Personalize.ai Zs
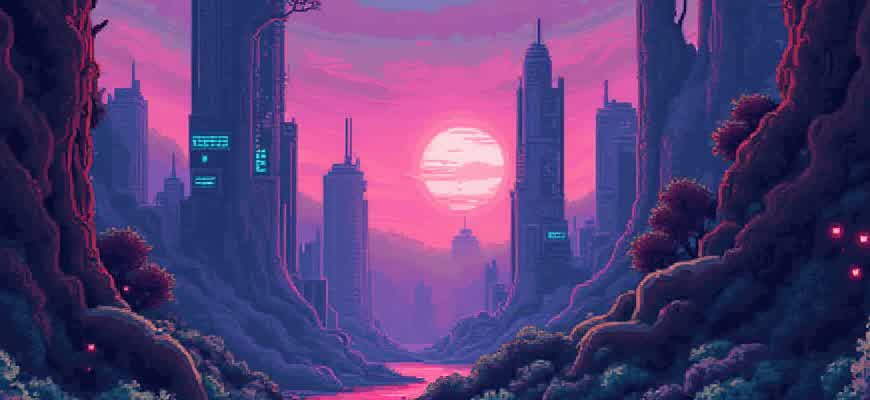
Personalize.ai Zs represents a significant advancement in the field of artificial intelligence, focusing on delivering highly tailored user experiences through machine learning. This cutting-edge system integrates diverse data sources to generate personalized interactions, improving both user engagement and satisfaction. The Zs model operates on a deep learning framework, where continuous training allows it to adapt and evolve with user behavior over time.
Key Features of Personalize.ai Zs:
- Advanced Natural Language Processing (NLP) capabilities
- Real-time data analysis for dynamic content adaptation
- Seamless integration with third-party platforms and tools
- Multi-modal user interaction support, including text, voice, and visual inputs
The architecture behind Personalize.ai Zs is built on scalable cloud infrastructure that allows it to handle vast amounts of data while ensuring low-latency processing. Here is a comparison of the Zs model with traditional AI personalization systems:
Feature | Traditional AI Systems | Personalize.ai Zs |
---|---|---|
Data Input Types | Limited (mainly text-based) | Multi-modal (text, voice, images) |
Real-time Adaptation | Delayed | Instantaneous |
Integration Flexibility | Rigid | Highly flexible and extensible |
"Personalize.ai Zs is not just an AI tool–it's a transformative platform that reshapes the way businesses interact with users, creating truly customized experiences that were previously unimaginable."
Setting Up Personalized Recommendations for E-commerce Platforms
Personalized recommendations are essential for enhancing the user experience on e-commerce platforms. They increase customer engagement, drive sales, and improve customer retention by providing tailored product suggestions based on individual preferences and behaviors. A well-designed recommendation system considers a variety of factors, including browsing history, previous purchases, and even real-time interactions with the site.
To set up an effective personalized recommendation system, businesses must leverage advanced algorithms and data analytics to predict customer preferences accurately. Machine learning models, such as collaborative filtering, content-based filtering, and hybrid approaches, can be integrated into the platform's infrastructure to analyze vast amounts of user data and generate relevant recommendations in real time.
Steps to Implement Personalized Recommendations
- Data Collection: Gather user data such as browsing history, purchase behavior, and search queries.
- Data Processing: Clean and organize the data to remove duplicates, irrelevant information, and inconsistencies.
- Algorithm Selection: Choose an appropriate recommendation algorithm based on the available data and business goals.
- Model Training: Use machine learning techniques to train the recommendation system, ensuring it can make accurate predictions.
- Real-Time Integration: Integrate the recommendation engine with the platform to provide personalized suggestions on the fly.
- Testing and Optimization: Continuously monitor performance and optimize algorithms to improve accuracy and relevance.
Types of Personalization Techniques
- Collaborative Filtering: Based on user similarities, this technique suggests products by analyzing behaviors of similar users.
- Content-Based Filtering: Recommends products based on their attributes, matching them with user preferences and past behavior.
- Hybrid Methods: Combines multiple recommendation techniques to enhance accuracy and mitigate individual method limitations.
“Personalized recommendations are not only about showing products; they are about creating an experience that feels tailored to each user’s unique needs.”
Key Metrics to Measure Success
Metric | Description |
---|---|
Conversion Rate | Measures the percentage of users who make a purchase after receiving a recommendation. |
Click-Through Rate (CTR) | Tracks how often recommended products are clicked by users. |
Revenue per User | Calculates the average revenue generated from a user who interacts with recommendations. |
Customer Retention Rate | Assesses how well personalized recommendations contribute to keeping users engaged over time. |
Measuring the Impact of Personalization on User Retention
Personalization plays a critical role in enhancing user retention, especially in digital platforms where engagement is key to long-term success. By tailoring experiences to individual preferences and behaviors, businesses can significantly improve their ability to retain users over time. This approach creates a sense of value and relevance, which strengthens the connection between users and the product or service.
To evaluate the effectiveness of personalized experiences in retaining users, businesses need to rely on specific metrics and analysis methods. These measurements help in understanding how customization influences user behavior, retention rates, and long-term engagement. Some of the most effective ways to assess the impact of personalization on retention include tracking key performance indicators (KPIs) such as return visits, customer lifetime value, and engagement metrics.
Key Metrics for Assessing Retention
- Return Frequency: Measures how often users return to the platform after initial engagement.
- Churn Rate: Tracks the percentage of users who stop using the service after a certain period.
- Active Session Duration: Reflects how long users engage with the platform during each visit.
Important Consideration: Personalization might not yield immediate results but tends to show its impact in the long term. Regularly reviewing these metrics ensures a clear understanding of the ongoing effect of personalized strategies.
Example of Personalized Strategy Impact
Strategy | Before Personalization | After Personalization |
---|---|---|
User Return Frequency | 2 visits per month | 4 visits per month |
Churn Rate | 30% | 15% |
Active Session Duration | 5 minutes | 10 minutes |
"Personalized experiences lead to deeper engagement, resulting in higher user retention rates over time. Even subtle adjustments to content or interface based on user preferences can have a significant impact on overall satisfaction and loyalty."