Ai for Writing Teacher Evaluations
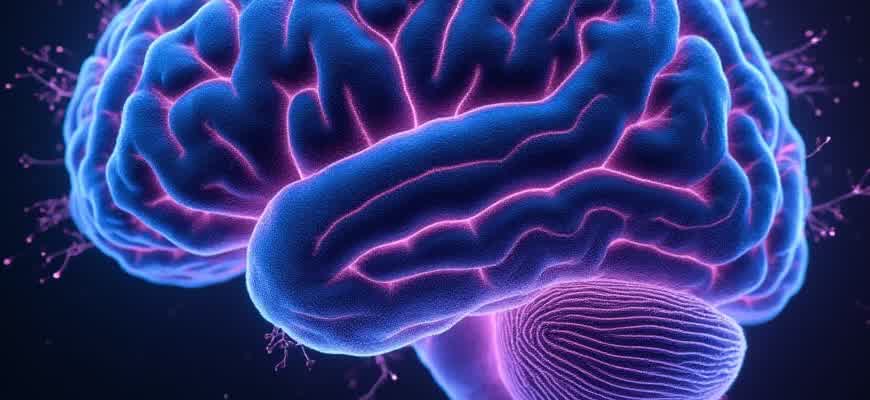
Artificial intelligence has increasingly become an essential tool for streamlining the process of teacher performance evaluations. AI algorithms can analyze vast amounts of classroom data, providing a more objective and comprehensive approach to assessing educators. By processing both quantitative and qualitative inputs, these systems can generate evaluations that are more detailed and personalized, helping institutions improve their feedback mechanisms.
AI-based systems can help administrators identify key trends in teaching effectiveness, student engagement, and overall educational outcomes. These systems not only track academic progress but also assess pedagogical approaches, allowing for a more holistic review. Below are some core advantages of using AI in teacher evaluations:
- Increased Objectivity: AI minimizes human bias by relying on data-driven insights.
- Time Efficiency: Automates repetitive tasks, reducing the time spent on manual evaluations.
- Personalized Feedback: Delivers tailored recommendations based on individual teacher performance.
- Comprehensive Analysis: Considers a wide range of factors, such as classroom dynamics, student feedback, and learning outcomes.
In the next section, we will explore how AI tools are implemented within educational institutions to enhance teacher evaluations and improve the overall teaching quality.
"AI can transform the way we assess teacher performance by providing actionable insights, ensuring that evaluations are not only fair but also constructive."
Key components of an AI-driven evaluation system often include:
Component | Description |
---|---|
Data Collection | AI gathers data from various sources like student surveys, test scores, and teacher behavior metrics. |
Analysis | The system processes data to identify trends and generate performance reports. |
Feedback Generation | Personalized feedback is automatically provided to teachers based on the analysis. |
AI for Writing Teacher Evaluations
In recent years, the integration of artificial intelligence into educational assessments has gained considerable attention. AI offers the potential to revolutionize the way teachers are evaluated for their writing skills, providing a more objective and data-driven approach. By analyzing vast amounts of writing samples, AI can detect patterns in writing quality, consistency, and effectiveness, ultimately aiding in more accurate and fair evaluations.
Unlike traditional evaluation methods that rely heavily on human judgment, AI tools can assess a variety of writing criteria, such as grammar, style, and overall coherence. These tools can quickly process and assess multiple writing pieces, offering timely feedback to both instructors and administrators. However, there are important factors to consider, including the ethical implications of using AI and the need for clear guidelines in its implementation.
Key Advantages of AI in Teacher Evaluations
- Objectivity: AI can reduce human bias by focusing solely on quantifiable aspects of writing, such as grammar, sentence structure, and vocabulary usage.
- Efficiency: AI can process large amounts of data quickly, allowing for real-time evaluations and feedback.
- Consistency: AI systems apply the same criteria across all evaluations, ensuring consistency in grading and assessment.
Challenges and Considerations
While AI offers numerous benefits, it also raises concerns about its ability to fully capture the nuances of teaching and writing. AI systems may struggle to assess creativity, context, and the unique voice of individual educators.
- Complexity of Human Writing: AI may not always understand the context or purpose of a writing piece, leading to inaccurate assessments.
- Privacy Concerns: The use of AI in evaluations requires careful handling of sensitive data, ensuring that teachers' work is protected.
- Dependence on Algorithms: Over-reliance on AI may result in a lack of nuanced feedback from human evaluators, which could hinder professional development.
Comparison of AI Evaluation Tools
Tool | Key Features | Advantages | Challenges |
---|---|---|---|
Grammarly | Grammar checking, style suggestions, readability analysis | Quick feedback, easy to use | Limited to surface-level feedback |
Turnitin | Plagiarism detection, writing analysis | Ensures originality, comprehensive evaluation | May miss nuanced writing skills |
ProWritingAid | Detailed reports on grammar, readability, and structure | In-depth feedback, customizable | Can be overwhelming for some users |
How AI Evaluates Teacher Performance Data for Objective Assessments
AI-driven systems are increasingly being used to assess teaching effectiveness through the analysis of various performance metrics. These technologies process large amounts of data, helping to identify trends and patterns that may not be immediately apparent through traditional evaluation methods. By leveraging machine learning algorithms and natural language processing, AI can provide an objective and data-driven overview of a teacher’s impact on student outcomes, classroom engagement, and instructional quality.
To ensure fairness and minimize bias, AI systems typically rely on structured data such as student grades, classroom observation reports, and feedback surveys. These data points are analyzed in a systematic manner, allowing AI to generate a comprehensive evaluation. The use of AI tools enhances consistency in evaluations by removing subjective interpretations, thus allowing for a more balanced and reliable assessment of teacher performance.
Key Areas of AI Evaluation
- Student Performance Analysis: AI examines test scores, homework completion rates, and progress over time to evaluate how effectively a teacher impacts learning outcomes.
- Classroom Interaction: AI tools can analyze audio and video recordings of classes to assess teacher-student interactions, identifying areas where communication and engagement can be improved.
- Feedback Aggregation: By processing surveys and feedback from students and peers, AI identifies recurring themes and trends, which contribute to a well-rounded evaluation.
Evaluation Process
- Data Collection: Relevant data is gathered from multiple sources, including student grades, classroom observations, and feedback surveys.
- Data Processing: AI systems clean and standardize the data, removing inconsistencies and preparing it for analysis.
- Pattern Recognition: Machine learning algorithms identify trends in student performance, teaching methods, and classroom dynamics.
- Objective Assessment: The system generates a performance report based on the analyzed data, highlighting strengths and areas for improvement.
AI offers a more consistent, data-driven approach to teacher evaluations, helping educational institutions make informed decisions based on objective insights rather than subjective judgments.
Performance Metrics Table
Metric | Data Type | Purpose |
---|---|---|
Student Grades | Quantitative | Measures academic achievement and learning progress |
Classroom Observations | Qualitative | Evaluates teaching strategies and classroom dynamics |
Student Feedback | Qualitative | Assesses student satisfaction and perceived teaching effectiveness |
Integrating AI with Existing Teacher Evaluation Frameworks
The application of Artificial Intelligence (AI) in educational assessments has shown significant potential for enhancing teacher evaluation processes. By incorporating AI tools into existing frameworks, educational institutions can streamline the collection, analysis, and interpretation of performance data. AI can assist in providing consistent, objective evaluations while also offering deeper insights into teacher effectiveness across various criteria. This integration can lead to more accurate, data-driven decisions for professional development and instructional improvement.
Traditional evaluation methods often rely heavily on subjective judgments, which can vary significantly depending on the evaluator. By leveraging AI, schools can introduce a more standardized approach, ensuring fairness and reliability. AI can analyze a wide range of data, including classroom interactions, lesson plans, student outcomes, and even teacher engagement in professional development. The result is a comprehensive evaluation system that complements the human element of feedback with data-backed insights.
Key Areas of Integration
- Data Collection and Analysis: AI can automate the collection of classroom data, including student performance metrics, teacher-student interactions, and attendance rates. This data can then be analyzed to identify trends and areas for improvement.
- Personalized Feedback: Using AI, feedback can be tailored to individual teachers, focusing on specific strengths and areas that require development. This personalization helps educators receive constructive guidance for professional growth.
- Predictive Analytics: AI-powered tools can analyze historical data to predict future teaching performance. This predictive approach can help identify potential challenges or highlight opportunities for early intervention and support.
Benefits of AI Integration
- Consistency: AI removes human biases and ensures evaluations are based on consistent criteria across all teachers.
- Efficiency: Automating data analysis and feedback generation can significantly reduce the time spent on administrative tasks, freeing up resources for more meaningful interaction.
- Informed Decisions: AI provides actionable insights derived from large datasets, enabling school leaders to make data-driven decisions regarding teacher professional development and student outcomes.
Challenges to Consider
Challenge | Solution |
---|---|
Data Privacy | Ensure compliance with data protection laws and establish secure systems for handling sensitive teacher and student data. |
Implementation Costs | Consider long-term benefits such as improved teacher performance and student outcomes to justify initial investment in AI tools. |
Resistance to Change | Provide clear communication about the benefits of AI integration and involve educators in the decision-making process to ease the transition. |
"AI is not here to replace teachers, but to empower them with the tools and insights that drive growth and improvement."
Customizing AI Models to Assess Specific Teaching Methods
When integrating AI into the process of evaluating teaching methods, it is essential to tailor the model to reflect the unique strategies and pedagogical approaches of each educator. Generic evaluation systems often fail to capture the nuances of individualized teaching styles. By customizing AI models, educators can receive more precise feedback that is aligned with their specific classroom practices and goals.
Customizing AI evaluation models involves training the system to recognize and assess the key components of different teaching methods. Whether the teacher uses a flipped classroom approach, project-based learning, or differentiated instruction, the model must be able to analyze the effectiveness of these strategies within the context of student engagement, learning outcomes, and classroom dynamics.
Key Elements for Customizing AI Models
- Data Input Customization: The AI should be trained on data specific to the teaching methods being used. This could include lesson plans, student performance data, and classroom interaction patterns.
- Evaluation Criteria: Clear criteria must be defined for each teaching method, such as student participation, critical thinking, or collaborative learning.
- Model Adaptation: The AI should be flexible enough to adapt to evolving teaching practices, providing ongoing feedback for continuous improvement.
Approaches to Tailor AI for Specific Teaching Methods
- Contextual Analysis: The model should consider the context in which the teaching method is applied, including subject matter, class size, and learning objectives.
- Real-Time Data Processing: By analyzing classroom interactions in real-time, the AI can offer immediate insights into how effectively a teaching strategy is being implemented.
- Student-Centric Evaluation: AI can assess how different teaching methods influence student engagement, retention, and satisfaction, providing a holistic view of the learning environment.
Sample Evaluation Table for Different Teaching Methods
Teaching Method | Key Criteria | AI Evaluation Focus |
---|---|---|
Flipped Classroom | Student preparation, class interaction | Assess how well students engage with pre-class materials and apply knowledge in discussions |
Project-Based Learning | Collaboration, problem-solving | Evaluate teamwork and critical thinking during projects |
Differentiated Instruction | Individualized support, student progress | Analyze how well the teacher adapts instruction to meet diverse student needs |
“Effective AI models for teaching evaluation must be designed to go beyond general metrics, allowing educators to receive feedback that is both actionable and tailored to their unique teaching methods.”
How AI Handles Subjectivity in Teacher Reviews and Feedback
AI systems are increasingly being used to evaluate teachers, offering a more standardized approach to feedback. While subjectivity is a natural part of human evaluations, it can lead to inconsistencies and biases. AI, with its ability to analyze large datasets, aims to address these issues by offering a data-driven, consistent approach to evaluating teaching performance. By integrating objective criteria with advanced algorithms, AI helps remove personal biases, providing more balanced and accurate assessments.
However, AI's ability to handle subjectivity depends on the data it has been trained on and the parameters set for its analysis. It can identify patterns that might be overlooked in human reviews, but the challenge lies in balancing human nuances with algorithmic assessments. Understanding this balance is crucial for developing fair and effective teacher evaluation systems.
AI in Teacher Evaluation: Reducing Bias and Enhancing Consistency
AI systems evaluate teacher performance using several factors, including lesson effectiveness, student engagement, and learning outcomes. These factors are typically gathered from various sources such as classroom observations, student surveys, and performance metrics. By analyzing this data, AI can identify trends and make recommendations that help improve teaching practices.
- Data-Driven Insights: AI algorithms use data from multiple sources to generate objective insights into teaching performance.
- Bias Reduction: By focusing on measurable outcomes rather than personal opinions, AI reduces the influence of individual biases in evaluations.
- Consistent Feedback: AI ensures that teachers receive consistent feedback, which is not influenced by varying human interpretations.
Challenges of Subjectivity in AI-Generated Feedback
Despite its advantages, AI still faces challenges when it comes to fully eliminating subjectivity. A key issue lies in the interpretation of qualitative data, such as open-ended comments from students or peers. AI must be trained to understand context, tone, and sentiment, which can be complex to capture through algorithms alone.
- Contextual Understanding: AI may struggle to fully grasp the context in which feedback is given, leading to misinterpretation.
- Sentiment Analysis: Understanding the emotional tone of feedback can be difficult for AI, which may miss subtleties in the language.
- Human Nuances: AI might not be able to capture the full range of human emotions and cultural differences that influence teacher assessments.
Comparing Human and AI-Based Evaluations
Aspect | Human Evaluations | AI Evaluations |
---|---|---|
Consistency | Varies depending on evaluator's mood and perspective | Consistent across all evaluations, as it relies on predefined criteria |
Bias | Subject to personal biases and assumptions | Reduces bias by focusing on objective data and predefined metrics |
Contextual Understanding | Can understand context, but may be influenced by personal experience | Struggles with complex context and sentiment nuances |
"AI systems are designed to offer more objective evaluations, yet they must be carefully calibrated to avoid overlooking human subtleties in teaching assessments."
Scaling Teacher Evaluations: AI's Role in Handling Large Data Sets
Evaluating teachers on a large scale presents significant challenges in terms of managing and analyzing vast amounts of data. Traditional methods of assessment often involve manual processes that are time-consuming and prone to human error. With the increasing number of teachers and the complexity of their performance data, it becomes essential to leverage advanced technologies to streamline the process and ensure accurate evaluations.
Artificial Intelligence (AI) has emerged as a powerful tool for automating and optimizing teacher evaluations, especially when dealing with large datasets. By utilizing AI's ability to process and analyze data quickly, it is possible to enhance the accuracy and efficiency of performance assessments, allowing for more informed decision-making and better insights into teaching quality.
AI's Impact on Data Processing in Teacher Evaluations
- Data aggregation: AI can gather data from multiple sources, including student surveys, classroom observations, and standardized test results, consolidating them into a unified system.
- Pattern recognition: AI systems can identify trends and patterns in teacher performance that might be overlooked by human evaluators, providing a deeper understanding of teaching effectiveness.
- Real-time feedback: AI can generate timely reports, enabling administrators to make immediate improvements and support for teachers when needed.
Key Benefits of AI in Large-Scale Evaluations
- Efficiency: AI drastically reduces the time needed to process large volumes of data, allowing for faster evaluations.
- Consistency: With AI, evaluations are based on predefined metrics, eliminating inconsistencies that can arise from subjective human judgment.
- Actionable insights: AI can generate data-driven insights that highlight areas of improvement for teachers, fostering a culture of continuous development.
AI offers an unparalleled advantage in processing large datasets, offering precise, unbiased assessments that human evaluators may find difficult to match in scale and consistency.
Comparison of Traditional vs. AI-Powered Evaluation Systems
Evaluation Criteria | Traditional Methods | AI-Powered Methods |
---|---|---|
Data Processing Speed | Slow, manual processing | Fast, automated analysis |
Data Consistency | Subjective, prone to errors | Objective, consistent results |
Scalability | Limited to small datasets | Highly scalable to large datasets |
How AI Reduces Bias in Teacher Evaluation Reports
Artificial intelligence can play a pivotal role in reducing bias during the teacher evaluation process. Traditional evaluation methods are often subject to human bias, whether it be conscious or unconscious, impacting the accuracy and fairness of feedback. By leveraging AI, evaluations can be more objective, ensuring that all aspects of a teacher's performance are assessed fairly and consistently. AI systems analyze large volumes of data, providing insights based on factual criteria rather than subjective opinions.
AI reduces the influence of personal biases that evaluators might unknowingly bring into their assessments. These biases can stem from factors like gender, ethnicity, or even the evaluator's mood or preferences. Through data-driven decision-making, AI ensures that evaluations are based solely on measurable outcomes, such as student performance, lesson delivery, and adherence to curriculum standards. This leads to more transparent and equitable evaluation reports, where teachers are judged for their actual performance rather than external factors.
AI’s Role in Minimizing Bias
- Objective Data Analysis: AI systems evaluate teachers using data points that are quantifiable and objective, such as test scores, student feedback, and classroom interactions.
- Consistent Standards: AI ensures that evaluation criteria remain consistent across all assessments, eliminating variations that could arise from human judgment.
- Unbiased Recommendations: AI can provide feedback and suggest improvements based on factual patterns, rather than subjective opinions or prejudices.
AI-driven evaluation systems promote fairness by focusing on performance metrics and data, minimizing the likelihood of evaluator bias affecting the outcomes.
Impact of AI on Teacher Feedback Quality
- Improved Accuracy: AI removes emotional and personal biases from evaluations, ensuring that feedback is based on facts rather than personal interpretations.
- Equal Opportunity: AI systems are impartial, offering the same evaluation standards for every teacher, regardless of their background or teaching style.
- Data-Backed Insights: Evaluators can rely on AI-generated reports that highlight areas for improvement, based on objective performance data, rather than anecdotal evidence.
Evaluation Process Comparison
Traditional Evaluation | AI-Driven Evaluation |
---|---|
Influenced by the evaluator's subjective perceptions | Based on objective, measurable data |
Possible bias based on gender, race, or personal preferences | Consistent across all teachers, regardless of background |
May lead to inconsistent feedback across teachers | Standardized evaluation ensures fairness and transparency |