Natural Interaction Ai
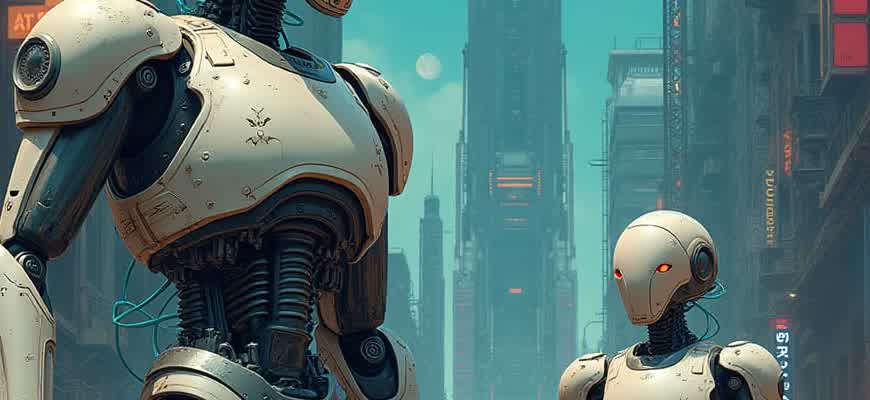
Natural interaction with artificial intelligence refers to the seamless communication between humans and machines, where the system interprets and responds in ways that feel intuitive. Unlike traditional interfaces, which rely heavily on structured input, this form of interaction leverages advanced algorithms to understand and replicate human-like conversation and actions.
The core of this technology lies in machine learning and natural language processing (NLP), enabling the AI to process and respond to verbal or written input in a meaningful way. Key components include:
- Speech Recognition - Converting spoken words into text.
- Text Analysis - Understanding context, sentiment, and intent from written text.
- Contextual Awareness - Recognizing the user's needs based on previous interactions.
"The goal of AI-driven natural interaction is not only to answer questions but to understand the deeper context, making the interaction feel more human-like and fluid."
This shift in how humans interact with machines is revolutionizing industries, from customer service to healthcare. As the AI’s ability to learn from past conversations improves, these interactions will become increasingly personalized and effective. In the next section, we will explore how these technologies are applied in real-world settings.
Technology | Applications |
---|---|
Speech Recognition | Voice assistants, transcription services |
Text Analysis | Chatbots, sentiment analysis tools |
Contextual Awareness | Personalized customer experiences, recommendation systems |
Natural Interaction AI: Enhance Your Business with Intelligent Automation
In today's fast-paced business environment, companies are turning to intelligent automation powered by natural interaction AI to streamline operations and improve customer experiences. These technologies enable seamless communication between businesses and customers, reducing manual work while ensuring efficiency and consistency in every interaction. This integration helps businesses increase productivity and reduce costs by automating routine tasks without sacrificing the quality of service.
By implementing natural interaction AI, companies can revolutionize how they engage with customers, manage processes, and make data-driven decisions. The combination of AI-powered chatbots, voice recognition, and predictive analytics allows businesses to gain valuable insights and respond to customer needs faster than ever before. Here's how businesses can benefit from this technology:
Key Benefits of Natural Interaction AI
- Customer Engagement: AI-powered solutions offer instant, personalized support, boosting customer satisfaction and loyalty.
- Operational Efficiency: Automating repetitive tasks frees up human resources to focus on more complex activities, improving overall productivity.
- Data-Driven Decisions: AI systems analyze vast amounts of data to provide actionable insights, helping businesses make informed decisions.
Practical Applications in Business
- Customer Support: Chatbots and virtual assistants provide 24/7 assistance, handling inquiries and resolving issues in real time.
- Sales Automation: AI can suggest personalized products, upsell, and cross-sell based on customer preferences and behavior.
- Supply Chain Optimization: AI algorithms predict demand and optimize inventory management, reducing costs and improving delivery times.
“Natural Interaction AI is not just about automating tasks; it’s about creating a more intelligent and responsive business environment that can adapt to the needs of both customers and employees.”
Impact on Business Growth
Integrating natural interaction AI into business processes has the potential to drive substantial growth. By reducing operational costs, increasing customer satisfaction, and enabling faster decision-making, businesses can stay ahead of the competition. Moreover, AI can continuously evolve and improve its performance over time, ensuring long-term benefits for organizations.
AI Application | Business Impact |
---|---|
Customer Support Chatbots | Improved customer satisfaction, 24/7 service availability |
Sales Automation Tools | Increased sales through personalized recommendations |
AI in Logistics | Optimized inventory and reduced operational costs |
How Natural Interaction AI Improves Customer Engagement
Natural Interaction AI has revolutionized the way businesses engage with their customers. By leveraging advanced machine learning algorithms and natural language processing, it allows for a more seamless and personalized interaction. This technology makes it possible to engage with customers in a way that feels intuitive, making the experience more satisfying for users and more effective for businesses.
One of the key benefits of Natural Interaction AI is its ability to handle a wide range of customer inquiries without the need for human intervention. This leads to faster response times, increased availability, and a higher level of customer satisfaction. The AI adapts to customer preferences over time, enabling more targeted and effective communication.
Key Features of Natural Interaction AI in Customer Engagement
- Personalization: AI can remember past interactions and tailor responses based on customer history, preferences, and behavior.
- 24/7 Availability: AI-driven systems ensure customers can get help at any time of day, offering uninterrupted support.
- Context-Aware Communication: The system understands the context of conversations, providing relevant and coherent responses.
"By improving the relevancy and speed of communication, businesses can increase their overall engagement rates and customer satisfaction."
How It Enhances Engagement: A Breakdown
Customer Need | AI Response |
---|---|
Instant support | AI provides real-time answers without delays. |
Personalized offers | AI suggests deals based on individual browsing or purchasing habits. |
Accurate answers | AI uses vast datasets to deliver precise and relevant information. |
With these capabilities, Natural Interaction AI doesn’t just assist customers–it transforms how businesses interact with their audience, creating deeper and more meaningful connections. The result is a more engaged customer base and a stronger brand presence in the market.
Optimizing Conversational Agents for Tailored User Interactions
Enhancing the user experience in chatbot interactions requires a deep understanding of the individual's preferences and needs. By leveraging user data, such as previous conversations, behavior patterns, and demographic information, chatbots can adjust their responses to create more personalized interactions. This involves shifting from generic replies to context-aware answers, thus improving overall satisfaction and engagement. The key to achieving this is through dynamic conversation models that respond and evolve based on the user’s ongoing input.
For optimal results, conversational agents must prioritize learning from interactions in real-time. This can be achieved by implementing algorithms that analyze user intent, emotional tone, and frequently asked queries. Additionally, maintaining user context throughout the conversation is crucial to avoid repetitive or irrelevant responses. By integrating feedback loops, chatbots can improve over time, creating a more natural flow in their interactions.
Key Strategies for Personalizing Chatbot Conversations
- Contextual Awareness: Maintaining an understanding of past user interactions helps provide more relevant responses.
- Emotion Recognition: Using sentiment analysis to adjust the tone and content of the conversation based on the user’s emotional state.
- Adaptive Response Models: Leveraging machine learning to continuously adjust the chatbot’s behavior to align with the user’s evolving preferences.
Steps to Achieve Personalized User Experience
- Gather User Data: Collect relevant information from previous interactions to build a profile.
- Utilize Sentiment Analysis: Implement algorithms that can detect mood and tone to adapt responses accordingly.
- Test and Optimize: Regularly evaluate the chatbot’s performance to refine its understanding of user intent.
Key takeaway: A well-optimized chatbot adapts to each user’s needs and preferences, offering a unique experience each time.
Table of Key Personalization Techniques
Technique | Purpose |
---|---|
Context Preservation | Ensures continuity of conversation and relevance of responses. |
Emotion Detection | Adjusts tone based on the user’s emotional state to foster empathy and engagement. |
Dynamic Response Generation | Offers flexible responses based on the evolving nature of the conversation. |
Reducing Response Time with AI-Powered Customer Support
In the age of instant communication, reducing response time is crucial for businesses to maintain customer satisfaction. AI-powered support systems are designed to streamline interactions by automating the first layer of communication. By using natural language processing (NLP) and machine learning, AI can instantly understand customer queries and provide relevant responses, improving overall efficiency. This ensures that customers receive quick assistance without long wait times or the need for human intervention in simple cases.
AI systems can also analyze past customer interactions and adapt to new inquiries, allowing them to handle more complex issues over time. With features like chatbots and virtual assistants, AI can manage a significant volume of support requests simultaneously. This technology not only reduces the burden on human agents but also provides businesses with valuable insights into customer behavior and pain points.
Key Benefits of AI in Customer Support
- Faster Response Times: AI can provide immediate answers to routine questions, ensuring customers aren't kept waiting.
- 24/7 Availability: AI systems operate around the clock, ensuring that support is always available, regardless of time zones.
- Cost Efficiency: Reducing the need for human agents for basic tasks can significantly lower operational costs.
How AI Reduces Response Time
- AI systems prioritize customer queries based on urgency, ensuring critical issues are handled first.
- Automated responses for common questions eliminate the need for human intervention, cutting down wait times.
- AI learns from past interactions and constantly improves its ability to handle various types of requests.
"AI-powered customer support systems have proven to reduce response times significantly, enabling businesses to scale their operations while maintaining high customer satisfaction levels."
Comparison of Response Time: AI vs. Human Support
Type of Support | Average Response Time |
---|---|
AI Chatbot | Instant |
Human Agent | 5-10 Minutes |
AI with Human Escalation | 2-3 Minutes |
Leveraging NLP for Enhanced Data Interpretation
Natural Language Processing (NLP) has become an essential tool in extracting meaningful insights from large volumes of textual data. By enabling machines to interpret and analyze human language, NLP provides an efficient method for identifying trends, sentiment, and key information buried in datasets. In industries such as healthcare, finance, and marketing, these insights can guide decision-making processes and improve operational efficiency.
One of the main advantages of using NLP is its ability to analyze unstructured data sources, such as customer reviews, social media posts, and medical records. With advanced techniques like sentiment analysis and named entity recognition (NER), NLP can transform raw text into structured data that is easily interpretable by both machines and humans. This capability enhances the accuracy of predictive models and supports data-driven strategies.
Key Benefits of NLP for Data Insights
- Automated Data Processing: NLP algorithms can automatically process vast amounts of textual data, saving time and resources compared to manual analysis.
- Improved Accuracy: By extracting relevant data points from unstructured sources, NLP reduces human error and ensures more reliable insights.
- Real-Time Analysis: NLP enables the real-time processing of data, offering up-to-the-minute insights that are crucial for timely decision-making.
Applications of NLP in Data Analytics
- Customer Sentiment Analysis: NLP can analyze customer feedback across multiple platforms, identifying overall sentiment and emerging trends.
- Financial Market Predictions: NLP can parse financial reports and news articles to predict market movements based on textual data.
- Healthcare Diagnostics: NLP helps in processing medical records, enabling more accurate diagnosis and personalized treatment plans.
"By incorporating NLP techniques, businesses can unlock deeper insights from their data, transforming raw information into actionable intelligence."
Example of NLP Application in Financial Data
Source | Textual Data | Insight Derived |
---|---|---|
Financial Reports | "The company reported a 10% increase in quarterly earnings." | Forecasted revenue growth of 8-12% based on historical data trends. |
Market News | "Stock prices surged after the merger announcement." | Positive sentiment indicating potential investment opportunities. |
Integrating Conversational AI into Existing CRM Systems
In the digital age, customer relationship management (CRM) systems have become an essential tool for businesses to manage interactions with clients. Integrating conversational AI technologies, such as natural language processing (NLP) and machine learning, into these systems can significantly enhance user experience and operational efficiency. This integration allows businesses to streamline communication, automate routine tasks, and offer personalized services, improving both customer satisfaction and internal workflows.
While the potential benefits are clear, the process of combining AI with CRM platforms can be complex. It requires a thorough understanding of both the CRM’s functionality and the AI’s capabilities to ensure seamless interaction between the two. Businesses must also consider factors like data security, scalability, and adaptability to avoid any disruption in their existing processes.
Key Benefits of Integration
- Improved Customer Support: Conversational AI can handle repetitive queries, enabling human agents to focus on more complex issues.
- Automated Data Entry: AI can automatically log customer interactions into the CRM system, reducing manual errors and saving time.
- Personalization: AI can analyze customer data and provide tailored recommendations or responses, enhancing customer satisfaction.
Challenges to Consider
- Data Compatibility: Ensuring that AI tools can access and process the data from various sources in the CRM system.
- Integration Costs: Initial setup and maintenance of the AI system may require significant investment.
- Employee Training: Staff need to be trained on how to effectively work with the AI-enhanced CRM system.
Considerations for a Smooth Integration
Factor | Consideration |
---|---|
Data Security | Ensure that customer data is encrypted and that the AI system complies with data privacy regulations. |
Scalability | The AI solution must be able to scale as the business grows, without requiring major overhauls. |
Customizability | Choose AI tools that can be tailored to your specific CRM system and business needs. |
"Integrating AI with CRM systems offers an opportunity to redefine customer engagement, driving both efficiency and satisfaction. However, it is essential to carefully plan and execute the integration to achieve the best results." - Expert Insights
Boosting Sales Conversions with AI-Driven Customer Engagement
In today's competitive market, businesses are turning to artificial intelligence (AI) to optimize their customer interactions and increase sales conversions. AI-driven tools can analyze vast amounts of data in real-time, allowing businesses to better understand customer preferences, behaviors, and needs. By leveraging AI, companies can offer personalized experiences that directly influence purchasing decisions.
AI technology has proven to be a game-changer in enhancing the quality and efficiency of customer interactions. It allows businesses to deliver tailored recommendations, automate responses, and even predict customer intent. This leads to more meaningful engagement, a streamlined sales process, and, ultimately, higher conversion rates.
Key Benefits of AI in Customer Interaction
- Personalization at Scale: AI can analyze customer data to provide highly personalized experiences, offering product suggestions based on past behaviors and preferences.
- Instant Response Time: AI-powered chatbots and virtual assistants can provide instant responses to customer queries, reducing waiting times and improving the overall experience.
- Predictive Analytics: AI tools can predict customer needs and preferences, allowing businesses to proactively offer relevant products or services, increasing the likelihood of conversion.
AI-Powered Tools for Enhancing Sales
- Chatbots and Virtual Assistants: These AI systems engage customers in real-time, answering questions, guiding them through product selections, and assisting with the checkout process.
- Recommendation Engines: By analyzing past purchase history and browsing behavior, AI can recommend relevant products, increasing cross-selling and upselling opportunities.
- Dynamic Pricing: AI can adjust pricing based on factors like demand, customer behavior, and market trends, ensuring competitive pricing that drives conversions.
Impact on Sales Conversion
"AI tools allow businesses to engage customers in a way that feels personal and efficient, directly impacting conversion rates by making the sales process smoother and more targeted."
AI Tool | Impact on Conversion |
---|---|
Chatbots | Faster response time, increased engagement, and higher satisfaction rates leading to more conversions. |
Recommendation Engines | Personalized product suggestions that increase cross-sell and upsell opportunities. |
Dynamic Pricing | Optimized pricing that drives customer decisions and boosts conversion rates. |
Building Scalable AI Solutions for High-Traffic Platforms
Designing AI systems that can handle large-scale usage is crucial for platforms that experience substantial user interaction. As user demand grows, the ability of the system to scale without compromising performance, speed, or accuracy becomes essential. High-traffic applications require a robust architecture that supports millions of requests per second, ensuring that AI services can process and respond efficiently to each interaction. Proper infrastructure planning is key to ensuring seamless operation, even during traffic spikes.
One of the fundamental aspects of scaling AI systems is the use of distributed computing, which divides workloads across multiple servers or data centers. This decentralization enables faster data processing and load balancing, ensuring that no single point of failure disrupts the service. Additionally, employing scalable machine learning models that adapt to increasing data volumes and more complex queries is essential for maintaining performance over time.
Key Strategies for Scalable AI Systems
- Microservices Architecture: Breaking down the AI system into smaller, independent components allows for better scaling and maintenance. Each microservice can be scaled individually based on demand.
- Data Partitioning: Splitting large datasets into smaller, manageable chunks ensures efficient processing and storage. This technique minimizes latency and maximizes throughput.
- Real-time Data Streaming: Implementing a real-time data pipeline for continuous data ingestion helps in keeping the AI system up-to-date with minimal delays, even with high user volumes.
Considerations for Performance and Reliability
- Load Balancing: Distributing incoming requests evenly across multiple servers ensures optimal resource utilization and prevents any server from becoming a bottleneck.
- Fault Tolerance: AI systems need to be resilient to failures. Implementing redundancy and automatic failover systems helps to minimize downtime and data loss.
- Data Caching: Storing frequently accessed data in memory speeds up response times and reduces the load on back-end systems.
"In high-traffic environments, a well-designed AI system must prioritize low-latency responses and high throughput to meet the demands of users."
Performance Metrics for Scaling AI
Metric | Description |
---|---|
Throughput | The number of requests the system can handle per second. |
Latency | The time taken to process a single request. |
Fault Tolerance | Ability of the system to recover from failures without downtime. |
Securing User Data and Ensuring Legal Compliance in AI Systems
As AI systems are increasingly integrated into daily operations, safeguarding sensitive information and ensuring adherence to legal requirements becomes critical. The interactions between users and AI-driven platforms involve vast amounts of data, which must be handled responsibly to protect privacy and maintain user trust. Therefore, ensuring data security and legal compliance is paramount for organizations deploying such technologies.
Key considerations for securing data privacy and compliance include encryption, transparent data usage policies, and a robust system for user consent management. AI systems should employ measures that minimize exposure to sensitive data and allow users to maintain control over their personal information. Compliance with regulations such as GDPR or CCPA further demands that businesses implement strict protocols regarding data handling and user rights.
Strategies for Safeguarding Data Privacy in AI Interactions
- Data Minimization: Collect only the essential information needed for the AI system to function effectively. This reduces the risk of exposing unnecessary data.
- Data Encryption: Encrypt sensitive information both in transit and at rest to prevent unauthorized access.
- User Consent Management: Clearly inform users about data collection practices and allow them to provide explicit consent before processing their data.
- Access Control: Implement role-based access controls to ensure that only authorized personnel can access sensitive data.
Ensuring Compliance with Regulations
- Regular Audits: Conduct periodic audits to ensure AI systems are in compliance with relevant regulations and industry standards.
- Right to Access and Deletion: Enable users to access their data and request deletion, in line with privacy rights under laws like GDPR.
- Data Breach Notification: Establish procedures for notifying users and authorities in case of a data breach, as required by data protection laws.
- Privacy by Design: Integrate privacy and security measures into the AI system's architecture from the beginning, rather than as an afterthought.
Organizations must implement stringent data protection measures and stay up-to-date with the evolving regulatory landscape to avoid legal risks and build user trust.
Data Protection and Compliance Frameworks
Regulation | Scope | Key Requirement |
---|---|---|
GDPR | EU and EEA | Data subject rights, consent, and transparency in data usage |
CCPA | California, USA | Consumer privacy rights, data access, and deletion requests |
PIPL | China | Personal information processing and consent management |