Ai in Higher Education Statistics
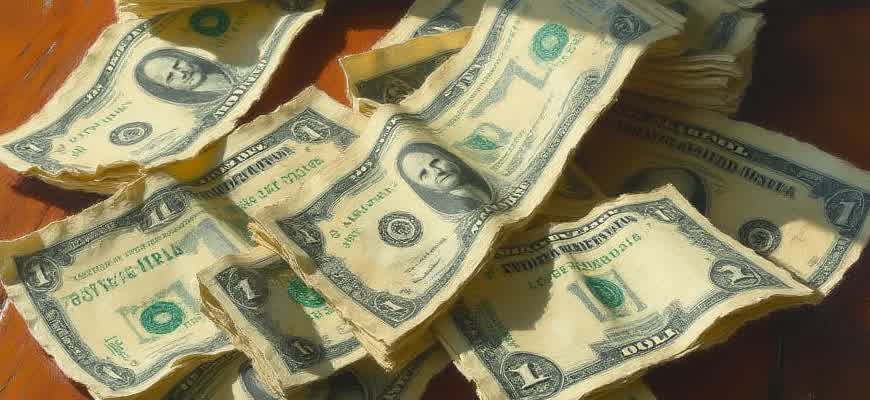
Artificial intelligence (AI) has made significant strides in revolutionizing the landscape of higher education, particularly in the realm of data analysis. Universities and research institutions are increasingly adopting AI technologies to streamline processes, enhance student learning, and optimize institutional management. With the growing integration of AI tools, higher education institutions are able to analyze large datasets more efficiently, making data-driven decisions that impact various aspects of academia.
Key Areas of Impact:
- Predictive analytics for student success
- Improvement of academic performance through personalized learning algorithms
- Data-driven decision-making for institutional management
- AI-powered platforms for research and collaboration
"AI is reshaping the way higher education institutions collect, analyze, and act on data, paving the way for more effective strategies in both teaching and administration."
AI in Education Statistics (Sample Data):
Year | Percentage of Institutions Using AI | Number of AI Tools Deployed |
---|---|---|
2020 | 45% | 150+ |
2021 | 60% | 200+ |
2022 | 75% | 250+ |
AI in Higher Education Data Analysis
The use of artificial intelligence (AI) in higher education statistics has grown significantly over the past decade. AI technologies have been applied to various aspects of educational data collection, analysis, and decision-making, enabling institutions to gain valuable insights that were previously difficult or time-consuming to obtain. With the help of AI, universities can better track student performance, predict enrollment trends, and optimize resource allocation. As a result, educational institutions are increasingly relying on AI-driven systems to improve operational efficiency and enhance the overall student experience.
AI in educational data analysis helps to identify patterns and trends that inform policy decisions, improve academic outcomes, and enhance the learning environment. From data mining to predictive analytics, AI technologies are transforming how educational data is utilized. These systems enable universities to analyze vast amounts of information quickly and accurately, providing a deeper understanding of academic trends, demographic shifts, and institutional performance.
Applications of AI in Higher Education Statistics
- Predictive Analytics: AI models can predict student success, identify at-risk students, and recommend interventions.
- Enrollment Forecasting: AI can analyze historical data to forecast future enrollment patterns, assisting in resource planning.
- Personalized Learning: AI systems help tailor educational content to individual student needs, optimizing learning outcomes.
AI’s ability to process large datasets in real-time significantly improves the accuracy and timeliness of statistical analysis in higher education.
AI-driven Tools and Techniques in Data Analysis
- Data Mining: AI algorithms can extract hidden patterns from large datasets, which may not be evident through traditional statistical methods.
- Machine Learning: AI models continuously improve by learning from new data, making predictions and recommendations more accurate over time.
- Natural Language Processing (NLP): AI-powered systems can analyze textual data from student feedback, research papers, and other academic materials to extract meaningful insights.
Example of AI in Statistical Reports
Statistic | AI Application | Outcome |
---|---|---|
Graduation Rates | Predictive analytics to identify at-risk students | Improved student retention through targeted interventions |
Course Enrollment Trends | Forecasting tools to predict demand for courses | Optimized scheduling and resource allocation |
How AI Revolutionizes Data Collection in Higher Education
Artificial Intelligence (AI) is reshaping how universities gather and manage data, providing more efficient and accurate solutions for academic institutions. By leveraging machine learning algorithms and automation, AI can streamline the collection of student performance data, attendance records, and even faculty feedback. This transformation allows for more dynamic and real-time analysis, ultimately improving decision-making processes across departments.
AI technologies have enabled universities to move beyond traditional data collection methods, such as paper surveys and manual data entry. With AI-powered tools, institutions can automatically collect and analyze data from various sources, including online platforms, learning management systems, and social media interactions. This not only saves time but also provides a richer and more detailed understanding of student behavior, preferences, and performance trends.
Key Ways AI Enhances Data Collection in Universities
- Automated Surveys and Feedback Collection: AI can automatically generate and distribute surveys to students and faculty, ensuring high response rates and consistent data collection.
- Predictive Analytics: By analyzing historical data, AI can predict future trends such as student dropout rates or academic performance, allowing universities to take proactive measures.
- Real-Time Performance Monitoring: AI systems can track student engagement and performance in real time, providing instant insights into areas that need attention.
Practical Applications of AI in Data Collection
- Student Engagement Analysis: AI tools assess how students interact with course content, identifying patterns in participation and highlighting areas where they may need additional support.
- Faculty Feedback and Evaluation: AI can process large volumes of feedback to provide a detailed analysis of teaching effectiveness and areas for improvement.
- Personalized Learning Pathways: By collecting data on individual learning styles, AI helps create customized educational experiences tailored to each student's needs.
"AI is not just about data collection; it's about transforming how we use data to drive educational outcomes and improve student success."
Data Collection Efficiency Comparison
Method | Time to Implement | Data Accuracy | Cost |
---|---|---|---|
Traditional Methods | High | Moderate | High |
AI-Powered Solutions | Low | High | Moderate |
Predictive Analytics: Using AI to Forecast Student Success
Predictive analytics in higher education leverages artificial intelligence to analyze vast amounts of data and predict students' future academic performance. By using machine learning algorithms, institutions can identify students at risk of underperforming, enabling early interventions to improve outcomes. AI tools track patterns such as attendance, participation, and assignment grades to forecast future success, helping universities design personalized academic support systems.
Through predictive analytics, higher education institutions can optimize resource allocation, ensuring that students who need the most help receive timely support. These AI-driven systems can also identify which factors most significantly affect student success, allowing educators to address potential issues before they impact academic achievement.
Key Factors in Predicting Student Success
- Previous Academic Performance: Past grades and course completion rates are critical indicators.
- Engagement and Participation: Active participation in class discussions and assignments can predict success.
- Behavioral Data: Patterns of attendance, online activity, and assignment submissions offer insights into student engagement.
- Demographic Information: Socioeconomic status and other demographic factors can influence performance outcomes.
Applications of Predictive Analytics in Higher Education
- Early Warning Systems: Predictive models can flag students at risk of failing or dropping out.
- Resource Allocation: Institutions can direct tutoring services or counseling to students who need it most.
- Curriculum Adjustments: AI can reveal areas of the curriculum that may need revision based on student performance trends.
Example of Predictive Model Results
Factor | Impact on Student Success |
---|---|
High School GPA | Strong predictor of college success |
Class Participation | Increased engagement correlates with higher grades |
Frequent Absences | Higher absenteeism linked to lower academic performance |
"AI tools provide real-time data on student performance, enabling proactive measures to support students before challenges become insurmountable."
AI-Driven Tools for Real-Time Learning Analytics
In recent years, the integration of artificial intelligence in higher education has led to a shift in how learning is tracked and analyzed. AI-powered tools are enabling real-time monitoring of student performance, providing valuable insights that can drive personalized educational experiences. These systems analyze various data points such as engagement, behavior, and academic progress, helping educators to intervene when necessary and improve learning outcomes.
Real-time analytics allows educators to make informed decisions about student support and curriculum adjustments. AI algorithms can instantly process data from different sources, offering a comprehensive understanding of each student's learning path. This approach moves away from traditional, periodic evaluations, ensuring that students receive continuous feedback, ultimately enhancing their academic journey.
Key Features of AI-Driven Learning Analytics
- Instant Feedback: AI systems can provide immediate feedback to students, helping them to address gaps in understanding right away.
- Personalized Learning Paths: By analyzing individual performance, AI tools can recommend customized learning resources and strategies.
- Predictive Insights: AI can forecast potential learning challenges, allowing educators to offer proactive support to at-risk students.
- Data-Driven Decisions: Faculty and administrators can use AI-generated data to shape course design and improve educational policies.
Examples of AI Tools in Real-Time Analytics
- Learning Management Systems (LMS): Platforms like Moodle and Canvas integrate AI to track student progress and suggest resources tailored to individual needs.
- Intelligent Tutoring Systems (ITS): These systems offer real-time, personalized instruction, adapting to the pace and performance of each student.
- Student Engagement Platforms: AI tools monitor student interactions with course content, providing insights into engagement levels and identifying disengaged students.
"AI-driven learning analytics provide a dynamic, data-rich approach to teaching, ensuring that educators can support students effectively and in real time."
Impact on Educational Institutions
Benefit | Description |
---|---|
Enhanced Student Retention | AI tools help identify at-risk students early, enabling timely interventions that increase retention rates. |
Improved Teaching Methods | Real-time data allows instructors to refine teaching strategies and respond to classroom dynamics more effectively. |
Data-Driven Course Design | AI analytics provide valuable insights for revising curricula to better meet student needs and optimize learning outcomes. |
Improving Student Retention with AI-Driven Predictive Analytics
In the context of higher education, student retention is a critical issue that institutions must address to ensure long-term success. Traditional methods of monitoring and supporting students often rely on manual tracking and subjective assessments. However, with advancements in AI, universities can now leverage predictive models to analyze vast amounts of student data, allowing them to identify at-risk students early and intervene with personalized strategies.
AI-powered tools utilize data from a variety of sources, including academic performance, attendance records, and engagement metrics, to create dynamic models that predict student outcomes. By doing so, institutions can take a more proactive approach to retention, targeting students who might otherwise fall through the cracks.
Key Benefits of AI in Student Retention
- Early Intervention: AI models identify at-risk students based on patterns that human advisors may miss, enabling timely intervention.
- Personalized Support: By analyzing individual student data, AI can recommend tailored academic resources, mentorship programs, and emotional support services.
- Data-Driven Decisions: Institutions can make more informed decisions about course offerings, faculty engagement, and student services based on predictive insights.
Examples of Predictive Factors in AI Models
- Academic Performance: Grade trends, homework completion, and test scores are analyzed to foresee potential academic struggles.
- Engagement Metrics: Data on class participation, online learning behaviors, and interaction with course materials help predict student involvement.
- Behavioral Patterns: Tracking attendance, social interactions, and mental health indicators can offer early signals of disengagement.
"AI-based predictive models can drastically improve student retention by identifying the specific needs of each student and offering timely, data-driven interventions."
Example of Predictive Model Application
Factor | Prediction Outcome | Recommended Action |
---|---|---|
Declining Grades | Potential for failing course | Offer tutoring services, schedule meeting with academic advisor |
Low Attendance | Increased risk of dropout | Send personalized reminders, arrange a check-in with a counselor |
Lack of Engagement | Reduced chance of completing program | Introduce peer mentoring, recommend study groups |
Automating Institutional Reporting with AI in Higher Education
In recent years, educational institutions have increasingly turned to artificial intelligence (AI) to streamline administrative tasks, including institutional reporting. The use of AI allows for faster, more accurate data processing and reporting, saving time and reducing human error. With the growing demand for real-time analytics, AI-driven tools provide higher education institutions with the ability to automatically generate detailed reports on student performance, financial health, enrollment trends, and faculty metrics.
AI-based systems can analyze vast datasets, producing comprehensive reports that would otherwise take weeks to compile. These automated processes enable university staff to focus on strategic decision-making rather than spending excessive time on routine data collection and analysis. By automating institutional reporting, universities improve efficiency, enhance decision-making, and maintain regulatory compliance without overburdening their staff.
Key Benefits of AI in Reporting
- Efficiency Gains: Automated reporting speeds up the process, allowing for more timely decision-making.
- Accuracy: AI tools reduce human errors, ensuring data consistency and reliability in reports.
- Cost Savings: By reducing manual labor, institutions can save on administrative costs.
How AI Tools Improve Reporting Systems
- Data Collection: AI can extract data from multiple sources, including student information systems, financial databases, and academic platforms.
- Data Analysis: AI algorithms can process complex data, identifying trends, patterns, and anomalies without human intervention.
- Report Generation: AI automatically formats and structures reports based on predefined templates, ensuring consistency across different departments.
Example: AI in Enrollment Reporting
Category | Traditional Method | AI-Driven Method |
---|---|---|
Data Collection | Manual entry of student records | Automatic extraction from student management systems |
Analysis | Manual trend analysis | Real-time data analysis with predictive modeling |
Reporting | Generated by administrative staff | Auto-generated and customized reports in minutes |
“AI-driven reporting systems help educational institutions improve the quality of decision-making by providing timely and accurate data that can guide future strategies.”
AI in Curriculum Design: Leveraging Data for Personalized Learning
Artificial intelligence has made significant strides in transforming the education sector, particularly in the realm of curriculum design. The integration of AI technologies enables educators to tailor learning experiences based on individual student data, ensuring a more personalized approach to education. By analyzing a vast array of student data, AI can identify patterns in learning behaviors, strengths, and areas of improvement, which can be leveraged to create dynamic and responsive curricula.
This shift from a one-size-fits-all model to a personalized learning approach is a direct result of AI's ability to process large amounts of data efficiently. As AI tools collect and analyze data on students' progress, preferences, and learning styles, they empower educators to adjust course content, pacing, and delivery methods to better meet the needs of each learner. This process not only increases engagement but also improves educational outcomes for diverse student populations.
How AI Enhances Curriculum Customization
AI’s role in curriculum design is multifaceted, offering several advantages for both students and educators. Below are some of the key benefits:
- Personalized Learning Paths: AI analyzes student performance to create customized learning journeys, allowing learners to progress at their own pace.
- Adaptive Content Delivery: AI adapts learning resources, such as assignments and assessments, to match individual learning speeds and styles.
- Predictive Analytics: AI predicts future learning behaviors, enabling educators to intervene early when students are at risk of falling behind.
- Data-Driven Insights: Teachers gain valuable insights into student performance, enabling informed decisions regarding curriculum adjustments.
Practical Examples of AI in Action
In practice, AI tools can be used to monitor various aspects of the curriculum, offering a deeper understanding of how students interact with content:
- Automated Assessment Feedback: AI tools provide real-time feedback on assignments, allowing students to address mistakes promptly and improve.
- Content Recommendation Systems: AI suggests additional resources or alternative learning materials based on a student's performance and interests.
- Collaborative Learning Environments: AI facilitates group dynamics by recommending teams based on complementary skill sets or shared learning goals.
Table: AI Tools and Their Impact on Learning
AI Tool | Impact on Learning |
---|---|
Adaptive Learning Platforms | Tailors content delivery to individual student needs, improving engagement and retention. |
AI-Powered Tutoring Systems | Provides one-on-one assistance, helping students master difficult concepts outside of class. |
Learning Analytics Dashboards | Visualizes student progress, enabling teachers to identify areas requiring intervention. |
"AI not only personalizes the learning experience but also empowers teachers to focus on the individual needs of each student, ultimately enhancing the educational process."
AI and Data Privacy: Striking the Balance in Higher Education
As artificial intelligence (AI) becomes an increasingly integral part of higher education, its potential to enhance learning and operational efficiency is undeniable. However, this technological advancement raises significant concerns regarding data privacy and security. Universities are leveraging AI tools to analyze student performance, streamline administrative tasks, and offer personalized educational experiences. But the question arises: how can institutions harness these benefits while ensuring the protection of sensitive personal data?
The integration of AI in higher education introduces a range of privacy challenges, especially when it comes to managing vast amounts of student data. Personal information, academic records, and behavioral patterns are often used to optimize AI systems, but this data must be handled with utmost care to avoid breaches or misuse. Balancing the need for insightful analytics with stringent security measures is crucial for institutions striving to maintain trust and comply with privacy regulations.
Key Considerations for Data Privacy in AI-Driven Higher Ed
- Data Collection and Usage: Institutions must clearly define what data is being collected and how it will be used by AI systems.
- Regulatory Compliance: Ensuring that AI applications adhere to data protection laws such as GDPR or FERPA is essential to avoid legal repercussions.
- Transparency and Consent: Students and staff should be fully informed about how their data will be used, with explicit consent obtained before processing.
Strategies for Protecting Privacy while Leveraging AI
- Data Anonymization: AI systems should anonymize data wherever possible to minimize the risk of identifying individuals.
- Decentralized Data Storage: Storing data in secure, localized systems can help reduce vulnerabilities associated with centralized databases.
- Continuous Monitoring: Institutions should implement ongoing monitoring to detect potential breaches or misuse of data.
“As AI continues to evolve, it’s essential that higher education institutions prioritize data privacy as a fundamental part of their AI strategy. Only by doing so can they ensure that innovation does not come at the expense of security.”
Impact of AI on Data Privacy: A Case Study
Aspect | AI-Driven Enhancement | Data Privacy Challenge |
---|---|---|
Personalized Learning | AI tailors content to individual student needs based on collected data. | Increased risk of exposing sensitive student information if not properly managed. |
Administrative Efficiency | AI automates administrative processes, reducing workload and errors. | Potential misuse of personal data in decision-making processes without oversight. |