Intelligent Information Management
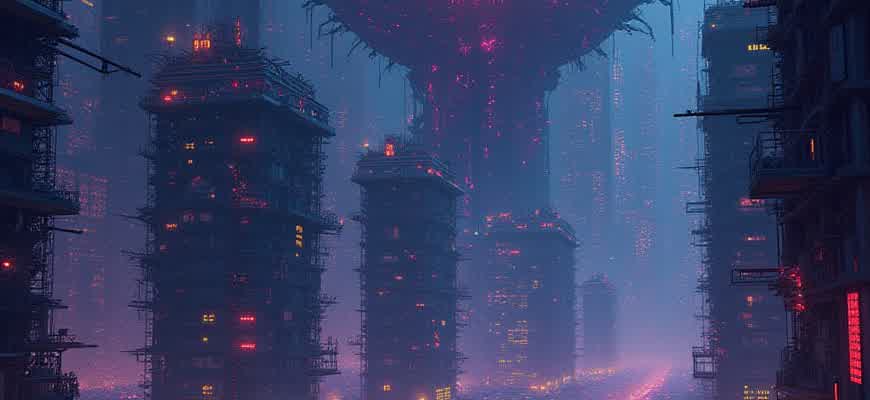
In today’s fast-paced digital environment, organizations require robust systems to handle vast amounts of data. Effective management of information is crucial not only for operational efficiency but also for strategic decision-making. By integrating intelligent technologies, companies can transform raw data into actionable insights, fostering innovation and improving performance.
Modern intelligent systems utilize advanced algorithms to streamline information management, reducing manual intervention and increasing data accessibility. These technologies incorporate machine learning, artificial intelligence, and natural language processing to automate categorization, retrieval, and analysis of data.
Key Benefits of Intelligent Information Systems:
- Enhanced data retrieval and storage efficiency.
- Automation of repetitive tasks, leading to time savings.
- Increased accuracy in decision-making through data-driven insights.
To illustrate the impact, consider the following comparison between traditional and intelligent systems in terms of data management:
Traditional System | Intelligent System |
---|---|
Manual data entry | Automated data capture |
Slow data processing | Real-time processing |
High risk of human error | Minimal errors through AI validation |
Implementing AI for Automated Data Classification and Retrieval
Artificial Intelligence (AI) is increasingly becoming a powerful tool in optimizing information management systems, especially for tasks like data classification and retrieval. With vast amounts of data generated daily, traditional manual methods are no longer efficient or scalable. AI-driven solutions offer automated approaches to streamline these processes, significantly improving both accuracy and speed.
AI can automatically analyze, categorize, and retrieve information based on pre-defined rules or patterns it has learned. This is especially useful for industries dealing with large volumes of unstructured data, such as healthcare, finance, and legal sectors. The deployment of AI tools for these tasks can reduce human error, ensure consistent data processing, and facilitate quicker access to relevant information when needed.
Key Benefits of AI for Data Management
- Enhanced Accuracy: AI algorithms minimize human errors in data categorization, ensuring more reliable outcomes.
- Increased Efficiency: Automation reduces the time required for data processing and retrieval, allowing businesses to focus on strategic tasks.
- Scalability: AI systems can handle large datasets and adapt to increasing data volumes without sacrificing performance.
How AI Automates Data Classification
AI uses machine learning algorithms to classify data into predefined categories. These algorithms are trained using labeled datasets, enabling the AI to recognize patterns and similarities in new, unseen data. The process typically follows these steps:
- Data Collection: The AI system gathers large datasets that contain both structured and unstructured data.
- Training: Machine learning models are trained using the collected data, identifying patterns and creating rules for classification.
- Classification: Once trained, the system applies the learned models to classify incoming data in real-time.
- Validation and Refinement: The system is continually refined based on user feedback and new data, improving its accuracy over time.
Data Retrieval Using AI
AI-based retrieval systems use natural language processing (NLP) to understand and respond to user queries. Unlike traditional keyword-based search engines, AI systems can interpret the meaning behind queries, delivering more relevant and contextually appropriate results. The retrieval process involves:
- Context Analysis: AI evaluates the context of the query, considering factors like the user’s previous searches and preferences.
- Content Indexing: AI indexes content based on the classification rules it has learned, making it easier to retrieve relevant information.
- Personalized Results: AI customizes retrieval results, offering more accurate information tailored to the user’s specific needs.
Summary
Implementing AI for data classification and retrieval streamlines information management processes, enabling faster, more accurate, and scalable systems. It not only optimizes operations but also enhances the ability to extract meaningful insights from vast amounts of data.
Examples of AI Tools for Data Management
Tool | Use Case | Industry |
---|---|---|
IBM Watson | Natural Language Processing for data retrieval | Healthcare, Finance |
Google AutoML | Automated machine learning for classification | Retail, Marketing |
Microsoft Azure AI | Cloud-based AI solutions for data management | Enterprise, IT |
Streamlining Collaboration through Centralized Data Access
Efficient collaboration within organizations is highly dependent on the ease of accessing and sharing data. By centralizing information storage, teams can eliminate silos and work from a unified source of truth, reducing the chances of errors and miscommunications. Centralized data access enhances decision-making by providing real-time updates and ensuring everyone is on the same page.
With a centralized data system, employees can quickly retrieve necessary files and documents, fostering quicker response times and more agile workflows. The reduction in redundant data entry and the elimination of inconsistent versions of documents ensures that everyone is working with the latest information, thus improving the overall effectiveness of collaboration.
Benefits of Centralized Data Access
- Improved Communication: With shared access to up-to-date data, teams can collaborate without barriers, improving communication across departments.
- Faster Decision-Making: Real-time access to information allows teams to make well-informed decisions quickly.
- Consistent Data: Centralization ensures that all employees work with the most accurate and recent data, reducing discrepancies and errors.
Key Strategies for Implementing Centralized Data Access
- Cloud-based Solutions: Utilizing cloud storage systems can provide secure, scalable, and easy access to data from any location.
- Integrated Tools: Adopting collaboration platforms that seamlessly integrate with the central data repository ensures smooth workflows.
- Access Control Policies: Implementing role-based access ensures that sensitive information is protected while enabling the right individuals to access the necessary data.
"Centralized data access is not just about storage, it's about empowering teams to collaborate more effectively, using accurate and timely information."
Challenges to Consider
Challenge | Solution |
---|---|
Data Security | Implement encryption and access control protocols to protect sensitive data. |
Resistance to Change | Provide training and demonstrate the efficiency improvements to encourage adoption. |
Data Overload | Implement filtering and tagging systems to ensure relevant data is easily accessible. |
Integrating Information Management Systems with Existing IT Infrastructure
Integrating an information management system (IMS) with an organization's existing IT infrastructure is crucial for ensuring smooth workflows and enhancing operational efficiency. The process requires careful consideration of the underlying technological landscape, as well as the interoperability between various systems already in place. A successful integration involves aligning the IMS with existing software, databases, and hardware to optimize the management and flow of information across all departments.
One of the key challenges during integration is ensuring that the new system can seamlessly interact with legacy systems, which often involve outdated technologies. This requires the use of adaptable, scalable solutions that can bridge gaps between old and new systems. Below are some important steps for a successful integration process.
Key Integration Steps
- Assess IT Infrastructure: Perform a thorough evaluation of the current IT infrastructure, including hardware, software, and network components.
- Define Compatibility Requirements: Identify compatibility requirements for the IMS to ensure it can work with existing systems.
- Data Migration Strategy: Plan how data will be transferred from older systems to the new IMS without loss or corruption.
- API and Middleware Usage: Utilize APIs or middleware to facilitate communication between the IMS and other systems.
Successful integration of an IMS enhances real-time data accessibility, improves decision-making, and reduces operational silos within the organization.
Common Tools and Techniques
Tool/Technique | Purpose | Benefits |
---|---|---|
Middleware | Acts as an intermediary between different systems to enable communication | Improves system compatibility and reduces integration complexity |
API Integration | Facilitates data exchange between systems | Enables seamless data synchronization between platforms |
Cloud Integration | Connects on-premise systems with cloud-based solutions | Enhances scalability and flexibility of the IT infrastructure |
Challenges to Consider
- Data security and compliance risks when transferring sensitive information between systems.
- Potential disruption to business operations during the transition phase.
- The need for training and change management to ensure smooth adoption by users.
Ensuring Data Security and Compliance with Intelligent Information Solutions
In the modern landscape of data management, securing sensitive information and ensuring compliance with industry regulations have become paramount concerns for organizations. With the exponential growth of data, businesses are turning to intelligent information management solutions to address these challenges. These systems use advanced technologies such as AI, machine learning, and automation to not only streamline data processes but also safeguard the integrity and privacy of the information they handle.
Intelligent solutions offer robust frameworks that help organizations maintain compliance with laws such as GDPR, HIPAA, and others by ensuring proper data classification, access control, and audit trails. These technologies automate compliance checks and data security protocols, reducing the risk of human error and ensuring that security measures are constantly up to date with evolving regulations.
Key Elements for Data Security and Compliance
- Data Encryption: Ensures that sensitive data is securely encoded, making it unreadable to unauthorized users.
- Access Control: Utilizes role-based access to limit who can view, edit, and share sensitive data.
- Data Classification: Categorizes data based on sensitivity levels to implement appropriate protection measures.
- Audit Trails: Provides detailed logs of who accessed data, when, and why, ensuring transparency and accountability.
- Automated Compliance Monitoring: Continuously checks data handling practices against legal and regulatory requirements.
Practical Benefits of Intelligent Solutions in Security
Adopting an intelligent information management system offers several practical advantages in securing data and ensuring compliance:
- Proactive Risk Management: AI-driven systems can predict and identify potential security threats before they become major issues.
- Efficient Compliance Reporting: Automates the generation of compliance reports, making audits smoother and reducing administrative overhead.
- Cost-Effective Security: By automating security measures and compliance checks, organizations can reduce the need for manual oversight, lowering operational costs.
Challenges and Solutions
Despite the significant benefits, organizations may face challenges when integrating intelligent information systems. These include data privacy concerns, resistance to new technologies, and the complexity of implementing such solutions across legacy systems. However, with proper planning and vendor support, these challenges can be overcome, ensuring that both security and compliance are robustly addressed.
"Intelligent systems transform how businesses manage security and compliance, offering automation, precision, and adaptability in an ever-evolving regulatory environment."
Compliance Guidelines Table
Regulation | Compliance Requirement | Impact on Data Security |
---|---|---|
GDPR | Data protection and privacy for all individuals within the EU | Requires encryption, data access control, and breach notification |
HIPAA | Protection of healthcare data in the US | Mandates secure storage, transmission, and access controls |
CCPA | Privacy rights for California residents | Demands transparency in data collection and allows consumer opt-out options |
Optimizing Document Workflow with Smart Tagging and Indexing
Efficient document management plays a crucial role in modern enterprises, as it directly impacts workflow productivity. A key strategy to streamline document handling is the implementation of advanced tagging and indexing techniques. By utilizing automated tagging systems and indexing protocols, organizations can significantly reduce the time spent searching for, categorizing, and retrieving files, thus improving operational efficiency.
Smart tagging and indexing allow for more precise document categorization and retrieval. These methods integrate AI-driven tools to automatically assign contextually relevant tags based on content analysis, facilitating faster sorting and access to critical information. Below are some of the key benefits:
- Faster Document Retrieval: Tags improve search accuracy and speed by creating a more intuitive categorization system.
- Reduced Errors: Automatic tagging reduces the risk of human error in document classification.
- Enhanced Collaboration: Teams can easily find and share documents, ensuring better collaboration across departments.
Effective indexing takes the process a step further by structuring documents into searchable categories. This ensures that once tagged, each document is indexed in a way that supports future retrieval, regardless of content format or type. Here's a comparison of manual vs. automated indexing methods:
Method | Advantages | Challenges |
---|---|---|
Manual Indexing | Customizable, suited for specific document needs | Time-consuming, prone to human error |
Automated Indexing | Efficient, scalable, reduces errors | Requires initial setup and ongoing adjustments |
"Implementing intelligent tagging and indexing systems is essential for businesses that need to handle large volumes of documents quickly and accurately."
Incorporating these technologies ensures that organizations can stay ahead in terms of both document management and workflow efficiency, fostering a more productive environment overall.
Anticipating Information Requirements through Predictive Analytics
In modern information management systems, the ability to foresee and address information needs is increasingly critical. Leveraging predictive analytics provides organizations with the tools to proactively predict what data will be required in the future, improving decision-making processes and enhancing operational efficiency. By analyzing historical data, trends, and patterns, predictive models help identify potential information gaps before they become a bottleneck, enabling smoother workflows and better resource allocation.
Through the application of machine learning algorithms and statistical models, organizations can anticipate not only immediate information needs but also long-term trends that influence business strategies. This capability can transform how information is managed and accessed, reducing response time and improving data-driven insights. Below are key benefits and methods used to apply predictive analytics in anticipating information requirements:
- Data-driven Forecasting: Predictive models analyze past usage patterns to forecast future demands for specific information or resources.
- Improved Decision-Making: By anticipating data needs, decision-makers can access the right information at the right time, reducing delays in critical business processes.
- Efficient Resource Allocation: Predictive analytics can help allocate resources more effectively by predicting data storage needs, infrastructure requirements, and personnel demands.
To illustrate this, consider the following table, which highlights how predictive models can forecast various aspects of information management:
Information Need | Prediction Method | Impact |
---|---|---|
Customer Support Queries | Analysis of past queries and sentiment trends | Optimized staffing and quicker response times |
Sales Data Analysis | Sales forecasting using historical transaction data | Better inventory management and demand forecasting |
Regulatory Compliance Updates | Risk assessment models based on past compliance trends | Timely compliance adjustments and proactive reporting |
"By predicting information needs before they arise, organizations can eliminate unnecessary delays and ensure the right resources are always available when needed."