Ai in Higher Education Symposium
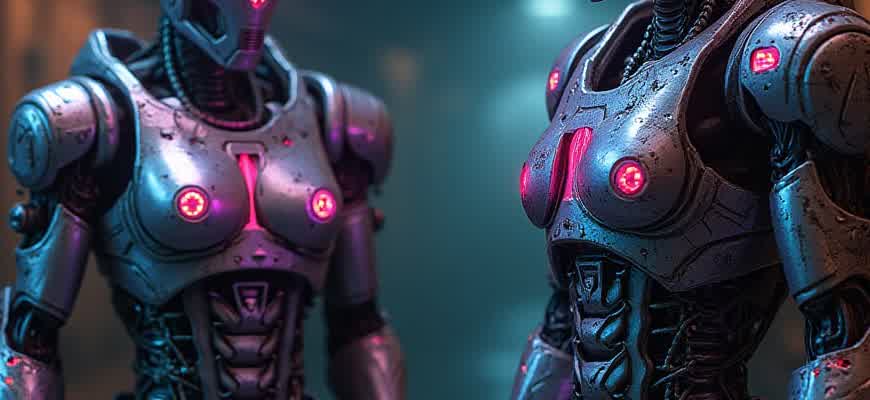
The upcoming symposium on the role of artificial intelligence in higher learning environments will explore the transformative potential of AI technologies in educational settings. This event will bring together experts, educators, and researchers to discuss AI’s evolving impact on teaching, learning, and institutional operations. Several key topics will be covered, focusing on both the opportunities and challenges AI presents within universities.
Attendees will engage in discussions on the following core themes:
- The application of AI in personalized learning experiences.
- AI-powered tools for administrative efficiency and decision-making.
- Ethical considerations and the future of AI in education.
The symposium will feature a structured series of presentations, workshops, and panel discussions. Below is a summary of the event’s agenda:
Time | Session | Speaker |
---|---|---|
09:00 - 10:00 | Keynote Address: The Role of AI in the Future of Higher Education | Dr. John Smith |
10:15 - 11:30 | Workshop: AI Tools for Faculty and Student Engagement | Prof. Emily Davis |
11:45 - 13:00 | Panel: Ethical AI in Education: Balancing Innovation and Responsibility | Various Speakers |
"AI's potential to reshape higher education is not just about technology–it's about how we rethink the way we teach, learn, and interact with knowledge." – Dr. John Smith, Keynote Speaker
How AI Can Revolutionize Teaching and Learning in Higher Education
Artificial Intelligence has the potential to reshape the educational landscape by providing personalized, data-driven learning experiences that meet the unique needs of every student. By leveraging AI tools, institutions can automate administrative tasks, enhance student engagement, and support educators in delivering more effective and tailored instruction.
The integration of AI in teaching and learning fosters a more interactive and adaptive environment. Machine learning algorithms can analyze student performance in real-time, offering educators actionable insights that help address learning gaps and customize lesson plans accordingly. This dynamic approach not only enhances the overall learning experience but also drives more efficient resource management within educational institutions.
AI-Driven Enhancements in Education
- Personalized Learning: AI enables individualized learning paths by adapting content based on students' strengths, weaknesses, and learning styles.
- Automation of Administrative Tasks: AI systems can handle grading, scheduling, and other administrative duties, freeing up time for instructors to focus on teaching.
- Improved Student Engagement: Intelligent tutoring systems and chatbots offer students instant assistance, making learning more interactive and engaging.
"AI-powered tools help create a dynamic classroom environment, where teaching is not just about delivering content, but about fostering a deeper, more personalized connection with students."
Examples of AI in Action
- Adaptive Learning Platforms: These platforms adjust the difficulty of content based on the learner’s progress, ensuring they are continuously challenged without feeling overwhelmed.
- AI Tutors and Assistants: Virtual tutors can provide additional support outside of class hours, answering questions and guiding students through complex topics.
- Predictive Analytics: AI can forecast student success and identify at-risk individuals by analyzing patterns in performance data, enabling early intervention strategies.
Key Considerations for AI Integration
Challenge | AI Solution |
---|---|
Limited Personalized Feedback | AI-driven systems can provide real-time, individualized feedback to students, addressing specific learning needs. |
Resource Allocation | AI helps optimize resource distribution, from managing class sizes to ensuring instructors’ workloads are balanced. |
Data Overload | AI algorithms can sift through large datasets and provide actionable insights to both educators and administrators. |
Practical Tools for Incorporating AI into University Programs
Integrating artificial intelligence into university curricula requires the use of various practical tools that can enhance learning outcomes and improve academic performance. Universities should embrace AI technologies that allow instructors to create dynamic, personalized learning environments. AI-powered platforms can help customize assignments and assessments based on individual student needs, providing a more tailored educational experience.
By adopting these technologies, universities can also equip students with essential skills for the future, preparing them for careers in AI-driven industries. The following tools and strategies are useful for integrating AI into academic settings effectively.
AI-Powered Tools and Platforms
- Learning Management Systems (LMS) with AI Integration: Platforms like Moodle or Canvas incorporate AI for personalized learning paths, automating tasks such as grading and feedback. These systems adjust content delivery according to student performance, ensuring engagement and progression.
- AI Tutoring Systems: Tools like Squirrel AI or Carnegie Learning use algorithms to offer personalized tutoring experiences. These systems identify knowledge gaps and adapt their approach to each student's pace and learning style.
- AI-Driven Assessment Tools: Tools like Gradescope and Turnitin employ AI to evaluate assignments, detect plagiarism, and provide instant feedback. This helps educators focus on more complex aspects of teaching, such as student interaction.
Implementation Strategies
- Curriculum Redesign: Faculties should redesign courses to incorporate AI-related content, such as machine learning algorithms, data analysis, and AI ethics. This prepares students for the growing demand in tech fields.
- Collaborative Projects: Integrating real-world AI applications into student projects allows learners to apply theoretical knowledge. Collaborative tools can facilitate group work, further reinforcing teamwork and problem-solving skills.
- Professional Development: Faculty members should undergo regular training to stay current with AI advancements, ensuring they are capable of teaching and mentoring students effectively.
Potential Benefits and Considerations
Benefit | Consideration |
---|---|
Personalized Learning: AI can provide custom-tailored learning experiences for each student. | Privacy Concerns: Collecting data on student behavior and performance must be done with strong ethical guidelines. |
Efficiency in Assessment: AI-powered tools streamline grading, allowing more time for instructional activities. | Bias in Algorithms: AI systems must be continuously monitored to ensure they are fair and free from bias. |
"Artificial intelligence can revolutionize how education is delivered, but careful attention must be paid to ethical considerations and data privacy."
AI's Impact on Tailored Student Assistance and Engagement
The introduction of AI-driven tools in higher education has significantly reshaped the landscape of student engagement and personalized support. These innovations focus on leveraging vast data pools to understand individual learning preferences, behavior patterns, and academic needs. By doing so, AI enables institutions to offer more targeted, efficient, and scalable assistance to each student, improving both academic outcomes and personal development. Personalized feedback, adaptive learning platforms, and virtual assistants are some of the key methods through which AI enhances student engagement and success rates.
AI systems can track real-time performance, assess strengths and weaknesses, and offer resources specific to each student's learning trajectory. This process not only addresses academic concerns but also fosters a more inclusive environment by catering to diverse learning styles. Below are the primary aspects in which AI contributes to personalized student engagement and support:
- Customized Learning Paths: AI can design dynamic learning experiences tailored to a student’s progress and comprehension, offering real-time adjustments based on performance.
- Behavioral Analytics: By analyzing student data, AI systems can predict challenges and suggest proactive interventions before academic issues escalate.
- Instant Access to Assistance: Virtual assistants powered by AI provide students with 24/7 support, answering questions and guiding them through academic or administrative processes.
- Engagement Monitoring: AI systems can track engagement levels, ensuring that students stay motivated and involved throughout their coursework.
In addition, AI plays a crucial role in enhancing communication between students and educators. Automated systems help identify when students may be falling behind, triggering personalized messages or even scheduling consultations with professors. The following table outlines AI’s contribution to personalized student support:
AI Feature | Impact on Students |
---|---|
Adaptive Learning Tools | Delivers content based on individual pacing and understanding, promoting better retention. |
Chatbots & Virtual Tutors | Offers 24/7 support for homework, exam preparation, and clarification of doubts. |
Learning Analytics | Identifies at-risk students and provides tailored interventions to ensure academic success. |
"AI in education is not about replacing teachers; it's about augmenting the learning process to make it more responsive to each student's unique needs."
Enhancing Academic Research with AI-Driven Solutions
Artificial intelligence (AI) is revolutionizing the landscape of academic research by offering powerful tools that enhance data processing, analysis, and collaboration. By integrating AI into research workflows, scholars can improve the speed and accuracy of their work, allowing them to focus on the creative and analytical aspects rather than mundane tasks. From data mining to predictive modeling, AI offers a range of solutions that streamline the research process and facilitate deeper insights.
One of the most significant contributions of AI in academia is its ability to handle large volumes of complex data. AI-driven algorithms can sift through vast datasets, identifying patterns and correlations that would be challenging for humans to detect. This capability not only accelerates research but also enables more accurate predictions and conclusions. AI tools are becoming indispensable in fields like genomics, climate science, and social research, where data complexity often exceeds traditional analytical methods.
Key Areas AI Enhances Research
- Data Analysis and Pattern Recognition: AI-powered algorithms help researchers identify trends and patterns in large datasets, facilitating faster discovery and more accurate results.
- Automated Literature Review: AI tools can scan academic papers and extract relevant information, saving researchers time and effort in the literature review process.
- Predictive Analytics: Machine learning models can predict outcomes and trends, enabling researchers to test hypotheses before conducting costly experiments.
- Collaboration and Communication: AI facilitates better collaboration through tools like virtual research assistants, automated project management, and seamless communication platforms.
"AI empowers researchers to move beyond manual processes, offering more robust and efficient solutions for academic inquiries."
Applications of AI in Different Fields
Field | AI Application |
---|---|
Genomics | AI analyzes genetic data to identify disease markers and predict genetic disorders. |
Climate Science | AI helps model and predict climate changes by analyzing complex environmental data. |
Social Sciences | AI processes large-scale survey data to uncover insights into social trends and behaviors. |
Medical Research | AI assists in drug discovery and patient diagnostics by analyzing vast medical datasets. |
By leveraging AI solutions, academic institutions can not only enhance the productivity of researchers but also foster more interdisciplinary collaborations. As AI tools continue to evolve, their role in advancing academic research will only become more central, paving the way for groundbreaking discoveries and innovations.
AI-Enhanced Evaluation: Streamlining Grading and Providing Instant Feedback
The integration of AI technologies in academic evaluation is transforming the way educators assess student performance. Machine learning algorithms, natural language processing (NLP), and data analytics are now being employed to automate grading and provide immediate feedback, eliminating the delays associated with traditional grading methods. This approach not only ensures consistency but also reduces the workload on instructors, allowing them to focus on more personalized aspects of teaching. AI-driven tools can grade essays, quizzes, and even complex assignments with remarkable precision, ensuring fairness and objectivity in assessments.
Automating the grading process through AI has the potential to increase the efficiency of educational institutions while maintaining high standards. AI systems can provide feedback that is both timely and actionable, helping students identify areas for improvement and enhancing their learning experience. Moreover, these technologies can be tailored to assess a wide range of student work, from multiple-choice tests to open-ended essays, making them versatile tools in diverse academic environments.
Key Advantages of AI in Assessment
- Efficiency: Grading is faster, allowing educators to allocate more time to individualized instruction.
- Consistency: AI systems can evaluate work based on predetermined criteria, ensuring uniformity in grading.
- Timely Feedback: Students receive immediate insights into their performance, fostering a quicker learning cycle.
Types of Assessments Suitable for AI Automation
- Multiple-Choice and True/False Tests: AI can automatically evaluate these types of questions with high accuracy.
- Short-Answer Questions: NLP techniques help assess open-ended responses, ensuring that answers are analyzed based on their content and relevance.
- Essays and Research Papers: AI can examine text coherence, grammar, and argumentation while also checking for originality and plagiarism.
Considerations for Implementing AI in Assessment
Consideration | Impact |
---|---|
Data Privacy | Ensuring that student data is secure and used ethically. |
Accuracy of AI Models | Ensuring that AI systems are trained effectively to minimize errors in grading. |
Faculty Acceptance | Educators may require training to integrate AI tools seamlessly into their grading workflows. |
“AI-driven assessments can dramatically enhance the efficiency of grading while maintaining accuracy, but their success depends on proper implementation and continuous evaluation of their effectiveness.”
Addressing Ethical Concerns in AI Use in Education
The integration of artificial intelligence in higher education raises significant ethical challenges that must be addressed for effective and responsible implementation. As AI tools are increasingly utilized for teaching, assessment, and administrative tasks, it is critical to ensure that they are designed and used in a manner that respects privacy, equity, and academic integrity. These challenges include data privacy concerns, algorithmic biases, and the potential for AI to replace human educators in ways that could undermine the personal touch in education.
In particular, ethical considerations must be made around the collection and use of student data. AI systems that analyze student performance or behavior rely on vast amounts of data, which can lead to privacy violations or the mismanagement of sensitive information. Additionally, there is a risk that AI tools, if not carefully designed, could perpetuate existing biases, disadvantaging certain student groups based on race, gender, or socioeconomic status. Therefore, the ethical deployment of AI in education requires transparent policies, careful oversight, and a commitment to fairness.
Key Ethical Concerns in AI in Education
- Privacy and Data Security: Ensuring that student data is protected from misuse or unauthorized access.
- Algorithmic Bias: Addressing potential biases in AI models that may lead to discriminatory outcomes for certain student populations.
- Impact on Educators: Evaluating the effects of AI on teaching roles, including the potential for job displacement and reduced human interaction.
- Accountability and Transparency: Ensuring that AI-driven decisions are transparent and that there is a clear accountability structure for outcomes.
Important considerations for AI in education:
Ethical AI use in education requires ongoing dialogue between educators, technologists, policymakers, and students to build systems that are both effective and equitable.
Strategies for Mitigating Ethical Risks
- Regular Audits of AI Systems: Implementing frequent audits to ensure AI models are operating fairly and without bias.
- Transparency in Data Collection: Informing students about what data is being collected and how it is being used.
- Human Oversight: Ensuring that AI decisions are subject to review by qualified human educators to avoid over-reliance on automated systems.
- Inclusive Design: Involving diverse stakeholders in the design of AI systems to minimize bias and promote fairness.
Best Practices for Ethical AI Use in Education
Ethical Principle | Recommended Practice |
---|---|
Privacy | Implement strong encryption and anonymization techniques for student data. |
Bias Reduction | Use diverse training datasets and continually test AI models for bias. |
Accountability | Establish clear policies for AI decision-making and ensure human oversight in critical decisions. |
Training Faculty and Staff for AI Integration in Higher Education
As Artificial Intelligence (AI) continues to shape the future of higher education, it becomes increasingly critical to equip faculty and staff with the necessary skills to implement and utilize AI tools effectively. This training not only empowers educators to integrate AI into their teaching but also helps administrative staff leverage AI to streamline operations, enhance student engagement, and improve institutional efficiency.
To ensure successful adoption, higher education institutions need to design training programs that address both the technical and pedagogical aspects of AI. The training should cover basic AI concepts, data ethics, AI applications in education, and hands-on usage of AI-powered platforms. Faculty and staff must also be prepared to adapt to the evolving nature of AI technologies and integrate them in ways that foster student-centered learning environments.
Key Training Components
- Understanding AI Basics: Introducing fundamental concepts such as machine learning, neural networks, and natural language processing.
- AI in Education: Exploring how AI can personalize learning, automate administrative tasks, and facilitate content creation.
- Ethical Considerations: Addressing data privacy, bias in algorithms, and the societal implications of AI technology.
- Hands-On AI Tools: Familiarizing faculty with AI-driven platforms for teaching, grading, and student support.
Steps for Effective Training
- Needs Assessment: Conducting surveys to identify specific knowledge gaps and areas for development.
- Modular Learning: Offering tiered courses that allow participants to start with foundational knowledge and progress to more advanced skills.
- Ongoing Support: Providing access to resources like online forums, workshops, and expert consultations for continuous learning.
Example Training Structure
Session | Topic | Duration |
---|---|---|
1 | Introduction to AI and Machine Learning | 2 hours |
2 | AI Tools for Teaching and Assessment | 3 hours |
3 | Ethical Implications of AI in Education | 2 hours |
4 | Hands-On AI Platforms for Faculty | 4 hours |
"For AI integration to be successful in higher education, it is essential that faculty and staff not only understand the technology but also have the skills to apply it in ways that enhance learning outcomes and institutional efficiency."