Self-learning Ai Examples
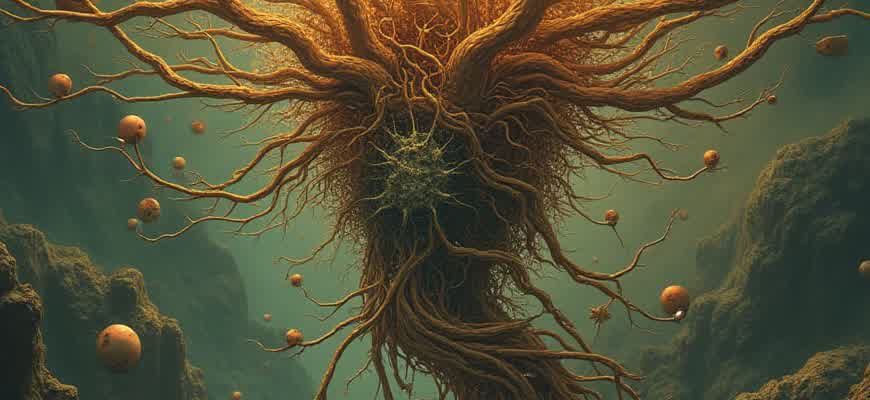
Self-learning algorithms have revolutionized artificial intelligence by allowing systems to improve their performance without human intervention. These algorithms use various methods such as reinforcement learning, neural networks, and unsupervised learning to autonomously learn from data and adapt to new situations.
Here are some prominent examples of autonomous learning AI applications:
- AlphaGo: A computer program developed by DeepMind, which learned to play the game of Go by playing against itself and improving its strategies.
- Self-driving cars: Autonomous vehicles use AI to learn from real-time traffic data and improve their navigation and decision-making processes.
- Robotic process automation (RPA): AI systems that autonomously learn repetitive tasks to optimize business processes.
Key Features of Self-learning AI:
Method | Application | Benefit |
---|---|---|
Reinforcement Learning | Robots learning tasks through trial and error | Improves decision-making over time |
Neural Networks | Image recognition in medical diagnosis | Higher accuracy and adaptability |
Unsupervised Learning | Customer segmentation in marketing | Discovers hidden patterns without labeled data |
"Self-learning AI enables machines to enhance their performance with minimal human input, opening the door to countless innovations across industries."
Examples of Self-Learning Artificial Intelligence
Self-learning artificial intelligence (AI) refers to systems that improve their performance and adapt based on new data without explicit human programming. These AI models can enhance their capabilities by recognizing patterns, testing hypotheses, and refining algorithms autonomously. This enables them to perform complex tasks, often surpassing human proficiency over time.
Various AI systems, such as reinforcement learning models and neural networks, demonstrate self-learning in diverse fields. These models continually improve through interaction with their environment or by processing large amounts of data. Below are some notable examples of self-learning AI applications.
Notable Applications of Self-Learning AI
- Autonomous Vehicles: AI in self-driving cars learns from real-time data to navigate complex environments, recognizing traffic signs, pedestrians, and obstacles.
- Personalized Recommendations: E-commerce platforms like Amazon use AI to tailor product suggestions by learning from users' browsing and purchasing history.
- Healthcare Diagnostics: AI systems in medical imaging evolve by analyzing new patient data, improving diagnostic accuracy over time.
- Financial Trading: AI algorithms in the stock market adapt to changing market conditions, learning from previous trade patterns to make informed decisions.
Key Features of Self-Learning AI Models
- Adaptation: Continuously improves by processing new data and refining its predictions.
- Autonomy: Operates without human intervention, making decisions and learning from experiences.
- Data-Driven: Learns from vast amounts of data, often leveraging deep learning or reinforcement learning techniques.
"The ability to learn from experience is what differentiates self-learning AI from traditional, static models. This allows for continual improvement and fine-tuning, which makes these models ideal for dynamic, unpredictable environments."
Comparison of Self-Learning AI Models
AI Type | Application | Learning Method |
---|---|---|
Reinforcement Learning | Robotics, Autonomous Vehicles | Learning through rewards and penalties |
Deep Learning | Medical Imaging, Speech Recognition | Learning from large datasets through neural networks |
Supervised Learning | Fraud Detection, Email Filtering | Learning from labeled data with guidance |
How Self-learning AI Can Automate Data Analysis for Businesses
Self-learning artificial intelligence (AI) is transforming the way businesses handle data by offering advanced automation capabilities. With its ability to analyze large datasets and adapt based on new information, AI can streamline data analysis processes, providing real-time insights and reducing human errors. These systems evolve over time, improving their performance and efficiency as they process more data, making them an invaluable asset for businesses across various industries.
By utilizing machine learning algorithms, businesses can automate complex data tasks such as trend detection, predictive analysis, and anomaly detection. This allows companies to make data-driven decisions faster, reducing the need for manual intervention and enabling employees to focus on higher-value activities. Below are key ways in which self-learning AI supports data analysis automation:
Key Benefits of Self-learning AI for Data Analysis
- Improved Accuracy: AI models continuously adjust based on new data, leading to more accurate predictions and insights.
- Faster Decision Making: Automation allows for quicker analysis, helping businesses respond to market changes in real-time.
- Cost Reduction: By reducing the reliance on manual data analysis, AI can help businesses cut operational costs.
- Scalability: Self-learning AI systems can handle increasing amounts of data without compromising performance.
Examples of Automated Data Analysis Tasks
- Customer Sentiment Analysis: AI can analyze customer feedback from various sources, identifying trends and providing actionable insights.
- Sales Forecasting: Using historical data, AI can predict future sales trends with a high degree of accuracy.
- Anomaly Detection: AI systems can automatically identify irregular patterns in data that may indicate potential issues, such as fraud or system malfunctions.
How Self-learning AI Helps Businesses Save Time and Resources
Task | Manual Approach | AI-Driven Automation |
---|---|---|
Data Cleaning | Time-consuming, prone to human error | Automated, more accurate and faster |
Data Insights | Requires extensive human analysis | Instant, driven by AI algorithms |
Reporting | Manual report generation, time-intensive | Automatic generation of detailed reports |
"With AI-powered data analysis, businesses can automate repetitive tasks, uncover valuable insights, and improve decision-making without increasing human workload."
Leveraging Autonomous AI for Enhanced Customer Support: Real-World Applications
As businesses increasingly look to streamline operations, the integration of self-learning artificial intelligence (AI) into customer support has proven to be a game changer. AI systems that can adapt to new information and improve over time offer unprecedented efficiencies in addressing customer needs. These systems can continuously analyze interactions, detect patterns, and optimize responses based on evolving customer expectations. Implementing such technology not only reduces the burden on human agents but also provides customers with faster, more accurate solutions.
In real-world applications, self-learning AI is revolutionizing customer service across various industries. By automating common inquiries and leveraging natural language processing, AI platforms can assist with everything from basic troubleshooting to complex service issues. Moreover, these AI models can be trained on past interactions, ensuring they evolve and improve with every customer engagement.
Key Use Cases of Self-Learning AI in Customer Support
- Automated Ticket Routing: AI can automatically categorize and prioritize support tickets, directing them to the appropriate human agent or resolving them autonomously.
- Personalized Customer Interactions: By analyzing past interactions, AI can tailor responses based on customer history, ensuring more relevant and effective communication.
- 24/7 Support Availability: Self-learning AI systems can provide round-the-clock customer service, handling issues at any time without the need for human intervention.
Benefits of Self-Learning AI in Customer Support
Benefit | Description |
---|---|
Efficiency | AI reduces response times by automating common inquiries, freeing human agents to focus on more complex issues. |
Scalability | Self-learning systems can easily scale to handle increased customer interactions without additional human resources. |
Continuous Improvement | The AI system refines its performance over time, learning from every interaction and optimizing future responses. |
"The true potential of self-learning AI lies in its ability to not only handle routine customer support tasks but to also continuously improve and adapt to new challenges."
Challenges and Considerations
- Data Privacy: Ensuring that AI systems comply with privacy regulations and handle sensitive customer data responsibly is critical.
- Training Data Quality: The effectiveness of AI systems heavily depends on the quality and diversity of the data they are trained on.
- Human-AI Collaboration: While AI can handle routine tasks, human agents remain essential for complex or nuanced situations that require empathy and judgment.
Enhancing Tailored Marketing Campaigns with Self-learning AI
Self-learning AI has become a game changer in creating personalized marketing strategies. By constantly analyzing customer data and adjusting to their preferences, AI models can deliver highly relevant and engaging content, improving customer interaction and satisfaction. This approach helps businesses refine their marketing efforts with a higher degree of precision, ensuring that every message reaches the right person at the right time.
These AI systems can dynamically optimize campaigns, shifting from static models to flexible and adaptive approaches. As AI learns more about consumer behavior, it tailors recommendations, advertisements, and offers that resonate with individual customers, leading to improved conversion rates and customer retention. In essence, self-learning algorithms turn data into actionable insights that directly influence marketing performance.
Key Benefits of AI in Personalized Marketing
- Real-time adjustments: AI continuously updates marketing strategies based on ongoing consumer interactions.
- Deep customer insights: AI analyzes behavior patterns to predict needs and preferences with greater accuracy.
- Improved targeting: AI refines audience segments, delivering the right content to the most relevant customers.
- Cost efficiency: By automating personalization, businesses can optimize their marketing budget and achieve better ROI.
Practical Applications
- Recommendation Systems: AI-powered algorithms suggest products or services based on a user's browsing history, preferences, and past purchases.
- Dynamic Pricing: AI can adjust prices in real-time according to market conditions, competition, and customer willingness to pay.
- Email Campaign Optimization: Personalized email content and offers are generated based on individual customer data, increasing open rates and engagement.
"Self-learning AI in marketing empowers businesses to create dynamic and individualized campaigns, ultimately driving customer satisfaction and business growth."
Performance Metrics with AI
Metric | Before AI Integration | After AI Integration |
---|---|---|
Conversion Rate | 4% | 10% |
Customer Retention | 65% | 85% |
Marketing ROI | 1.2 | 3.5 |
Using Self-learning AI for Predictive Maintenance in Manufacturing
In the manufacturing industry, machine downtime can lead to significant losses, both in terms of productivity and cost. Predictive maintenance powered by self-learning artificial intelligence (AI) is emerging as a vital tool for minimizing these disruptions. Self-learning AI systems continuously analyze real-time sensor data from machinery, recognizing patterns and predicting when maintenance is required before failures occur. This technology not only reduces operational downtime but also extends the life of equipment, ultimately leading to cost savings and higher operational efficiency.
By leveraging self-learning algorithms, manufacturing plants can move away from traditional reactive maintenance methods, which often result in unplanned outages. Instead, AI systems can predict potential issues, allowing for scheduled interventions. This approach significantly improves overall system reliability and minimizes unnecessary maintenance activities. Below are key components and benefits of using self-learning AI for predictive maintenance in manufacturing:
- Data Collection: Continuous monitoring of machinery using sensors to gather data on temperature, vibrations, pressure, and other key indicators.
- Real-time Analysis: AI algorithms process this data, detecting deviations from normal patterns and identifying potential failure risks.
- Automated Alerts: The system generates maintenance alerts for operators, highlighting potential problems before they escalate.
AI-driven predictive maintenance not only helps avoid unplanned downtime but also ensures that spare parts and human resources are available when needed.
Key benefits of self-learning AI include:
- Cost Efficiency: Minimizes costly unplanned repairs by addressing issues early.
- Increased Machine Lifespan: Prevents excessive wear and tear by predicting and addressing maintenance needs in a timely manner.
- Optimized Resource Allocation: Maintenance teams are only deployed when necessary, optimizing labor and parts management.
Implementation of this technology leads to smarter manufacturing environments that can adapt, learn, and self-optimize, driving both operational excellence and cost-effectiveness.
Feature | Description |
---|---|
Real-time Monitoring | Continuous data collection from sensors installed on machinery. |
Failure Prediction | AI models predict potential equipment failures based on historical data patterns. |
Automated Decision-Making | AI systems generate maintenance recommendations, enabling timely actions. |
Optimizing Content Recommendations Through Self-learning AI Algorithms
Self-learning AI algorithms have revolutionized the way content is recommended to users by adapting to their preferences over time. These systems leverage large datasets to continuously refine their suggestions based on individual behavior, improving user engagement and satisfaction. As users interact with content, the AI adjusts its recommendations, ensuring they remain relevant and personalized.
The core strength of these algorithms lies in their ability to evolve. Unlike traditional methods that rely on fixed rules or predefined parameters, self-learning algorithms can modify their models autonomously, learning from new data and adjusting recommendations in real time. This leads to more accurate, user-centric content delivery.
Key Mechanisms of Self-learning AI in Content Optimization
- Behavioral Analysis: The algorithm tracks user interactions (clicks, likes, shares, etc.) to understand preferences and predict future behavior.
- Contextual Learning: Self-learning AI can factor in contextual elements, such as time of day or location, to refine content suggestions.
- Collaborative Filtering: The system identifies patterns by analyzing similar users' behaviors, helping recommend content that appeals to similar audiences.
"Self-learning AI systems not only track user behavior but also predict trends, improving content personalization by anticipating what users might enjoy next."
Impact on User Experience
- Increased Engagement: By providing relevant recommendations, users spend more time interacting with content, enhancing platform engagement.
- Higher Retention Rates: Personalized content encourages users to return, as they feel understood by the system.
- Better Conversion: With more targeted suggestions, self-learning AI can drive higher conversions in e-commerce and media platforms.
Example Comparison of AI Algorithms
Algorithm Type | Recommendation Method | Key Advantage |
---|---|---|
Collaborative Filtering | Recommends based on similarities between users | Effective for discovering new content based on user group trends |
Content-Based Filtering | Recommends similar content based on user history | Personalized content suggestions based on past preferences |
Hybrid Model | Combines collaborative and content-based approaches | More balanced recommendations, reducing bias from either method |
Utilizing Self-learning AI for Detecting Fraud in Financial Systems
The integration of self-learning artificial intelligence in fraud detection is transforming the landscape of financial security. Traditional methods rely on predefined rule-based systems, but they often struggle to adapt to evolving fraudulent techniques. By leveraging AI models that continuously learn from data, financial institutions can improve their ability to detect and prevent fraud in real-time.
Self-learning AI can analyze large volumes of transactions, identify patterns, and autonomously adapt to new threats without manual intervention. This dynamic learning capability allows these systems to not only detect known fraudulent activities but also uncover previously unseen behaviors that indicate potential fraud. The main advantage lies in the AI's ability to evolve alongside fraudsters, reducing the time gap between attack occurrence and detection.
Key Benefits of AI-driven Fraud Detection
- Real-time detection: AI models can analyze transactions in real-time, identifying suspicious activity as it occurs.
- Adaptive learning: Self-learning algorithms continuously update their models based on new data, staying ahead of evolving fraud strategies.
- Reduced false positives: With better accuracy, AI minimizes unnecessary alerts, allowing security teams to focus on genuine threats.
How Self-learning AI Works in Fraud Detection
- Data collection: The AI system gathers data from a variety of sources, including transaction logs, user behavior, and historical fraud cases.
- Pattern recognition: Using machine learning algorithms, the AI detects patterns and anomalies that could indicate fraudulent activity.
- Continuous learning: The system updates its models as new data is fed in, adapting to changes in fraud tactics.
Self-learning AI significantly enhances fraud detection by reducing the reliance on predefined rules and allowing systems to autonomously adjust to new fraud patterns. This adaptability is critical in combating increasingly sophisticated financial crimes.
AI Fraud Detection Performance Metrics
Metric | Impact |
---|---|
Detection Speed | AI detects fraudulent transactions instantly, minimizing financial loss. |
Accuracy | AI algorithms improve over time, reducing false positives and increasing detection accuracy. |
Scalability | AI systems can scale to handle millions of transactions without degradation in performance. |
Scaling Healthcare Solutions with Self-learning AI for Diagnosis Assistance
In the rapidly evolving healthcare sector, integrating self-learning artificial intelligence (AI) into diagnostic processes offers unprecedented opportunities to enhance accuracy and efficiency. These advanced systems can continuously improve through exposure to vast amounts of medical data, making them increasingly effective at identifying conditions early and suggesting treatment options. By incorporating such technologies, healthcare professionals can provide more personalized and precise care, while also reducing the workload of medical staff.
One of the major advantages of self-learning AI in healthcare is its ability to scale across different medical specialties and patient populations. As AI systems learn from diverse datasets, they can offer generalized and specific support for various diagnoses, improving both speed and reliability in clinical decision-making. In addition, the ability of AI to analyze data patterns from multiple sources, including medical records, imaging, and genetic information, can lead to groundbreaking insights in personalized medicine.
Key Benefits of AI in Healthcare Diagnostics
- Enhanced Diagnostic Accuracy: Self-learning AI continuously improves through training with large, diverse datasets, reducing human error in diagnoses.
- Scalability: AI systems can be scaled to support multiple specialties and geographies, making it feasible to address healthcare challenges globally.
- Real-time Insights: AI provides immediate recommendations based on current data, supporting quick decisions in high-pressure situations.
Examples of Self-learning AI in Healthcare
- Radiology: AI algorithms help in detecting anomalies in X-rays, CT scans, and MRIs, improving the detection of conditions like cancer or fractures.
- Pathology: AI models analyze tissue samples for early signs of diseases such as cancer, providing more accurate and faster results.
- Predictive Analytics: AI systems predict patient outcomes based on historical data, helping clinicians identify those at risk for conditions like heart disease.
“AI systems not only assist with diagnoses but also improve operational efficiencies, enabling healthcare providers to focus on patient care rather than administrative tasks.”
Challenges to Consider
Despite the potential of self-learning AI in healthcare, several challenges need to be addressed before widespread adoption:
Challenge | Impact |
---|---|
Data Privacy and Security | Ensuring patient data is kept secure while being used to train AI models is a critical issue. |
Bias in Training Data | If AI systems are trained on biased datasets, they could produce inaccurate or unequal results for certain populations. |
Integration into Clinical Workflow | AI solutions must be seamlessly integrated into existing healthcare systems to ensure efficient use by medical professionals. |