Ai Curriculum Automation
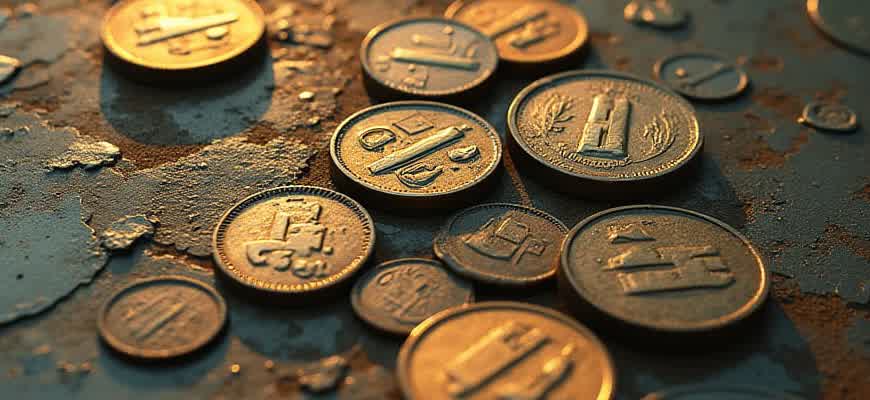
Artificial Intelligence is transforming traditional educational frameworks by enhancing the process of curriculum management. By leveraging AI-driven tools, educational institutions can streamline the creation, adaptation, and delivery of learning content. This shift allows educators to focus on more personalized teaching methods while leaving the administrative burden to automated systems.
Key Benefits of AI in Curriculum Development:
- Improved content adaptation based on individual learning styles.
- Faster identification of knowledge gaps and student performance trends.
- Optimized course delivery for diverse learner needs.
How AI Optimizes Curriculum Design:
AI systems analyze vast amounts of educational data to identify patterns and suggest necessary adjustments to the curriculum. These systems not only adapt in real-time but also predict future learning outcomes based on historical data, ensuring a proactive approach to education.
Key Elements in AI Curriculum Automation:
Element | Function |
---|---|
Content Personalization | Adapts learning materials based on individual student performance. |
Predictive Analytics | Forecasts student success and helps redesign courses accordingly. |
Automated Assessment | Provides real-time feedback and streamlines grading processes. |
AI-Driven Curriculum Optimization: Revolutionizing Content Development
As educational systems continue to evolve, the integration of artificial intelligence into curriculum design is becoming a critical factor in enhancing efficiency and customization. AI technologies are streamlining the creation of tailored learning materials, reducing the time required for manual development and ensuring content relevance to specific student needs.
By leveraging machine learning algorithms and data-driven approaches, educators can automate the process of content generation, offering a more dynamic and personalized learning experience. AI models analyze vast amounts of data from student interactions, performance metrics, and educational resources, enabling the creation of optimized curricula that are both adaptive and scalable.
Benefits of AI in Curriculum Design
- Time Efficiency: AI automates repetitive tasks, freeing up educators to focus on more complex teaching aspects.
- Personalization: AI adapts content to suit individual learning paces and preferences, ensuring a more tailored educational experience.
- Data-Driven Insights: AI identifies trends and patterns from student data, informing decisions for content improvements.
How AI Improves Content Creation
- Dynamic Content Generation: AI generates new educational resources based on current trends and gaps in existing materials.
- Automated Feedback: Instant feedback on assignments and assessments helps instructors fine-tune the curriculum in real-time.
- Adaptive Learning Paths: AI customizes learning paths, ensuring that students receive the most relevant content based on their progress.
"AI-powered curriculum design is not just about automating tasks but also enhancing educational effectiveness by personalizing learning experiences."
AI-Enhanced Content Development Workflow
Step | Description |
---|---|
Data Collection | AI collects student performance data to understand needs and gaps. |
Content Creation | AI algorithms generate customized educational content based on data analysis. |
Content Evaluation | AI evaluates content effectiveness through feedback loops and real-time adjustments. |
Implementation | Personalized curriculum is deployed to enhance student engagement and learning outcomes. |
How AI Enhances the Curriculum Design Process
AI technology has significantly transformed the way educational programs are created, offering tools that automate and improve various stages of curriculum development. By leveraging machine learning algorithms and data analytics, AI allows educators and administrators to develop more personalized, effective, and adaptive learning experiences. Through AI-powered systems, curriculum design is not only more efficient but also aligns better with the needs of diverse student populations.
AI streamlines curriculum development by automating repetitive tasks, analyzing student performance, and providing insights into curriculum gaps. This enables educators to focus more on the creative and pedagogical aspects of curriculum planning, while AI takes care of the data-heavy aspects, ensuring that the curriculum remains relevant and responsive to student needs.
Key Benefits of AI in Curriculum Development
- Data-Driven Insights: AI systems can analyze large sets of educational data to identify trends and patterns in student performance, enabling curriculum developers to make informed decisions about content delivery and instructional strategies.
- Personalization: AI enables the creation of adaptive learning paths, where curriculum content can be tailored to the individual learning pace and style of each student.
- Efficiency: By automating administrative tasks, AI reduces the time spent on curriculum planning, allowing educators to focus on enhancing the quality of their content.
AI-Driven Curriculum Development Process
- Data Collection: AI collects and analyzes data from various sources, including student performance metrics, feedback, and external learning resources.
- Gap Analysis: Machine learning models assess where the current curriculum is lacking or needs improvement based on data-driven insights.
- Content Customization: Using the data from the gap analysis, AI suggests content modifications, such as adjusting the difficulty level of materials or adding new resources to support students' learning needs.
- Ongoing Assessment: AI systems continuously monitor the effectiveness of the curriculum by tracking student outcomes, allowing for real-time adjustments.
AI's ability to automate and optimize curriculum design ensures that educational content is more relevant, personalized, and responsive to student needs, enhancing both teaching and learning outcomes.
Examples of AI Tools in Curriculum Design
AI Tool | Function |
---|---|
Content Recommendation Engine | Suggests personalized learning resources based on student preferences and performance. |
Automated Assessment Tools | Grades and provides feedback on assignments, saving time for educators. |
Predictive Analytics | Anticipates student performance trends, helping to refine instructional methods. |
Integrating AI with Existing Educational Systems
Incorporating artificial intelligence (AI) into traditional educational frameworks presents both opportunities and challenges. The seamless integration of AI tools within established systems can significantly enhance personalized learning, streamline administrative processes, and offer more dynamic curriculum options. However, doing so requires careful planning to ensure that AI complements rather than disrupts existing structures. Schools and institutions must navigate the complexities of technology adoption while maintaining the integrity of current educational practices.
The key to successful AI integration lies in identifying areas where AI can provide the most value. This involves leveraging AI to support teachers in data analysis, automate repetitive tasks, and provide personalized learning experiences for students. Additionally, AI-powered platforms can help identify gaps in students' understanding and adapt the curriculum in real time to meet diverse learning needs.
Key Strategies for Effective Integration
- Personalized Learning: AI can analyze individual student data to tailor lesson plans and assessments that match each student's learning pace and style.
- Data-Driven Decision Making: Educators can use AI to analyze trends, track student progress, and predict potential issues, allowing for more informed decisions.
- Automation of Administrative Tasks: Tasks such as grading, scheduling, and student feedback can be automated, freeing up time for instructors to focus on teaching.
Challenges to Overcome
- Infrastructure Limitations: Not all educational institutions have the technological infrastructure to support advanced AI systems, which could lead to disparities in access.
- Resistance to Change: Educators and administrators may be hesitant to adopt AI tools, fearing a loss of control or a shift in traditional teaching methods.
- Data Privacy Concerns: The collection and analysis of student data must adhere to strict privacy regulations to avoid misuse or breaches.
"AI integration should be viewed as an enhancement to education, not a replacement. The goal is to empower educators and students, not to replace human interaction."
Key Components of AI Implementation
Component | Description |
---|---|
Learning Analytics | AI tools that track and analyze student performance to provide actionable insights for teachers and administrators. |
Adaptive Learning Systems | Personalized learning environments that adjust content delivery based on real-time assessments of student progress. |
Virtual Teaching Assistants | AI-powered assistants that support teachers by handling routine tasks, answering student questions, and providing additional resources. |
Customizing Learning Paths with AI Algorithms
AI-based algorithms are revolutionizing the way personalized learning paths are created, providing students with adaptive learning experiences tailored to their individual needs. These algorithms process vast amounts of data to adjust the learning trajectory based on the user's progress, strengths, weaknesses, and learning preferences. By analyzing patterns in student behavior and performance, AI can create a roadmap that optimizes learning efficiency and improves outcomes.
Incorporating machine learning into curriculum design allows for continuous refinement of educational experiences. Through real-time feedback and data analysis, AI can help educators identify areas where students are struggling and suggest personalized resources to address knowledge gaps. This creates a dynamic learning environment where paths evolve as students progress, ensuring a more engaging and effective educational journey.
Key Components of AI-Driven Learning Paths
- Data Collection: Continuous tracking of student interactions, test scores, and engagement metrics.
- Personalized Recommendations: AI systems suggest tailored resources and activities based on individual learning needs.
- Adaptive Learning Systems: Learning paths adjust based on real-time feedback, offering the right challenges at the right time.
- Real-Time Monitoring: AI monitors student progress and makes adjustments to learning materials or strategies when needed.
Advantages of AI-Powered Learning Paths
- Efficiency: Students learn more effectively by focusing on their weak areas and skipping content they have already mastered.
- Scalability: AI enables the creation of individualized paths for a large number of students without requiring additional manual effort.
- Engagement: Tailored content and personalized feedback keep students more engaged and motivated to continue learning.
Example of a Personalized Learning Path
Phase | Activity | Goal |
---|---|---|
Initial Assessment | Diagnostic test | Identify strengths and weaknesses |
Learning Path Creation | AI-generated recommendations | Provide a customized sequence of lessons |
Ongoing Evaluation | Progress tracking | Adjust content based on student performance |
"AI-powered learning paths provide students with a personalized roadmap that evolves based on their individual progress, ensuring a more effective learning experience."
Ensuring Scalability in AI-Driven Curriculum Automation
As educational institutions increasingly turn to AI for curriculum development, ensuring scalability becomes a critical factor. AI-driven systems must be able to handle growing student populations, diverse learning paths, and evolving educational standards. Without scalable infrastructure, AI tools may fail to deliver consistent, personalized learning experiences across large-scale deployments.
The key to scalability lies in designing AI systems that are both adaptable and robust. This requires a combination of flexible algorithmic frameworks, cloud-based solutions, and efficient data management systems. Proper scalability ensures that as the user base grows, the system can maintain high performance and reliability without compromising the quality of education delivered.
Key Factors for Achieving Scalability
- Cloud Integration: Leveraging cloud computing for storage and processing allows AI systems to scale dynamically based on user demand.
- Modular Frameworks: Developing AI models in modular components ensures that different parts of the curriculum can be updated or expanded without disrupting the entire system.
- Data-Driven Optimization: Continuously analyzing and optimizing data pipelines ensures the AI remains efficient as it handles increasing amounts of student data.
Important: Scalability not only involves technological infrastructure but also the ability to adjust AI models to suit varying educational contexts across different regions and curricula.
Strategies for Effective Scaling
- Distributed Computing: Using distributed computing networks helps balance the load and minimizes performance degradation during peak usage times.
- Adaptive Learning Paths: Creating AI systems that adapt to individual students’ needs allows them to personalize the learning experience at scale.
- Regular Updates and Maintenance: Routine updates to AI models and educational content ensure the system stays relevant and high-performing.
Strategy | Benefit |
---|---|
Cloud Integration | Scalable storage and processing capacity. |
Modular Frameworks | Easy updates and customization. |
Data-Driven Optimization | Efficient handling of growing datasets. |
Leveraging Data for Personalizing Educational Experiences
Data-driven approaches in education can significantly enhance the learning experience by tailoring content to individual student needs. By collecting and analyzing various data points, institutions can adjust the pace, difficulty, and delivery of materials to better align with each learner's progress and preferences. This process not only boosts engagement but also improves retention rates and outcomes.
One of the primary ways data is utilized in personalizing education is through real-time feedback systems that monitor student performance. These systems can track a learner’s strengths, weaknesses, and engagement levels, providing educators with valuable insights to refine their teaching strategies and create customized learning paths.
Key Data Points for Personalization
- Student performance history
- Learning style preferences
- Real-time interaction data (e.g., time spent on tasks, completion rates)
- Behavioral patterns and engagement trends
Methods for Implementing Personalization
- Adaptive Learning Systems: These systems adjust content in real-time based on the learner's pace and performance.
- Intelligent Tutoring: AI-driven tutors provide personalized guidance and feedback tailored to individual student needs.
- Predictive Analytics: Data is used to predict student outcomes and recommend interventions before performance issues arise.
"By leveraging data, educational institutions can transform traditional one-size-fits-all approaches into dynamic, student-centered experiences."
Benefits of Data-Driven Personalization
Benefit | Impact |
---|---|
Enhanced Student Engagement | Increased motivation and active participation in learning. |
Improved Learning Outcomes | Higher retention rates and academic performance. |
Efficient Resource Allocation | More targeted support for students who need it most. |
Reducing Manual Work: How AI Saves Time for Educators
AI has become a powerful tool for educators by automating several tasks that would otherwise consume a large portion of their time. From grading assignments to creating personalized learning plans, artificial intelligence streamlines many of the administrative processes in education. This allows teachers to focus more on student interaction and less on repetitive, time-consuming activities.
By leveraging machine learning algorithms and data-driven approaches, AI can manage tasks such as content generation, assessment, and even classroom management, reducing the need for manual input. This not only enhances efficiency but also improves the quality of education by providing teachers with valuable insights into student performance and progress.
Key Benefits of AI in Reducing Educator Workload
- Automated Grading: AI systems can quickly grade assignments, quizzes, and exams, providing instant feedback to students. This frees up valuable time for educators to engage in more personalized instruction.
- Content Customization: AI can generate tailored learning materials based on individual student needs, ensuring that each learner receives content suited to their progress.
- Classroom Analytics: AI can analyze student behavior and engagement, providing teachers with insights that help in optimizing teaching methods and classroom strategies.
Examples of AI Tools Used in Education
- Automated Essay Scoring: AI systems that evaluate written responses with high accuracy, saving educators hours of grading time.
- Chatbots: AI-powered virtual assistants that can answer student questions, offer support, and manage scheduling without teacher intervention.
- Learning Management Systems (LMS): AI-integrated platforms that adapt the learning path for each student based on their strengths and weaknesses.
"AI enables educators to focus on what truly matters – student interaction – by removing the burden of administrative and grading tasks."
Impact on Time Management
Task | Time Saved with AI |
---|---|
Grading Assignments | Up to 80% of the time spent manually grading |
Creating Personalized Content | Automated content generation in minutes |
Classroom Monitoring | Automated insights in real-time, reducing time spent on observation |
Addressing Challenges in Implementing AI-Based Solutions in Education
Integrating artificial intelligence into educational systems presents numerous challenges that need to be addressed for effective implementation. One of the primary obstacles is the disparity in technological infrastructure across institutions. Many schools and universities still lack the necessary hardware and software to support advanced AI systems, which can lead to uneven access to AI-driven learning tools. Additionally, a lack of digital literacy among educators and students further exacerbates this problem, hindering the adoption of AI technologies in classrooms.
Another significant challenge is the concern over data privacy and security. AI systems require large datasets to function effectively, which often includes personal and sensitive information about students. Protecting this data from breaches while ensuring its ethical use is a critical issue. Furthermore, biases present in training data can result in AI tools that perpetuate existing inequalities, making it essential to ensure that AI algorithms are transparent and fair.
Key Challenges to Overcome
- Technological Infrastructure: Insufficient hardware and software in educational institutions.
- Digital Literacy: Lack of knowledge and skills to operate AI tools among teachers and students.
- Data Privacy and Security: Protecting personal information and ensuring ethical data use.
- Algorithmic Bias: Ensuring AI systems do not perpetuate discrimination or inequality.
Strategies for Overcoming Challenges
- Investing in Technology: Schools and universities should allocate resources towards upgrading their infrastructure, ensuring access to the latest AI tools.
- Training Educators: Providing comprehensive training programs for teachers to improve their understanding of AI applications.
- Enhancing Data Protection: Developing strict policies and safeguards to protect student data and prevent breaches.
- Eliminating Bias: Regularly auditing AI systems to detect and eliminate biases, promoting fairness in learning environments.
"For AI to be successfully integrated into education, we must prioritize fairness, transparency, and the responsible use of data."
AI Implementation Framework
Challenge | Solution |
---|---|
Technological Gaps | Invest in hardware and software upgrades. |
Data Privacy Issues | Implement strong encryption and data protection policies. |
Teacher Readiness | Offer specialized AI training and resources for educators. |
Bias in AI | Regular audits and adjustments to AI models to ensure fairness. |
Assessing the Effect of AI on Student Engagement and Academic Performance
Integrating artificial intelligence into educational curricula is transforming the way students interact with learning materials. By automating certain aspects of the learning process, AI tools provide personalized experiences that cater to individual needs, potentially increasing student engagement. However, measuring the true impact of these technologies requires a clear understanding of both qualitative and quantitative factors affecting student performance and participation. Factors like time spent on learning platforms, completion rates, and the quality of student interactions with AI-driven resources can offer insights into how AI impacts learning engagement.
Furthermore, understanding the relationship between AI-enhanced education and learning outcomes is crucial for evaluating its effectiveness. Are students retaining knowledge more effectively? Do AI tools contribute to better academic performance? To gauge this, assessments need to focus on both immediate feedback and long-term academic achievements. Schools and universities can benefit from incorporating both data-driven metrics and qualitative feedback to get a comprehensive view of AI’s impact.
Key Factors for Measuring AI Impact
- Engagement Levels: Tracking time spent interacting with AI-based learning tools, participation in discussions, and the frequency of revisiting content.
- Completion and Achievement Rates: Comparing student success rates before and after the implementation of AI systems, such as completion rates of assignments or projects.
- Personalized Learning Outcomes: Evaluating how well AI adapts to individual learning styles and how it affects the retention and application of knowledge.
- Student Feedback: Gathering insights from students regarding their satisfaction with AI tools and how these tools align with their learning preferences.
Methods of Evaluation
- Data Analytics: Analyzing behavioral data such as time spent on tasks and interaction frequency with AI-driven content.
- Pre and Post-Assessments: Conducting standardized tests before and after the introduction of AI tools to measure improvements in academic performance.
- Qualitative Surveys: Gathering student opinions through surveys and interviews to assess how AI impacts motivation, engagement, and the perceived value of their learning experiences.
AI Impact on Learning Outcomes: A Sample Comparison
Metric | Before AI Integration | After AI Integration |
---|---|---|
Completion Rate | 65% | 85% |
Average Test Score | 75% | 80% |
Student Engagement (Hours per Week) | 5 | 8 |
Important: Although AI technologies have shown positive effects in certain areas, the overall impact varies depending on the specific implementation and the demographic of students using these tools.