Ai and Machine Learning Companies
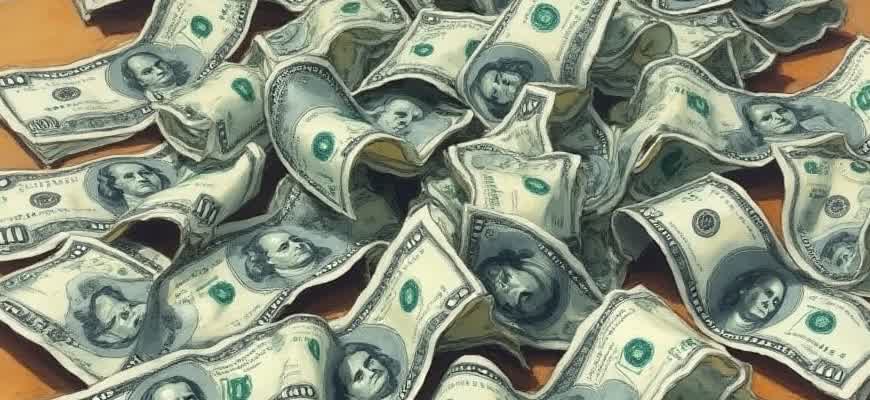
The rapid evolution of artificial intelligence and machine learning technologies has fostered the emergence of numerous companies at the forefront of innovation in this field. These companies span various industries, from healthcare to finance, offering solutions that leverage advanced algorithms, neural networks, and data-driven insights to optimize operations and improve decision-making processes.
Key players in this space include:
- Google DeepMind - Focuses on deep learning and neural networks to create cutting-edge AI models.
- OpenAI - Known for developing large language models and reinforcement learning systems.
- IBM Watson - Provides AI-powered solutions for businesses, including natural language processing and data analytics.
Prominent sectors impacted by AI and machine learning technologies:
- Healthcare - AI is used for predictive diagnostics, drug discovery, and personalized medicine.
- Finance - Machine learning helps in fraud detection, algorithmic trading, and risk management.
- Retail - AI enhances customer experience through recommendation systems and inventory management.
"AI and machine learning are not just technologies; they are transforming industries and shaping the future of business operations."
Companies leading the AI revolution:
Company | Primary Focus | Notable Contribution |
---|---|---|
Google DeepMind | Deep learning, Reinforcement learning | AlphaGo, AI-driven research |
OpenAI | Natural Language Processing, Reinforcement learning | GPT-3, Chatbots |
IBM Watson | AI for business, Natural Language Understanding | AI solutions for healthcare and enterprises |
AI and Machine Learning Companies: A Comprehensive Guide
Artificial Intelligence (AI) and Machine Learning (ML) have rapidly evolved, becoming integral parts of various industries. These technologies enable companies to automate processes, enhance decision-making, and drive innovation. From startups to established giants, AI and ML companies are transforming sectors like healthcare, finance, and retail.
In this guide, we will explore the key players in the AI and ML industry, outlining their services and contributions. Understanding the role of these companies is crucial for anyone looking to stay ahead in the technology landscape.
Top AI and ML Companies
- Google DeepMind: Known for groundbreaking advancements in reinforcement learning, DeepMind focuses on developing AI that mimics human problem-solving skills.
- OpenAI: A leader in natural language processing, OpenAI created the GPT models, which power various applications, from chatbots to content creation tools.
- IBM Watson: IBM Watson offers AI solutions that help businesses improve efficiency, analyze data, and enhance customer experiences through cognitive computing.
- Amazon Web Services (AWS) AI: AWS provides a comprehensive suite of machine learning services, including SageMaker, which helps businesses build, train, and deploy models at scale.
- Microsoft Azure AI: Azure AI is an integrated cloud platform that offers machine learning tools, AI services, and data analytics to help businesses leverage AI technologies effectively.
Key Areas of AI and ML Applications
- Healthcare: AI is used in diagnostics, drug discovery, and personalized treatment plans.
- Finance: AI-driven algorithms help in fraud detection, investment analysis, and credit scoring.
- Retail: AI enhances customer experience through recommendation systems and demand forecasting.
- Automotive: Machine learning optimizes self-driving technologies and predictive maintenance systems.
AI and ML companies are not just tech providers but also innovators, helping businesses adapt to new technological landscapes and improve their competitive edge.
Comparison Table of Key Companies
Company | Specialization | Notable Product/Service |
---|---|---|
Google DeepMind | Reinforcement Learning | AlphaGo |
OpenAI | Natural Language Processing | GPT-3, DALL·E |
IBM Watson | Cognitive Computing | Watson AI Services |
AWS AI | Cloud-Based ML Solutions | SageMaker |
Microsoft Azure AI | Cloud and AI Integration | Azure Machine Learning |
How AI and Machine Learning Companies Transform Business Processes
Artificial Intelligence (AI) and Machine Learning (ML) companies have fundamentally altered how businesses approach decision-making, optimization, and automation. By leveraging data-driven models and predictive analytics, these companies enable organizations to uncover insights, streamline workflows, and improve operational efficiency. As a result, traditional business methods are being replaced with innovative solutions powered by intelligent algorithms and automated systems.
From enhancing customer service to refining supply chain management, the applications of AI and ML are far-reaching. Businesses are now able to process vast amounts of data in real-time, offering personalized services and making proactive decisions that were once impossible. AI and ML technologies empower businesses to anticipate market trends, adjust to customer preferences, and increase overall productivity.
Key Benefits of AI and Machine Learning in Business
- Enhanced Decision Making: Machine learning models analyze historical data to forecast future trends, enabling businesses to make informed, data-driven decisions.
- Automation of Repetitive Tasks: AI solutions help automate routine tasks such as data entry, processing, and customer support, freeing up valuable time for employees to focus on higher-value work.
- Improved Customer Experience: AI-driven chatbots and recommendation systems personalize customer interactions, providing faster and more accurate responses.
Key Industries Benefiting from AI and Machine Learning
- Healthcare: AI is revolutionizing diagnostics, predictive medicine, and personalized treatment plans, improving patient outcomes.
- Retail: Machine learning algorithms optimize inventory management, demand forecasting, and customer personalization, driving sales and enhancing customer satisfaction.
- Finance: AI enhances fraud detection, risk management, and automated trading, helping financial institutions stay ahead in a competitive market.
AI and Machine Learning are not just tools, they are key drivers of transformation that allow businesses to operate smarter, faster, and more cost-effectively.
Impact on Business Efficiency
Process | AI Impact | Result |
---|---|---|
Supply Chain Management | AI algorithms optimize routes, manage inventory, and predict disruptions. | Reduced operational costs and improved delivery times. |
Customer Service | AI chatbots provide 24/7 support and handle common inquiries. | Improved customer satisfaction and reduced support costs. |
Marketing | Machine learning models analyze consumer behavior to tailor marketing strategies. | Higher conversion rates and more effective campaigns. |
Leading Technologies in AI and Machine Learning Companies
The rapid evolution of artificial intelligence (AI) and machine learning (ML) has been significantly driven by a few key technologies. These technologies are central to developing intelligent systems, improving accuracy, and automating complex tasks across various industries. Many AI companies rely on specific tools, frameworks, and platforms to build solutions that power advancements in areas like automation, robotics, and predictive analytics.
This article explores some of the top technologies that are commonly used by leading AI and machine learning companies today. From cloud-based services to advanced algorithms, these technologies are the backbone of AI-driven innovation.
Core Technologies Driving AI and ML Innovation
- Deep Learning Frameworks: Popular frameworks such as TensorFlow, PyTorch, and Keras are fundamental to the development of neural networks. These frameworks are widely used to design and implement deep learning models that mimic human brain functions.
- Natural Language Processing (NLP): Tools like GPT, BERT, and spaCy enable machines to understand, interpret, and generate human language. NLP plays a vital role in chatbots, translation services, and voice recognition systems.
- Cloud Computing Platforms: Cloud services such as AWS, Google Cloud, and Microsoft Azure provide scalable infrastructure for AI and ML applications. These platforms offer the necessary computational power to run large-scale models and process big data.
Key Algorithms and Techniques
- Reinforcement Learning: Used for training agents through reward-based systems, this method is popular in robotics and autonomous systems.
- Supervised Learning: A technique where models are trained on labeled datasets to predict outcomes, widely used for classification and regression tasks.
- Unsupervised Learning: Algorithms such as clustering and association allow machines to find patterns in data without explicit labels, used in market segmentation and anomaly detection.
Important Information to Note
Machine learning companies are constantly evolving, and the integration of new technologies such as quantum computing and edge AI is likely to redefine the capabilities of AI systems in the near future.
Comparison of Popular AI Tools
Tool | Primary Use | Key Feature |
---|---|---|
TensorFlow | Deep Learning | Open-source library for training models across multiple platforms |
PyTorch | Deep Learning | Dynamic computation graphs, better for research and prototyping |
AWS Sagemaker | Cloud-based Machine Learning | Fully managed service to build, train, and deploy ML models |
Choosing the Right AI Company for Your Custom Machine Learning Needs
When selecting an AI company to develop custom machine learning solutions, it's crucial to consider a number of factors that can ensure a successful collaboration. A deep understanding of your business needs, the company’s technical expertise, and the flexibility of their solutions will play a key role in achieving the desired outcomes. Whether you're seeking predictive models, automation systems, or recommendation engines, the right AI partner will tailor their approach to your unique requirements.
Additionally, it's important to look for companies that have proven experience in building scalable and robust machine learning models. The right company will guide you through the entire process, from identifying the problem to implementing the solution, ensuring smooth integration with existing systems. Here are the key aspects to evaluate when choosing a machine learning partner.
Factors to Consider When Selecting an AI Company
- Expertise and Experience: The company should have a strong portfolio in machine learning projects that closely resemble your own needs. Ask for case studies or examples that demonstrate their capabilities.
- Technology Stack: Ensure the company uses advanced tools and platforms that are suitable for your project's complexity. Machine learning frameworks like TensorFlow, PyTorch, or custom solutions should align with your technical requirements.
- Customization and Flexibility: Machine learning solutions often need to be tailored. Choose a company that listens to your specific needs and can provide flexible solutions, not off-the-shelf products.
- Communication and Collaboration: A good AI company should maintain clear and ongoing communication. Their ability to explain technical concepts in a straightforward way is essential for seamless cooperation.
Evaluation Criteria
- Technical Competence: Ensure that the AI company has deep technical knowledge in both machine learning algorithms and the specific domain you are working in.
- Cost-Effectiveness: Assess whether the company’s pricing aligns with your budget without compromising the quality of service.
- Post-Implementation Support: Machine learning models often require fine-tuning and ongoing monitoring. Ensure the company provides robust post-deployment support.
"Choosing an AI partner is not just about finding a service provider, but a collaborative team that understands your vision and can help bring it to life through data and technology."
Comparison Table of Key AI Companies
Company | Expertise | Customization | Support |
---|---|---|---|
Company A | Finance, Retail | High | 24/7 Support |
Company B | Healthcare, Manufacturing | Medium | Limited |
Company C | Automotive, Logistics | Low | Standard Support |
Integrating AI and Machine Learning into Existing Business Models
Integrating artificial intelligence (AI) and machine learning (ML) into existing business structures requires a strategic approach. Businesses must ensure that the introduction of these technologies enhances operational efficiency, customer experience, and overall productivity. With the right planning, AI can be seamlessly incorporated into various facets of the organization, including marketing, sales, customer support, and logistics.
To ensure successful integration, companies should first identify areas that can benefit from AI and ML. This involves evaluating current business processes and determining where automation, data analysis, or predictive models can add the most value. Below is a guide on how to effectively incorporate AI and ML into your operations.
Key Steps for Successful AI and ML Integration
- Identify Business Needs: Determine which areas within the business can benefit from AI-driven improvements. This could include customer service automation, sales forecasting, or predictive maintenance.
- Data Collection and Management: Ensure that quality data is available to train machine learning models. This may require updating data storage systems or adopting new data collection tools.
- Choose the Right AI Tools: Select AI and ML tools that fit your business model. This might involve custom development or using pre-built solutions.
- Train and Test Models: Build and test machine learning models on historical data before deployment to ensure accuracy and reliability.
- Integrate AI into Existing Systems: AI models should be integrated with current business software and workflows to maximize efficiency.
Important: Ensure that the AI solution is scalable. As your business grows, the AI system should be able to accommodate increasing data volumes and evolving business requirements.
Example Integration Process
Phase | Action | Outcome |
---|---|---|
Planning | Identify the problem to be solved and select relevant AI tools. | Clear objectives for AI implementation. |
Data Collection | Gather and clean relevant business data. | Data ready for analysis and model training. |
Model Training | Develop machine learning models using collected data. | AI models capable of making predictions or automating processes. |
Integration | Incorporate AI solutions into existing systems. | Seamless use of AI tools within business operations. |
Understanding the Cost Structure of AI and Machine Learning Services
When evaluating AI and machine learning services, it is crucial to understand the different components that influence the cost structure. These services can range from simple algorithm development to advanced predictive modeling, each with its own pricing model. A deep understanding of how these services are priced helps businesses make informed decisions about which solutions align with their budgets and objectives.
The cost structure can vary depending on factors such as the scope of the project, the complexity of the algorithms, and the type of service required. In general, AI and ML providers charge based on several key elements, each contributing to the overall cost of the service.
Key Factors Influencing AI and ML Service Pricing
- Project Scope: Larger and more complex projects often incur higher costs due to the increased resources and time required.
- Data Requirements: The amount and quality of data needed for training models significantly impacts pricing. More data requires better infrastructure and additional processing time.
- Algorithm Complexity: Advanced algorithms demand more computational power and specialized knowledge, which can lead to higher costs.
- Custom Development: Tailored AI solutions are generally more expensive than off-the-shelf products due to their unique nature.
Types of Pricing Models
- Hourly Rates: This model is commonly used for consulting or smaller-scale projects, where the client is charged based on the amount of time spent on development or research.
- Fixed Pricing: A set price for a specific project or service. This model is common for well-defined projects with clear requirements.
- Subscription Model: Ongoing services or software provided on a subscription basis, often including updates and continuous support.
Cost Breakdown for AI and ML Services
Component | Cost Range |
---|---|
Data Collection and Preparation | $10,000 - $100,000+ |
Model Development | $50,000 - $500,000+ |
Deployment and Maintenance | $20,000 - $200,000+ |
Consulting/Expertise | $100 - $500 per hour |
The pricing structure for AI and machine learning services can be highly variable, depending on project complexity and the level of expertise required. It is important for businesses to fully understand these factors when budgeting for such services.
Case Studies: Successful Applications of AI in Various Sectors
Artificial Intelligence has made significant strides in transforming various industries by offering innovative solutions to complex challenges. From enhancing operational efficiency to creating personalized customer experiences, AI technologies have proven their worth across diverse fields. Here are some examples where AI has been successfully applied, driving substantial improvements in performance and growth.
In the healthcare sector, AI-powered tools have revolutionized diagnosis, treatment planning, and patient care. In retail, companies have integrated AI to optimize supply chains, personalize marketing efforts, and streamline customer service. These applications illustrate how AI can improve decision-making, reduce costs, and increase profitability across industries.
Healthcare Industry
AI has become an invaluable asset in healthcare by assisting doctors in diagnosing diseases more accurately and speeding up the drug discovery process.
- Early Diagnosis: AI algorithms analyze medical imaging data to identify early signs of diseases like cancer, often more accurately than human doctors.
- Personalized Medicine: Machine learning models help tailor treatments based on individual genetic profiles, optimizing therapeutic outcomes.
- Drug Discovery: AI systems analyze vast datasets to identify potential drug candidates, significantly reducing the time and cost of bringing new drugs to market.
AI-driven diagnostics have reduced diagnostic errors in radiology by up to 40%, saving lives and improving overall treatment efficiency.
Retail Industry
In retail, AI is reshaping customer experience, inventory management, and sales forecasting. By processing vast amounts of data, AI solutions help businesses become more agile and customer-centric.
- Inventory Management: Machine learning models predict demand fluctuations, ensuring optimal stock levels and reducing overstocking.
- Customer Insights: AI analyzes purchase behaviors to offer personalized recommendations, improving sales and customer satisfaction.
- Chatbots: AI-powered chatbots provide round-the-clock customer support, enhancing the shopping experience and reducing operational costs.
Application | Impact |
---|---|
Personalized Recommendations | Increased sales conversion rates by 25% |
AI Chatbots | Reduced customer service costs by 30% |
Demand Forecasting | Reduced stockouts by 15% |
AI and Machine Learning Data Security: What You Need to Know
As artificial intelligence (AI) and machine learning (ML) continue to evolve, so do the risks associated with data security. Organizations harnessing these technologies must understand the vulnerabilities tied to the vast amounts of data they process. With the increasing reliance on algorithms, securing sensitive information and preventing data breaches becomes a critical concern.
In this environment, AI and ML are not only powerful tools for automating tasks but also introduce new challenges in ensuring that data remains protected from malicious actors. Whether it’s preventing unauthorized access or ensuring compliance with data privacy laws, businesses need to develop robust security frameworks that address these emerging risks.
Key Risks in AI and Machine Learning Data Security
- Data Exposure: Sensitive data can be exposed if not properly encrypted or anonymized, leaving it vulnerable to cyber-attacks.
- Model Poisoning: Attackers may intentionally inject harmful data into AI/ML models, undermining their accuracy and reliability.
- Adversarial Attacks: AI/ML systems can be tricked into making incorrect decisions through subtle changes in input data, potentially leading to incorrect conclusions or actions.
- Data Integrity: Ensuring that data used for training models is accurate and untainted is vital to prevent misleading results that could damage the system's effectiveness.
"Data security in AI/ML systems is not just about protecting the data itself but also about maintaining the integrity and trustworthiness of the algorithms that process it."
Best Practices for Securing AI and Machine Learning Data
- Implement Encryption: Always encrypt sensitive data both at rest and in transit to ensure unauthorized parties cannot access it.
- Data Anonymization: Use techniques such as data anonymization to protect the identities of individuals in datasets used for training AI models.
- Regular Audits and Monitoring: Conduct regular audits to monitor potential vulnerabilities and ensure that all systems are up-to-date with the latest security protocols.
- Model Testing and Validation: Continually test models for robustness against adversarial inputs and implement validation processes to identify weaknesses early.
Data Security Tools for AI and Machine Learning
Tool | Function |
---|---|
Homomorphic Encryption | Enables computations on encrypted data without decrypting it, maintaining privacy during processing. |
Federated Learning | Allows machine learning models to be trained across decentralized devices without sharing raw data, improving security and privacy. |
Adversarial Training | Incorporates adversarial examples into training data to help models learn to resist potential attacks. |