Data Driven Assessments
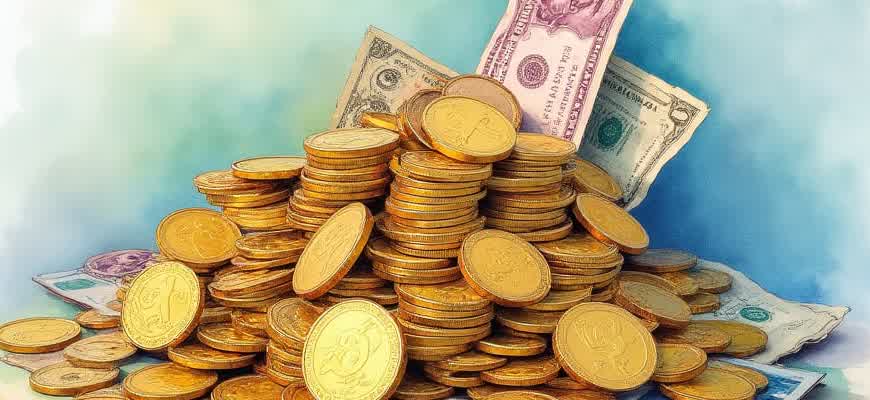
Data-driven assessments have become a fundamental approach for evaluating performance across various sectors. These methods rely on quantitative data to provide an objective analysis of outcomes and trends, minimizing bias and offering more accurate insights into effectiveness.
Key features of data-driven evaluations include:
- Systematic collection of relevant data
- Continuous monitoring and adjustment of evaluation criteria
- Use of advanced analytics to interpret complex datasets
These techniques are widely used in educational, corporate, and governmental assessments. The focus lies in leveraging real-time data to refine strategies and make informed decisions.
"Data-driven assessments offer a structured way to evaluate performance, ensuring that decisions are based on evidence rather than intuition."
The process typically follows these steps:
- Data collection: Gathering relevant metrics from diverse sources.
- Analysis: Applying statistical models and algorithms to understand patterns.
- Action: Implementing changes based on the insights gained from the analysis.
In practice, this approach can be visualized in a data summary table:
Metric | Current Value | Target Value |
---|---|---|
Customer Satisfaction | 85% | 90% |
Revenue Growth | 7% | 10% |
Incorporating Data Analytics into Your Evaluation Framework
Integrating data analytics into your assessment process allows for a more objective and effective evaluation of performance. By leveraging data, organizations can make informed decisions, identify trends, and predict future outcomes. This approach moves away from traditional subjective assessments, offering insights that are grounded in measurable evidence.
To successfully incorporate data analytics, it's essential to align your assessment criteria with the data you're collecting. This ensures that the metrics used for evaluation are relevant and actionable, providing real-time feedback that enhances the overall assessment process.
Steps to Integrate Analytics into Assessments
- Define Clear Objectives: Ensure the assessment goals are well-aligned with business outcomes. These objectives should guide the selection of relevant data points.
- Collect Comprehensive Data: Gather data from multiple sources such as surveys, employee performance records, and engagement metrics to provide a well-rounded view of the situation.
- Implement Tools for Analysis: Use advanced analytics tools to process and interpret data. Software like Tableau, Power BI, or custom-built dashboards can provide deeper insights.
- Utilize Predictive Analytics: Leverage predictive models to forecast potential outcomes based on current data, helping to identify trends and make proactive decisions.
Best Practices for Data-Driven Assessments
- Ensure Transparency: Communicate how data is being used and the methods for analysis. Transparency fosters trust and ensures stakeholders understand the assessment process.
- Refine Metrics Continuously: Regularly review and refine the data points you’re tracking to ensure they remain relevant to evolving objectives.
- Act on Insights: It’s essential to not only gather and analyze data but to also implement changes based on the findings. This ensures continuous improvement and value from the process.
"Data analytics provides a solid foundation for decision-making, ensuring that assessments are not based on assumptions, but on concrete evidence."
Example Data Analytics Framework
Stage | Action | Expected Outcome |
---|---|---|
Data Collection | Gather performance, engagement, and feedback data | Comprehensive data set for analysis |
Data Analysis | Analyze trends, patterns, and correlations | Identification of key performance drivers |
Actionable Insights | Implement changes based on findings | Improved performance and alignment with goals |
Choosing the Right Metrics for Data-Driven Evaluations
When assessing performance or outcomes based on data, selecting the correct metrics is crucial for obtaining accurate and actionable insights. Metrics serve as the foundation for decision-making and enable organizations to align their evaluation process with specific goals. An improper choice of metrics can lead to misleading conclusions, misdirected efforts, and inefficiencies in strategy development. Therefore, understanding the context and objectives of the evaluation is essential before determining which indicators to track.
To ensure that the right metrics are chosen, it's important to consider a few guiding principles. The selected metrics should be aligned with the specific goals of the evaluation and should provide measurable, relevant, and timely insights. Below are several steps to follow when choosing the most suitable metrics for data-driven evaluations:
Key Considerations in Metric Selection
- Relevance: The metric must directly relate to the objective of the evaluation.
- Actionability: Metrics should be actionable, meaning they should provide insights that lead to specific, actionable recommendations.
- Measurability: Metrics must be quantifiable and collected in a way that can be accurately tracked over time.
- Timeliness: Choose metrics that provide insights in real-time or within an appropriate timeframe to drive decision-making.
Common Metric Categories
- Efficiency Metrics: Track how well resources (time, budget, personnel) are utilized.
- Effectiveness Metrics: Measure the degree to which objectives are achieved.
- Quality Metrics: Evaluate the quality of outcomes, products, or services delivered.
- Impact Metrics: Assess the broader effects or outcomes of an initiative over time.
Example of a Metric Framework
Metric Category | Example Metrics | Use Case |
---|---|---|
Efficiency | Cost per Acquisition, Time to Market | Evaluating operational processes to optimize resource allocation. |
Effectiveness | Conversion Rate, Customer Satisfaction Score | Measuring the success of a marketing campaign or product launch. |
Quality | Error Rate, Customer Complaints | Monitoring product quality and user experience post-launch. |
Impact | Long-term Customer Retention, Market Share Growth | Assessing the long-term success and sustainability of a business initiative. |
“The right metrics don’t just measure performance; they guide strategy, identify opportunities for improvement, and predict future outcomes.”
Key Tools and Technologies for Implementing Data Assessments
To effectively implement data-driven evaluations, leveraging the right tools and technologies is essential. These tools allow organizations to collect, analyze, and interpret data with precision, ensuring that the assessment process remains accurate and efficient. From data collection platforms to advanced analytics software, the right technology stack enables more informed decision-making and insight generation. Below are some of the key solutions and methods used in the process.
Modern data assessment requires a combination of technologies that work together to streamline the collection, processing, and visualization of information. These technologies not only automate the evaluation process but also provide actionable insights that can drive improvements. The most important tools include data analytics platforms, data visualization software, and specialized algorithms that allow for predictive and prescriptive analytics.
Essential Tools for Data Assessments
- Data Analytics Platforms: These tools process large volumes of data to uncover patterns and trends. They include platforms such as Tableau, Power BI, and Google Data Studio.
- Data Collection Systems: Tools like SurveyMonkey, Qualtrics, and custom APIs are essential for gathering relevant data from different sources.
- Advanced Statistical Software: For deeper analysis, statistical tools like R, Python, and SAS are indispensable, especially in predictive and prescriptive analytics.
Technologies Supporting Data Evaluation
- Machine Learning Algorithms: These technologies are used for automated decision-making and prediction based on historical data, improving the precision of assessments.
- Cloud Computing: Cloud-based platforms such as AWS and Google Cloud offer scalable resources for storing and analyzing large datasets, providing flexibility for data-driven assessments.
- Business Intelligence Tools: BI tools like Qlik and Looker help organizations visualize complex data, making it easier to interpret and act upon findings.
Effective data-driven assessments rely heavily on the integration of various technologies. Combining these tools ensures that the data collection process is efficient, the analysis is accurate, and the results are actionable.
Comparison of Tools
Tool | Use Case | Example Platforms |
---|---|---|
Data Collection | Gathering data from users, surveys, or external systems | SurveyMonkey, Qualtrics |
Data Analytics | Analyzing and finding patterns in datasets | Tableau, Power BI |
Statistical Software | Performing advanced data analysis and statistical modeling | R, Python, SAS |
Machine Learning | Automating prediction and decision-making | TensorFlow, Scikit-learn |
Analyzing Patterns: Understanding Data Trends in Assessments
Identifying patterns within assessment data is crucial for deriving meaningful insights that drive educational improvements. These patterns help to uncover areas of strength and weakness among students, allowing educators to make informed decisions based on empirical evidence rather than intuition. The process of analyzing trends in assessment results often requires the application of statistical methods and data visualization techniques to ensure clarity and accuracy in interpreting the outcomes.
By recognizing recurring trends in assessment data, schools and institutions can optimize teaching strategies, adjust curricula, and provide personalized support to students. However, this process goes beyond simply recognizing numbers and scores; it involves understanding the underlying factors that contribute to these patterns, whether they be instructional methods, student engagement levels, or environmental influences.
Key Factors in Data Trend Analysis
- Performance Variability: Identifying fluctuations in student scores over time can highlight issues such as inconsistent teaching methods or external disruptions.
- Demographic Impact: Analyzing trends across different student demographics, such as age, gender, or socio-economic status, reveals how these factors influence academic outcomes.
- Curriculum Effectiveness: Trends in assessment scores before and after curriculum changes can provide insight into the effectiveness of new teaching approaches or materials.
Steps to Analyzing Data Trends
- Data Collection: Gather relevant assessment data from various sources, ensuring consistency and accuracy across all datasets.
- Data Cleaning: Eliminate outliers and errors in the data to ensure the reliability of the analysis.
- Data Visualization: Use graphs, charts, and tables to clearly present the data, making it easier to identify significant trends.
- Pattern Identification: Look for recurring patterns or anomalies that might indicate areas requiring attention or further investigation.
Examples of Data Trends in Assessments
Assessment Type | Trend Observed | Possible Cause |
---|---|---|
Standardized Testing | Higher scores in the spring semester | Increased preparation time and focused review sessions before the test period |
Formative Assessments | Improvement in areas with targeted interventions | Implementation of personalized tutoring sessions |
"The power of data analysis lies not only in identifying trends but in using those insights to make actionable decisions that foster continuous improvement in educational practices."
Improving Decision-Making through Data-Based Insights
In today's fast-paced business environment, the ability to make informed decisions is crucial. Data-driven insights empower organizations to move beyond intuition and guesswork, allowing for more accurate and actionable decisions. By analyzing large sets of data, companies can identify trends, predict future outcomes, and optimize strategies in real time. This shift from traditional decision-making to a data-centric approach leads to better resource allocation, improved operational efficiency, and a stronger competitive advantage.
Organizations are increasingly adopting data-driven decision-making (DDDM) to enhance their strategic planning. Using tools like data visualization and predictive analytics, businesses can extract valuable insights from vast datasets. These insights provide a clearer understanding of customer behaviors, market conditions, and operational performance, which directly influences the quality of decisions at every organizational level.
Key Benefits of Data-Driven Decision Making
- Improved Accuracy: Decisions are based on concrete data, reducing the risks of human error and bias.
- Faster Decision-Making: Automated data analysis speeds up the decision-making process, enabling quicker responses to market changes.
- Cost Optimization: By analyzing operational data, businesses can identify inefficiencies and reduce unnecessary costs.
- Risk Mitigation: Predictive analytics helps foresee potential challenges, allowing for proactive risk management strategies.
Steps to Implement Data-Driven Decision Making
- Data Collection: Gather relevant data from various sources, including internal operations, customer feedback, and market trends.
- Data Analysis: Utilize advanced analytics tools to interpret the data, identify patterns, and extract meaningful insights.
- Integration: Incorporate data insights into decision-making processes at all levels of the organization.
- Review and Refine: Regularly assess the accuracy and effectiveness of decisions, and adjust strategies as needed.
"Data-driven decision making isn't just a luxury–it's a necessity for businesses to remain competitive and relevant in the modern market."
Impact of Data-Based Insights on Key Performance Indicators (KPIs)
Key Performance Indicator | Impact of Data-Based Insights |
---|---|
Customer Satisfaction | Identifying patterns in customer feedback allows for tailored services that improve satisfaction. |
Revenue Growth | Data-driven insights into market trends help uncover new revenue streams and optimize pricing strategies. |
Operational Efficiency | Data analysis highlights inefficiencies, enabling process improvements and cost reductions. |
Measuring the Impact of Data-Driven Evaluation Programs
Assessing the effectiveness of data-driven evaluation programs requires a comprehensive approach that captures both qualitative and quantitative outcomes. It’s crucial for organizations to identify clear metrics that reflect the program’s contribution to decision-making, learning processes, and organizational growth. By focusing on measurable results, stakeholders can evaluate the return on investment (ROI) and assess whether the resources allocated are delivering substantial value. The challenge lies in selecting relevant metrics and interpreting them within the broader context of the organization's goals and operations.
One key component in measuring ROI is tracking improvements in performance that can be directly attributed to insights gained from data-driven assessments. This process involves linking changes in outcomes to specific interventions informed by data analysis. Below, we outline several important factors for evaluating the ROI of such programs:
Key Factors to Consider in ROI Evaluation
- Cost Savings: Reduction in resources spent due to more targeted decision-making.
- Performance Improvement: Measurable enhancements in employee, team, or organizational performance.
- Time Efficiency: Faster decision-making processes that minimize downtime and operational delays.
“Investing in data-driven assessments should ideally lead to both tangible and intangible returns that improve overall organizational efficiency and learning outcomes.”
Common Metrics for ROI Analysis
- Cost Reduction: Monitor savings in resources, such as time, money, or human capital, due to optimized processes.
- Productivity Gains: Track improvements in task completion rates, project success, and overall productivity.
- Engagement and Retention: Evaluate how data-driven insights enhance employee or customer engagement and reduce turnover.
Example of a Data-Driven Assessment ROI Calculation
Metric | Before Implementation | After Implementation | Change |
---|---|---|---|
Employee Training Time | 50 hours | 30 hours | -40% |
Operational Costs | $100,000 | $80,000 | -20% |
Project Completion Rate | 75% | 90% | +15% |
Ensuring Data Privacy and Security in Assessment Systems
As organizations increasingly adopt data-driven solutions for evaluations, maintaining the confidentiality and protection of personal information becomes paramount. Assessment systems are responsible for handling a significant volume of sensitive data, making them a target for malicious activities. To safeguard against these threats, robust strategies must be implemented at multiple levels, ensuring that the data remains secure throughout its lifecycle. This involves both technical and organizational measures to maintain trust and compliance with legal frameworks.
Data privacy and security in assessment systems require a comprehensive approach, including encryption, access control, and continuous monitoring. Organizations must assess their infrastructure for vulnerabilities and adopt best practices for handling personal data. In this regard, security features should be considered from the design phase and remain a priority throughout the system's operation.
Key Measures to Safeguard Data
- Data Encryption: All sensitive data, both in transit and at rest, should be encrypted using the latest standards to prevent unauthorized access.
- Access Control: Implement strict access control policies to ensure that only authorized individuals can interact with sensitive information. Multi-factor authentication (MFA) is crucial for enhancing security.
- Regular Audits: Conduct regular security audits to identify potential vulnerabilities and fix them promptly.
- Compliance with Regulations: Adhere to data protection laws, such as GDPR or CCPA, to ensure legal compliance and secure data handling practices.
Data Breach Response and Mitigation
In case of a data breach, swift action is required. A well-defined incident response plan should be in place to contain the breach, mitigate its impact, and notify affected parties promptly.
- Immediate Containment: Isolate affected systems to prevent further compromise.
- Investigation: Conduct a thorough investigation to understand the scope of the breach and identify the source.
- Communication: Notify stakeholders and regulators as required by law, offering transparency on the nature and impact of the breach.
Security and Privacy Best Practices
Best Practice | Description |
---|---|
Data Masking | Mask sensitive data in non-production environments to ensure no exposure during testing or development. |
Role-Based Access Control (RBAC) | Define clear roles and responsibilities to limit user access to only the data necessary for their function. |
End-to-End Encryption | Ensure all data is encrypted at every stage, including data collection, processing, and storage. |