Ai Solutions for Higher Education
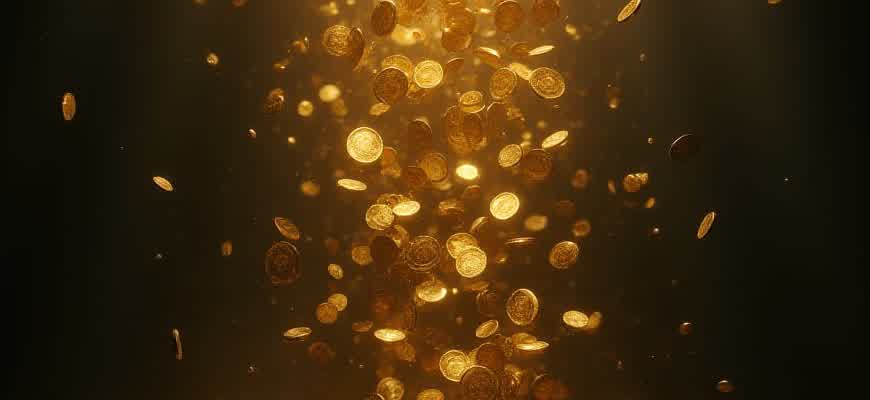
Artificial intelligence is becoming a driving force in transforming higher education systems. Universities and academic institutions are increasingly leveraging AI technologies to improve teaching, learning, and administrative processes. These solutions provide enhanced efficiency and personalized experiences for both students and faculty members.
Key AI Solutions for Academic Institutions:
- Adaptive Learning Platforms: AI-powered systems that adjust to the learning pace and style of individual students, offering tailored educational content.
- Automated Grading Systems: AI-driven algorithms that can assess assignments and exams, providing instant feedback to students.
- Chatbots for Student Support: Virtual assistants available 24/7 to answer questions and assist students with common academic queries.
"AI technologies in higher education have the potential to revolutionize traditional methods of teaching, creating more personalized, accessible, and efficient learning environments."
AI's Impact on Administrative Efficiency:
- Enrollment and Admissions: AI algorithms can assist in streamlining the application process, predicting student success, and improving recruitment strategies.
- Course Scheduling: AI systems help institutions optimize class schedules based on student demand and faculty availability.
- Data Analytics for Performance Monitoring: AI tools analyze student data to identify trends, allowing for more informed decision-making and interventions.
AI-Powered Tools in Higher Education: A Comparison
Tool | Function | Impact |
---|---|---|
AI Chatbots | Student interaction and support | Improved student engagement and support availability |
Adaptive Learning | Personalized learning experiences | Enhanced student success rates and learning efficiency |
Automated Grading | Grading assignments and assessments | Faster feedback and reduced faculty workload |
Artificial Intelligence in Higher Education: Transforming Learning and Administration
Artificial intelligence (AI) is revolutionizing the higher education sector by enhancing both teaching and administrative processes. Through intelligent systems, institutions are improving student engagement, optimizing resource management, and providing personalized learning experiences. AI tools are also increasingly used to streamline administrative tasks, allowing universities to allocate resources more efficiently and enhance overall operational effectiveness.
One key area where AI is making a significant impact is in the realm of student learning. By leveraging data analytics and adaptive learning algorithms, AI systems can provide tailored educational content that meets individual student needs. This not only improves learning outcomes but also enables institutions to support diverse student populations more effectively.
AI-Powered Solutions in Education
- Personalized Learning Paths: AI-driven platforms can analyze students’ performance and learning styles, delivering customized content and feedback.
- Automated Administrative Tasks: Routine tasks like scheduling, grading, and record-keeping can be automated, reducing administrative overhead and allowing staff to focus on more critical tasks.
- Predictive Analytics for Student Success: AI models analyze student data to predict academic performance and identify at-risk students early, enabling timely intervention.
- Virtual Assistants: AI-powered chatbots and assistants help students with course selection, administrative inquiries, and technical support, providing immediate assistance around the clock.
Examples of AI Tools in Higher Education
- Learning Management Systems (LMS): Platforms like Blackboard and Canvas incorporate AI to track student progress and offer tailored recommendations.
- AI Tutors: Virtual tutors like Squirrel AI offer personalized help, simulating one-on-one tutoring experiences.
- AI in Admissions: AI tools assist universities in streamlining the admissions process by analyzing application data and predicting student success.
“AI in higher education is not just about enhancing efficiency; it's about providing each student with an individualized educational experience that can lead to better outcomes and greater engagement.”
Benefits and Challenges of AI Integration
Benefits | Challenges |
---|---|
Improved student engagement and retention through personalized learning experiences. | High initial investment in AI technology and training. |
Enhanced administrative efficiency with automation of routine tasks. | Privacy and data security concerns with AI-driven systems. |
Better insights into student performance through predictive analytics. | Resistance to change from faculty and staff. |
Enhancing Student Engagement with Personalized Learning Paths
Artificial Intelligence (AI) is revolutionizing the way educational institutions cater to the needs of students. By leveraging AI-driven solutions, universities and colleges can create individualized learning experiences that boost student motivation and retention. Personalized learning paths, tailored to each student's unique strengths and weaknesses, offer a more engaging educational experience compared to traditional, one-size-fits-all approaches.
AI can analyze student performance data to create dynamic learning paths that evolve in response to progress, challenges, and preferences. This customization not only helps students focus on areas where they need the most improvement but also promotes a sense of ownership and control over their learning journey. The result is enhanced engagement and a deeper connection to the material being taught.
Key Benefits of Personalized Learning Paths
- Individualized Progression: AI systems assess students' abilities and provide content that matches their pace, ensuring that no one is left behind.
- Increased Motivation: When students see continuous progress in areas that are relevant to them, they remain more motivated to engage with the coursework.
- Focused Attention: By reducing unnecessary content and honing in on key concepts, AI ensures that students spend more time on what matters most for their growth.
How AI Personalizes Learning
- Adaptive Assessments: AI-driven tools create personalized quizzes and assignments that adjust in difficulty based on student performance.
- Real-Time Feedback: Through constant evaluation, AI offers immediate, actionable feedback, enabling students to adjust their learning approach promptly.
- Learning Style Recognition: AI algorithms can identify preferred learning styles (visual, auditory, kinesthetic) and recommend resources that align with those preferences.
"AI-driven personalization not only empowers students to take control of their learning but also ensures that instructors can focus their efforts on guiding learners through the most critical points of their educational journey."
Example of AI-Powered Personalization in Practice
Student | Initial Skill Level | Recommended Learning Path |
---|---|---|
John | Beginner | Introductory courses with interactive exercises and gradual progression to advanced topics. |
Alice | Intermediate | Focused practice on complex problems, with visual aids and peer collaboration options. |
Mike | Advanced | Self-paced learning with in-depth resources and advanced project-based challenges. |
Automating University Administrative Processes with AI
Universities deal with a wide range of administrative tasks, from student admissions to scheduling and managing resources. Traditional methods of handling these tasks are time-consuming and prone to human error, leading to inefficiency and frustration for both staff and students. By incorporating AI-powered solutions, institutions can streamline many of these administrative processes, freeing up valuable time for more strategic and student-focused activities.
AI technologies are capable of automating repetitive tasks, improving accuracy, and enhancing the overall administrative experience. From chatbots that answer common queries to AI systems that manage resource allocation, these solutions not only reduce workload but also improve the speed and consistency of services. Below are some key areas where AI is transforming university administration:
Key Areas for AI Integration in University Administration
- Student Admissions: AI can automate the processing of applications, grading, and initial interviews, reducing the workload on admissions teams.
- Scheduling: AI can optimize timetables for classes, faculty, and resources, minimizing conflicts and improving utilization.
- Data Management: AI helps organize and analyze student records, grades, and performance, ensuring compliance with regulations and improving decision-making.
Benefits of AI in Administrative Automation
By automating routine tasks, AI helps administrative staff focus on higher-level duties, improving efficiency and reducing errors.
- Improved Efficiency: Automating tasks such as attendance tracking or document processing cuts down on manual effort.
- Cost Reduction: Less reliance on human labor for repetitive tasks lowers operational costs.
- Enhanced Student Experience: Quicker responses to queries and streamlined services result in higher satisfaction levels.
Example AI Applications in University Administration
AI Tool | Application |
---|---|
Chatbots | Answer student queries on admissions, exams, deadlines, and more. |
AI Scheduling Systems | Automatically allocate classrooms, professors, and other resources. |
Document Recognition | Scan and categorize student records, enabling quick access and review. |
AI-Powered Virtual Assistants for Continuous Student Engagement and Query Resolution
As educational institutions increasingly adopt AI-driven technologies, virtual assistants have emerged as essential tools for improving student support services. These AI systems can offer 24/7 assistance, providing immediate responses to students' questions, thus reducing wait times and enhancing overall satisfaction. Unlike traditional support channels, which often rely on office hours or scheduled appointments, AI chatbots are always available, offering timely and efficient solutions across various platforms. Whether answering frequently asked questions or providing real-time updates, these intelligent systems can significantly improve the student experience.
AI-powered virtual assistants are designed to interact with students in a conversational manner, allowing them to easily find the information they need without the need for human intervention. They can handle a wide range of inquiries, from administrative matters like registration processes to academic concerns such as course details and deadlines. By leveraging natural language processing (NLP), these AI tools understand and respond to student inquiries in a manner that feels natural and intuitive.
Advantages of AI Chatbots in Higher Education
- 24/7 Availability: Virtual assistants are always accessible, offering continuous support beyond traditional office hours.
- Instant Response: Students receive quick answers, minimizing delays in getting information.
- Personalized Experience: AI can tailor responses based on a student's profile and preferences.
- Scalability: AI chatbots can handle a large volume of queries simultaneously without a drop in performance.
Common Uses of AI Chatbots in Educational Institutions
- Enrollment and Registration Assistance: Guiding students through the steps of course selection and registration.
- Academic Advising: Offering advice on course prerequisites, scheduling, and degree requirements.
- Technical Support: Troubleshooting common technical issues with learning platforms or institutional websites.
- Event and Deadline Reminders: Notifying students of upcoming deadlines, exams, or important institutional events.
"AI chatbots provide immediate assistance, removing the barriers of time and geography. They enhance the educational experience by ensuring students always have access to the information they need."
Comparison of Traditional Support vs. AI-Powered Chatbots
Aspect | Traditional Support | AI-Powered Chatbots |
---|---|---|
Availability | Limited to office hours | 24/7 |
Response Time | Variable, may take time to connect with an agent | Instant responses |
Handling Multiple Queries | Limited to a few simultaneous queries | Handles large volumes of queries simultaneously |
Personalization | Standard responses | Customized based on student profile |
Data-Driven Approaches to Enhance Student Retention in Universities
Leveraging advanced data analytics can significantly improve retention rates in higher education institutions. By closely monitoring student behaviors and performance, universities can identify early signs of disengagement and academic challenges. These insights enable targeted interventions that address specific issues before they escalate, ultimately leading to higher student satisfaction and success rates.
Institutions that harness the power of data can proactively develop personalized support systems, optimize resource allocation, and tailor student engagement strategies. As a result, they can enhance retention by addressing the individual needs of students, providing a more supportive learning environment, and fostering long-term academic commitment.
Identifying Key Factors Affecting Retention Rates
Several data points contribute to understanding retention trends within an institution. Some of the key indicators include:
- Academic performance and grades
- Engagement with campus activities and resources
- Social integration and peer connections
- Attendance patterns and class participation
- Financial aid status and student debt levels
By analyzing these factors, universities can pinpoint students at risk of dropping out and intervene with personalized strategies tailored to their unique circumstances.
Actionable Strategies for Improving Retention
Data-driven insights can lead to the development of targeted actions to improve retention. A few effective strategies include:
- Implementing predictive models to identify students who may need additional academic support.
- Personalizing communication and academic advisement based on data from previous interactions.
- Offering financial planning resources to students at risk due to financial pressures.
- Encouraging peer mentoring and participation in campus activities to enhance social integration.
"Data is a powerful tool in identifying at-risk students early and developing tailored interventions that can significantly improve retention outcomes."
Utilizing Data for Continuous Improvement
By continuously collecting and analyzing data, universities can adapt their retention strategies in real-time. A data-driven feedback loop ensures that the strategies evolve in response to changing student needs and emerging trends. Regular assessments of retention rates and the effectiveness of interventions provide valuable insights for further refinement.
Factor | Impact on Retention |
---|---|
Academic Performance | Strong correlation with continued enrollment; poor grades often signal risk of dropout |
Campus Engagement | Active participation in clubs, events, and peer groups increases sense of belonging, reducing dropout risk |
Financial Stability | Financial stress can lead to high dropout rates, requiring intervention from financial aid offices |
AI in Course Design: Tailoring Learning Experiences for Diverse Student Populations
Artificial intelligence is reshaping the way academic curricula are structured, enabling educators to create personalized learning paths for students. This technology allows for the dynamic adjustment of course materials and assessments, ensuring that each student’s individual needs are met. Whether it’s through adapting the difficulty level of assignments or offering additional resources based on student performance, AI provides a level of customization that traditional methods cannot achieve. This results in a more inclusive environment where students with varying learning styles and paces can thrive.
One of the key advantages of using AI in curriculum development is its ability to analyze vast amounts of data about student interactions, engagement, and outcomes. By processing this information, AI can suggest real-time modifications to course content, helping instructors better understand which concepts students are struggling with and which are already mastered. These insights help to fine-tune learning materials, creating a more effective and responsive teaching approach.
Benefits of AI-Driven Curriculum Customization
- Personalized Learning Paths: AI can identify individual student strengths and weaknesses, enabling the creation of tailored educational experiences.
- Adaptive Learning: Courses can automatically adjust in difficulty based on student performance, ensuring that all learners are appropriately challenged.
- Real-Time Feedback: AI provides instant feedback on assignments and activities, allowing students to learn from their mistakes and improve continuously.
- Inclusive Learning: With AI, it is possible to cater to students with diverse learning styles, including those with disabilities, by offering accessible learning options.
Key Elements of AI-Enhanced Curriculum Design
- Data Collection and Analysis: AI systems track student performance and interaction with the course content to identify trends and needs.
- Content Adaptation: AI can modify the complexity of the material or suggest additional resources such as videos, articles, and exercises.
- Progress Monitoring: AI continuously monitors how students are progressing, providing instructors with insights for further curriculum adjustments.
"AI-driven curriculum design ensures that every student receives a unique learning experience, not only improving engagement but also enhancing academic outcomes."
Example of AI-Based Customization in Higher Education
Feature | Traditional Approach | AI-Enhanced Approach |
---|---|---|
Assignment Difficulty | Standard difficulty for all students | Adjusts based on individual performance |
Learning Resources | Predefined set of resources | Personalized content recommendations based on learning style |
Assessment Feedback | Delayed feedback after grading | Instant, actionable feedback after each activity |
Leveraging Machine Learning to Forecast Academic Outcomes
In higher education, predicting student success is a critical task that can significantly enhance academic support systems. By utilizing machine learning models, universities can analyze vast amounts of student data to identify patterns that may indicate future performance. This approach allows institutions to implement targeted interventions, improving student retention rates and overall academic achievement.
Machine learning algorithms can be trained on various student data points such as grades, attendance, participation, and even socio-economic factors. These predictive models provide instructors and administrators with actionable insights, enabling them to offer personalized academic assistance and resources based on individual student needs.
Key Techniques in Predicting Student Performance
- Regression Analysis: Helps forecast continuous outcomes, such as final grades, by analyzing historical academic data.
- Classification Algorithms: Used to categorize students into groups (e.g., at-risk, average, or high-performing) based on multiple features.
- Clustering: Identifies patterns among students who share similar characteristics, which can assist in creating personalized learning strategies.
Important Considerations for Implementing Machine Learning
Data quality and accuracy are crucial when developing machine learning models. Inaccurate or incomplete data can lead to flawed predictions and ineffective interventions.
Moreover, it is essential to continually update the models with fresh data to maintain accuracy over time. The results from these models can then be integrated into student management systems, enabling automated alerts for instructors about students who may need additional support.
Example of Predictive Model Performance
Algorithm | Accuracy | Application |
---|---|---|
Decision Trees | 85% | Identifying at-risk students based on their academic history |
Support Vector Machines | 88% | Classifying students by performance groups (low, medium, high) |
Neural Networks | 90% | Predicting long-term student success based on a range of factors |
Enhancing Collaborative Research with AI-Driven Solutions
AI tools are increasingly transforming the way academic researchers collaborate. These advanced systems enable seamless data sharing, real-time communication, and intelligent insights that foster more effective teamwork across institutions. Researchers, regardless of their geographic location, can now engage in collaborative projects with greater efficiency, powered by AI technologies that facilitate knowledge exchange and streamline the research process.
By leveraging AI-driven platforms, universities and research institutes can break down traditional barriers in academic collaboration. These tools help manage vast datasets, analyze trends, and suggest relevant research areas based on real-time data processing. As a result, collaboration becomes more dynamic and data-driven, allowing teams to focus on innovative problem-solving rather than administrative challenges.
Key Benefits of AI for Research Collaboration
- Faster Data Analysis: AI-powered tools can process and analyze large datasets in a fraction of the time it would take manually, offering researchers deeper insights quickly.
- Enhanced Communication: AI-based platforms enable real-time discussions and collaboration among research teams, regardless of location, fostering better teamwork.
- Improved Research Matching: AI can match researchers with similar or complementary interests, facilitating more targeted and effective partnerships.
AI Tools for Collaborative Research
- AI-Powered Literature Review Platforms: These systems can automatically scan academic databases and suggest relevant papers, saving time and ensuring comprehensive research reviews.
- Collaborative Project Management Tools: AI tools assist in task management, progress tracking, and resource allocation, optimizing workflows among distributed teams.
- AI-Enhanced Data Sharing Platforms: These platforms use AI to organize and categorize shared data, making it more accessible and useful for collaborative efforts.
Example of AI-Assisted Research Collaboration
AI Tool | Function | Research Outcome |
---|---|---|
AI Literature Review System | Automatically scans and filters relevant papers | Time-saving and more comprehensive literature review |
Collaborative Research Platforms | Facilitates communication and document sharing | Improved teamwork and faster decision-making |
"AI tools are transforming the landscape of research collaboration, enabling more efficient, data-driven teamwork that leads to faster innovation."