Role of Machine Learning in Education
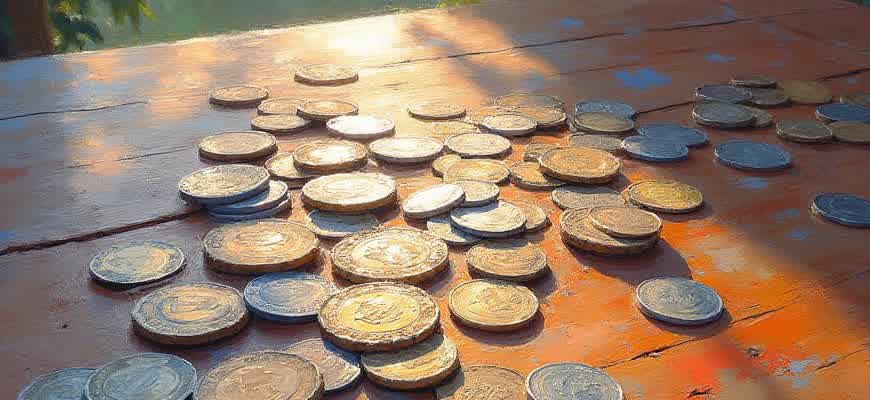
Machine learning (ML) is revolutionizing education by providing personalized learning experiences, optimizing administrative tasks, and enhancing content delivery. Through advanced algorithms, ML can analyze vast amounts of student data to identify patterns, predict future performance, and tailor educational approaches. This technology not only improves learning outcomes but also empowers teachers to make data-driven decisions for better student engagement.
The integration of ML in education brings several advantages, which can be grouped into the following categories:
- Personalized Learning: ML adapts lessons to fit individual learning styles, offering customized recommendations based on performance data.
- Administrative Efficiency: Automates grading, scheduling, and resource management, reducing the workload of educators.
- Data-Driven Insights: Helps educators track progress and predict student needs, allowing for proactive intervention.
"Machine learning provides a unique opportunity to bridge educational gaps by offering real-time feedback and adjustments tailored to individual students."
The following table summarizes the key benefits and challenges of implementing ML in education:
Benefit | Challenge |
---|---|
Improved student engagement and performance | Data privacy concerns and ethical considerations |
Efficient use of resources | Initial implementation cost and training requirements |
Real-time feedback and adaptability | Risk of over-reliance on technology |
How Machine Learning Customizes Learning Paths for Students
Machine learning (ML) algorithms have the potential to dramatically transform the way education systems approach individualized learning. By leveraging vast amounts of student data, ML models can design personalized learning experiences that adapt to the strengths, weaknesses, and learning styles of each student. This level of customization is made possible through the use of predictive analytics, which identify patterns in student behavior and performance to offer the most relevant resources, assignments, and instructional approaches.
One of the key benefits of this approach is that it enables educators to move away from the "one-size-fits-all" teaching model and instead offer a learning journey that is aligned with each student's pace and preferences. ML systems can track progress in real-time and adjust content accordingly, ensuring that students are neither overwhelmed nor under-challenged.
Personalization Techniques in Machine Learning
- Adaptive Learning Systems: These systems use real-time data to adjust the difficulty level of lessons based on student performance.
- Content Recommendation: ML algorithms analyze past interactions to suggest relevant reading materials, exercises, or multimedia content.
- Behavioral Tracking: By continuously monitoring student engagement and responses, machine learning tools can predict potential areas of struggle.
"Machine learning enables the creation of dynamic learning environments where each student's path is continuously tailored to their unique needs and capabilities."
Impact on Learning Outcomes
The impact of ML-driven personalization is significant. Studies show that students benefit from an environment where they can progress at their own pace, reducing frustration and increasing engagement. Personalized pathways also foster deeper learning by targeting areas where the student may need the most support. The following table highlights the core differences between traditional and ML-enhanced education systems:
Traditional Education | Machine Learning-Powered Education |
---|---|
Standardized curriculum for all students | Adaptive learning paths tailored to individual progress |
Limited feedback and static assessments | Real-time feedback and dynamic adjustments based on performance |
Generalized teaching methods | Personalized content recommendations and instructional strategies |
Using Predictive Analytics to Enhance Real-Time Student Performance
Predictive analytics is revolutionizing the educational landscape by offering real-time insights into student performance. By analyzing historical data, learning behaviors, and other relevant metrics, machine learning algorithms can predict how a student will perform in future assessments, enabling educators to intervene promptly. This approach not only assists in identifying students at risk of underperforming but also provides actionable insights to tailor instructional strategies effectively.
Through real-time data collection and analysis, predictive models are able to offer dynamic insights that adjust as new information becomes available. These models help educators monitor students' progress and make immediate changes to their teaching methods, leading to a more personalized learning experience that fosters better outcomes.
Key Benefits of Predictive Analytics in Education
- Early Identification of At-Risk Students: By analyzing student data, early signs of academic struggles can be detected, allowing timely interventions.
- Personalized Learning: Teachers can customize lessons based on the individual needs of students, ensuring more effective learning experiences.
- Optimized Resource Allocation: Resources such as tutoring and extra help can be allocated where they are most needed, improving student engagement and outcomes.
Implementation Steps for Educators
- Data Collection: Gather data on students' previous academic performance, attendance, engagement, and behavior.
- Model Training: Use machine learning algorithms to analyze this data and develop predictive models based on trends and patterns.
- Real-Time Monitoring: Continuously track student progress through integrated tools that provide up-to-date insights into performance.
- Intervention and Adjustment: Use the predictive insights to adjust teaching methods, offer additional support, or modify the curriculum for students who need it.
Example: Data Utilization in Classroom Settings
Metric | Impact on Student |
---|---|
Attendance Rates | Predictive models identify a student’s likelihood of poor performance due to irregular attendance, allowing for early intervention. |
Participation Levels | Low participation can signal a lack of understanding, which triggers personalized instructional support to boost engagement. |
Past Academic Performance | Historically underperforming students can be flagged, prompting additional resources to improve their outcomes. |
"Real-time data, when paired with predictive analytics, transforms the traditional education model, allowing for proactive engagement and support for each student based on their unique needs."
Enhancing Student Engagement with Adaptive Learning Platforms
Adaptive learning platforms leverage machine learning algorithms to personalize educational content and activities, ensuring that students are challenged at the right level. These platforms analyze learners' progress and adjust the material accordingly, providing targeted feedback and exercises. By creating tailored learning experiences, adaptive systems encourage deeper engagement and foster continuous improvement. This dynamic interaction with the content can keep students motivated and invested in their educational journey.
As educational institutions embrace these platforms, they can help mitigate the one-size-fits-all approach, which often leaves some students struggling while others are under-stimulated. With adaptive learning, each student receives a unique set of resources designed to maximize their learning potential. This individualized approach not only enhances engagement but also optimizes the learning process itself.
Key Benefits of Adaptive Learning Platforms
- Personalization: Learning material is tailored to the specific needs of each student, promoting mastery of content.
- Immediate Feedback: Students receive real-time feedback on their performance, allowing them to address gaps in knowledge promptly.
- Increased Motivation: By continuously adjusting difficulty levels, adaptive platforms keep students engaged without overwhelming them.
Impact on Learning Outcomes
"Adaptive learning allows learners to progress at their own pace, enhancing their ability to retain and apply knowledge."
- Better retention of concepts due to repeated exposure to critical material.
- Improved performance in assessments by targeting specific areas of weakness.
- Higher levels of student satisfaction and engagement with course content.
Challenges and Considerations
Challenge | Consideration |
---|---|
Data privacy concerns | Ensuring secure handling of students' personal and academic data. |
Access to technology | Providing equitable access to the necessary devices and internet connectivity for all students. |
Teacher adaptation | Teachers must learn how to integrate adaptive learning systems effectively into their curriculum. |
Streamlining Administrative Tasks with Automated Grading Systems
The integration of machine learning into educational systems has brought about significant improvements in administrative efficiency. One of the key areas where technology has proven indispensable is in grading systems. Automated grading systems powered by artificial intelligence (AI) are transforming how assessments are conducted, saving educators countless hours while ensuring consistent and objective evaluation. These systems can handle a wide variety of tasks, from grading multiple-choice exams to more complex assessments like essays.
Through the use of advanced algorithms, automated grading tools can quickly assess large volumes of student submissions, providing immediate feedback. This allows educators to focus on more meaningful aspects of teaching, such as personalized instruction. Below are the primary benefits of these systems:
- Time Efficiency: Educators can grade assignments in a fraction of the time it would take manually.
- Accuracy and Consistency: AI systems are not prone to human error, ensuring that each student's work is evaluated fairly and consistently.
- Instant Feedback: Students can receive immediate results, enabling them to learn from their mistakes and make improvements faster.
- Scalability: Automated grading systems can handle a high volume of assessments, making them ideal for large courses or institutions.
Automated grading systems ensure that educators can spend more time on teaching rather than administrative tasks, allowing for a more personalized learning experience for each student.
In addition to these benefits, these systems can also improve data analysis capabilities. For instance, tracking student progress over time becomes more straightforward as AI tools can compile and analyze performance trends, offering educators valuable insights into areas where students may need additional support.
Type of Assessment | Automated Grading Capability |
---|---|
Multiple-Choice | Instant grading with immediate feedback |
Essays | AI-driven analysis based on grammar, structure, and content relevance |
Assignments/Projects | Automated assessment using predefined criteria and rubrics |
By automating grading tasks, educational institutions can enhance operational efficiency, reduce the workload on teachers, and provide a better experience for students. As technology advances, these systems are likely to become even more sophisticated, further shaping the future of education.
Machine Learning’s Influence on Educational Curriculum Design
Machine learning (ML) is reshaping the way educational content is developed and delivered. By analyzing vast amounts of student data, ML models can provide insights into how learners engage with material, their strengths and weaknesses, and how content can be adjusted to improve outcomes. This level of personalized learning is now integrated into curriculum design, allowing educational institutions to create more dynamic and responsive teaching materials.
Curriculum development, once a static and one-size-fits-all process, is now being transformed into a more adaptive system. Educators can use predictive algorithms to determine the most effective teaching strategies for different learning styles and proficiency levels. By leveraging data-driven insights, institutions can optimize course content, pacing, and assessments for individual students or groups, ensuring a more tailored learning experience.
Key Areas Affected by ML in Curriculum Design
- Personalized Learning Paths: ML algorithms can suggest customized learning journeys based on a student’s previous performance, preferences, and learning speed.
- Adaptive Content Delivery: ML allows for the real-time adjustment of content difficulty, based on how well students are grasping the material.
- Assessment Optimization: Automated grading and feedback mechanisms ensure that evaluations are both consistent and timely, allowing for quick adjustments to teaching strategies.
Impact on Course Development Workflow
- Data Collection and Analysis: ML models analyze student interactions and behaviors to determine where the curriculum can be improved.
- Course Customization: Based on the analysis, educators can fine-tune lesson plans and resource allocation to better align with student needs.
- Continuous Feedback Loop: Using predictive models, institutions can continuously update and optimize courses to stay relevant to the changing needs of students.
"Machine learning allows educational systems to move beyond standardized teaching methods, offering more adaptive and personalized pathways for students."
Example: Data-Driven Curriculum Adjustments
Aspect | Traditional Approach | ML-Enhanced Approach |
---|---|---|
Course Pacing | Fixed schedule, one pace for all | Adaptive pacing based on student progress |
Assessments | Periodic exams | Continuous formative assessments with instant feedback |
Content Delivery | Standardized content for all | Customized content for individual needs |
Addressing Learning Gaps: Machine Learning in Special Education
Machine learning (ML) plays an important role in overcoming challenges in special education by offering personalized learning experiences. It allows educators to tailor content based on individual needs, ensuring that students with disabilities receive the support they need to thrive. Machine learning algorithms can analyze students' learning patterns and provide insights into their strengths and weaknesses, leading to more effective interventions and targeted learning strategies.
Through adaptive technologies, machine learning can assist in creating a more inclusive environment by addressing the unique learning gaps of each student. These technologies help identify the most suitable learning materials and methods, which can be particularly beneficial for students with learning disabilities, autism spectrum disorders, and other challenges. With data-driven insights, educators can adjust their teaching methods to better accommodate each student's learning pace and style.
How Machine Learning Supports Special Education
- Personalized Learning Paths: Machine learning algorithms analyze students' performance data and adapt learning materials accordingly, ensuring each student receives content that matches their current level of understanding.
- Real-Time Feedback: ML-powered tools provide instant feedback, allowing students to improve continuously without waiting for traditional assessments or evaluations.
- Identifying Learning Disabilities: Machine learning can detect early signs of learning disabilities by monitoring patterns in a student’s performance, enabling early interventions.
Machine learning technology in special education creates personalized, data-driven learning experiences, helping students overcome barriers that would otherwise limit their educational progress.
Key Technologies and Tools in Special Education
- Speech Recognition Software: Useful for students with speech impairments or difficulties in communication, this tool helps convert speech to text, facilitating better interaction and learning.
- Assistive Learning Apps: ML-powered apps adapt to the specific needs of students, offering tailored exercises and practice sessions.
- Behavioral Analysis Tools: These tools track students' behaviors and provide valuable insights to educators, helping them adjust teaching strategies in real-time.
Benefits for Students with Special Needs
Benefit | Explanation |
---|---|
Improved Engagement | Students become more engaged when learning materials are tailored to their abilities and needs. |
Faster Progress | Personalized support ensures that students can progress at their own pace, leading to quicker learning outcomes. |
Increased Independence | ML tools enable students to take control of their learning, reducing dependency on traditional teaching methods. |
AI-Driven Tools for Teachers: Automating Routine Classroom Tasks
In modern education, teachers are often burdened with time-consuming tasks that can detract from their primary role of teaching. Artificial Intelligence (AI) technologies are increasingly being used to streamline these tasks, allowing educators to focus more on student interaction and less on administrative work. By automating routine processes, AI tools help in enhancing the efficiency and effectiveness of classroom management.
AI-driven tools are designed to assist teachers in a variety of ways, reducing their workload and improving the overall classroom experience. These tools use machine learning algorithms to perform tasks such as grading assignments, creating lesson plans, and tracking student progress. The result is a more organized and productive learning environment, where educators can dedicate more time to personalized teaching.
Key AI Tools for Teachers
- Automated Grading Systems: AI can evaluate assignments and quizzes, offering instant feedback to students. These systems use natural language processing and machine learning to assess written content.
- Lesson Plan Creation: AI-driven platforms can suggest personalized lesson plans based on student data, ensuring that the material is tailored to their learning needs.
- Progress Tracking: AI can monitor student performance over time, identifying trends and offering insights into areas that require improvement.
- Administrative Assistance: AI tools can automate scheduling, attendance tracking, and report generation, significantly reducing the administrative burden on teachers.
Benefits of AI Tools in Classroom Management
By automating repetitive tasks, AI allows teachers to dedicate more time to individualized instruction and foster stronger student-teacher relationships.
- Time-Saving: Automating routine administrative tasks frees up valuable time for more engaging and interactive teaching methods.
- Enhanced Accuracy: AI tools reduce human errors in grading and record-keeping, ensuring a more consistent and reliable educational process.
- Personalized Learning: AI-powered platforms adapt to each student's learning pace and style, providing customized educational experiences.
Example AI Tools in Use
Tool | Function | Impact |
---|---|---|
Grammarly | Automated writing feedback | Improves writing quality and saves grading time |
Google Classroom | Assignment distribution and grading | Streamlines assignment management and enhances communication |
Kahoot! | Interactive quizzes and assessments | Engages students and provides instant feedback |