What Is Deep Learning in Education
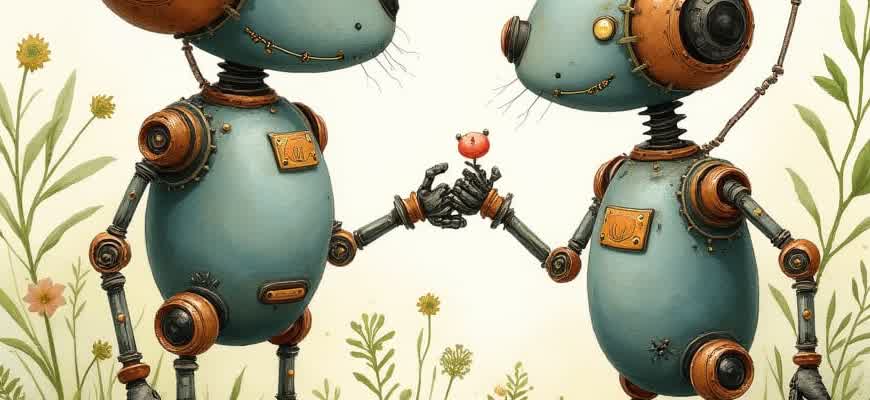
Deep learning technologies have emerged as a powerful tool in the transformation of education. They utilize advanced algorithms to mimic the brain's neural networks, enabling systems to process and analyze large sets of data to make intelligent decisions. In education, this technology can be applied in various ways to enhance both teaching and learning experiences.
One of the key areas where deep learning is being integrated is personalized learning. By analyzing individual students' progress and learning patterns, systems powered by deep learning can adapt the learning materials to better suit each student's needs. This offers a more tailored approach, unlike traditional education systems, which often follow a one-size-fits-all model.
- Custom learning pathways
- Data-driven insights for student performance
- Automation of grading and assessments
"Deep learning allows for personalized experiences, transforming the way we approach education."
Moreover, deep learning can be used to create intelligent tutoring systems. These systems are designed to simulate human-like tutoring, providing students with interactive lessons and real-time feedback. They are not limited by time or location, making them accessible to a wider range of students.
- Interactive lesson plans
- Immediate feedback on assignments
- Real-time adjustments to difficulty levels
Application | Benefit |
---|---|
Personalized Learning | Improved learning outcomes |
Automated Grading | Faster feedback, less teacher workload |
Intelligent Tutoring | More accessible learning resources |
How Deep Learning Enhances Custom Learning Journeys
Deep learning technologies have revolutionized the ability to tailor educational experiences to individual needs. By analyzing vast amounts of student data, these systems can identify unique learning styles, preferences, and challenges. As a result, learners receive content that adapts in real-time, optimizing engagement and retention. This personalization isn't just limited to the content itself but also extends to the pace and method of delivery, making education more flexible and accessible.
As deep learning models process data, they gain a better understanding of how each student learns best. The system can adjust the level of difficulty, suggest alternative learning materials, or recommend targeted exercises. This level of precision is impossible with traditional teaching methods, making deep learning a powerful tool for creating more effective and efficient educational experiences.
Key Ways Deep Learning Improves Personalized Learning:
- Adapting Content to Learning Styles: Deep learning identifies patterns in how students interact with learning materials and adjusts content accordingly.
- Real-time Feedback: Immediate insights help instructors or automated systems to offer personalized suggestions or corrections to students.
- Individualized Pacing: Students can progress at their own pace, with systems adjusting the difficulty of tasks based on their performance.
- Predictive Analysis: Deep learning can predict future challenges a student might face, allowing for preemptive intervention.
Deep learning algorithms provide a dynamic, responsive educational environment that allows every learner to thrive at their own pace and according to their own needs.
Examples of Personalized Learning in Action:
Feature | Impact |
---|---|
Adaptive Assessments | Assessments adjust based on student performance, ensuring questions match the student's current understanding. |
Custom Recommendations | Based on previous learning behavior, students are given tailored resources (videos, readings, quizzes) to improve understanding. |
Dynamic Content Adjustment | Learning materials are continuously adjusted based on real-time analysis of student progress, providing targeted challenges. |
Leveraging Deep Learning for Adaptive Assessment Tools
Deep learning can play a crucial role in transforming assessment tools used in education by adapting them to individual learner needs. By analyzing large datasets of student performance, deep learning models can identify patterns and provide personalized assessments that evolve as a student progresses. This dynamic approach helps ensure that the assessment process is not only more efficient but also more aligned with the learner’s current level and abilities.
Adaptive assessment tools powered by deep learning offer several advantages. These systems continually update their algorithms to match the learner’s cognitive state, adjusting the difficulty and content of questions in real-time. As a result, the assessments become more responsive, offering a highly customized experience for each student, which ultimately leads to more accurate evaluations of their skills.
Key Benefits of Adaptive Assessment Tools
- Personalized Learning: Tailors assessments to individual progress, ensuring that each student is challenged appropriately.
- Real-Time Feedback: Provides immediate insights into areas where the student needs improvement, supporting timely intervention.
- Efficient Resource Allocation: Allows educators to focus on specific learning gaps, making resource usage more effective.
How Deep Learning Models Adapt Assessments
- Data Collection: Collect data from a variety of assessments, including responses, time spent on each task, and accuracy.
- Pattern Recognition: Deep learning models analyze data to identify performance trends, such as strengths and weaknesses.
- Adaptive Questioning: Based on the student’s performance, the system adjusts the difficulty of subsequent questions, creating a unique assessment pathway for each student.
“By continuously analyzing learner data, deep learning models help design assessments that evolve in real-time, providing a more accurate measure of a student’s abilities.”
Comparison of Traditional and Adaptive Assessment Systems
Feature | Traditional Assessment | Adaptive Assessment |
---|---|---|
Customization | Standardized for all students | Personalized for each learner |
Feedback Speed | Delayed, often after grading | Instant, during the assessment |
Difficulty Level | Fixed questions | Dynamic, adjusts based on performance |
Integrating Deep Learning in Virtual Classrooms and Online Courses
Deep learning technologies offer new opportunities for enhancing the learning experience in online education platforms. By utilizing algorithms that adapt to individual learning patterns, virtual classrooms can be personalized to meet the needs of each student. These systems can analyze students' progress, predict future challenges, and adjust content delivery accordingly, providing a more engaging and efficient learning environment.
One of the most significant benefits of deep learning in virtual classrooms is the ability to provide real-time feedback and tailored instruction. This helps instructors track student performance, identify gaps in understanding, and intervene proactively. Additionally, deep learning can enable automated grading, reducing the administrative burden on educators while maintaining objectivity in assessments.
Applications of Deep Learning in Virtual Learning Environments
- Personalized Learning: Deep learning can create adaptive learning paths based on individual student behavior, performance, and preferences.
- Automated Grading: Machine learning models can evaluate assignments and exams with high accuracy, offering instant feedback to students.
- Content Recommendation: Algorithms can suggest supplementary materials to students based on their learning history, improving retention and understanding.
- Intelligent Tutoring Systems: Virtual tutors powered by deep learning can guide students through complex topics, answering questions and providing explanations in real-time.
Challenges and Considerations
"Although deep learning presents immense potential in online education, it is essential to address privacy concerns, data security, and the need for equitable access to technology."
- Data Privacy: With the collection of detailed data about students’ interactions, it is crucial to ensure that this data is securely stored and not misused.
- Bias in Algorithms: Deep learning models can inadvertently reinforce biases if trained on non-representative datasets, leading to unfair outcomes for certain student groups.
- Technology Access: Not all students may have access to the necessary hardware or internet connectivity to fully benefit from AI-powered learning systems.
Examples of Deep Learning Integration
Tool/Technology | Application | Benefit |
---|---|---|
AI-Based Tutoring Systems | Real-time feedback and adaptive problem-solving assistance | Personalized learning experience for each student |
Automated Content Delivery | Customized lessons based on individual performance data | Increased engagement and optimized learning paths |
Learning Analytics Tools | Tracking student progress and predicting future challenges | Proactive interventions and data-driven insights for educators |
How AI-Driven Content Enhances Student Engagement
Artificial Intelligence (AI) has brought a significant transformation to educational methodologies by improving how content is delivered. Deep learning, as a subset of AI, is particularly effective in tailoring learning experiences to individual needs. By analyzing patterns and behaviors, AI-driven systems can create dynamic and interactive content that resonates with students on a deeper level. This personalization leads to increased student engagement, as the content becomes more relevant, challenging, and stimulating.
Through intelligent algorithms, deep learning systems can adapt content in real-time, adjusting the difficulty, presentation style, and pacing based on individual progress. This not only fosters a more engaging learning environment but also ensures that students remain motivated to continue their education without feeling overwhelmed or bored. The power of deep learning in education lies in its ability to make the learning process feel more interactive and responsive.
Key Benefits of AI-Driven Content for Engagement
- Personalized Learning Paths: AI systems track student performance and create custom learning pathways, ensuring each learner receives content suited to their needs and abilities.
- Real-Time Feedback: Instant feedback keeps students on track, offering insights into their progress and suggesting areas for improvement.
- Interactive Elements: AI can incorporate gamification, simulations, and multimedia elements to make learning more immersive.
"AI transforms education by allowing content to evolve with each student's learning style, making the classroom experience more engaging and impactful."
Impact of AI-Driven Engagement Strategies
- Improved student participation due to relevant and adaptive content.
- Higher retention rates as students engage with personalized materials that match their pace and level of understanding.
- More efficient learning processes with tailored feedback and continuous adjustments based on performance.
AI Feature | Impact on Engagement |
---|---|
Personalized Learning | Keeps students motivated by offering content that matches their skills. |
Real-Time Adjustments | Enhances engagement through constant adaptation of difficulty levels. |
Interactive Content | Encourages active participation with multimedia, simulations, and games. |
Utilizing Deep Learning for Early Detection of Learning Disabilities
Early identification of learning disabilities is crucial for providing timely interventions and tailored support for students. Traditional methods of diagnosis, which rely on subjective assessments and observational evaluations, often lead to delayed or inaccurate results. Deep learning offers a more objective and data-driven approach, allowing educators and specialists to identify learning disabilities at an earlier stage and implement appropriate strategies for support.
By analyzing large sets of data, including behavioral patterns, academic performance, and cognitive assessments, deep learning models can detect subtle signs of learning difficulties that might otherwise go unnoticed. These models can be trained to recognize patterns in student responses, learning behaviors, and even eye movement during reading tasks. Such technologies help in identifying disabilities like dyslexia, ADHD, and other cognitive challenges at an early stage.
Key Advantages of Using Deep Learning in Early Detection
- Objective Assessments: Deep learning models analyze data without human biases, providing more accurate and consistent evaluations of student abilities.
- Real-time Monitoring: These models can track progress and performance continuously, ensuring that potential issues are identified promptly.
- Personalized Learning: With early detection, educators can develop individualized learning plans tailored to the specific needs of students.
How Deep Learning Works for Early Detection
- Data Collection: Gather extensive data sets from student assessments, classroom interactions, and behavioral observations.
- Model Training: Use deep learning algorithms to train models on the collected data, enabling them to identify patterns related to learning difficulties.
- Pattern Recognition: Once trained, the models can detect irregularities or signs of disabilities in a student's learning process, such as inconsistencies in performance or abnormal cognitive patterns.
Example: Comparing Traditional vs Deep Learning Approaches
Approach | Advantages | Challenges |
---|---|---|
Traditional Methods | Personalized evaluations, familiar to educators | Subjective, time-consuming, potential for bias |
Deep Learning Models | Objective, scalable, real-time insights | Requires large datasets, technical expertise for implementation |
"The integration of deep learning into educational settings can significantly enhance the speed and accuracy of diagnosing learning disabilities, providing students with the opportunity for earlier interventions and better outcomes."
Optimizing Administrative Tasks with Deep Learning Algorithms
In educational institutions, administrative tasks can be time-consuming and complex, requiring significant human resources. By leveraging deep learning techniques, institutions can streamline many of these processes, enhancing efficiency and reducing errors. Deep learning models can automate routine administrative duties such as scheduling, data entry, and student enrollment, saving valuable time for staff to focus on more critical activities.
These algorithms can process vast amounts of data, recognizing patterns and making decisions that traditionally required manual intervention. Deep learning can also predict trends, such as student performance, helping administrators make proactive decisions. The following are key areas where deep learning algorithms can optimize administrative workflows:
- Student Enrollment and Registration: Automating the processing of student data, reducing manual errors and ensuring smoother registration processes.
- Schedule Management: Analyzing past data to generate optimized class schedules that minimize conflicts and optimize room utilization.
- Communication Systems: Using natural language processing to automate responses to student and parent queries, improving communication efficiency.
- Data Analysis and Reporting: Generating real-time reports on various administrative metrics, from student performance to resource allocation.
Deep learning algorithms can significantly reduce the administrative burden on staff by automating repetitive tasks and improving decision-making accuracy.
Applications in Task Optimization
Task | Deep Learning Role | Benefits |
---|---|---|
Student Enrollment | Automated data processing, error detection | Reduced manual errors, faster registration |
Class Scheduling | Pattern recognition for schedule optimization | Minimized conflicts, improved resource usage |
Report Generation | Automated generation of performance reports | Real-time insights, improved decision-making |
Transforming Teacher Support Systems with Deep Learning Technology
Deep learning is reshaping the landscape of education by providing innovative solutions for teacher support systems. With the increasing complexity of classrooms and diverse student needs, traditional methods of teacher support are no longer sufficient. Leveraging deep learning allows educators to receive real-time insights and tailored assistance, enhancing their teaching strategies and improving student outcomes.
By implementing deep learning technology, educational institutions can create more personalized, effective support networks for teachers. These systems can automate administrative tasks, predict student performance, and offer recommendations for professional development, leading to a more streamlined and efficient teaching environment.
Key Benefits of Deep Learning in Teacher Support
- Real-time Analytics: Deep learning algorithms can process large datasets quickly, providing teachers with up-to-date information about student progress and classroom dynamics.
- Personalized Guidance: Systems can recommend specific teaching strategies based on student data, enabling more effective and individualized instruction.
- Automated Administrative Tasks: Tasks such as grading and report generation can be automated, allowing teachers to focus more on instruction and less on administrative work.
How It Works
- Data Collection: Teacher performance, student interactions, and classroom dynamics are continuously monitored through various sensors and data sources.
- Model Training: Deep learning models are trained using this data to identify patterns and trends that can inform decision-making.
- Predictive Analysis: Based on these patterns, the system can predict student outcomes and suggest personalized teaching strategies for the teacher.
Example: Deep Learning Integration in Classroom Management
Traditional Methods | Deep Learning-Enhanced Methods |
---|---|
Manual tracking of student progress | Automated, real-time tracking and feedback |
Generic, one-size-fits-all teaching methods | Personalized teaching suggestions based on student data |
Time-consuming administrative tasks | Automated grading and report generation |
“By adopting deep learning technologies, teachers can transform their approach to instruction, creating more dynamic and responsive learning environments.”
The Future of Curriculum Development Using Deep Learning Models
Deep learning models are transforming the way educational curricula are developed, offering new opportunities for personalized and adaptive learning experiences. These models have the potential to automate the creation of curriculum content, identify learning gaps, and even predict the future needs of students. By analyzing vast amounts of data from student interactions, deep learning algorithms can provide insights into the most effective teaching methods, materials, and pacing for individual learners or groups of students.
As deep learning models continue to evolve, they could redefine the role of educators, shifting from content delivery to the facilitation of personalized learning paths. The integration of these models into curriculum development will enable more dynamic, student-centered approaches, where learning experiences are continuously refined based on real-time data analysis.
Key Opportunities in Curriculum Development
- Personalized Learning: Deep learning can tailor content to the individual learning needs, pace, and preferences of students.
- Real-Time Adaptation: Curriculum can be adjusted based on ongoing student performance, ensuring the right level of challenge and support.
- Improved Engagement: Interactive learning materials powered by deep learning algorithms can enhance student engagement and motivation.
Challenges and Considerations
- Data Privacy: The use of student data for training deep learning models must be carefully managed to ensure privacy and security.
- Bias in Algorithms: There is a risk that deep learning models may perpetuate biases in educational content or assessment.
- Teacher Training: Educators need to be equipped with the skills to effectively integrate and interpret deep learning-driven tools in the classroom.
"As deep learning becomes more integrated into educational systems, the potential for creating adaptive, scalable, and personalized learning experiences grows exponentially."
Future Implications
Area | Potential Impact |
---|---|
Curriculum Customization | Curricula can be automatically adjusted for students’ strengths and weaknesses. |
Learning Pathways | Personalized pathways based on deep learning predictions can guide students through their educational journey. |
Assessment | Continuous and real-time assessments can be integrated, reducing the reliance on traditional exams. |