Best Ai Platform for Finance
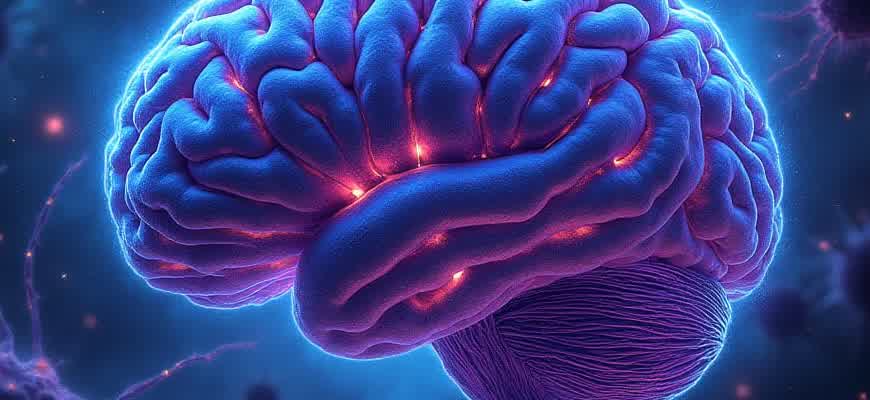
As the financial industry continues to evolve, AI platforms are becoming essential tools for institutions to stay competitive. These platforms help streamline operations, enhance decision-making, and predict market trends with remarkable accuracy. Below, we explore some of the top AI platforms that are reshaping finance.
1. IBM Watson stands as one of the most robust AI platforms for financial services. It offers powerful tools for data analytics, fraud detection, and customer insights, making it an invaluable asset for banks and insurance companies.
"IBM Watson is designed to analyze complex data and provide actionable insights in real-time, empowering businesses to make informed decisions faster." – AI Review
2. Google Cloud AI is increasingly used in financial markets for predictive analytics and trading algorithms. Its advanced machine learning capabilities help companies optimize their operations and create smarter financial strategies.
- Advanced Machine Learning Tools
- Scalable Data Infrastructure
- Integration with Existing Systems
3. Microsoft Azure AI offers a comprehensive set of AI-powered services that support everything from risk management to algorithmic trading. Its cloud-based solutions allow financial institutions to enhance productivity while reducing operational costs.
- Real-time Fraud Detection
- Personalized Customer Experiences
- Predictive Analytics for Market Trends
Platform | Key Features |
---|---|
IBM Watson | Data Analytics, Fraud Detection, Customer Insights |
Google Cloud AI | Predictive Analytics, Trading Algorithms |
Microsoft Azure AI | Risk Management, Personalized Experiences |
Top AI Platforms for Financial Sector: A Comprehensive Overview
Artificial intelligence is revolutionizing the finance industry, offering numerous applications ranging from fraud detection to automated trading. As financial institutions and companies continue to explore AI-driven solutions, it's essential to choose the right platform that aligns with specific needs such as data analysis, risk management, and customer service.
This guide outlines the best AI platforms currently shaping the financial landscape. We'll focus on features that can help businesses improve operational efficiency, reduce risk, and enhance decision-making processes through advanced machine learning and data analytics tools.
Key AI Platforms for Finance
- IBM Watson Financial Services: A comprehensive AI platform offering machine learning, data analytics, and cognitive computing to enhance decision-making and automate financial operations.
- Google Cloud AI for Finance: Provides AI-driven solutions for big data processing, financial forecasting, and automated compliance checks.
- Microsoft Azure AI: A powerful cloud-based AI platform offering predictive analytics, fraud detection, and risk management tools tailored for the finance sector.
Features to Look for in an AI Platform for Finance
- Data Security: The platform should comply with industry regulations and offer robust security features to protect sensitive financial data.
- Scalability: The ability to scale the platform as business needs grow is essential. Choose a platform that can handle large datasets and high transaction volumes.
- Integration Capabilities: Ensure that the AI platform can seamlessly integrate with existing financial systems and third-party tools.
- Real-Time Analytics: The platform should provide real-time insights, enabling quick decision-making and immediate action based on financial data.
Important: Choose a platform that aligns with your organization's long-term goals and regulatory requirements. Always consider future growth and ensure the AI platform can scale accordingly.
Comparison of AI Platforms for Finance
Platform | Key Features | Best For |
---|---|---|
IBM Watson | Machine learning, cognitive computing, fraud detection | Risk management and compliance |
Google Cloud AI | Big data processing, predictive analytics, financial forecasting | Data-driven financial analysis |
Microsoft Azure AI | Predictive analytics, fraud detection, risk management | Financial decision-making and automation |
Choosing the Right AI Tool for Financial Analysis
In the financial industry, the selection of an appropriate AI tool for analysis can significantly enhance decision-making processes. Financial professionals must focus on platforms that offer precision, scalability, and advanced analytical capabilities tailored to the unique requirements of financial markets. AI tools that provide robust data processing, real-time insights, and predictive models are key to driving profitability and reducing risks.
When evaluating AI tools, it’s essential to consider factors such as the platform’s integration with existing systems, ease of use, and the depth of machine learning models offered. With the rise of automated financial forecasting, risk assessment, and fraud detection, selecting the right AI solution can provide a competitive edge in today's fast-paced market.
Key Considerations in Selecting an AI Tool
- Data Accuracy - Ensure the platform uses high-quality, clean data to provide reliable insights.
- Scalability - Choose tools that can grow with your financial institution’s evolving needs.
- Real-time Analysis - The ability to process and analyze data in real time is critical for effective decision-making.
- Integration - Ensure seamless integration with existing financial systems and tools.
Evaluating AI Platforms: Key Metrics
Criteria | Importance | Explanation |
---|---|---|
Model Accuracy | High | Accurate predictions and insights are essential for financial decision-making. |
Speed of Data Processing | High | Real-time data analysis ensures faster response to market changes. |
Customizability | Medium | The ability to tailor the platform to specific financial goals and strategies. |
Security | Critical | Ensure that the platform adheres to industry standards for data privacy and security. |
Important: A robust AI tool should offer advanced security features, such as encryption and access control, to protect sensitive financial data.
How AI Platforms Enhance Risk Management in Finance
Artificial intelligence has revolutionized risk management in the financial sector by offering powerful tools to assess, predict, and mitigate potential threats. AI platforms analyze large datasets in real-time, enabling financial institutions to identify risk factors much faster than traditional methods. Machine learning algorithms detect patterns in historical data, allowing for more accurate risk assessments and proactive decision-making.
These platforms use advanced predictive models to provide insights into market volatility, credit risk, fraud detection, and compliance issues. By automating repetitive tasks, AI also frees up human resources, enabling financial professionals to focus on more complex problems. This significantly improves operational efficiency and reduces human error in risk management processes.
Key Advantages of AI in Risk Management
- Predictive Analytics: AI models forecast potential risks, including market fluctuations, credit defaults, and liquidity issues.
- Fraud Detection: Machine learning algorithms can identify suspicious transactions by analyzing patterns and detecting anomalies in real-time.
- Credit Scoring: AI platforms evaluate the creditworthiness of borrowers more accurately by considering a wider range of factors, reducing default risks.
- Regulatory Compliance: AI helps monitor and ensure compliance with ever-changing regulations by automating compliance checks and flagging violations.
AI vs Traditional Risk Management
Aspect | Traditional Risk Management | AI-Driven Risk Management |
---|---|---|
Data Analysis | Manual analysis of historical data | Automated analysis of large-scale, real-time data |
Risk Detection | Periodic risk assessment | Continuous monitoring and real-time risk detection |
Decision Speed | Slower, human-dependent decision-making | Instantaneous decision-making based on AI models |
Fraud Detection | Reactive fraud detection | Proactive fraud prevention with anomaly detection |
"AI platforms are transforming risk management by providing financial institutions with predictive insights, enabling faster and more accurate responses to emerging threats."
Utilizing Machine Learning for Predictive Financial Models
Machine learning has emerged as a critical tool in developing predictive financial models, enabling financial institutions to anticipate market trends and make data-driven decisions. By leveraging historical financial data, machine learning algorithms can uncover patterns that might otherwise remain hidden. These algorithms enhance the accuracy and speed of predictions, making them invaluable in risk management, portfolio optimization, and fraud detection.
In particular, machine learning techniques allow for real-time analysis of vast amounts of financial data, providing insights that support better decision-making. Predictive models powered by machine learning can forecast asset prices, market volatility, and consumer behavior with remarkable precision, enhancing overall investment strategies and operational efficiency.
Key Benefits of Machine Learning in Financial Prediction
- Improved Accuracy: ML models learn from historical data and continuously adjust, providing more precise forecasts over time.
- Real-time Analysis: Machine learning can process large datasets quickly, allowing for near-instantaneous predictions and analysis.
- Risk Management: Predictive models help identify potential risks early, reducing exposure to financial downturns.
Popular Machine Learning Techniques for Financial Modeling
- Linear Regression: Often used to predict the relationship between different financial variables, such as stock prices and interest rates.
- Decision Trees: Useful for decision-making, identifying key factors that influence financial outcomes.
- Neural Networks: These are used for complex prediction tasks, capturing non-linear relationships between financial variables.
- Random Forests: An ensemble method that combines multiple decision trees to improve prediction accuracy.
“Machine learning allows financial analysts to process vast amounts of data in real-time, uncovering trends and insights that traditional methods might overlook.”
Example: Predictive Model for Stock Market
Feature | Prediction Method | Outcome |
---|---|---|
Historical Stock Prices | Linear Regression | Price Movement Forecast |
Market Sentiment | Neural Networks | Price Fluctuation Predictions |
Economic Indicators | Decision Trees | Market Volatility Prediction |
Integrating Artificial Intelligence for Automated Trading and Investment Decisions
Artificial Intelligence (AI) is transforming the landscape of financial markets, offering significant improvements in both the speed and accuracy of trading and investment decisions. By leveraging AI, financial institutions and individual investors can make data-driven decisions with minimal human intervention, optimizing the efficiency of their portfolios and strategies.
AI-powered systems in finance are designed to analyze vast amounts of market data, including historical prices, trends, and external factors such as news and social sentiment. These systems use algorithms to predict market movements, automatically execute trades, and adjust strategies in real-time, providing a competitive edge in fast-paced markets.
Key Benefits of AI in Automated Trading
- Real-time Decision Making: AI models can process real-time data, enabling traders to react instantly to market fluctuations.
- Data-Driven Insights: Machine learning algorithms can analyze complex datasets, identifying patterns and trends that may not be immediately apparent to human traders.
- Reduced Human Error: Automated trading systems reduce the risk of emotional decision-making and human error, providing more consistent performance.
- 24/7 Market Monitoring: AI systems are capable of monitoring markets around the clock, ensuring no opportunities are missed during off-hours.
How AI Optimizes Investment Decisions
- Risk Assessment: AI models can calculate risk levels for different investment strategies, providing insights into potential returns versus risks.
- Portfolio Diversification: AI can assist in creating a diversified portfolio by analyzing correlations between assets and minimizing overall risk.
- Predictive Analysis: AI algorithms use past performance and current market conditions to forecast future asset prices, helping investors make informed decisions.
"AI in finance is not just about automating tasks; it's about enhancing decision-making with precision and speed, creating new opportunities for traders and investors alike."
Example: AI-Based Trading Platform
Feature | Benefit |
---|---|
Machine Learning Algorithms | Predict price movements based on historical data |
Real-Time Market Monitoring | Monitor market conditions 24/7, ensuring timely execution of trades |
Automated Trading | Execute trades based on pre-set conditions without manual intervention |
Ensuring Data Privacy and Security with AI in Finance
In the financial sector, data security and privacy are of utmost importance, especially when integrating artificial intelligence (AI) for analysis, decision-making, and automation. AI systems handle vast amounts of sensitive financial data, making it crucial to ensure that this information remains secure and confidential. Financial institutions must implement strong data protection measures to mitigate risks such as data breaches, unauthorized access, and cyber threats, while also complying with regulatory standards like GDPR and CCPA.
The use of AI in finance introduces new complexities in data protection. While AI offers advanced capabilities like predictive analytics and real-time fraud detection, it also raises concerns about how data is collected, processed, and stored. Therefore, financial organizations must adopt robust frameworks and technologies to protect data integrity and maintain customer trust.
Key Measures to Ensure Data Privacy
- Data Encryption: Encrypting sensitive data both in transit and at rest is essential to prevent unauthorized access.
- Anonymization and Pseudonymization: Techniques like anonymizing data can ensure that personal information is not exposed in AI models and analytics.
- Access Control: Strict role-based access control limits who can access sensitive data and AI models, ensuring only authorized personnel can interact with it.
- Data Minimization: Collecting only the essential data needed for AI models reduces the risk of exposure.
Security Measures for AI Models
- Model Integrity Protection: Techniques such as adversarial training help ensure AI models are resistant to manipulation or attacks aimed at altering their behavior.
- Secure AI Frameworks: Using secure and well-tested AI platforms helps reduce vulnerabilities that could be exploited in financial applications.
- Continuous Monitoring: Regular monitoring and auditing of AI systems detect any anomalies or breaches in real-time, ensuring timely intervention.
“Incorporating AI into financial systems is not just about innovation but also about maintaining the highest standards of data security and privacy to safeguard both institutions and customers.”
Compliance with Regulations
Regulation | Description |
---|---|
GDPR | Requires strict data protection measures and transparency for customers in the European Union, ensuring their personal data is secure when used by AI systems. |
CCPA | Ensures consumers' right to privacy and control over their personal data, impacting AI-driven solutions in the U.S. |
PCI DSS | Outlines security standards for handling credit card transactions and sensitive financial data in AI systems. |
Enhancing Client Support with AI-Powered Chatbots in the Financial Sector
AI-driven chatbots are revolutionizing customer service in the finance industry by offering faster and more efficient communication. These intelligent systems can handle a wide range of inquiries and tasks, from answering basic questions to performing complex transactions. By integrating natural language processing (NLP) and machine learning, chatbots can provide personalized responses and learn from each interaction, improving the customer experience over time.
In the context of financial services, AI chatbots are being deployed across various touchpoints, including banking apps, websites, and social media platforms. They help streamline customer support, reduce wait times, and enhance user satisfaction by providing 24/7 assistance. This allows financial institutions to allocate human resources to more critical tasks, improving overall operational efficiency.
Key Benefits of AI Chatbots in Finance
- Improved Customer Engagement: Chatbots can interact with customers in real-time, offering immediate solutions to their queries.
- Cost Efficiency: Automation reduces the need for large customer service teams, lowering operational costs for financial institutions.
- Personalized Services: AI systems can learn from customer interactions, offering tailored recommendations and support.
- Scalability: Chatbots can easily handle an increasing volume of requests, especially during peak times, without compromising service quality.
Use Cases for AI Chatbots in Financial Services
- Account Inquiries: Customers can check balances, recent transactions, and other account details without speaking to a human agent.
- Loan Applications: Chatbots guide users through the application process, answering questions and providing required information instantly.
- Fraud Detection: AI chatbots can alert users about suspicious activities or security threats related to their accounts.
- Personal Finance Advice: Some advanced chatbots offer tailored financial advice based on user data and preferences.
AI-powered chatbots are transforming the customer experience by making banking services more accessible, responsive, and efficient, paving the way for a smarter future in finance.
Challenges in Implementing AI Chatbots in Finance
Challenge | Impact | Solution |
---|---|---|
Data Privacy Concerns | Chatbots collect sensitive customer data, raising privacy issues. | Implement robust encryption and comply with data protection regulations (e.g., GDPR). |
Accuracy of Responses | Inaccurate or unclear responses can lead to dissatisfaction. | Regular training and updates to improve NLP models and response accuracy. |
Integration with Legacy Systems | Older systems may not easily integrate with modern AI solutions. | Adopt flexible, scalable integration solutions that support legacy technologies. |
Real-time Market Monitoring and Insights with AI Solutions
Artificial Intelligence (AI) tools have revolutionized the way financial institutions track and analyze market trends. These tools leverage real-time data to provide accurate, timely insights that are crucial for decision-making. Financial professionals use AI systems to monitor market fluctuations, analyze large datasets, and generate predictive models that can guide trading strategies. By using advanced algorithms, AI platforms can analyze market conditions almost instantaneously, allowing firms to stay ahead of competitors and make informed choices.
The ability of AI to process and interpret massive amounts of data in real-time helps identify patterns that human analysts might miss. This has become essential in a market where speed and precision are critical. AI-powered tools provide not only insights into market movements but also forecast future trends, enabling companies to react promptly to potential risks or opportunities.
Key Benefits of AI for Market Monitoring
- Instant Analysis: AI platforms can process data from multiple sources in seconds, offering real-time insights into market conditions.
- Predictive Capabilities: Through machine learning models, AI tools predict market trends, providing a competitive edge in anticipating market shifts.
- Data Integration: AI systems aggregate data from diverse financial instruments, making it easier to gain a holistic view of the market.
- Risk Management: AI tools help detect emerging risks and alert traders to potential disruptions before they occur.
How AI Tools Enhance Market Insights
- Real-time Alerts: AI-driven platforms send automatic notifications based on market volatility, asset price movements, or news events.
- Sentiment Analysis: Natural language processing (NLP) techniques are used to analyze financial news, social media, and reports to gauge market sentiment.
- Pattern Recognition: Machine learning models identify recurring market patterns, helping to make sense of complex data sets.
AI tools offer not just data analysis but also actionable recommendations, empowering financial professionals to execute strategies with confidence.
AI Platform Features for Real-time Market Monitoring
Feature | Description |
---|---|
Real-time Data Streams | Continuous updates from global markets, ensuring that traders have the latest information at their fingertips. |
Machine Learning Models | Predict future market movements based on historical data and current trends. |
Automated Insights | AI systems automatically analyze data and provide clear insights, reducing the need for manual intervention. |
Custom Alerts | Allow users to set up personalized notifications based on specific market criteria. |