Data Driven Language
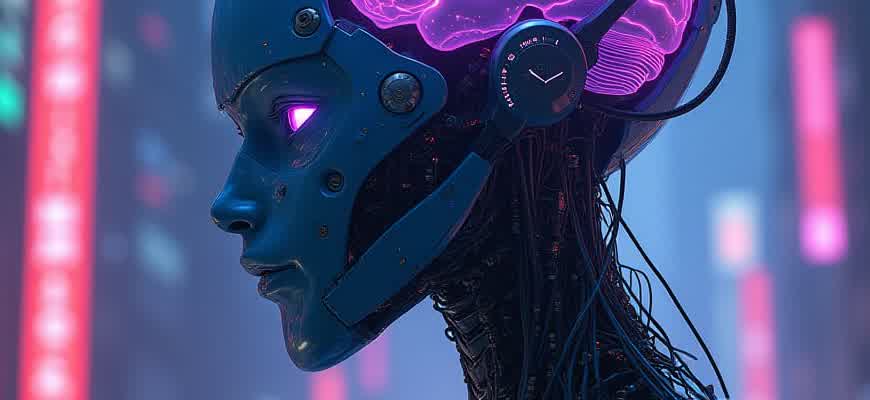
Data-driven language refers to the use of data and analytical tools to shape how we communicate. It is a growing trend in many fields, especially in business, technology, and digital media. By examining patterns in language usage and interactions, organizations and individuals can optimize their communication for more efficient and targeted outcomes.
This method relies heavily on collecting and analyzing large volumes of textual data to understand user behavior, preferences, and trends. Below are some core components that define data-driven language:
- Text Analytics: The process of extracting meaningful insights from written content.
- Behavioral Analysis: Examining how audiences interact with language, often through sentiment analysis or keyword tracking.
- Personalization: Tailoring messages to specific audiences based on their data profiles.
"Incorporating data into communication strategies allows for precise targeting and improved engagement, making content not only relevant but also impactful."
Here’s a breakdown of how this methodology impacts different sectors:
Sector | Application of Data-Driven Language |
---|---|
Marketing | Personalized advertising based on user interaction data. |
Healthcare | Improved patient communication using behavioral insights. |
Education | Adaptive learning systems that cater to individual student needs. |
How Data-Driven Communication Enhances Customer Interaction
The use of data in communication strategies has fundamentally changed how companies engage with their customers. By leveraging insights derived from user behavior, preferences, and past interactions, businesses can tailor their messages to meet the specific needs of their audience. This approach ensures that communication is relevant, timely, and personalized, leading to stronger connections with customers.
Data-driven language focuses on real-time data collection and analysis, which allows brands to fine-tune their communication strategies in ways that were not possible before. Whether through automated messages or human interactions, the power of data enhances the precision of the language used to address individual customers, making each conversation more impactful.
Key Benefits of Data-Driven Customer Communication
- Personalization: Content and messages are tailored to the specific preferences and needs of each customer.
- Relevance: Communication becomes more relevant by using customer data to predict their needs and interests.
- Efficiency: Automated systems powered by data can quickly respond to customer queries, reducing wait times and increasing satisfaction.
How Data Improves Messaging Across Channels
- Email Campaigns: By analyzing open rates, click-through rates, and customer behavior, companies can customize email content to increase engagement.
- Social Media: Data helps brands understand customer sentiment and respond with tailored content in real time.
- Customer Support: Insights from past interactions allow support teams to address issues faster, with a more personalized approach.
"Data-driven language shifts the focus from broad, generalized communication to highly targeted and specific messages, creating a more meaningful interaction with each customer."
Examples of Data-Driven Communication in Action
Customer Interaction | Data Used | Outcome |
---|---|---|
Product Recommendations | Purchase history, browsing patterns | Highly personalized suggestions that increase sales |
Email Follow-ups | Customer feedback, previous purchase data | Increased open rates and engagement |
Support Chatbots | Previous chat history, frequent issues | Faster resolution and better customer satisfaction |
Leveraging Natural Language Processing for Business Insights
Natural Language Processing (NLP) provides businesses with the ability to extract actionable insights from unstructured data such as customer feedback, social media posts, and support tickets. By using advanced algorithms, NLP helps in analyzing the meaning and sentiment behind text, allowing companies to understand customer preferences, detect market trends, and improve decision-making processes. This can directly impact areas like marketing, customer service, and product development.
As businesses face the challenge of handling massive volumes of data, NLP offers a powerful solution for automating data processing and uncovering hidden patterns. By converting qualitative data into quantitative metrics, companies can identify valuable opportunities for growth, risk mitigation, and competitive advantage. The application of NLP tools can also streamline workflow by automatically categorizing, summarizing, and prioritizing information.
Key Benefits of NLP in Business
- Sentiment Analysis: Determine customer opinions and emotions, enabling better service and product positioning.
- Market Trend Detection: Analyze trends and shifts in consumer behavior to stay ahead of competitors.
- Operational Efficiency: Automate routine tasks, such as customer inquiry responses and data categorization, reducing time and resources.
Applications of NLP in Different Industries
- Retail: Monitor online reviews and social media conversations to gauge customer satisfaction and product feedback.
- Finance: Analyze financial reports, news articles, and analyst opinions to predict market trends.
- Healthcare: Extract valuable insights from medical records, patient feedback, and clinical notes to improve patient care.
Impact on Decision-Making
Decision Area | NLP Contribution |
---|---|
Product Development | Identifying features or improvements based on customer feedback and sentiment analysis. |
Customer Experience | Optimizing customer interactions by analyzing common issues and sentiments from support tickets. |
Marketing Strategy | Analyzing consumer behavior and predicting trends to tailor campaigns more effectively. |
By utilizing NLP, businesses can gain real-time insights into customer needs and market dynamics, ultimately leading to more informed and responsive strategies.
Building a Data-Driven Content Strategy for Targeted Marketing
Creating an effective content strategy requires more than just producing engaging material. To reach the right audience, businesses need to leverage data, understanding user behavior, preferences, and trends to shape their approach. By gathering insights from various data sources, marketers can personalize their content and optimize engagement across channels.
A data-driven strategy allows businesses to precisely tailor their messaging and create content that resonates with specific segments. This involves continuous analysis, testing, and adapting based on real-time data, ensuring that every campaign reaches its intended audience with maximum impact.
Key Steps in Developing a Data-Driven Content Plan
- Audience Analysis: Study audience demographics, interests, and online behavior to identify key segments.
- Content Personalization: Utilize insights to create personalized content that speaks directly to each segment’s needs.
- Platform Optimization: Use data to determine the most effective channels and formats for content delivery.
- Continuous Testing: Regularly test content performance and adjust based on real-time results.
Steps for Implementing a Data-Driven Approach
- Define Metrics: Identify specific KPIs (Key Performance Indicators) to track success.
- Collect Data: Use analytics tools to gather data from web traffic, social media, and other touchpoints.
- Analyze Data: Assess trends, patterns, and behaviors to create actionable insights.
- Refine Content Strategy: Continuously adapt content based on feedback and data insights to improve engagement.
"Data-driven strategies are essential for creating content that not only attracts attention but also converts visitors into loyal customers."
Common Metrics for Tracking Success
Metric | Description | Purpose |
---|---|---|
Engagement Rate | Measures how users interact with content (likes, shares, comments) | Assess audience interest and interaction |
Conversion Rate | Tracks how many visitors take the desired action (purchase, sign-up) | Evaluate content effectiveness in driving business goals |
Click-Through Rate | Measures how often users click on content links or calls to action | Gauge the success of specific content or offers |
Leveraging AI for Real-Time Personalization of User Interactions
AI-driven personalization allows for dynamic user engagement based on real-time data. By leveraging algorithms that process user behavior, preferences, and previous interactions, platforms can offer highly customized experiences. This approach not only increases user satisfaction but also drives higher retention and conversion rates. The ability to adapt content, recommendations, and even interface elements to individual users in real time is transforming how businesses approach customer engagement.
Real-time personalization using AI involves analyzing a wide range of data inputs, including location, browsing history, purchase patterns, and even social media activity. This wealth of data allows AI systems to tailor content, recommendations, and responses instantly, ensuring that each user receives a unique experience that feels relevant and timely. Such personalization requires a robust, data-driven architecture capable of processing and interpreting vast amounts of information within milliseconds.
Key Benefits of AI-Driven Personalization
- Enhanced User Engagement: AI personalizes experiences, making interactions more relevant and engaging, which results in longer session times and increased interaction.
- Improved Conversion Rates: Customizing offers and content based on user preferences leads to more successful conversions and higher sales figures.
- Real-Time Adaptability: AI can continuously adapt to changing user behaviors, ensuring content is always relevant and timely.
How AI Adapts in Real-Time
- Behavior Tracking: By monitoring user actions, AI systems can understand preferences and anticipate future needs.
- Content Adjustment: Content, product recommendations, and even UI elements are dynamically adjusted to reflect the user’s current context.
- Instant Feedback: AI can provide personalized feedback or recommendations immediately, improving user satisfaction.
"Real-time AI adaptation ensures that each user experiences a tailor-made journey, which improves not only satisfaction but also business outcomes."
Example: Personalized E-Commerce Experience
Stage | AI-Driven Personalization |
---|---|
Initial Visit | AI analyzes browsing history to recommend products based on user preferences. |
Product Interaction | AI suggests complementary items based on past purchases or browsing behavior. |
Checkout | AI offers personalized discounts or loyalty rewards based on user activity. |
Enhancing Customer Retention with Data-Driven Communication
Effective communication with customers is vital to maintaining long-term relationships and increasing brand loyalty. By leveraging data, businesses can personalize messages and improve engagement, leading to better retention rates. Data-based messaging allows for more relevant interactions with customers, making them feel valued and understood. Understanding the needs and behaviors of customers through data insights helps craft targeted messages that resonate with the individual.
To optimize customer retention, companies should focus on delivering timely, relevant, and personalized content. Using customer behavior data such as purchase history, browsing patterns, and engagement with previous communications, businesses can tailor their messages to specific segments of their audience. This method fosters a deeper connection and encourages customers to return, boosting lifetime value and reducing churn.
Strategies for Data-Driven Messaging
- Personalized Offers: Use purchase history and browsing data to provide targeted discounts and promotions.
- Automated Follow-Ups: Send messages at optimal times based on user activity patterns to encourage repeat engagement.
- Behavioral Triggers: Trigger messages based on specific customer actions, such as cart abandonment or product reviews.
Important Consideration: Not all data is relevant. It is crucial to focus on actionable insights that directly impact customer engagement and retention. A flood of irrelevant messages could overwhelm customers and have the opposite effect.
Metrics to Track for Retention Success
Metric | Purpose |
---|---|
Customer Lifetime Value (CLV) | Measures the total value a customer brings to the business over time. |
Churn Rate | Tracks the percentage of customers who stop engaging or purchasing. |
Engagement Rate | Monitors how frequently customers interact with the messages and content. |
Key Insight: Data-driven messaging isn't just about sending the right offer; it's about sending it at the right time with a message that aligns with customer behavior and preferences.
Analyzing the Influence of Data-Driven Language on Conversion Metrics
Understanding the role of language in conversion optimization has gained increasing attention, especially as data-driven methodologies become more common. By tailoring language based on user preferences and behaviors, businesses can improve customer engagement and encourage action. Through careful analysis of linguistic choices, companies can leverage data to craft messages that resonate more deeply with their audience.
The integration of data insights into communication strategies allows for a more personalized approach. This not only boosts relevance but also strengthens the likelihood of conversions. To measure the impact effectively, it is essential to track various metrics such as click-through rates, engagement levels, and the final conversion outcomes of tailored messaging.
Key Factors to Measure the Impact of Language on Conversion Rates
- Personalization – How well does the language reflect individual user behaviors and preferences?
- Clarity of Call-to-Action (CTA) – Is the CTA clearly defined and compelling enough to drive action?
- Urgency – Does the language effectively convey a sense of urgency or importance to prompt immediate action?
To properly evaluate these factors, businesses can look at several performance indicators:
- Click-through Rates (CTR): Measure the number of clicks on targeted messages compared to the number of times those messages are viewed.
- Engagement Metrics: Track how users interact with content such as videos, images, and text.
- Conversion Rates: The ultimate goal, measuring how many visitors take the desired action after encountering a data-driven message.
"Using language grounded in data can lead to more meaningful interactions with potential customers, ultimately driving higher conversion rates."
Here's an example of how varying data-driven language can influence conversion results:
Language Approach | Conversion Rate (%) |
---|---|
Personalized message with urgent CTA | 18.2% |
Generic message with standard CTA | 9.5% |
Optimizing Multilingual Support with Data-Driven Approaches
In today's globalized world, providing seamless multilingual support is a key factor for businesses aiming to reach diverse audiences. Leveraging data to drive language solutions enhances the accuracy and efficiency of multilingual systems, allowing businesses to better connect with customers in their native languages. By applying data analytics and machine learning, companies can streamline the translation process and maintain high-quality communication across various languages.
Data-powered solutions facilitate the adaptation of content to different linguistic and cultural contexts. With the increasing availability of large datasets, businesses can utilize advanced algorithms to predict language preferences, optimize translations, and identify regional nuances. This leads to more personalized customer interactions and a stronger global presence.
Key Techniques for Multilingual Optimization
- Machine Translation (MT): The use of automated systems to translate content between languages, supported by continuous training using data from user interactions.
- Natural Language Processing (NLP): Enhances language understanding by analyzing linguistic data, allowing for context-aware translations and accurate sentiment analysis.
- Localization and Personalization: Data helps tailor content to specific regions, considering not only linguistic differences but also cultural preferences and local customs.
Steps for Effective Data-Driven Multilingual Solutions
- Collect diverse linguistic data from various regions to build accurate models.
- Utilize AI-powered translation tools to streamline content conversion.
- Implement quality assurance processes using data insights to ensure translations maintain context and meaning.
- Regularly update models with new data to adapt to changing language trends and user feedback.
"The effectiveness of multilingual support is determined not just by translation accuracy but by understanding the cultural context and nuances of each language." – Expert on Data-Driven Solutions
Challenges and Considerations
Challenge | Solution |
---|---|
Data Scarcity in Low-resource Languages | Use of transfer learning to adapt models trained on high-resource languages to low-resource ones. |
Maintaining Quality Across Multiple Languages | Continuous improvement of machine translation models through user feedback and linguistic data updates. |
Enhancing Language Models with Data Analytics for Improved Results
Integrating data analytics into language models enables more precise predictions and context-aware outputs, making them more effective in real-world applications. By leveraging large datasets, you can fine-tune model behavior and improve accuracy. Data-driven approaches provide valuable insights into user preferences, allowing for personalized experiences that go beyond generic responses.
To achieve better outcomes, incorporating advanced analytics such as sentiment analysis, trend detection, and user behavior modeling is essential. These data-driven methods allow language models to adapt to varying contexts, improving their relevance and responsiveness over time.
Key Steps to Integrate Data Analytics
- Data Collection: Gather diverse datasets, including structured and unstructured data, to ensure the model receives comprehensive input.
- Feature Engineering: Extract meaningful features from raw data that will guide the model in understanding context, sentiment, and nuances.
- Model Tuning: Continuously fine-tune the model by incorporating feedback loops that adjust the model based on real-world data performance.
By combining data analytics and language models, you are not just improving performance, but also ensuring that the model becomes increasingly sophisticated in predicting and generating relevant responses.
Types of Analytics to Apply
- Sentiment Analysis: Understanding the emotional tone of user inputs allows for more empathetic and appropriate responses.
- Contextual Awareness: Analytics tools can help the model detect situational context, ensuring outputs align with user intent.
- Trend Analysis: Identifying patterns in user behavior helps to predict future interactions, leading to more accurate recommendations.
Data-Driven Approaches in Language Models
Data Analytics Technique | Benefit for Language Model |
---|---|
Sentiment Analysis | Enables the model to gauge emotional tone for more sensitive and relevant responses. |
Behavioral Insights | Improves the model's ability to personalize interactions based on historical user data. |
Pattern Recognition | Helps predict user needs, enhancing user engagement and satisfaction. |