Ai and Healthcare Analytics
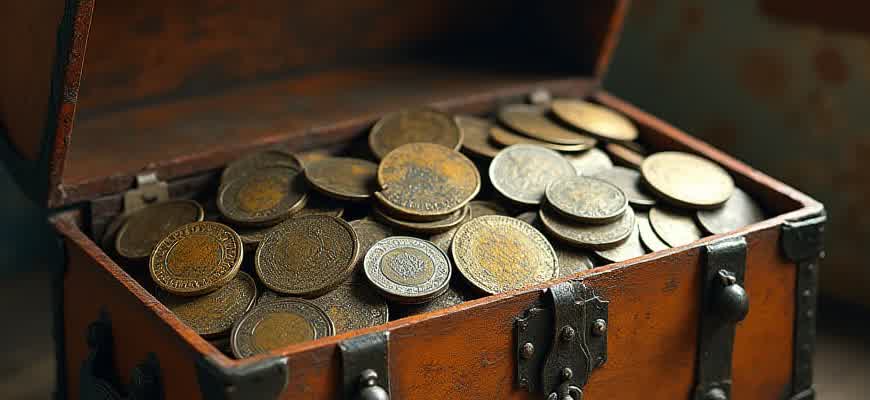
Artificial intelligence (AI) is transforming the way healthcare data is processed, analyzed, and interpreted. By leveraging machine learning algorithms, AI systems can identify patterns in vast datasets, providing insights that were previously difficult to detect. This has led to improvements in diagnosis accuracy, patient care, and operational efficiency within healthcare organizations.
Key applications of AI in healthcare analytics include:
- Predictive analytics for patient outcomes
- Automated medical image analysis
- Personalized treatment recommendations
AI models have the ability to process and analyze medical data at an unprecedented speed, enabling healthcare professionals to make faster, data-driven decisions.
Examples of AI-driven healthcare technologies:
- IBM Watson Health for data interpretation
- Google Health’s AI-assisted diagnostics tools
- Machine learning-based predictive models for disease outbreaks
Comparison of Traditional and AI-powered Analytics:
Aspect | Traditional Analytics | AI-powered Analytics |
---|---|---|
Data Processing Speed | Slower, manual processes | Rapid, real-time analysis |
Accuracy | Human error-prone | High, with machine learning optimization |
Scalability | Limited to small datasets | Capable of handling vast datasets |
Artificial Intelligence in Healthcare Analytics: Enhancing Patient Care
The integration of artificial intelligence in healthcare analytics has proven to be a game-changer in improving patient outcomes. By leveraging machine learning and big data, AI systems can analyze vast amounts of patient information, allowing healthcare providers to make more informed and timely decisions. These technologies help identify patterns that would be difficult for human doctors to detect, leading to earlier diagnosis and more personalized treatment plans.
AI-driven analytics also support predictive modeling, which can forecast potential health risks and recommend preventative measures. By continuously analyzing patient data, these systems can adapt over time, providing increasingly accurate insights that aid in reducing errors and enhancing care efficiency. The result is a healthcare system that is more proactive and responsive to individual patient needs.
Key Benefits of AI in Healthcare Analytics
- Personalized Treatment Plans: AI helps create tailored treatment plans based on a patient's unique medical history and data.
- Predictive Diagnostics: Machine learning models predict the likelihood of diseases, enabling early intervention.
- Improved Operational Efficiency: AI streamlines administrative processes, reducing waiting times and improving resource allocation.
- Reduced Human Error: AI assists doctors in making data-driven decisions, decreasing the chances of mistakes in diagnosis and treatment.
AI Applications in Healthcare Analytics
- Data Processing and Analysis: AI systems process vast amounts of unstructured data (e.g., medical records, imaging, and genomics) to extract valuable insights.
- Predictive Health Monitoring: AI monitors patient data in real-time to identify early warning signs of health deterioration.
- Clinical Decision Support: AI algorithms recommend treatment options, ensuring that decisions align with the best available evidence.
"AI in healthcare is revolutionizing patient care by enabling real-time analysis of data, helping clinicians make more precise decisions and ultimately improving patient outcomes." – Healthcare Innovators Report, 2024
Data-Driven Decision Making
AI systems are equipped to handle large-scale data from diverse sources, such as wearables, electronic health records (EHRs), and medical imaging. These systems use complex algorithms to detect trends and anomalies in patient data, providing healthcare professionals with accurate and timely insights. This facilitates a shift from a reactive to a proactive approach in patient care, where decisions are made based on comprehensive data rather than just symptoms.
Key Metrics for Evaluating AI's Impact in Healthcare
Metric | Impact |
---|---|
Patient Outcome Improvement | Early detection of diseases and optimized treatment plans lead to higher survival rates and improved quality of life. |
Cost Efficiency | Reducing unnecessary treatments and hospital readmissions through accurate predictions lowers overall healthcare costs. |
Operational Productivity | Automation of routine tasks and better resource management improve hospital workflow efficiency. |
Improving Diagnostic Accuracy with AI-driven Data Analysis
Artificial intelligence is increasingly enhancing the diagnostic processes in healthcare by leveraging data analysis techniques to identify patterns that might be missed by human clinicians. Machine learning algorithms, particularly those trained on vast datasets, can significantly improve the precision of diagnoses. By analyzing large volumes of clinical data, AI models can predict outcomes, highlight potential risks, and suggest more accurate diagnostic pathways.
AI-powered systems utilize various types of medical data such as imaging, patient histories, and genetic information. The ability to process and analyze this data in real-time helps clinicians make faster and more informed decisions. Moreover, these systems are continually learning from new data, which means their accuracy improves over time, further reducing the likelihood of human error in diagnostic processes.
Key AI Benefits for Diagnostics
- Enhanced Precision: Machine learning models detect patterns that might be overlooked by humans, improving diagnostic accuracy.
- Data Integration: AI systems integrate diverse types of medical data, providing a holistic view of the patient's condition.
- Real-time Processing: AI tools process data in real-time, enabling quicker decision-making for better patient outcomes.
Examples of AI in Diagnostic Applications
- AI in medical imaging: AI algorithms identify abnormalities in X-rays, MRIs, and CT scans more precisely than traditional methods.
- Genetic testing: AI systems analyze genetic data to predict the likelihood of diseases and suggest preventive measures.
- Predictive analytics: AI models can predict disease progression, offering early intervention opportunities and reducing treatment costs.
"AI-driven systems are not only transforming diagnostics but also reshaping the way healthcare providers approach patient care and treatment plans."
AI-Driven Diagnostic Accuracy in Numbers
Technology | Accuracy Rate | Common Use Cases |
---|---|---|
Deep Learning in Imaging | 95% | X-ray and MRI analysis |
Predictive Analytics | 90% | Disease progression forecasts |
Genomic AI Models | 85% | Genetic risk assessment |
Leveraging Predictive Analytics to Prevent Hospital Readmissions
Predictive analytics plays a pivotal role in reducing the occurrence of patient readmissions. By analyzing vast amounts of patient data, healthcare providers can forecast which individuals are at a higher risk of returning to the hospital after discharge. This approach empowers medical teams to offer more personalized care, reducing unnecessary costs and improving overall patient outcomes.
Hospitals are increasingly adopting advanced algorithms to monitor patient conditions and identify potential risks early. By integrating predictive models into daily workflows, healthcare professionals can make data-driven decisions that directly impact the likelihood of readmission, ultimately enhancing patient care quality and resource management.
How Predictive Models Work in Reducing Readmissions
Predictive models use historical patient data, clinical information, and real-time monitoring to forecast readmission risks. Key factors that influence these models include:
- Patient medical history (chronic conditions, past hospitalizations)
- Social determinants of health (living conditions, access to transportation)
- Discharge planning and follow-up care
- Medication adherence
By evaluating these data points, models can flag patients who may need additional attention. Once identified, healthcare providers can take preventive measures to avoid readmissions.
Actionable Steps to Prevent Readmissions
- Improved Discharge Planning: Tailored discharge plans based on individual risk factors can ensure patients understand their follow-up care and medication regimen.
- Early Interventions: By monitoring high-risk patients through telemedicine or home visits, caregivers can address potential complications before they result in hospital readmissions.
- Medication Management: Ensuring that patients are adhering to prescribed medications is critical in preventing complications and readmissions.
Predictive analytics has shown to reduce readmission rates by up to 20%, saving hospitals millions annually while improving patient care and satisfaction.
Example of Predictive Analytics in Action
Factor | Impact on Readmission Risk |
---|---|
Chronic Conditions | Higher risk due to ongoing management needs |
Recent Surgery | Increased risk if post-operative care is inadequate |
Social Support | Lower risk with adequate home care and family support |
Medication Non-Adherence | Significantly increases the chance of complications and readmission |
Using AI to Streamline Patient Data Management Systems
Efficient management of patient data is essential for improving healthcare outcomes and optimizing operational workflows. Artificial Intelligence (AI) technologies provide powerful solutions for automating and enhancing the management of vast amounts of patient information. By leveraging AI, healthcare organizations can reduce administrative burdens, improve data accuracy, and ensure that critical health information is readily accessible for clinical decision-making.
AI-driven tools offer advanced capabilities, such as data cleaning, real-time analytics, and predictive insights. These technologies can identify patterns and trends in patient data, enabling healthcare providers to make more informed decisions and deliver personalized care. The integration of AI in patient data management can also facilitate seamless communication between different healthcare systems and professionals, ultimately leading to improved coordination and better patient outcomes.
Key Benefits of AI in Patient Data Management
- Data Organization: AI helps categorize and index patient records, making it easier to search and retrieve relevant information quickly.
- Predictive Analytics: Machine learning models can predict potential health risks, allowing for early interventions and proactive care management.
- Improved Accuracy: AI minimizes human error in data entry and processing, ensuring that patient records are more accurate and complete.
- Automation of Routine Tasks: Administrative tasks such as appointment scheduling, billing, and follow-up reminders can be automated, freeing up healthcare staff to focus on patient care.
AI Solutions for Data Integration and Analysis
- Natural Language Processing (NLP): NLP algorithms can extract meaningful insights from unstructured data such as doctor’s notes and patient histories.
- Machine Learning Algorithms: These algorithms analyze historical data to predict patient outcomes and suggest personalized treatment plans.
- Data Visualization Tools: AI-powered dashboards and visualizations help healthcare providers easily interpret complex patient data in real time.
"AI-driven systems not only streamline data management but also enhance clinical decision-making by providing healthcare professionals with actionable insights derived from comprehensive data analysis."
Example of AI-Enhanced Data Management Workflow
Stage | AI-Driven Action | Outcome |
---|---|---|
Data Collection | Automated data input from various sources (e.g., sensors, EHR) | Accurate and real-time patient data |
Data Processing | AI algorithms detect anomalies and clean up inconsistencies | Improved data quality and completeness |
Data Analysis | Predictive models generate patient risk profiles and recommendations | Informed decision-making and personalized care plans |
Improving Individualized Treatment Strategies through Machine Learning
In modern healthcare, personalized treatment plans are becoming increasingly feasible due to advancements in machine learning (ML). By analyzing vast amounts of patient data, ML algorithms are able to identify patterns and insights that help tailor medical care to each individual’s unique needs. These technologies enable the creation of more precise treatment protocols, taking into account factors such as genetics, lifestyle, and response to previous therapies.
Machine learning also plays a critical role in predictive analytics, where models can forecast the outcomes of various treatments based on historical data. This approach not only improves the accuracy of diagnoses but also enhances the ability to predict the most effective treatment options, significantly reducing trial-and-error in therapy selection.
Key Benefits of Machine Learning in Personalized Treatment
- Improved Diagnosis Accuracy: By analyzing patient data, machine learning can help detect diseases at an earlier stage, allowing for timely intervention.
- Data-Driven Treatment Choices: ML systems can suggest personalized medication and treatment strategies based on an individual’s medical history and genetic profile.
- Adaptive Treatment Models: As new patient data becomes available, machine learning models continuously update, ensuring that treatment plans remain optimal over time.
"Machine learning not only personalizes treatment but also continuously adapts, offering a dynamic approach to patient care that evolves with emerging data."
Example of ML in Action: Oncology
One area where machine learning is particularly transformative is in oncology. By analyzing genetic mutations and biomarkers, ML can predict how different cancer types will respond to specific drugs, allowing doctors to select the most effective therapy based on the patient’s profile.
Cancer Type | ML-Predicted Best Treatment |
---|---|
Lung Cancer | Targeted therapy based on EGFR mutation |
Breast Cancer | Personalized chemotherapy regimen based on gene expression profiling |
Colorectal Cancer | Immunotherapy based on MSI-H status |
Reducing Operational Costs with AI-based Healthcare Automation
In the rapidly evolving healthcare landscape, organizations are increasingly relying on automation powered by artificial intelligence (AI) to optimize operational efficiency. AI-based systems can perform routine administrative tasks, reduce human error, and streamline complex processes, leading to significant cost savings. This technology has the potential to transform healthcare operations by automating everything from patient intake and scheduling to billing and claims processing.
Automation helps healthcare providers reduce operational costs by eliminating manual, time-consuming tasks, thus allowing staff to focus on more value-driven activities. Furthermore, AI can predict trends, improve resource allocation, and enhance decision-making, all of which contribute to better cost management across the organization.
Key Benefits of AI-driven Automation in Healthcare
- Efficient Resource Management: AI systems analyze real-time data to predict demand and allocate resources efficiently, reducing both overstaffing and underutilization.
- Enhanced Billing and Claims Processing: Automation minimizes errors in billing, reduces claim rejections, and speeds up the reimbursement process, leading to lower administrative costs.
- Improved Patient Scheduling: AI algorithms optimize appointment scheduling based on patient needs and healthcare provider availability, reducing no-show rates and improving patient throughput.
AI Automation in Action: A Case Example
Task | AI Impact | Cost Reduction |
---|---|---|
Patient Data Entry | Automated data capture from digital forms and wearable devices | Reduces administrative workload by 30%, saving time and labor costs |
Claims Processing | AI algorithms validate claims and detect errors in real time | Improves first-pass claim approval rate by 40%, reducing delays and rework costs |
Staff Scheduling | AI-driven tools predict peak hours and adjust staffing needs | Decreases overtime and underutilization, leading to a 15% reduction in staffing costs |
"By automating routine tasks with AI, healthcare organizations can focus on improving patient care, while reducing unnecessary operational expenditures."
AI in Predicting and Managing Disease Outbreaks in Healthcare Settings
In healthcare settings, the application of AI is becoming increasingly crucial in forecasting and controlling disease outbreaks. By analyzing historical and real-time data, machine learning algorithms can identify patterns and predict the likelihood of disease spread. These predictive capabilities enable healthcare facilities to take proactive measures to contain outbreaks before they escalate, ultimately saving lives and resources.
AI models utilize vast amounts of data from various sources, such as patient records, environmental factors, and even social media trends, to create accurate predictions. The use of predictive analytics can enhance decision-making in hospitals, clinics, and public health agencies, allowing them to respond swiftly and effectively to emerging health threats.
Key Methods in Disease Outbreak Prediction
- Real-time data analysis from hospital systems and electronic health records.
- Social media and news analytics for early detection of symptoms and disease trends.
- Machine learning algorithms trained on historical outbreak data to predict future occurrences.
AI’s Role in Outbreak Management
- Resource Allocation: AI can predict the demand for medical supplies and personnel, ensuring optimal use of resources during outbreaks.
- Containment Strategies: Machine learning helps in identifying high-risk areas and populations, guiding quarantine measures and targeted healthcare interventions.
- Monitoring and Reporting: AI tools automate the reporting of cases, providing real-time insights into disease spread and severity.
"Artificial intelligence allows healthcare professionals to forecast disease outbreaks more accurately, ensuring timely intervention and reducing the impact on public health."
Data Integration for Accurate Predictions
Data Source | Usage |
---|---|
Electronic Health Records (EHR) | Identifying patient symptoms, treatment responses, and demographic data to predict potential outbreaks. |
Environmental Data | Assessing climate and pollution factors that could influence the spread of infectious diseases. |
Social Media Data | Detecting early signs of disease through online discussions and reports of unusual symptoms. |
Enhancing Healthcare Analytics by Integrating AI with EHR Systems
Artificial intelligence is transforming the way healthcare providers analyze patient data, offering the potential to improve clinical decision-making and patient outcomes. By incorporating AI tools with Electronic Health Records (EHR), healthcare institutions can streamline processes, uncover valuable insights, and enhance predictive capabilities. This synergy allows for better management of patient information and supports more personalized treatment plans.
Integrating AI-driven analytics with EHR systems can help healthcare professionals identify patterns, predict disease progression, and recommend appropriate interventions. As a result, the healthcare industry stands to benefit from improved workflow efficiency and enhanced accuracy in diagnosis and treatment planning.
Key Benefits of AI-EHR Integration
- Data-Driven Insights: AI tools can analyze vast amounts of patient data to identify trends and anomalies that might otherwise go unnoticed.
- Predictive Modeling: By leveraging historical patient data, AI algorithms can forecast future health outcomes, enabling proactive care.
- Automated Administrative Tasks: AI can help reduce administrative burdens by automating routine processes such as data entry and report generation.
Challenges in AI-EHR Integration
- Data Privacy Concerns: Integrating AI into EHR systems raises issues regarding the security and confidentiality of sensitive patient data.
- Interoperability Issues: Ensuring that AI tools can seamlessly communicate with diverse EHR platforms remains a significant challenge.
- Regulatory Compliance: Healthcare providers must ensure that AI solutions comply with medical standards and regulations, including HIPAA in the U.S.
"Integrating AI with EHR systems represents a key milestone in leveraging healthcare data to improve patient care, though significant challenges such as data security and regulatory compliance must be addressed."
Impact of AI Integration on Healthcare Outcomes
AI Application | Impact |
---|---|
Predictive Analytics | Improved early detection of chronic diseases and reduced emergency room visits. |
Natural Language Processing (NLP) | Better extraction of relevant information from unstructured clinical notes. |
Clinical Decision Support Systems (CDSS) | Enhanced decision-making with real-time, data-driven recommendations for treatment. |
Addressing Privacy Concerns in AI-powered Healthcare Analytics
As artificial intelligence becomes increasingly integrated into healthcare analytics, concerns regarding the privacy and security of sensitive patient data have become more pronounced. AI technologies in healthcare leverage vast datasets, including personal medical histories, diagnostic results, and treatment plans, making it crucial to ensure that privacy is maintained throughout the data processing and analysis stages. These concerns primarily revolve around unauthorized access, data breaches, and the ethical use of personal health information.
To mitigate these risks, healthcare providers and AI developers must adopt a multi-layered approach that combines technical measures, legal frameworks, and transparent practices. Striking the right balance between maximizing the potential of AI in improving patient outcomes and protecting individual privacy is essential to ensure trust in these advanced technologies.
Key Strategies for Enhancing Privacy in AI Healthcare Analytics
- Data Encryption: All sensitive data should be encrypted during both storage and transmission to prevent unauthorized access.
- Access Control: Strict access control policies should be enforced, limiting data access to authorized personnel only.
- De-identification of Data: De-identifying personal information helps reduce the risks associated with potential data breaches while preserving the value of the data for analysis.
- Regular Audits: Ongoing security audits can help identify vulnerabilities and ensure compliance with privacy regulations.
Legal Frameworks and Ethical Considerations
- Compliance with Regulations: AI-powered healthcare systems must comply with healthcare data protection regulations such as HIPAA (Health Insurance Portability and Accountability Act) or GDPR (General Data Protection Regulation).
- Patient Consent: Obtaining explicit consent from patients for the collection, storage, and use of their data is crucial for ensuring ethical use of AI technologies in healthcare.
- Transparency in Data Use: AI developers should provide clear information to patients about how their data will be used and the potential risks involved.
"Ensuring the privacy of patient data in AI-powered healthcare analytics is not just a regulatory requirement but a critical factor in maintaining public trust and confidence in these technologies."
Impact of Privacy Measures on AI Healthcare Solutions
Privacy Measure | Impact on AI Healthcare Analytics |
---|---|
Data Encryption | Increases security and reduces the risk of data breaches. |
Access Control | Ensures that only authorized personnel have access to sensitive information. |
De-identification | Reduces privacy risks while maintaining the utility of data for analysis. |
Patient Consent | Builds trust and ensures compliance with ethical and legal standards. |