Ai-supported Learning Analytics
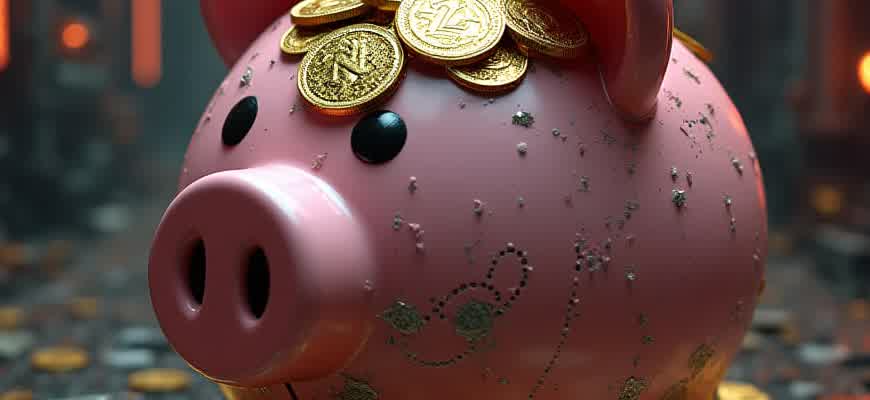
Artificial intelligence (AI) has transformed the field of educational data analysis by offering more sophisticated tools to track and improve student performance. These AI-driven systems collect vast amounts of learning data and utilize algorithms to identify patterns, providing deeper insights into student behavior, engagement, and overall learning outcomes.
With AI-supported learning analytics, educational institutions can:
- Personalize learning experiences based on individual progress and challenges.
- Detect early signs of academic struggles and intervene proactively.
- Optimize resource allocation based on data-driven insights.
Moreover, AI enables real-time feedback, helping both educators and students identify areas for improvement quickly and efficiently.
"AI technologies in education are not just about automating processes, but about enhancing the learning experience by providing actionable insights that foster personalized growth."
Key components of AI-based learning analytics include:
Component | Description |
---|---|
Data Collection | Continuous tracking of student activities, grades, and interactions within learning platforms. |
Predictive Analytics | Using historical data to forecast student outcomes and identify potential issues before they arise. |
Personalization | Adapting the learning environment to suit individual student needs based on analytics. |
AI-Enhanced Learning Analytics: A Practical Guide
Artificial Intelligence has revolutionized various industries, and education is no exception. By incorporating AI into learning analytics, educators and institutions can gain valuable insights into student performance, personalize learning paths, and optimize teaching strategies. This guide provides a comprehensive overview of how AI tools can be effectively implemented to enhance learning outcomes.
Learning analytics refers to the measurement, collection, analysis, and reporting of data about learners and their contexts. When augmented with AI, this process becomes more efficient, offering data-driven insights and predictive models that can significantly improve educational practices.
Key Benefits of AI in Learning Analytics
- Personalization: AI helps create tailored learning experiences based on individual student data.
- Predictive Insights: AI can predict student performance, enabling early intervention for at-risk learners.
- Real-Time Feedback: Students and educators receive immediate, actionable feedback to improve outcomes.
How AI Supports Learning Analytics
- Data Collection: AI tools automate the gathering of large-scale data, reducing manual work and increasing accuracy.
- Pattern Recognition: Machine learning algorithms analyze historical and real-time data to uncover patterns and trends in student behavior.
- Decision-Making: Based on data insights, AI can recommend actionable steps for instructors and administrators to improve learning outcomes.
"The power of AI lies in its ability to process vast amounts of data quickly, providing educators with the information needed to enhance the learning experience in real-time."
Implementation Strategies
Step | Action |
---|---|
1 | Identify learning objectives and determine what data is relevant for analysis. |
2 | Integrate AI tools into existing educational platforms. |
3 | Monitor the effectiveness of AI-driven analytics through continuous evaluation and adjustments. |
How AI Can Tailor Learning Experiences Based on Student Behavior
Artificial intelligence has the potential to revolutionize how we approach education by creating individualized learning pathways based on real-time data from student interactions. By monitoring how students engage with content, AI can adapt lessons to meet their specific needs, ensuring they stay challenged yet supported throughout their learning journey.
AI-driven systems analyze various metrics, including time spent on tasks, error patterns, and content preferences. This data allows for the dynamic adjustment of learning materials, providing more personalized experiences. AI can identify the learning style of each student, whether they require more visual aids, interactive exercises, or simplified explanations, ensuring that no student is left behind.
Key Behaviors AI Analyzes for Personalization
- Engagement Levels: How actively a student interacts with the content.
- Response Accuracy: The rate at which a student answers questions correctly, highlighting strengths and weaknesses.
- Time Management: Patterns in how long a student spends on various tasks, helping to identify struggles with specific topics.
- Content Preferences: Which types of resources (videos, quizzes, readings) a student engages with the most.
How AI Customizes Learning Paths
- Adjusts content complexity: Based on past performance, the system increases or decreases the difficulty level of exercises.
- Suggests additional resources: If a student struggles with a particular topic, AI suggests supplementary materials for better understanding.
- Modifies pacing: The system can slow down or speed up lessons to match a student's learning tempo, ensuring optimal engagement.
Important: AI systems continuously track and adapt based on student progress, ensuring that learning remains both challenging and achievable at every stage.
Example of AI Personalization in Action
Student Behavior | AI Response |
---|---|
Frequent incorrect answers in math problems | AI offers simpler exercises and more interactive tutorials to strengthen fundamental concepts. |
Extended time spent on reading comprehension tasks | AI suggests visual aids or alternative formats like audio books to enhance understanding. |
High engagement with interactive content | AI provides more gamified experiences and simulation-based exercises for deeper learning. |
Leveraging Real-Time Data for Enhanced Learning Outcomes
In today's digital learning environments, the ability to capture and analyze student data in real-time offers a transformative approach to improving educational results. By utilizing advanced analytics, educators can gain immediate insights into student progress and make data-driven decisions to personalize learning experiences. This capability helps to identify learning gaps early, providing opportunities for timely intervention and tailored support.
The integration of AI-supported tools allows for dynamic adjustments to teaching methods, ensuring that every student’s needs are met based on their individual performance. These insights can range from assessing engagement levels to understanding comprehension patterns, all of which directly influence instructional strategies. Through this continuous feedback loop, educators are equipped to foster more effective learning environments.
Key Benefits of Real-Time Data Insights in Education
- Immediate Interventions: Teachers can identify students struggling with specific concepts and offer targeted support before gaps widen.
- Personalized Learning: AI tools help tailor content to each student's learning pace, enhancing engagement and retention.
- Data-Driven Instruction: Real-time analytics enable instructors to adjust their teaching methods based on current student performance and engagement.
- Optimized Student Performance: Continuous monitoring allows educators to track progress and adjust strategies to ensure optimal learning outcomes.
How Data Insights Drive Educational Adjustments
One of the most powerful aspects of AI-supported learning analytics is its ability to drive immediate changes in instructional methods. Through constant feedback, instructors can make real-time adjustments to their teaching practices. This dynamic approach significantly improves the learning experience and encourages better outcomes.
“The integration of real-time analytics provides a clear view of student performance, which not only highlights challenges but also enables quick, actionable responses to improve learning outcomes.”
Examples of Real-Time Analytics in Action
Analytics Type | Insight Provided | Actionable Response |
---|---|---|
Engagement Levels | Identifies students who are disengaged or distracted. | Teachers can initiate interactive activities to recapture attention. |
Assessment Scores | Shows areas where students are consistently underperforming. | Targeted review sessions or tutoring can be provided. |
Learning Pace | Reveals if students are moving too quickly or slowly through material. | Adjustments can be made to pace or complexity of the content. |
Integrating Learning Analytics with Classroom Tools for Seamless Monitoring
To effectively track student progress and performance, integrating learning analytics with classroom tools becomes essential. By embedding analytic functions into everyday teaching platforms, educators can make data-driven decisions in real time, enhancing the learning experience for both students and instructors. This seamless integration allows for proactive intervention, personalized learning, and better resource allocation, ultimately improving educational outcomes.
Modern learning environments utilize a variety of tools ranging from Learning Management Systems (LMS) to digital collaboration platforms. By connecting these tools with robust analytics, educators can monitor and assess student engagement, track learning patterns, and identify areas that need attention. These integrations can work behind the scenes to provide actionable insights without disrupting the flow of the classroom.
Key Benefits of Integration
- Real-time data collection: Continuous monitoring allows teachers to adjust instructional strategies instantly based on student needs.
- Personalized learning: Data insights help tailor content and activities to individual student preferences, improving engagement.
- Early intervention: Predictive analytics can flag struggling students, enabling timely support and guidance.
How It Works
- Data Integration: Classroom tools automatically feed data into an analytics platform, such as participation rates, quiz results, or time spent on assignments.
- Analysis and Feedback: The analytics engine processes the data to generate actionable insights, which are presented to teachers in easy-to-understand reports.
- Actionable Outcomes: Teachers use these insights to adapt their lessons, suggest additional resources, or provide individual support where necessary.
"The integration of learning analytics into classroom tools has transformed the role of educators from merely instructors to data-informed facilitators of learning."
Example of Integrated Tools
Tool | Purpose | Analytics Features |
---|---|---|
LMS (Learning Management System) | Content delivery and tracking | Engagement metrics, performance tracking |
Collaborative Platforms | Group work and discussion forums | Participation levels, peer interactions |
Assessment Tools | Testing and quizzes | Result analysis, progress tracking |
Predicting Student Performance: Leveraging AI to Identify Students at Risk
In the modern educational landscape, AI-supported analytics have become essential in helping educators identify students who may be struggling academically. By analyzing vast amounts of data, AI systems can predict potential academic failures and provide early intervention opportunities. This proactive approach not only enhances student support but also allows for more personalized learning paths. Leveraging AI tools, educational institutions can pinpoint students who are at risk of falling behind, ensuring that timely assistance can be provided to improve their chances of success.
Artificial intelligence models work by analyzing a combination of historical performance data, attendance records, participation levels, and behavioral patterns to predict students’ future outcomes. The key benefit of such systems is the ability to detect subtle trends that may otherwise go unnoticed by human instructors. This allows for early identification of students who need additional resources, such as tutoring, mentorship, or other personalized interventions, before issues become severe.
Key Features of AI-Based Early Warning Systems
- Data Integration: AI systems can integrate diverse data points, such as grades, assignment completion rates, and engagement in class activities.
- Predictive Models: Algorithms can forecast academic performance based on historical trends, identifying patterns that suggest potential risks.
- Real-Time Alerts: Faculty and staff receive immediate notifications when a student shows signs of struggling, enabling prompt intervention.
AI can enhance the accuracy of predictions by considering a variety of data inputs that are often overlooked in traditional evaluation methods. This creates a comprehensive and more precise view of student performance.
Methods for Identifying At-Risk Learners
- Monitoring Engagement: Tracking student interactions with online platforms, participation in discussions, and submission of assignments can indicate low engagement, which correlates with poorer academic outcomes.
- Analysis of Past Performance: Students with a history of low grades or inconsistent progress are more likely to face difficulties in the future, allowing AI to forecast potential risks.
- Behavioral Indicators: AI can also detect changes in student behavior, such as increased absenteeism or lack of communication, which are strong indicators of academic struggles.
Predicting Student Outcomes with AI: A Comparative Overview
Method | Strength | Limitation |
---|---|---|
Predictive Analytics | Accurately forecasts future performance based on historical data | May not account for external factors like personal circumstances |
Behavioral Analysis | Tracks non-academic factors like engagement and participation | Can be affected by incomplete data or atypical student behavior |
Engagement Tracking | Provides real-time insights into student activity and involvement | May not reflect deeper learning issues not observable through engagement |
Streamlining Administrative Decisions with AI-Driven Data Visualization
AI-powered data visualization tools have revolutionized administrative decision-making in educational institutions. By translating complex data sets into visual formats such as dashboards, heatmaps, and interactive charts, AI allows administrators to quickly grasp trends and insights that would otherwise take hours to analyze manually. This streamlining of data enables faster, more accurate decision-making, reducing human error and improving overall efficiency in management processes.
Moreover, AI-driven systems provide real-time insights that can be tailored to specific needs of administrators. Whether it's monitoring student performance, identifying at-risk learners, or evaluating resource allocation, data visualization enhances the ability to make informed decisions that align with institutional goals. With these tools, decision-makers are no longer overwhelmed by raw data but are empowered to act on the insights provided.
Key Benefits of AI in Administrative Data Visualization
- Real-Time Analysis: Provides up-to-the-minute data, allowing for swift interventions and timely decision-making.
- Customizable Dashboards: Offers tailored views based on roles, making it easier for staff to access relevant information.
- Enhanced Accuracy: AI algorithms reduce the likelihood of errors in data interpretation and presentation.
- Predictive Insights: Identifies trends and makes forecasts, aiding proactive planning and decision-making.
Data-Driven Decision Framework
By utilizing AI, administrative decision-making processes become more systematic and data-driven. Below is a framework that highlights how AI visualization supports key areas in education management:
Area | AI Visualization Role | Outcome |
---|---|---|
Student Performance | AI tracks academic progress and identifies areas requiring intervention. | Faster identification of struggling students, enabling targeted support. |
Resource Allocation | AI visualizes resource distribution across departments and highlights inefficiencies. | Optimized use of resources, ensuring more effective allocation. |
Faculty Management | AI analyzes workload distribution and faculty performance metrics. | Improved faculty scheduling and workload balancing. |
“With AI-powered visualization, administrators can access actionable insights instantly, leading to decisions that are both timely and based on data-driven evidence.”
Conclusion
The integration of AI-driven data visualization is transforming how administrative decisions are made in educational settings. By converting complex data into digestible visual formats, institutions can enhance operational efficiency, improve responsiveness, and make decisions that are both informed and impactful. As these technologies continue to evolve, their role in educational management will only become more crucial.
Optimizing Curriculum Design with AI-Based Learning Pattern Analysis
AI-based learning pattern analysis offers a transformative approach to curriculum development. By examining students' behavior, progress, and engagement through advanced algorithms, AI can uncover hidden learning patterns that were previously difficult to detect. This allows educators to make data-driven decisions for improving the curriculum structure, enhancing student learning experiences, and addressing gaps in educational content.
AI’s ability to track and analyze vast amounts of student data in real-time empowers educational institutions to create more personalized and effective learning pathways. By identifying strengths and weaknesses in students' comprehension and participation, educators can adjust course materials to better align with learners' needs, ensuring more efficient learning outcomes.
Key Benefits of AI-Driven Curriculum Optimization
- Personalized Learning Paths: AI analyzes individual progress, suggesting tailored materials for each student based on their strengths and weaknesses.
- Real-Time Feedback: With AI monitoring student behavior, immediate adjustments can be made to teaching strategies or content delivery.
- Identifying Knowledge Gaps: AI helps detect common areas where students struggle, allowing for the targeted revision of curriculum content.
- Efficient Resource Allocation: AI optimizes the distribution of teaching resources, ensuring that educators can focus on areas where students need the most support.
Steps in Implementing AI for Curriculum Design Enhancement
- Data Collection: Collect comprehensive data on student interactions with the course content, including time spent on tasks, assessment results, and participation levels.
- Pattern Detection: Use machine learning algorithms to identify learning trends, common challenges, and areas where students excel.
- Curriculum Refinement: Adjust the curriculum by modifying content, pacing, and assessment strategies based on insights gained from AI analysis.
- Continuous Monitoring: Keep track of student progress and continuously refine the curriculum using new data collected over time.
AI-based learning analytics helps institutions continuously evolve their curriculum to meet changing educational needs, ensuring better learning outcomes and a more adaptive teaching environment.
Example of AI-Driven Curriculum Adjustment
Student Group | Initial Performance | Suggested Curriculum Adjustment |
---|---|---|
Group A | High engagement, low retention in final assessments | Increase interactive review sessions and adaptive quizzes before assessments. |
Group B | Consistent struggle with specific concepts | Introduce supplemental materials and videos focused on those concepts. |
Group C | Average performance with steady improvement | Maintain current pace and introduce more advanced exercises for further enrichment. |
Leveraging AI for Automating Feedback and Evaluations in Educational Systems
The integration of AI in education is transforming the way assessments and feedback are delivered. By utilizing machine learning algorithms, AI systems can analyze student performance in real-time and provide instant, personalized feedback. This shift significantly reduces the time educators spend on grading and allows them to focus more on individualized instruction. AI tools can assess written content, multiple-choice questions, and even complex problem-solving tasks, making the evaluation process faster and more objective.
AI-powered systems can adapt to the needs of each learner, offering tailored suggestions and resources based on their progress. This approach not only enhances learning experiences but also ensures consistent, unbiased assessments. As these technologies become more advanced, they have the potential to support diverse educational environments, from K-12 to higher education, by offering scalable solutions to grading and feedback processes.
Key Benefits of AI in Automated Feedback
- Efficiency: AI accelerates the feedback cycle, providing students with immediate insights into their performance.
- Personalization: AI systems can offer customized feedback based on individual learning patterns and needs.
- Scalability: AI tools can manage large volumes of assessments, making them ideal for institutions with large student populations.
How AI Supports Assessment Automation
- Instant Grading: AI tools are capable of automatically grading assignments, such as essays, quizzes, and tests, using predefined rubrics.
- Feedback Generation: Based on the assessment results, AI provides immediate, constructive feedback that guides students toward improvement.
- Pattern Recognition: AI can detect common mistakes or trends in student responses, allowing for more effective targeted interventions.
AI-Assisted Assessment Framework
Assessment Type | AI Role | Benefit |
---|---|---|
Multiple-Choice Questions | Automated grading and analytics | Faster evaluations and insight into common misconceptions |
Essays | Natural language processing for grading and feedback | Objective scoring and personalized feedback |
Projects | Assessment based on predefined criteria and peer review | Scalable evaluations and real-time updates |
AI not only helps to reduce the workload of educators but also empowers students to track their own progress more effectively, creating a dynamic learning environment that adapts to the needs of each individual.