-modal learning approaches, combining different types of data to better address varying learning styles.
"The success of AI in education hinges not just on how much data it can process, but on how inclusively that data reflects the needs of all learners."
Comparison of Traditional vs AI-Driven Approaches:
Traditional Approach | AI-Driven Approach |
---|---|
Standardized content delivery with limited customization for individual needs. | Adaptive learning that adjusts content based on individual student performance and preferences. |
Requires manual intervention for personalization and adjustments. | Automated personalization through machine learning models analyzing student data. |
Potentially slow to identify learning gaps or struggles. | Real-time tracking and adaptation, identifying student challenges as they arise. |
Opportunities for Scalable Adaptive Learning in Remote Education
The integration of adaptive learning technologies into remote education offers several advantages, particularly in the realm of scalability. Remote learning platforms, empowered by artificial intelligence (AI), can tailor content delivery to the needs of individual learners. These systems are capable of analyzing students' progress in real-time, identifying areas of strength and weakness, and adjusting learning paths accordingly. This dynamic approach ensures that students receive personalized instruction, which is crucial for maintaining engagement and improving outcomes in online education environments.
One of the key benefits of this adaptive learning model is its ability to scale. AI-driven systems can handle an enormous volume of learners without compromising the quality of education. Unlike traditional methods, where instructors may struggle to provide individualized attention to large classes, AI systems can monitor and guide countless students simultaneously, ensuring a level of personalized learning that was previously unattainable in remote education.
Key Advantages of Scalable Adaptive Learning
- Personalized Learning Pathways: AI algorithms adjust content based on real-time performance data, offering each student a unique learning experience.
- Improved Retention and Engagement: By addressing students' specific needs and preferences, adaptive learning fosters deeper engagement and enhances long-term knowledge retention.
- Automated Feedback: Instant feedback allows learners to correct mistakes and refine their understanding without waiting for instructor intervention.
- Cost Efficiency: Scalable solutions reduce the need for large numbers of instructors, making education more affordable for institutions and students alike.
Challenges and Solutions in Implementing Scalable Adaptive Learning
- Data Privacy Concerns: Adaptive systems require access to vast amounts of student data to function effectively. Ensuring the security and privacy of this data is paramount.
- Technology Access: In remote settings, not all students have equal access to the required hardware or internet connectivity, which may hinder the effectiveness of adaptive learning systems.
- Teacher Training: Educators may need specific training to use adaptive learning technologies effectively, which can be a barrier to widespread adoption.
"Scalable adaptive learning solutions not only enhance educational outcomes but also open the door for greater accessibility and inclusivity in remote education."
Potential for Future Growth
Opportunity | Impact |
---|---|
Integration with Virtual Reality (VR) | Offers immersive learning experiences that can be personalized, improving engagement and retention. |
Enhanced AI Algorithms | Improves the adaptability of learning paths, making them even more precise and responsive to individual needs. |
Cross-Cultural Education | Adaptive systems can tailor content to different cultural contexts, ensuring more relevant learning experiences for diverse global populations. |
Addressing Bias in AI Algorithms for Education
Artificial intelligence (AI) has the potential to transform the educational landscape, making learning more personalized and effective. However, when AI models are trained on biased datasets, they can perpetuate existing stereotypes, leading to unfair treatment of certain groups. Addressing these biases is essential to ensure AI systems in education promote inclusivity and equity for all learners. A key challenge lies in identifying and mitigating bias within the data and algorithms used in adaptive learning platforms.
Bias in AI systems can emerge from multiple sources, including historical inequalities, skewed data, and biased design choices made by developers. It is crucial to understand the implications of these biases, as they can affect decision-making in student assessments, personalized learning recommendations, and educational content delivery. The goal is to design AI algorithms that are not only efficient but also fair and transparent, ensuring that all students benefit equally from technological advancements in education.
Sources of Bias in Educational AI
- Data-driven bias: AI models trained on biased or incomplete datasets may reproduce these biases in their predictions and recommendations.
- Algorithmic bias: Bias can be unintentionally introduced through the algorithms used to process data, even when the data itself is neutral.
- Human bias: Developers' personal biases can influence the design and development of AI models, inadvertently leading to biased outcomes.
Strategies for Mitigating Bias in AI
- Diverse training data: Ensuring that AI systems are trained on diverse, representative datasets can reduce bias in their predictions.
- Regular audits: Implementing routine audits of AI models to check for biases and ensure that algorithms are working as intended.
- Bias detection tools: Utilizing tools specifically designed to identify and address bias in AI algorithms, ensuring they are continually refined.
- Transparency and accountability: Developers should be transparent about how AI systems are designed and the steps taken to mitigate bias.
"Addressing bias in AI is not just about fairness; it's about ensuring that every student has equal access to the benefits that adaptive learning technologies provide."
Impact of Bias on Student Outcomes
Type of Bias | Potential Impact on Students |
---|---|
Gender Bias | Reinforcement of gender stereotypes, affecting engagement and achievement levels in certain subjects. |
Racial Bias | Discriminatory recommendations or assessments that may disproportionately affect students from marginalized racial groups. |
Socioeconomic Bias | AI-driven recommendations may favor students from wealthier backgrounds, excluding those with fewer resources. |