Ai Based Personalized Learning Platform
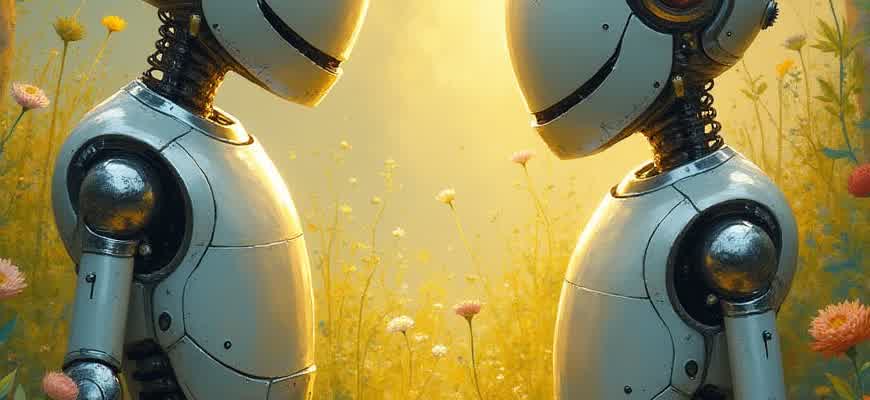
Modern educational platforms are evolving to integrate artificial intelligence (AI) technologies, providing a more tailored and efficient learning experience. These systems leverage advanced algorithms to assess individual learner needs and adapt content accordingly. With AI, learning journeys are no longer one-size-fits-all; instead, students receive personalized instruction based on their strengths, weaknesses, and progress.
AI-powered platforms enable the automation of assessments and feedback, offering continuous, real-time evaluation. This allows learners to advance at their own pace, while ensuring they grasp core concepts before moving on to more complex material.
Key Features of AI-Driven Learning Systems:
- Adaptive content delivery based on performance
- Real-time feedback and progress tracking
- Personalized learning pathways
- Automatic identification of knowledge gaps
For educational institutions, these platforms provide valuable insights that inform teaching strategies and curriculum design. Below is a table comparing traditional learning approaches with AI-powered systems:
Aspect | Traditional Learning | AI-Based Learning |
---|---|---|
Content Delivery | Fixed curriculum | Dynamic, personalized content |
Progress Monitoring | Periodic assessments | Continuous, real-time tracking |
Learning Pace | Standard pace for all | Flexible, learner-paced |
AI-Driven Custom Learning Platforms
AI-powered educational platforms offer tailored learning experiences by analyzing user behavior, preferences, and performance. These systems utilize machine learning algorithms to adapt content and learning pathways to individual needs, ensuring that each user receives the most relevant material at the right time. By leveraging data-driven insights, these platforms can identify gaps in knowledge and areas for improvement, allowing students to progress at their own pace.
Incorporating artificial intelligence into personalized learning allows for more efficient and effective educational journeys. AI systems continuously adjust the complexity of content based on real-time data, creating a dynamic environment where learners are neither overwhelmed nor under-challenged. The result is a highly individualized experience that can significantly improve student engagement and retention.
Key Features of AI-Based Learning Platforms
- Adaptive Learning Algorithms: These algorithms adjust the difficulty of tasks based on a student's progress, ensuring that they are always engaged at an appropriate level.
- Personalized Content: AI generates learning materials tailored to the unique needs and interests of each user, increasing relevance and motivation.
- Continuous Assessment: Real-time feedback is provided, allowing learners to track their performance and make immediate adjustments to their study strategies.
- Data-Driven Insights: AI analyzes learning patterns and provides actionable insights to educators, helping them to support students more effectively.
Benefits of AI in Education
- Efficiency: Automates administrative tasks, giving educators more time to focus on direct interaction with students.
- Scalability: Provides access to quality learning materials for a wide range of learners, regardless of location or background.
- Customization: Tailors educational experiences to suit diverse learning styles and needs, promoting inclusivity.
Impact on Learning Outcomes
Metric | Before AI Implementation | After AI Implementation |
---|---|---|
Student Engagement | Medium | High |
Completion Rates | Low | Increased |
Test Scores | Average | Improved |
"AI in education is not about replacing teachers but empowering them with the tools to better support each student."
How AI Adapts Learning Pathways to Individual Needs
AI-driven learning platforms leverage advanced algorithms to personalize educational content, ensuring that each learner's unique strengths and weaknesses are addressed effectively. By collecting data on the learner’s behavior, preferences, and performance, these systems can dynamically adjust learning pathways to provide tailored experiences. This allows students to engage with material at their own pace, focusing on areas where they need the most improvement while skipping topics they have already mastered.
Through continuous analysis, AI can create personalized content delivery strategies. This adaptability is achieved by identifying patterns in user interaction, allowing the platform to continuously refine the learning process. As a result, students experience an optimized, individualized learning journey that promotes better engagement and improved outcomes.
Dynamic Learning Adjustments
- Adaptive content delivery: AI customizes the sequence and difficulty level of lessons based on learner progress.
- Real-time feedback: Instant feedback is provided, helping learners identify and correct mistakes as they occur.
- Personalized assessments: AI designs quizzes and tests that align with the learner's current level of understanding.
AI-powered learning systems use performance metrics to tailor lessons, optimizing the educational experience in real-time.
How AI Personalizes Learning Paths
- Data Collection: Learner performance, engagement, and preferences are tracked.
- Pattern Recognition: AI identifies areas where the learner excels and struggles.
- Content Adjustment: Based on insights, the AI modifies learning resources and strategies accordingly.
Learning Path Optimization Example
Stage | AI Action | Effect on Learning |
---|---|---|
Initial Assessment | AI evaluates learner’s knowledge | Identifies knowledge gaps and strengths |
Lesson Customization | AI adjusts difficulty based on learner performance | Ensures optimal challenge and engagement |
Feedback Loop | AI provides instant feedback and adapts the path | Promotes continuous improvement and retention |
Enhancing Student Engagement with Data Analytics
In the era of personalized learning, data analytics plays a critical role in improving student engagement by providing insights into learning patterns, preferences, and progress. By analyzing various data points such as time spent on tasks, response times, and interaction rates, educators can gain a deeper understanding of each student's strengths and weaknesses. This allows for the customization of learning experiences that are tailored to the individual needs of each student, thus increasing their involvement and motivation in the learning process.
Through the application of data analytics, educators can identify trends, track student progress, and make real-time adjustments to learning materials. These insights help in determining which learning strategies are most effective, creating a more interactive and engaging environment. Students, in turn, benefit from a learning approach that adapts to their unique preferences and pace, fostering greater engagement and improving overall academic outcomes.
Data-Driven Insights for Personalized Learning
- Student Interaction Patterns: Tracking how often students interact with different types of content helps determine their engagement level and preferred learning style.
- Progress Monitoring: Continuous data collection enables the tracking of each student's learning curve, identifying areas of strength and areas needing improvement.
- Behavioral Analytics: Analyzing students' responses to quizzes, assignments, and assessments provides insight into which topics are most challenging, allowing for targeted interventions.
"Data-driven personalization enables educators to craft learning paths that are both engaging and effective, improving retention and performance."
Using Data to Shape Learning Environments
- Real-Time Feedback: Analytics allow for immediate feedback to students, keeping them motivated and informed about their progress.
- Adaptive Learning Paths: Based on the insights gathered from student data, educators can create flexible learning paths that adjust to the student’s pace and needs.
- Resource Allocation: Data helps in identifying the most effective resources and tools, ensuring that students are provided with the best materials for their learning journey.
Analytics Type | Impact on Engagement |
---|---|
Learning Speed Data | Helps to adjust content delivery to avoid overwhelming or under-challenging students. |
Content Interaction Frequency | Indicates student interest, helping to tailor future content to maintain high engagement. |
Assessment Scores | Identifies areas of difficulty, enabling the creation of targeted review materials and focused support. |
Integrating Real-Time Feedback for Improved Performance
Real-time feedback is essential for accelerating the learning process within AI-powered educational platforms. By providing instant responses to learners’ actions, these systems help in identifying gaps in knowledge and offering tailored recommendations for improvement. This approach not only boosts engagement but also enhances the overall effectiveness of personalized learning pathways. In contrast to traditional feedback models, which often rely on periodic assessments, real-time feedback enables students to immediately recognize areas of strength and weakness.
To optimize performance and encourage continuous progress, integrating a dynamic feedback loop allows for more precise adjustments in content delivery and learning strategies. This method also empowers students by giving them control over their learning journey, leading to improved outcomes. Below are key benefits of integrating real-time feedback in personalized learning environments:
- Immediate Adjustments: Provides instant insights into mistakes or misunderstandings, allowing students to make corrections on the spot.
- Adaptive Learning Paths: Feedback guides the AI to modify the content and difficulty level, ensuring that each learner faces an appropriate level of challenge.
- Motivation and Engagement: Instant acknowledgment of progress can enhance motivation, fostering a sense of accomplishment and encouraging further effort.
Real-time feedback should be multi-dimensional, not only providing answers but also offering suggestions for improvement and next steps. Effective integration requires the AI system to continuously analyze learner responses and provide both qualitative and quantitative data. The following table highlights different types of real-time feedback and their impact on performance:
Feedback Type | Impact on Performance |
---|---|
Corrective Feedback | Helps learners identify and correct mistakes quickly, preventing misconceptions from spreading. |
Guided Feedback | Offers suggestions and resources to help learners improve their approach or understanding. |
Motivational Feedback | Reinforces positive behavior and achievements, boosting self-esteem and encouraging persistence. |
Real-time feedback creates a seamless learning experience that adapts to each student’s pace and needs, enhancing both retention and understanding.
AI Algorithms for Optimizing Learning Content Delivery
Artificial Intelligence (AI) plays a crucial role in adapting learning experiences to meet individual needs. AI algorithms allow platforms to analyze vast amounts of data to customize content delivery. These algorithms can optimize when, how, and in what format learning materials are presented, enhancing student engagement and retention. By tracking progress and behavior, AI adjusts the difficulty and pacing of lessons in real time, making the learning experience more efficient and personalized.
One of the main goals of AI in education is to ensure that the right content reaches the right learner at the right time. Through predictive analytics and machine learning models, AI systems are able to understand individual learning patterns, preferences, and performance trends. This results in content being dynamically adjusted, improving knowledge acquisition and minimizing cognitive overload.
Key Algorithms Used for Optimizing Content Delivery
- Collaborative Filtering: This algorithm recommends learning resources based on similarities between learners or content, facilitating personalized learning paths.
- Natural Language Processing (NLP): NLP is used to analyze learner responses and adapt instructional content in real time, ensuring that lessons are relevant and comprehensible.
- Reinforcement Learning: Instructors can utilize this method to identify the most effective teaching strategies, allowing the system to evolve and improve over time.
- Content-Based Filtering: This method focuses on learner preferences, recommending materials similar to those previously engaged with to maximize content relevance.
AI's Impact on Content Delivery Optimization
AI algorithms provide the opportunity to create highly tailored learning experiences. By continuously assessing learner performance, the system can adapt content delivery to avoid stagnation or overload. Key areas that AI optimizes include:
- Personalized Pacing: AI adjusts lesson speed to match a learner's comprehension level.
- Content Customization: It selects and organizes content based on past interactions and performance data.
- Adaptive Feedback: AI provides instant feedback, ensuring students receive timely corrections or encouragement.
AI-driven learning platforms significantly enhance the learning experience by offering a dynamic and tailored approach that traditional educational models cannot match.
Comparison of Common AI Algorithms for Learning Optimization
Algorithm | Purpose | Key Benefit |
---|---|---|
Collaborative Filtering | Suggests content based on learner behavior similarities. | Enhances discovery of relevant materials and peer learning. |
Natural Language Processing | Analyzes text and learner responses for content adaptation. | Improves content comprehension and engagement. |
Reinforcement Learning | Optimizes teaching strategies through trial and error. | Ensures continuous improvement in instructional methods. |
Ensuring Scalability in AI-Powered Education Systems
As educational platforms evolve, ensuring their ability to scale is a fundamental aspect of integrating AI-based systems. Scalability refers to the capacity of an education platform to handle a growing number of users, courses, and data processing demands without compromising performance. To achieve scalability, AI systems must be built on robust architectures that can expand dynamically, accommodating the increasing complexity of educational content and student interactions.
For AI-powered educational systems to scale effectively, it is crucial to consider factors such as cloud infrastructure, modular design, and continuous optimization. This approach not only supports a growing user base but also ensures that the platform remains efficient and adaptive as educational needs evolve over time.
Key Strategies for Scalability
- Cloud Infrastructure: Leveraging cloud services allows the platform to grow in capacity as demand increases without major hardware investments.
- Modular Architecture: Designing systems with modular components ensures that new features or updates can be added seamlessly without disrupting the entire platform.
- Data Optimization: Optimizing algorithms and database management systems is essential to efficiently process large amounts of user data and provide real-time insights.
Important Considerations
“A well-designed AI-powered platform should handle spikes in user activity, like peak exam periods, without degradation in performance.”
- Load Balancing: Distributing the workload evenly across multiple servers prevents any one system from becoming a bottleneck, ensuring consistent performance even during high-demand periods.
- Real-Time Data Processing: AI systems must be capable of processing data instantaneously, providing personalized feedback and adaptive learning pathways.
- Integration of New Technologies: The platform should be capable of integrating emerging technologies (e.g., augmented reality, IoT) without requiring significant restructuring.
Scalability Metrics
Metric | Description |
---|---|
System Load | Measures the platform's capacity to handle simultaneous users and data requests. |
Response Time | Tracks how quickly the system responds to user queries and interactions. |
Data Throughput | Evaluates the system's ability to process large volumes of data without delays. |
Measuring Learning Outcomes Through AI-Driven Assessments
AI-based assessments are transforming how educational progress is tracked and evaluated. By utilizing intelligent algorithms, these systems are capable of adapting to the unique learning styles and abilities of each individual, offering a more accurate representation of their performance. This technology enables the creation of personalized assessment environments that adjust in real-time to the learner's responses, promoting a deeper understanding of the subject matter.
Traditional assessment methods often fail to account for the full scope of a learner's capabilities. In contrast, AI-driven evaluations are dynamic and flexible, allowing for continuous feedback and personalized learning paths. These systems not only measure performance but also identify areas of improvement, enabling more targeted instructional support.
AI in Formative and Summative Assessments
AI technologies can be employed in both formative and summative assessments, offering insights into the learner's development throughout their educational journey.
- Formative Assessments: Real-time data collection allows AI to provide ongoing feedback, ensuring that learning interventions are timely and relevant.
- Summative Assessments: These assessments, typically used to gauge overall progress, can benefit from AI’s ability to analyze trends in learning and predict final performance outcomes.
Key Features of AI-Driven Assessments
Feature | Description |
---|---|
Personalization | Adapts to each learner's strengths, weaknesses, and pace of learning. |
Real-Time Feedback | Provides immediate responses to learner actions, enhancing engagement and knowledge retention. |
Data-Driven Insights | Collects performance data to identify learning trends and inform instructional decisions. |
"AI-driven assessments not only gauge what learners know but also how they learn, enabling educators to tailor their teaching strategies effectively."
Security and Privacy Considerations in AI-Driven Educational Platforms
As AI-based personalized learning platforms become increasingly prevalent, it is crucial to address concerns related to security and privacy. The integration of machine learning and data analytics in education requires platforms to process large amounts of sensitive user data, including personal information, learning behaviors, and progress. Ensuring the confidentiality, integrity, and availability of this data is essential to maintaining trust among users, including students, parents, and educational institutions.
In order to safeguard user privacy and ensure platform security, several key considerations must be taken into account during the design and implementation of these systems. AI technologies not only have the potential to enhance educational experiences but also introduce significant risks related to data breaches, unauthorized access, and the misuse of personal information. Addressing these concerns effectively is vital to promoting the ethical use of AI in education.
Key Security Risks
- Data Breaches: Unauthorized access to student data could expose sensitive information, such as learning history, grades, and personal details.
- Insecure Communication: Lack of encryption in data transmission could lead to data interception during interactions between students and the platform.
- Malware and Cyberattacks: Platforms could become targets for malicious activities aimed at stealing or corrupting data.
- Data Misuse: Inadequate controls might result in the inappropriate use of user data, such as for commercial purposes or unauthorized profiling.
Privacy Protection Measures
Privacy by Design is an essential concept for AI-driven educational platforms, where privacy features are incorporated into the platform's core structure from the outset. Below are some of the strategies that can be implemented:
- Data Anonymization: Personal identifiers should be removed or obfuscated to protect students' identities during data analysis.
- Encryption: Encrypting all sensitive data both in transit and at rest helps ensure that only authorized users can access it.
- Access Control: Implementing strong user authentication mechanisms to ensure that only authorized individuals can access or modify sensitive data.
- Regular Audits: Continuous monitoring of data access and usage should be performed to detect potential security breaches early.
Key Legal and Regulatory Compliance
AI-powered educational platforms must adhere to strict data protection regulations to ensure compliance with privacy laws such as the GDPR and FERPA. Failure to comply with these regulations can result in severe penalties and loss of trust. Some key aspects include:
Regulation | Key Requirements |
---|---|
GDPR | Requires platforms to obtain explicit consent for data collection, allow users to access and delete their data, and implement robust security measures. |
FERPA | Mandates strict guidelines for the protection of educational records and limits access to student information without consent. |
Note: Compliance with legal frameworks is not only a legal obligation but also an essential practice for building trust and accountability in AI-based educational platforms.