Ai in Higher Education Survey
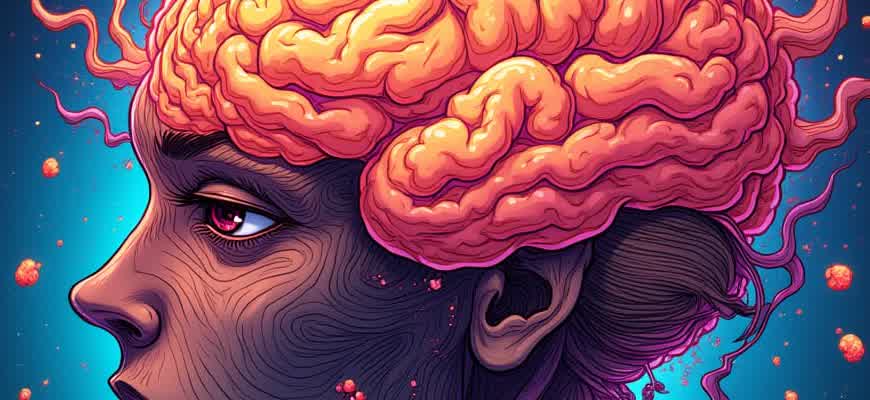
The application of artificial intelligence (AI) in academic institutions has gained significant traction in recent years, reshaping both teaching and administrative processes. Universities across the globe are adopting AI-driven solutions to enhance the student experience, improve efficiency, and foster innovation. This report presents insights into the current state of AI implementation in higher education, analyzing its impact on various academic sectors.
Key Areas of AI Adoption in Universities
- Personalized learning systems
- Automated grading and assessment tools
- AI-driven academic advising
- Data analytics for student performance monitoring
Survey Findings on AI Use in Higher Education
Technology | Usage Rate | Impact on Students |
---|---|---|
AI Tutors | 55% | Improved academic performance |
Grading Automation | 65% | Faster feedback and reduced bias |
Predictive Analytics | 50% | Better retention and graduation rates |
"AI is not just about enhancing education but transforming the way institutions operate and engage with students, paving the way for more personalized, accessible, and efficient learning experiences."
Artificial Intelligence in Higher Education Survey
In recent years, the integration of artificial intelligence (AI) in higher education has sparked a significant transformation in teaching and learning methods. As institutions worldwide explore the potential of AI technologies, understanding their impact on students, faculty, and administrative processes is essential. Surveys conducted within academic environments provide crucial insights into how AI is shaping the educational landscape, offering a comprehensive look at its effectiveness, challenges, and future prospects.
These surveys often focus on a variety of aspects, including the adoption of AI tools for personalized learning, automated grading systems, and AI-driven administrative functions. By analyzing the responses from various stakeholders, educational institutions can make informed decisions about further AI integration and its alignment with academic goals.
Key Findings of AI in Education Surveys
- Personalized Learning: AI technologies are increasingly used to create tailored educational experiences for students, allowing for customized learning paths and assessments.
- AI in Administrative Tasks: Many institutions report that AI has helped streamline administrative functions such as scheduling, student performance tracking, and resource allocation.
- Faculty Support: AI tools offer instructors new methods for evaluating student progress, identifying learning gaps, and automating routine tasks.
Challenges Identified:
- Data Privacy Concerns: Many respondents express concern over the security of personal data used by AI systems, especially in sensitive academic environments.
- Resistance to Change: Some faculty members and administrators show reluctance to adopt AI, citing fear of job displacement or skepticism about the technology’s effectiveness.
"AI has the potential to enhance educational experiences, but it must be implemented with a clear understanding of its ethical implications, particularly regarding data privacy and algorithmic bias."
Survey Results Overview:
Aspect | Positive Feedback | Negative Feedback |
---|---|---|
Personalized Learning | 65% of respondents agree AI provides valuable individualized learning experiences. | 15% worry AI cannot replace human interaction in education. |
Administrative Efficiency | 70% report AI improves efficiency in administrative tasks. | 10% cite integration costs as a barrier. |
Faculty Support | 60% of instructors see AI as a valuable tool for grading and feedback. | 20% feel AI reduces their role in the classroom. |
Understanding the Current Landscape of AI Integration in Universities
The use of artificial intelligence (AI) in higher education has seen significant growth over the past decade, driven by advancements in machine learning, data analytics, and natural language processing. Universities are increasingly adopting AI technologies to enhance educational outcomes, streamline administrative processes, and foster innovative research. As AI tools become more accessible, universities are exploring ways to integrate these technologies into various facets of academic life, from teaching and learning to student support services and research capabilities.
AI’s role in academia is evolving rapidly, with both opportunities and challenges for institutions. On one hand, AI offers the potential to personalize learning experiences, automate administrative tasks, and improve student engagement. On the other hand, institutions face challenges in terms of infrastructure, training, and ethical considerations. Understanding the current landscape of AI in higher education involves examining how universities are implementing these technologies, what barriers they face, and how they are adapting to a changing technological environment.
Key Areas of AI Integration in Universities
- Learning and Teaching: AI-powered tools, such as intelligent tutoring systems, personalized learning platforms, and automated grading systems, are enhancing the educational experience by tailoring content to individual students’ needs.
- Administrative Functions: Universities are utilizing AI for scheduling, resource management, and student admissions, reducing the workload on staff and improving efficiency.
- Research: AI is helping researchers analyze large datasets, identify patterns, and make predictions, thereby accelerating the pace of academic discovery.
Challenges in AI Adoption
- Infrastructure: Many institutions struggle with the necessary hardware and software infrastructure to support large-scale AI implementation.
- Faculty Training: Educators may lack the knowledge or skills to effectively integrate AI tools into their teaching methods.
- Ethical Concerns: There are ongoing debates surrounding privacy, bias, and fairness in AI systems, which raise questions about their impact on students and faculty.
“AI is not just about technology; it’s about reshaping how we approach education, learning, and research in profound ways.”
Current Statistics on AI Adoption in Universities
AI Technology | Adoption Rate (%) | Primary Use |
---|---|---|
AI Tutoring Systems | 45% | Personalized Learning |
Automated Grading Tools | 30% | Assessment |
AI in Research | 60% | Data Analysis |
Impact of AI Technologies on Curriculum Design and Delivery
AI technologies are rapidly transforming how educational content is designed and delivered across higher education institutions. By leveraging machine learning, natural language processing, and data analytics, educators are now able to tailor learning experiences that align with individual student needs, enhancing both engagement and academic success. As these tools evolve, they are enabling more personalized approaches to instruction and making the educational process more efficient and adaptable.
One of the most significant impacts of AI in curriculum development is the ability to create adaptive learning environments. These systems use real-time data to adjust the difficulty of tasks or suggest personalized resources, ensuring that students are constantly challenged without being overwhelmed. Moreover, AI-driven platforms offer teachers valuable insights into student performance, allowing for more informed decisions about course adjustments.
AI's Role in Designing and Delivering Curriculum
- Personalized Learning Pathways: AI tools analyze student performance and customize learning paths to fit individual needs, ensuring a more personalized and effective learning experience.
- Automated Assessments: Machine learning algorithms are used to automatically grade assignments and quizzes, freeing up instructors to focus on more complex tasks like facilitating discussions or providing individualized feedback.
- Curriculum Optimization: Data analytics are utilized to monitor course effectiveness, allowing educators to refine the curriculum based on real-time student data.
- Content Recommendation: AI systems suggest relevant learning materials such as articles, videos, or exercises, enhancing the depth of knowledge for students based on their interests and academic progress.
“AI is not just a tool for automating tasks but a partner in creating a more responsive and adaptive learning environment that fosters deeper engagement and better learning outcomes.”
Example AI Applications in Higher Education
AI Tool | Purpose | Impact |
---|---|---|
Intelligent Tutoring Systems | Provide personalized feedback and learning paths. | Improves student retention and understanding of complex topics. |
AI-Based Content Generators | Automatically generate quizzes, essays, or practice exercises. | Reduces instructor workload and ensures a continuous stream of learning materials. |
Learning Analytics Tools | Track student progress and identify at-risk students. | Enables proactive intervention and personalized support. |
Assessing AI's Influence on Student Learning and Engagement
Artificial Intelligence (AI) has made a significant impact in the realm of higher education, particularly in the ways it influences student learning outcomes and engagement. AI-powered tools are transforming the traditional classroom environment, enabling personalized learning experiences and improving overall academic performance. By analyzing vast amounts of data, AI can identify students' strengths and weaknesses, providing instructors with insights to tailor their teaching strategies accordingly. Furthermore, AI has introduced various interactive learning platforms that actively engage students and foster deeper understanding of course material.
However, the true extent of AI's impact on student success is still under evaluation. Some studies indicate that when integrated effectively, AI tools can enhance motivation and engagement, while others point to concerns about the over-reliance on technology. The key question remains: how do these AI applications influence student outcomes and engagement in the long term? To address this, several factors must be considered, including the type of AI technology used, the nature of the course content, and the level of faculty involvement in utilizing AI tools.
Factors Affecting AI's Impact on Learning Outcomes
- Personalization: AI enables adaptive learning platforms that adjust content and pacing to match individual student needs, promoting mastery of topics.
- Real-time Feedback: AI systems provide immediate feedback, which helps students identify errors and correct them quickly, improving retention and understanding.
- Engagement Tools: Interactive AI applications, such as chatbots and gamified learning platforms, increase student participation and foster a more engaging learning environment.
Challenges and Concerns
"While AI holds great promise, it is essential to address issues such as data privacy, bias in algorithms, and potential disengagement from traditional learning methods."
- Data Privacy: The use of AI often requires collecting vast amounts of personal data, which raises concerns about security and student privacy.
- Bias: AI algorithms may unintentionally reinforce biases, leading to unequal learning experiences for different student demographics.
- Human Element: Over-reliance on AI could diminish face-to-face interaction, potentially hindering the development of critical thinking and communication skills.
AI's Role in Enhancing Student Engagement
AI Tool | Impact on Engagement |
---|---|
Adaptive Learning Platforms | Increases personalized interaction with course material, keeping students more engaged and motivated. |
Virtual Assistants | Provide immediate responses to student queries, ensuring a seamless learning experience. |
Gamified Learning Systems | Enhances engagement by integrating game-like elements, making learning more enjoyable and motivating. |
Implementing AI Tools for Administrative Tasks: Opportunities and Challenges
The integration of artificial intelligence (AI) tools into the administrative tasks of higher education institutions presents both significant opportunities and notable challenges. AI technologies can automate repetitive tasks, streamline workflows, and enhance decision-making processes, leading to increased efficiency and reduced operational costs. For instance, AI can be used for student admissions, scheduling, grading, and resource management, transforming how institutions manage their day-to-day operations.
However, despite the advantages, the adoption of AI in administrative functions is not without obstacles. Institutions must address concerns related to data privacy, security, and the need for staff retraining. Furthermore, there are challenges in ensuring the quality of AI-driven processes, as well as the potential resistance from faculty and staff who may be apprehensive about the role of AI in their work environments.
Opportunities
- Efficiency Gains: AI can automate time-consuming tasks, such as processing applications, answering routine queries, and managing schedules, freeing up staff to focus on more strategic activities.
- Data-Driven Decisions: AI tools can analyze large datasets to provide insights that assist in decision-making, whether it be in admissions, budgeting, or academic planning.
- Personalization: AI systems can offer personalized experiences for students and staff by recommending courses, resources, or professional development opportunities based on data analysis.
Challenges
- Data Privacy Concerns: Handling sensitive student data raises concerns about potential breaches and misuse. Institutions must ensure robust security protocols are in place to protect information.
- Staff Resistance: Faculty and administrative staff may be reluctant to adopt AI systems due to fears of job displacement or unfamiliarity with new technologies.
- Quality Assurance: AI tools may require continuous monitoring and calibration to ensure that their outputs remain accurate and aligned with institutional goals.
"The successful implementation of AI tools in administrative tasks requires a balanced approach, addressing both the technological potential and the human factors involved."
Key Considerations
Consideration | Impact |
---|---|
Data Privacy | Ensuring compliance with regulations and protecting sensitive information is crucial to maintain trust. |
Training and Support | Effective training for staff ensures smooth integration of AI tools into existing workflows. |
Cost of Implementation | Initial investment in AI technology may be high, but long-term benefits include cost savings and efficiency improvements. |
AI-Driven Evaluation Methods: Real-World Applications and Case Studies
The integration of artificial intelligence into the assessment processes in higher education has opened up new possibilities for both students and educators. AI-powered tools are being used to streamline grading, provide instant feedback, and offer more personalized learning experiences. These advancements not only save time but also ensure greater consistency and objectivity in evaluating students' performance. Many institutions are already experimenting with AI to improve efficiency, scalability, and the overall educational experience.
AI-based assessment technologies encompass a range of tools that can analyze written content, solve complex problems, and even evaluate creative outputs. Real-world applications are evident in various case studies where institutions have successfully implemented AI for assessment. From automated essay grading to adaptive learning platforms, these innovations are changing how academic performance is measured and how students engage with course materials.
Real-World Case Studies
- University of Michigan - Utilized AI algorithms to assess large-scale student surveys, identifying key trends and providing actionable insights into learning behaviors.
- University of Edinburgh - Deployed AI-powered tools for automated grading of essays, improving grading consistency while allowing instructors to focus on higher-order tasks.
- Georgia Tech - Implemented an AI-driven platform to provide real-time feedback on coding assignments, which helped students improve their programming skills faster.
Types of AI in Educational Assessment
- Automated Grading Systems: AI tools that use natural language processing (NLP) and machine learning algorithms to grade written responses, essays, and short answers.
- Adaptive Learning Platforms: AI systems that adjust the difficulty level of questions or content based on a student's performance, providing personalized learning experiences.
- Plagiarism Detection: Advanced AI systems can detect plagiarism by analyzing writing patterns and comparing them with a vast database of sources.
"AI-powered assessments are changing the way educational institutions approach grading. These tools are not just time-saving but also provide more accurate, unbiased, and scalable solutions to traditional evaluation methods."
AI Assessment Outcomes
Outcome | Impact |
---|---|
Efficiency | AI tools streamline the grading process, enabling quicker turnaround times and freeing up instructors to focus on personalized student support. |
Consistency | AI eliminates human biases, ensuring a more objective evaluation process across large cohorts of students. |
Scalability | AI-powered systems allow institutions to assess larger numbers of students without compromising quality. |
Challenges in Scaling AI Solutions Across Different Educational Institutions
Implementing AI solutions in educational institutions poses a unique set of challenges when it comes to scaling across various types of organizations. While large universities with substantial resources may have the infrastructure to support AI applications, smaller colleges and community institutions often struggle with the financial and technical requirements. The gap between institutions with high technological capabilities and those without leads to disparities in how AI is adopted and integrated into curricula.
Another major obstacle is the diversity of needs across different educational levels and regions. Each institution has distinct priorities and approaches to teaching, creating difficulty in developing standardized AI tools that cater to a broad range of educational contexts. Addressing these challenges requires a tailored approach that considers not only technological factors but also the specific educational goals of each institution.
Key Challenges in Scaling AI Solutions
- Resource Allocation: Larger universities have the financial and human resources to invest in cutting-edge AI tools, while smaller institutions often struggle to fund such initiatives.
- Technological Infrastructure: Institutions with outdated IT systems may find it difficult to integrate AI solutions that demand high-performance computing power.
- Data Privacy Concerns: Different institutions may have varying policies regarding the handling of student data, which complicates the use of AI tools that rely on personal data analysis.
- Faculty Training: Successful AI adoption requires proper training for educators, which is often lacking due to time and budget constraints.
- Customization for Different Needs: Educational institutions have distinct needs, making it hard to create AI solutions that serve all sectors effectively.
Factors Influencing AI Adoption in Different Institutions
- Institutional Size: Larger institutions have more resources to experiment with and implement AI, while smaller ones face greater financial constraints.
- Technological Maturity: Institutions with advanced tech infrastructures are better positioned to integrate AI solutions effectively.
- Government Regulations: Regional differences in educational policies and AI regulations can impact the adoption rate of AI in different areas.
- Faculty Acceptance: The level of comfort and willingness among faculty to adopt AI in teaching and learning processes significantly influences its implementation.
"The scaling of AI tools in educational settings demands a careful balance between innovation and practicality, especially when different institutions have varying levels of readiness and capacity."
Comparative Challenges Across Institutions
Challenge | Large Universities | Smaller Institutions |
---|---|---|
Financial Resources | Ample funding for AI research and tools | Limited budgets, fewer AI investments |
Technological Infrastructure | Advanced computing resources | Outdated systems, limited technical support |
Faculty Training | Dedicated training programs for AI tools | Lack of professional development in AI |
Data Security | Robust data management protocols | Potential gaps in data security measures |
Privacy, Security, and Ethical Issues in AI-Enhanced Education Platforms
The integration of artificial intelligence in higher education systems has brought numerous benefits, but it also raises concerns regarding privacy, data security, and ethics. As AI systems gather and process personal data from students, educators, and administrative staff, the risk of unauthorized access or misuse of sensitive information becomes significant. Institutions must balance the advantages of AI-powered tools with the need to protect the privacy of individuals involved.
Additionally, the ethical implications of AI in education demand careful consideration. Automated decision-making processes can unintentionally perpetuate biases or make flawed judgments based on incomplete data. Moreover, AI-driven educational technologies may inadvertently exacerbate inequalities, particularly when they rely on datasets that do not adequately represent diverse groups of learners.
Privacy and Data Security
The use of AI systems in educational settings often involves collecting large amounts of personal and academic data. This information can include sensitive details such as students' academic performance, behavioral data, and even personal identifiers. Safeguarding this data against breaches and unauthorized use is crucial for maintaining trust between students and educational institutions.
Important: Ensuring secure storage, transmission, and access control is paramount to protect against data breaches.
- Data encryption during transmission and storage
- Limiting access to data based on roles
- Regular security audits and vulnerability assessments
Ethical Considerations in AI in Education
AI systems must be designed to prioritize fairness and inclusivity. However, if not carefully implemented, they may inadvertently introduce biases that affect certain groups of students more than others. These biases may stem from the data used to train AI models, which can reflect existing societal prejudices.
- Bias in AI models: AI systems trained on non-representative datasets may reinforce discriminatory patterns.
- Decision-making transparency: Educators and students should be able to understand how AI systems arrive at certain conclusions.
- Accountability: Institutions should ensure that AI-driven decisions are subject to human review and oversight.
Concern | Implication | Solution |
---|---|---|
Data Privacy | Risk of sensitive student information being exposed or misused | Data encryption and strict access controls |
Bias in AI Models | Unfair treatment of specific student groups | Ensuring diverse and representative datasets |
Lack of Transparency | Students and educators may not understand AI decision-making | Clear explanations and visibility into AI processes |