Deep Learning in Education Pdf
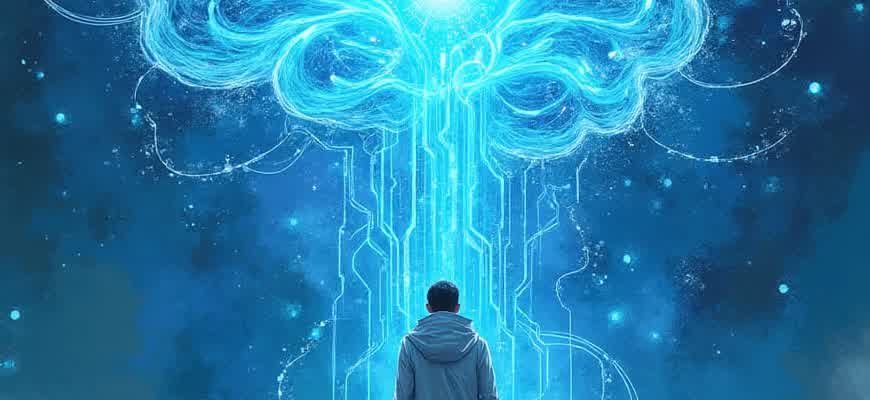
Deep learning, a subset of artificial intelligence (AI), has emerged as a transformative force in the educational sector. By leveraging neural networks, educational systems can now personalize learning experiences, automate administrative tasks, and enhance student performance tracking.
Key Areas of Impact:
- Personalized Learning: Tailoring content and resources to individual learning styles and abilities.
- Automated Grading: Utilizing AI to assess assignments and exams efficiently and accurately.
- Student Support Systems: Creating intelligent tutoring systems that offer real-time assistance.
"The integration of deep learning into education enables a more adaptive and scalable learning environment."
Challenges and Considerations:
- Data Privacy: Protecting student information while utilizing AI-driven tools.
- Accessibility: Ensuring that deep learning-based tools are available to all students, regardless of socioeconomic status.
- Teacher Training: Educators must be equipped with the necessary skills to effectively use AI technologies.
Technologies Used in Deep Learning for Education:
Technology | Description |
---|---|
Natural Language Processing (NLP) | Enables automated interaction with students through chatbots and virtual assistants. |
Computer Vision | Allows for the analysis of visual data, such as handwriting or student engagement during video lessons. |
Reinforcement Learning | Helps in the development of systems that adapt and learn from student feedback to improve teaching strategies. |
Deep Learning in Education: Practical Applications and Insights
Deep learning technologies are making their mark in various educational domains, offering tailored solutions to improve both teaching and learning processes. By leveraging powerful algorithms, these methods can analyze vast amounts of data and create personalized learning experiences that adapt to individual needs. This offers educators a more effective way to address student weaknesses and optimize their learning paths. Practical applications of deep learning in education are continuously evolving, with numerous case studies and success stories emerging globally.
From intelligent tutoring systems to advanced content recommendations, deep learning facilitates a range of tools designed to enhance educational outcomes. The key advantage lies in its ability to analyze patterns and predict future learning behaviors. These technologies not only help identify gaps in knowledge but also provide actionable insights for curriculum design and student engagement. Below are some of the most impactful applications of deep learning in the educational sector.
Key Applications of Deep Learning in Education
- Personalized Learning - Adaptive learning platforms use deep learning algorithms to adjust lessons in real-time based on student performance.
- Intelligent Tutoring Systems - AI-powered tutors provide one-on-one support, guiding students through complex concepts.
- Automated Grading and Feedback - Deep learning models can grade assignments and provide feedback, saving time for educators and offering instant responses to students.
- Learning Analytics - By analyzing students' interaction patterns with content, deep learning helps educators predict at-risk students and suggest timely interventions.
Insights from Recent Research
Recent studies have demonstrated how deep learning can transform traditional classrooms into interactive and highly responsive learning environments.
"Deep learning systems are not just tools for automation; they have the potential to drive pedagogical innovation by continuously refining the teaching process based on real-time data."
Challenges and Considerations
While the potential of deep learning in education is immense, challenges remain in terms of data privacy, implementation costs, and teacher training. Institutions must address these hurdles to fully integrate AI technologies into their ecosystems. Additionally, the risk of over-reliance on automated systems may hinder the development of critical thinking and creativity in students.
Application | Benefits | Challenges |
---|---|---|
Personalized Learning | Enhanced student engagement and optimized learning pace | Requires extensive data collection and system adjustments |
Intelligent Tutoring Systems | 24/7 student support and personalized guidance | Potential for lack of human empathy in tutoring |
Automated Grading | Efficiency in grading and consistency | Risk of overlooking nuances in creative responses |
How Deep Learning Enhances Personalized Learning Experiences
Deep learning technologies have significantly transformed educational approaches, enabling more personalized and adaptive learning. Through the use of sophisticated neural networks, deep learning systems can analyze large datasets, including student interactions, learning progress, and preferences. This allows for the creation of tailored educational pathways that align with each learner's unique needs. By recognizing patterns and predicting learning outcomes, deep learning can offer students a more dynamic and responsive educational experience.
The adaptability of deep learning models allows for real-time adjustments to content delivery, ensuring that the pace, difficulty, and type of material match the student's current level of understanding. This level of customization not only enhances engagement but also optimizes knowledge retention and performance. Teachers and educational platforms can leverage these insights to provide targeted interventions and track individual growth over time.
Key Benefits of Deep Learning in Personalized Education
- Real-time Feedback: Deep learning systems can instantly evaluate a learner’s responses and provide personalized feedback, helping to address misunderstandings before they escalate.
- Adaptive Learning Paths: Based on continuous data input, the system can modify the learning journey to fit the student’s evolving needs, ensuring that challenges are appropriately balanced.
- Data-Driven Insights: Educators can access detailed analytics about student behavior, performance, and learning styles, which guide more informed decision-making.
How Deep Learning Models Personalize Content
- Content Adaptation: By analyzing past learning behavior, the system identifies which types of content (text, video, interactive exercises) are most effective for a student.
- Skill Gap Identification: Deep learning can spot areas where a student may be struggling and provide additional resources or practice exercises tailored to those specific gaps.
- Learning Speed Adjustment: The system can dynamically alter the pace at which content is presented based on how quickly a student grasps new concepts.
"Deep learning not only predicts how students will perform, but also actively shapes their learning journey, making education more efficient and personalized."
Personalized Learning Aspect | Deep Learning Contribution |
---|---|
Real-time Adjustments | Instant feedback and content adjustments based on student input. |
Content Delivery | Dynamic alteration of material type and complexity to fit student needs. |
Performance Tracking | Continuous data analysis to monitor and guide individual progress. |
Leveraging Neural Networks for Real-Time Student Performance Assessment
In the modern educational landscape, timely feedback on student performance is crucial for effective learning. Traditional assessment methods, often based on periodic exams or assignments, cannot always capture the evolving learning process of each student. The integration of artificial intelligence, specifically neural networks, offers the potential to provide real-time analysis of student progress, allowing educators to tailor their teaching strategies to individual needs.
Neural networks can analyze a variety of data sources, including student responses, participation levels, and even behavioral patterns in online learning platforms. By leveraging deep learning algorithms, these systems are capable of assessing student performance continuously, offering a dynamic and personalized approach to education. This ensures that students receive instant feedback, enabling them to address areas of weakness before they fall behind.
How Neural Networks Contribute to Real-Time Assessment
Neural networks are well-suited to evaluate complex and dynamic student data. Below are some key areas where they enhance real-time performance assessments:
- Personalized Feedback: By analyzing patterns in student behavior, neural networks provide targeted suggestions that adapt to individual learning styles.
- Adaptive Learning Paths: Algorithms can adjust the difficulty level of assignments or quizzes in real time, ensuring that each student is continuously challenged according to their ability.
- Instant Identification of Struggling Students: Neural networks can quickly identify students who are falling behind or not engaging, enabling early intervention.
Furthermore, these technologies can help reduce the administrative burden on educators, allowing them to focus more on pedagogical strategies and less on grading and performance tracking. Below is a table highlighting the comparison between traditional assessment methods and neural network-based assessments:
Feature | Traditional Assessment | Neural Network-Based Assessment |
---|---|---|
Data Collection | Periodic exams, assignments | Continuous data from multiple sources |
Feedback Timing | Delayed feedback | Real-time feedback |
Adaptability | Static | Dynamic and personalized |
"Real-time performance analysis through neural networks not only benefits students by providing immediate guidance, but also empowers educators to adjust their teaching methods based on up-to-the-minute data."
Incorporating Neural Networks in Online Classrooms for Engaging Education
Integrating artificial intelligence and machine learning, particularly deep learning, in virtual learning environments offers opportunities for dynamic and personalized teaching methods. By utilizing advanced algorithms, educators can transform passive learning experiences into highly interactive and engaging sessions. These technologies enable the creation of intelligent systems capable of understanding student behaviors and adapting content accordingly, fostering an environment that tailors to individual needs.
With the rise of virtual classrooms, leveraging deep learning models can enhance interaction through automated feedback, predictive assessments, and real-time content adaptation. The key challenge lies in designing models that balance personalization with scalability, ensuring that each student receives a unique experience while maintaining an effective and manageable course structure for educators.
Applications of Deep Learning in Virtual Classrooms
- Personalized Learning Paths: AI systems can analyze student performance and tailor content to suit their individual pace and learning style.
- Real-Time Assessments: Algorithms can track student engagement and knowledge retention, providing instant feedback and guidance.
- Automated Grading: Deep learning models can grade assignments and tests, ensuring consistency and freeing up time for instructors to focus on interaction.
Benefits and Challenges
Deep learning in virtual classrooms enables a more responsive, efficient, and customized learning experience. However, its integration presents challenges, such as ensuring data privacy and providing equitable access to technology.
Benefits | Challenges |
---|---|
Improved student engagement | Privacy concerns and data security |
Instant and accurate feedback | Technical accessibility for all students |
Personalized learning experiences | Bias in algorithms and content recommendation |
Conclusion
As deep learning continues to evolve, its role in virtual education becomes increasingly prominent. By facilitating real-time, personalized, and adaptive learning experiences, these technologies have the potential to revolutionize education. However, their effective implementation requires addressing technical, ethical, and accessibility concerns to ensure a fair and enriching environment for all students.
Using Deep Learning to Automate Grading and Feedback in Educational Systems
Automating the grading and feedback process is one of the most significant advantages that deep learning offers in the educational domain. By leveraging neural networks and natural language processing (NLP) techniques, it is possible to provide quick, accurate, and personalized assessments of student performance. Such systems can evaluate a wide range of assignments, including essays, quizzes, and problem-solving exercises, offering immediate results and detailed feedback.
Through deep learning, educational systems can alleviate the burden on educators, allowing them to focus on more complex tasks such as one-on-one student interactions. This transformation not only improves efficiency but also provides students with timely guidance, helping them to understand their mistakes and improve over time. The automated feedback loop promotes a more dynamic learning environment, making it possible to scale educational practices to a larger number of students.
How Deep Learning Enhances Grading Systems
Deep learning algorithms can process vast amounts of student data, enabling the automation of grading tasks that would traditionally require significant human effort. These systems are particularly effective at recognizing patterns in text, images, and even spoken language. Below are some key components of how deep learning contributes to this process:
- Essay Evaluation: Neural networks can analyze essays by recognizing grammar, sentence structure, and contextual relevance, providing meaningful scores and detailed comments.
- Multiple Choice and Short Answer Responses: Deep learning models can classify and score student responses with a high degree of accuracy.
- Image-based Assignments: Models trained on visual data can automatically grade assignments that involve diagrams, charts, or sketches.
Benefits of Automating Feedback
Automating feedback through deep learning allows for personalized and adaptive guidance tailored to each student's unique needs. Below are some advantages:
- Speed: Instant feedback helps students identify areas of improvement immediately after completing tasks.
- Consistency: Algorithms ensure that grading and feedback remain consistent across all students, eliminating biases.
- Scalability: Automation makes it feasible to handle large class sizes while maintaining quality feedback.
"By integrating deep learning models into educational platforms, the time spent on grading and feedback can be drastically reduced, allowing educators to focus on fostering student engagement."
Challenges and Considerations
Despite the potential benefits, there are several challenges when implementing deep learning systems for grading:
Challenges | Considerations |
---|---|
Data Quality | Deep learning models rely heavily on large datasets; poor-quality data can negatively impact their performance. |
Interpretability | Ensuring that students and educators understand how the model arrives at its feedback is essential for trust and transparency. |
Bias | Careful attention must be given to ensure that the models do not inadvertently reinforce biases or unfair grading practices. |
Enhancing Personalized Learning Systems with Deep Neural Networks
Recent advancements in artificial intelligence have paved the way for more effective and dynamic educational tools, with adaptive learning systems standing out as a prime example. These systems are designed to adjust the learning path for each student, based on their unique needs and progress. By leveraging deep neural networks, these systems can become more accurate and efficient in predicting student behavior, improving content delivery, and providing real-time feedback. This personalized approach helps to ensure that every learner receives tailored instruction, fostering deeper engagement and better outcomes.
Deep learning models, especially those using neural networks, can enhance adaptive learning platforms in several ways. These models excel at recognizing complex patterns in student data, such as performance trends, preferences, and cognitive abilities. This allows them to continuously refine the learning experience, making adjustments that go beyond simple rule-based approaches. The result is an intelligent, responsive system that not only adapts to the learner’s pace but also anticipates future challenges, creating a more interactive and personalized environment.
Key Benefits of Using Deep Neural Networks in Adaptive Learning
- Improved Accuracy: Deep learning models can process vast amounts of student data, identifying subtle patterns and correlations that might be missed by traditional systems.
- Real-time Adaptation: Neural networks allow systems to continuously monitor student progress and modify learning content in real-time, ensuring that students are always working within their optimal zone of challenge.
- Personalized Feedback: The system can offer tailored recommendations and guidance based on individual learning styles and progress, leading to better student performance.
Application Areas of Deep Learning in Adaptive Systems
- Course Recommendation: Neural networks can suggest relevant courses or learning modules based on a learner's previous performance and preferences.
- Progress Prediction: Deep models can predict a student’s future performance, allowing educators to intervene early if necessary.
- Content Customization: By analyzing students' interaction with materials, deep learning can create personalized content that is more engaging and effective.
"The integration of deep neural networks into adaptive learning systems allows for the creation of an environment that is not only more efficient but also more engaging for the student, improving both learning outcomes and satisfaction."
Challenges in Implementing Deep Learning for Adaptive Learning
Challenge | Description |
---|---|
Data Privacy | Handling sensitive student data requires robust security measures to protect privacy. |
Computational Complexity | Deep learning models are computationally intensive, requiring significant resources and infrastructure. |
Model Interpretability | Interpreting how deep learning models make decisions can be difficult, posing a challenge for educators and developers. |
Exploring Natural Language Processing in Educational Content Creation
Natural Language Processing (NLP) plays a pivotal role in the development of educational resources, offering innovative solutions for creating personalized and interactive content. By leveraging NLP technologies, educators can automate the process of generating instructional materials that are tailored to the specific needs and learning preferences of students. This opens the door for more effective, adaptive learning environments that cater to diverse educational settings.
Furthermore, NLP enables the analysis of large amounts of educational data, extracting meaningful insights that help refine content delivery and improve student engagement. With the ability to process text, recognize patterns, and offer contextual understanding, NLP transforms the way educational content is crafted and delivered to learners.
Applications of NLP in Educational Content Creation
- Automated Content Generation: NLP systems can create quizzes, essays, summaries, and other educational content based on specific learning objectives.
- Personalized Learning Experiences: NLP can analyze students' responses and tailor educational content to match their individual learning styles and progress.
- Language Support: NLP allows for real-time translation and language adaptation, making educational materials accessible to a global audience.
Key Benefits of NLP in Education
- Improved Efficiency: Automating content creation reduces time spent on manual preparation, enabling educators to focus on other critical tasks.
- Enhanced Engagement: Personalized and interactive materials increase student motivation and active participation in learning.
- Data-Driven Insights: NLP can analyze student performance data to provide educators with actionable insights on areas for improvement.
Examples of NLP Tools in Educational Content
Tool | Function | Application in Education |
---|---|---|
Grammarly | Grammar and spell-checking | Assist students with writing assignments, enhancing language proficiency |
QuillBot | Paraphrasing and summarization | Helps students rephrase and summarize texts for better understanding |
Duolingo | Language learning | Provides personalized language lessons using NLP for feedback and practice |
"NLP technologies can revolutionize educational content creation by offering scalability, accessibility, and real-time adaptation to the needs of learners."
Challenges in Integrating Deep Learning in Educational Institutions
Integrating deep learning technologies into educational settings presents several obstacles. Educational institutions face challenges such as limited access to advanced hardware and a lack of technical expertise among faculty and staff. While the potential benefits of deep learning are clear, the infrastructure and resource requirements often hinder widespread adoption. Furthermore, the transition from traditional teaching methods to those powered by artificial intelligence demands significant training and adjustment for both instructors and students.
Another key challenge is the ethical implications and data privacy concerns that arise when utilizing AI-driven learning systems. Educational institutions must ensure the responsible use of data while providing personalized learning experiences. The complexity of managing large datasets, ensuring fairness in AI algorithms, and addressing biases are crucial issues that need to be addressed to successfully implement deep learning solutions.
Key Obstacles in Adopting Deep Learning
- Lack of Resources: Educational institutions often struggle with limited budgets and outdated technology, making it difficult to implement deep learning solutions effectively.
- Insufficient Expertise: Many educators lack the specialized knowledge required to integrate deep learning tools into their curriculum.
- Data Privacy Concerns: Collecting and managing student data raises significant privacy and security issues.
- Resistance to Change: Faculty and students accustomed to traditional educational methods may resist the transition to AI-powered learning platforms.
Ethical and Practical Considerations
- Bias in Algorithms: AI models may inadvertently perpetuate biases, leading to unfair learning outcomes.
- Data Security: Ensuring that student data is securely handled and that AI systems comply with regulations such as GDPR is a significant challenge.
- Adapting Curriculum: Current educational content and methods may need to be completely restructured to leverage deep learning technologies effectively.
To successfully integrate deep learning into education, institutions must invest in the right infrastructure, develop the necessary expertise, and ensure ethical practices in data management.
Key Considerations for Successful Implementation
Consideration | Details |
---|---|
Infrastructure Investment | Schools must upgrade hardware and software to support AI and machine learning applications. |
Teacher Training | Educators should receive professional development to effectively utilize deep learning tools in the classroom. |
Privacy Protection | Institutions must implement stringent data security measures to protect student information. |