Learning Analytics in a Nutshell
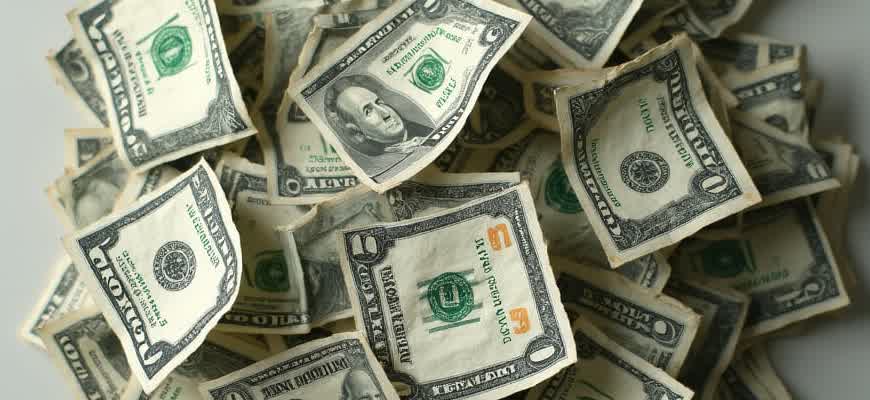
Learning analytics involves the collection, analysis, and interpretation of data related to students' learning processes and outcomes. By leveraging this data, educators can improve learning experiences, predict academic performance, and identify areas for improvement.
Key components of learning analytics:
- Data Collection: Gathering information from various educational tools and platforms.
- Data Analysis: Using statistical methods to process and extract meaningful patterns.
- Data Interpretation: Translating data insights into actionable strategies for both students and educators.
Common Benefits:
- Personalized Learning: Tailoring education to individual student needs.
- Performance Tracking: Monitoring progress over time for early intervention.
- Informed Decision Making: Empowering educators with data-driven strategies.
“Learning analytics is not just about numbers; it’s about understanding the story behind the data to improve student outcomes.”
Example of Learning Analytics in Action:
Data Type | Purpose | Outcome |
---|---|---|
Online Activity Logs | Assess student engagement with course material | Identify students who may need additional support |
Test Scores | Track academic performance | Pinpoint areas of struggle for targeted interventions |
Enhancing Student Engagement through Real-Time Learning Analytics
Real-time data monitoring and analysis significantly boost student involvement in the learning process. By tracking individual progress, engagement metrics, and participation rates, instructors can identify struggling students before they fall behind. This instant feedback loop enables quick intervention, offering targeted support that aligns with the student's needs.
Through continuous analysis of engagement levels, both educators and learners gain a clearer understanding of the learning environment. Analytics allow teachers to adapt their methods dynamically, ensuring that instructional strategies remain effective throughout the course. The focus is not only on content delivery but also on fostering active participation and collaboration within the learning community.
Key Benefits of Real-Time Analytics in Student Engagement
- Instant Feedback: Allows immediate responses to student performance, ensuring timely assistance.
- Identifying At-Risk Students: Real-time data helps spot learners who may be disengaged or struggling with the material.
- Personalized Learning Paths: Analytics provide the ability to create custom learning experiences tailored to individual needs.
- Improved Course Design: Data-driven insights help instructors refine teaching methods to keep students motivated.
Real-Time Engagement Tracking Methods
- Participation Metrics: Measures student interaction during live sessions or online forums.
- Time Spent on Activities: Tracks how long a student spends on specific tasks or materials.
- Response Time: Assesses how quickly students engage with assignments or questions posed by instructors.
"Learning analytics not only provides instructors with valuable insights into student behavior but also creates a more responsive and personalized learning experience."
Example of Engagement Metrics
Metric | Goal | Real-Time Impact |
---|---|---|
Participation Rate | Increase student interaction in class | Enables quick adjustments in teaching methods to enhance interaction |
Time Spent on Task | Ensure students are actively engaged with the material | Identifies disengagement patterns and allows for intervention |
Response Time | Improve student response and feedback cycles | Promotes quicker reactions to academic difficulties, preventing learning gaps |
Setting Up Key Metrics for Monitoring Academic Performance
To effectively track and improve student learning, it is essential to define precise metrics that align with desired academic outcomes. These metrics should reflect both students' mastery of content and their level of engagement with the learning process. By selecting the right indicators, educators can monitor performance in real time, allowing for timely interventions and adjustments to improve overall student success.
Establishing robust data collection methods enables instructors to gather valuable insights. These insights help to identify patterns of success and areas requiring further attention, ensuring a balanced and targeted approach to student performance management.
Key Metrics for Academic Monitoring
- Test and Quiz Scores: These provide a clear picture of a student's understanding of specific content, highlighting areas of strength and weakness.
- Assignment Completion Rates: A measure of how many students are submitting their work on time, offering insight into both performance and engagement.
- Discussion Participation: Reflects how actively students are participating in class discussions, both in physical and virtual spaces.
- Attendance and Engagement: Tracks how regularly students attend class and engage with online learning platforms.
Methods for Data Collection
- Learning Management System (LMS) Analytics: These provide a detailed breakdown of students' activities, including quiz scores, submission patterns, and engagement levels.
- Direct Feedback: Regular surveys and polls can help gather subjective insights into student satisfaction and understanding.
- Frequent Assessments: Implementing quizzes and assignments throughout the course allows instructors to track progress and identify areas of concern early.
By combining both performance-based and engagement metrics, instructors can gain a comprehensive understanding of student learning, enabling them to make data-driven decisions that support individual and group success.
Sample Metrics Overview
Metric | Purpose | Data Source | ||||||||||||||||||||||||||||||||||||||||||||||||||||||||
---|---|---|---|---|---|---|---|---|---|---|---|---|---|---|---|---|---|---|---|---|---|---|---|---|---|---|---|---|---|---|---|---|---|---|---|---|---|---|---|---|---|---|---|---|---|---|---|---|---|---|---|---|---|---|---|---|---|---|
Midterm Exam Scores | Measures the student's comprehension of course materials up to that point. | LMS Gradebook | ||||||||||||||||||||||||||||||||||||||||||||||||||||||||
Participation in Online Discussions | Tracks active involvement in course discussions, reflecting engagement with course material. | LMS Activity Log | ||||||||||||||||||||||||||||||||||||||||||||||||||||||||
Assignment Timeliness | Assesses how well students manage deadlines and the consistency of their work submission. | LMS Submission Tracker |
Pattern Type | Visualization Tool | Insight Gained |
---|---|---|
Engagement | Heatmaps | Identifies periods of high or low activity |
Performance Tracking | Time Series Graphs | Tracks student progress over time |
Collaboration | Pie Charts | Analyzes group contributions and interactions |
Integrating Learning Analytics with Existing LMS Platforms
As educational institutions continue to evolve, integrating data-driven insights into Learning Management Systems (LMS) has become increasingly essential for enhancing student outcomes. By incorporating learning analytics into LMS platforms, educators and administrators can gain a deeper understanding of student engagement, performance, and overall progress. This integration allows for a more personalized learning experience, improving the ability to identify at-risk students and offering targeted interventions at the right moment.
Integrating analytics tools with LMS platforms is not just about data collection but also about transforming the learning process. Such integration enables real-time tracking, feedback, and insights into how learners are interacting with course content, which can directly influence the development of teaching strategies. Furthermore, it helps institutions make data-informed decisions about curriculum improvements, resource allocation, and student support services.
Key Benefits of Integration
- Improved Decision Making: Real-time data insights allow educators to make immediate adjustments to teaching strategies, course materials, and assessments.
- Enhanced Student Support: Early identification of students who are struggling allows for timely interventions, personalized support, and improved academic performance.
- Data-Driven Curriculum Design: Understanding learner behavior and engagement patterns can help redesign courses to be more effective and engaging.
Challenges in Integration
Integrating learning analytics with LMS platforms often presents challenges such as data privacy concerns, the need for advanced technological infrastructure, and the training of staff to use the analytics tools effectively. Overcoming these challenges requires collaboration between IT, faculty, and administrators.
Steps for Successful Integration
- Identify Key Metrics: Determine which student data is most useful for your learning objectives, such as engagement rates, quiz performance, or assignment completion.
- Select the Right Analytics Tools: Choose analytics solutions that seamlessly integrate with your existing LMS and support your data collection goals.
- Train Faculty and Staff: Ensure that educators and administrators understand how to use the analytics tools to interpret data and make informed decisions.
- Ensure Data Privacy and Security: Make sure that student data is protected according to institutional policies and regulatory requirements.
Examples of Integration
Analytics Tool | Functionality | LMS Compatibility |
---|---|---|
Google Analytics | Track student interactions, engagement rates, and progress | Compatible with platforms like Moodle, Blackboard |
Learning Locker | Data collection and analysis for learning pathways | Works with Canvas, Moodle |
Tableau | Visualize and report on learning data | Integrates with a variety of LMS platforms |
Practical Steps for Analyzing and Interpreting Student Data
Effective analysis and interpretation of student data are essential for understanding learning patterns and making data-driven decisions. The process involves identifying key indicators, gathering relevant data, and applying appropriate methods for analysis. Teachers and administrators need to be strategic in utilizing various tools to extract insights that can guide improvements in teaching and learning outcomes.
By following a structured approach, educators can transform raw data into actionable insights. This allows them to monitor student progress, identify gaps, and implement targeted interventions to enhance the learning experience.
Steps for Analyzing Student Data
- Data Collection: Gather comprehensive student data, including assessments, attendance records, and participation metrics.
- Data Cleaning: Ensure data accuracy by removing duplicates, correcting errors, and filling in missing values.
- Data Segmentation: Segment students based on criteria such as performance, demographics, or behavior.
- Analysis Methods: Apply statistical tools, such as regression analysis, to identify trends and correlations within the data.
Key Interpretation Techniques
- Trend Analysis: Identify patterns over time to track student progress and detect areas of improvement or decline.
- Comparative Analysis: Compare data across different groups to assess the effectiveness of instructional methods.
- Predictive Modeling: Use historical data to predict future student outcomes, allowing for proactive intervention strategies.
Understanding the nuances of student data requires a blend of both qualitative and quantitative approaches to create a full picture of student performance and behavior.
Example of Data Analysis
Metric | Average Score | Improvement Rate |
---|---|---|
Math Test | 85% | +5% |
Reading Comprehension | 90% | +8% |
Using Predictive Models to Anticipate Academic Performance
In modern educational environments, forecasting students' future performance is increasingly dependent on sophisticated analytics tools. By leveraging historical data, instructors and administrators can identify at-risk students early in the semester. These predictive models utilize a variety of factors, such as past grades, attendance, and even participation levels, to build accurate predictions about a student's likelihood of success. The use of predictive analytics allows educational institutions to implement targeted interventions before issues become critical.
One of the key advantages of using predictive models is their ability to personalize learning paths. With insights gained from data analysis, teachers can offer customized support, ensuring that students receive the resources and encouragement they need. By focusing on students most likely to struggle, institutions can improve retention rates and overall academic achievement, making learning more efficient and effective for all involved.
Key Factors in Predictive Models
- Academic history – Previous performance, including grades and test scores, plays a major role in predicting future success.
- Engagement metrics – Tracking participation in class discussions, group work, and online platforms can offer clues about a student's commitment.
- Behavioral data – Regularity of attendance and submission of assignments helps to gauge a student's work ethic and potential for success.
- External factors – Personal circumstances or financial difficulties may also influence a student’s performance and can be factored into predictions.
Predictive Analytics Process
- Data Collection: Gathering relevant student data from various sources, such as academic records, attendance logs, and engagement platforms.
- Model Development: Applying statistical algorithms to detect patterns and create a forecast model based on past trends.
- Risk Assessment: Analyzing the outputs to classify students into categories based on their likelihood of success or failure.
- Targeted Interventions: Using the predictions to implement focused support for students at risk, such as tutoring or counseling.
"Predictive analytics empowers educators to act proactively, rather than reactively, offering students the best possible chances of success before problems arise."
Example of a Predictive Model
Factor | Weight | Impact on Success |
---|---|---|
Past Academic Performance | High | Strong predictor of future grades and performance. |
Class Participation | Medium | Indicates engagement and likely retention of course material. |
Attendance Rate | High | Consistent attendance is linked to better academic outcomes. |
Assignment Timeliness | Medium | Reflects a student’s organizational skills and discipline. |
Privacy and Ethical Considerations in Learning Analytics
As the integration of learning analytics continues to grow, ensuring that the data collected is handled responsibly is essential. The ability to track and analyze students' activities can provide valuable insights for improving educational outcomes, but it also raises significant concerns regarding privacy and ethical practices. Without proper safeguards, personal information can be exposed, leading to risks of misuse, discrimination, or manipulation of students' behaviors and decisions.
Ethical considerations in learning analytics primarily revolve around how data is collected, stored, and used. Transparent practices, informed consent, and clear data governance policies must be in place to protect students' rights and foster trust. There is also the need to ensure that learning analytics do not inadvertently reinforce biases or inequalities by being applied in ways that favor certain groups over others.
Key Ethical and Privacy Issues
- Data Consent: Students should have a clear understanding of what data is being collected, how it will be used, and who will have access to it.
- Data Security: Robust security measures are essential to prevent unauthorized access and protect sensitive information.
- Bias and Discrimination: Analytics tools should be designed and applied in ways that avoid reinforcing existing biases, whether racial, socioeconomic, or otherwise.
- Transparency: Institutions should be open about their data collection practices and how insights derived from analytics are used.
"Ethical issues in learning analytics require a balance between the benefits of personalized education and the protection of students' rights."
Best Practices in Handling Student Data
- Obtain informed consent: Ensure that students are aware of the data being collected and have the option to consent to its use.
- Limit data retention: Only retain data for as long as necessary for the intended purpose and delete it when it is no longer required.
- Ensure anonymization: Where possible, anonymize or de-identify student data to minimize privacy risks.
- Establish data governance frameworks: Create clear policies for managing, accessing, and sharing data across different stakeholders.
Privacy Risks and Mitigation Strategies
Risk | Mitigation Strategy |
---|---|
Data Breaches | Implement advanced encryption methods and secure data storage systems. |
Misuse of Data | Establish strict guidelines for data access and usage, including oversight and accountability measures. |
Bias in Analytics | Regularly audit and update algorithms to ensure fairness and equity in predictions and insights. |