Collaborative A.i. Responsibility Lab
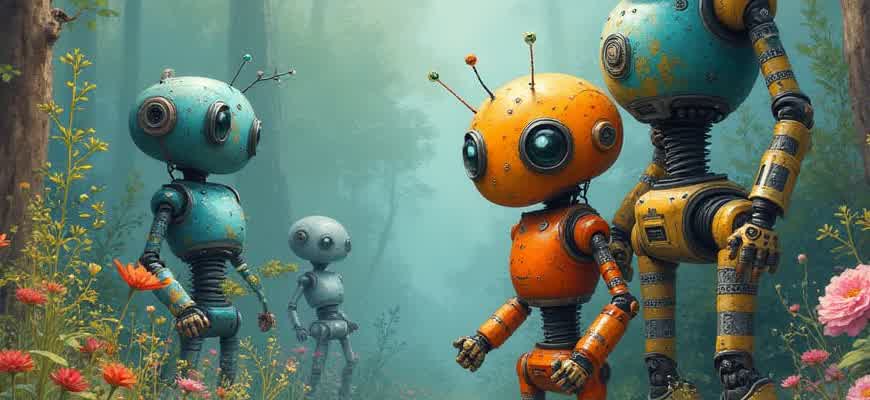
The integration of artificial intelligence (AI) into various sectors demands a structured approach to understanding its ethical implications and ensuring accountability. A dedicated research initiative focused on AI responsibility brings together interdisciplinary teams to explore methods for creating transparent, fair, and ethically sound AI systems. This collaborative environment fosters knowledge exchange and the development of best practices across industries.
Key Objectives:
- Development of ethical frameworks for AI deployment.
- Identification of risks and biases in AI algorithms.
- Promotion of accountability and transparency in AI systems.
Research Focus Areas:
- Ethical governance and policy guidelines for AI systems.
- AI transparency and decision-making processes.
- Collaborative model building with emphasis on diverse datasets.
"Incorporating ethics into AI development is not just a technical challenge, but a societal one that requires cooperation among researchers, developers, and policy-makers."
Key Challenges in AI Responsibility:
Challenge | Impact |
---|---|
Algorithmic Bias | Can lead to discrimination in automated decision-making. |
Lack of Transparency | Reduces public trust in AI technologies. |
Data Privacy | Risk of misuse or unauthorized access to personal information. |
Collaborative A.I. Responsibility Lab: Product/Service Promotion Plan
The Collaborative A.I. Responsibility Lab offers a comprehensive platform for the development and implementation of AI systems that emphasize ethics, transparency, and accountability. It is designed to support businesses, research institutions, and developers who are committed to creating AI that aligns with global standards and social expectations. This plan outlines strategies for effectively promoting this service to a wide range of potential stakeholders, from technology companies to regulatory bodies and advocacy groups.
To achieve long-term success and widespread adoption, it is essential to craft a robust promotional strategy that highlights the unique benefits of the platform while also engaging in clear and meaningful conversations about A.I. ethics. This approach will involve targeted campaigns, strategic partnerships, and educational outreach to raise awareness and foster trust in the lab's services.
Promotion Strategies
- Targeted Campaigns: Focus on specific industries, such as healthcare, finance, and manufacturing, where AI integration is rapidly increasing.
- Partnership Development: Establish collaborations with universities, research institutes, and governmental organizations to validate the lab’s credibility.
- Content Marketing: Create informative articles, whitepapers, and webinars on AI ethics and responsibility that position the lab as a thought leader in the space.
Key Channels for Promotion
- Social Media: Utilize platforms like LinkedIn, Twitter, and specialized forums to reach professionals interested in AI advancements.
- Industry Conferences: Attend or sponsor events focused on AI ethics, law, and governance.
- Collaborative Webinars: Host joint webinars with industry experts to discuss the importance of ethical AI development and responsibility.
Promotional Timeline
Phase | Activity | Duration |
---|---|---|
Phase 1 | Social Media Outreach & Content Marketing | 3 months |
Phase 2 | Strategic Partnerships & Industry Conferences | 6 months |
Phase 3 | Collaborative Webinars & Thought Leadership Campaigns | Ongoing |
"The success of any AI-driven initiative relies on transparency and shared responsibility. The Collaborative A.I. Responsibility Lab is committed to supporting the development of AI systems that are not only intelligent but also ethical and accountable."
How to Embed Ethical AI Practices in Your Organization
As AI becomes an integral part of organizational operations, it is essential to implement responsible AI practices that align with ethical standards. This ensures that AI systems are not only efficient but also transparent, unbiased, and aligned with the values of your organization. Ethical AI can help mitigate risks, build trust with customers, and ensure compliance with regulatory frameworks. It also serves to protect against potential harms that might arise from AI decisions.
To integrate responsible AI practices, an organization must adopt a structured approach, involving key stakeholders from various departments. This holistic approach ensures AI systems are designed, deployed, and monitored with a focus on fairness, transparency, and accountability. Below are key steps for embedding ethical AI principles effectively within your organization.
Steps for Integrating Ethical AI
- Establish a Cross-functional Ethics Team: Create a dedicated team consisting of AI developers, ethicists, legal advisors, and business leaders to oversee AI-related decisions.
- Implement Clear Governance Frameworks: Define policies, roles, and responsibilities to ensure AI practices align with the organization's values and regulatory requirements.
- Regularly Audit AI Systems: Set up processes to continuously evaluate AI performance, identifying and correcting any biases or errors in data and algorithms.
- Promote Transparency: Ensure AI systems are explainable, providing clear insights into how decisions are made and what data is used in the decision-making process.
"Ethical AI practices are not only about minimizing harm, but also about maximizing fairness and opportunity for all stakeholders."
Key Considerations for Successful AI Integration
- Data Integrity: Ensure that data used in AI models is clean, representative, and free from biases to avoid skewed outcomes.
- Compliance with Regulations: Stay updated with AI-related laws, ensuring that your AI implementations comply with both local and international standards.
- Continuous Employee Training: Equip your team with the knowledge of ethical AI principles, enabling them to make responsible choices throughout the lifecycle of AI systems.
AI Ethics Framework Comparison
Framework | Focus Areas | Key Principles |
---|---|---|
AI Ethics Guidelines by OECD | Accountability, Fairness, Transparency | Human rights, Inclusive growth, Environmental sustainability |
AI Ethics Guidelines by EU | Safety, Security, Accountability | Autonomy, Privacy, Non-discrimination |
Steps to Build Collaborative A.I. Systems with Ethical Guidelines
Creating AI systems that work collaboratively requires careful planning to ensure that ethical considerations are at the forefront. A well-structured approach helps in defining responsibilities, accountability, and transparent communication among all involved parties. As AI technology advances, it is crucial to establish frameworks that guide the development and deployment of these systems in a responsible manner.
By integrating ethical guidelines into AI systems, developers can ensure fairness, reduce bias, and promote trust between AI agents and human users. Here are some key steps for building AI systems with ethical considerations:
Key Steps in Building Collaborative A.I. Systems
- Define Clear Ethical Principles
- Establish a set of core ethical guidelines tailored to the specific collaborative environment.
- Focus on transparency, fairness, and accountability to minimize harm and bias.
- Ensure all stakeholders understand and adhere to these principles from the outset.
- Ensure Stakeholder Engagement
- Involve diverse stakeholders, including developers, end-users, and ethicists, in the design process.
- Regularly review AI system outcomes to ensure alignment with ethical standards and adjust accordingly.
- Implement Bias Mitigation Strategies
- Conduct audits of data and algorithms to identify and remove bias.
- Use techniques such as fairness testing to evaluate the system’s impact across different groups.
- Transparency and Explainability
- Ensure AI systems provide explanations for decisions in a way that humans can understand.
- Implement mechanisms for users to contest or challenge AI decisions when necessary.
It is critical that AI systems are designed with an ethical framework that prioritizes human well-being, fairness, and privacy. These principles must be upheld throughout the development, deployment, and operational phases.
Ethical Guidelines in Collaborative AI Systems
Guideline | Objective |
---|---|
Fairness | Ensure equal treatment of all stakeholders and avoid discriminatory practices. |
Accountability | Establish clear responsibility for AI behavior and outcomes. |
Transparency | Provide clear insights into AI decision-making processes. |
Privacy | Safeguard user data and ensure that AI respects individual privacy rights. |
Best Practices for Aligning AI with Social Impact Goals
As AI technologies evolve, their potential to create positive societal change grows. However, to ensure AI development supports social impact goals, careful planning and ethical considerations must be embedded into each phase of the AI lifecycle. This includes prioritizing accessibility, fairness, and sustainability to address critical challenges such as poverty, inequality, and climate change. By aligning AI with these broader goals, we ensure the technology serves humanity in an equitable and responsible manner.
Effective AI solutions require continuous reflection, collaboration, and accountability. It is crucial to involve diverse voices throughout the development process, particularly those from marginalized communities, to ensure that AI systems are inclusive and equitable. Furthermore, real-time monitoring and impact assessments are necessary to evaluate how AI models are performing in real-world applications and make necessary adjustments.
Strategies for Responsible AI Development
- Inclusive Development: Involve a broad range of stakeholders in the design and implementation phases to ensure AI systems are built with diverse perspectives in mind.
- Algorithmic Transparency: Clearly document and share the methodologies, data sources, and decision-making processes used by AI systems to ensure transparency and foster public trust.
- Accountability Systems: Establish clear responsibility structures to ensure that developers, deployers, and other stakeholders are held accountable for the social impact of AI systems.
Continuous Monitoring and Ethical Oversight
- Impact Assessment: Continuously assess the societal effects of AI technologies, including potential risks or unintended consequences, and adjust policies or models as needed.
- Compliance with Regulations: Ensure AI systems are compliant with existing laws, such as data privacy laws, anti-discrimination laws, and other ethical standards to safeguard public interest.
- Community Engagement: Involve communities directly impacted by AI technologies in decision-making processes to ensure their needs and concerns are effectively addressed.
Measuring AI's Impact on Society
Metric | Focus Area | Example |
---|---|---|
Accessibility | Ensuring all groups benefit from AI technologies | Evaluating AI-powered healthcare tools to make sure they are accessible in low-income or rural areas |
Bias Reduction | Minimizing discriminatory outcomes in AI decision-making | Reviewing AI hiring algorithms to eliminate gender or racial biases in recruitment processes |
Environmental Sustainability | Reducing the ecological footprint of AI systems | Measuring energy consumption in large-scale AI models and optimizing for efficiency |
"To truly benefit society, AI must be crafted with an unwavering commitment to fairness, inclusion, and sustainability across all stages of its lifecycle."
Measuring and Ensuring Transparency in A.I. Operations
Ensuring clarity in artificial intelligence (AI) processes is essential for fostering trust and accountability within AI systems. As AI technologies increasingly impact various sectors, understanding their internal workings is crucial for both developers and end-users. Transparent AI operations allow for better scrutiny, identification of biases, and the overall improvement of AI decision-making processes. Furthermore, transparency is vital in aligning AI development with ethical standards and regulatory frameworks.
Effective measurement of transparency in AI systems involves assessing various components, including the interpretability of algorithms, data sources, and the decision-making processes behind AI actions. By ensuring transparency, stakeholders can verify that AI systems perform as expected, avoid unexpected outcomes, and remain aligned with ethical guidelines.
Key Approaches to Measuring AI Transparency
- Interpretability of Algorithms: The ability of human users to understand and explain the decision-making logic of AI systems.
- Data Provenance: The transparency regarding the origin, quality, and integrity of the data used to train AI models.
- Decision Traceability: Maintaining clear records of AI’s decision paths, allowing for audits and assessments.
Methods for Ensuring Transparency
- Explainable AI (XAI): Developing models that can provide understandable and interpretable outputs for human users.
- Open Source Frameworks: Making AI models and algorithms publicly available for inspection and modification by the broader community.
- Regular Audits: Implementing periodic reviews and assessments of AI systems to ensure compliance with transparency standards.
Importance of Transparency in AI Operations
"Transparency in AI not only improves the trustworthiness of systems but also helps prevent harmful practices such as bias, discrimination, or exploitation of vulnerable populations."
Challenges to Achieving Transparency
Challenge | Explanation |
---|---|
Lack of Standardization | There is no unified framework or set of metrics to measure AI transparency consistently across different sectors. |
Complexity of AI Models | Many AI systems, especially deep learning models, are difficult to interpret due to their intricate structure and large data sets. |
Data Privacy Concerns | Providing transparency might require revealing sensitive data, raising privacy and security concerns. |
Identifying and Mitigating A.I. Risks Through Collaborative Efforts
The rise of artificial intelligence presents numerous challenges that must be tackled through a concerted, multi-disciplinary approach. A major concern is the potential for A.I. systems to make decisions that can unintentionally harm society or reinforce biases. These risks can arise due to flaws in design, lack of transparency, or unethical data usage. Identifying these risks early is essential to ensure that A.I. technology benefits everyone and does not perpetuate existing inequalities or generate new dangers. Collaborative efforts among researchers, industry experts, policymakers, and communities are key in addressing these challenges.
By pooling expertise and resources, we can develop strategies to prevent the negative consequences of A.I. deployment. Through shared responsibility and constant feedback loops, we can ensure that A.I. evolves in ways that are both beneficial and safe. Below are some of the key risks associated with A.I., as well as methods to mitigate them through collective action.
Key Risks of A.I. and How to Address Them Together
- Algorithmic Bias: A.I. systems can unintentionally perpetuate societal biases embedded in their training data, leading to unfair outcomes in areas like hiring or law enforcement.
- Lack of Accountability: When A.I. makes decisions that impact individuals or groups, it can be difficult to determine who is responsible for any resulting harm.
- Security Threats: A.I. systems are vulnerable to attacks or exploitation, which could have catastrophic consequences if used maliciously.
Mitigation Approaches:
- Continuous Monitoring: Regular audits of A.I. systems to identify and address biases before deployment in critical areas.
- Establishing Clear Accountability: Defining legal frameworks that assign responsibility for A.I. decisions to human operators and organizations.
- Collaborative Research on Security: Partners from academia, industry, and government working together to build secure systems and defend against vulnerabilities.
"Addressing A.I. risks is not the responsibility of a single entity. It is through collaboration that we can develop standards, best practices, and safeguards to ensure the safe integration of A.I. into society."
Collaborative Structures for Risk Mitigation
Key Players | Role |
---|---|
Researchers | Conduct studies to identify risks and propose solutions in algorithmic fairness and security. |
Governments | Implement policies and regulations that enforce accountability and promote ethical A.I. usage. |
Technology Companies | Design and test A.I. systems that prioritize safety, fairness, and transparency. |
Public Advocacy Groups | Ensure that marginalized communities are considered in the development and deployment of A.I. systems. |
Building Cross-Disciplinary Teams for A.I. Accountability
To address the complexities of A.I. responsibility, it is essential to form teams with diverse expertise. These teams should be designed to bring together individuals from various fields to ensure comprehensive oversight and balanced decision-making. Integrating expertise from ethics, law, data science, and engineering helps identify and address challenges related to fairness, transparency, and accountability in A.I. systems.
Such collaborations enhance the ability to foresee potential risks and mitigate them effectively. For example, legal professionals can offer insights into compliance and regulation, while ethicists can guide the team in identifying moral considerations that may arise in the use of artificial intelligence. Together, these multidisciplinary perspectives will create more robust frameworks for responsible A.I. deployment.
Key Components of Cross-Disciplinary A.I. Teams
- Ethicists: Provide guidance on moral dilemmas and social impact.
- Legal Experts: Ensure compliance with existing regulations and anticipate future laws.
- Data Scientists: Analyze the accuracy, bias, and fairness of datasets used in A.I. systems.
- Engineers: Design and develop algorithms with accountability in mind.
- Psychologists/Sociologists: Study the societal impacts of A.I. and user interaction.
Approaches to Building Effective Teams
- Shared Vision: Establish a clear, unified goal that prioritizes responsible A.I. usage.
- Open Communication: Foster an environment where all disciplines can share perspectives freely.
- Continuous Learning: Provide ongoing training and updates on emerging ethical, technological, and legal issues.
- Collaboration with External Stakeholders: Engage with regulatory bodies, academics, and community representatives to ensure inclusive development.
“When different disciplines converge, the resulting outcomes are more balanced, innovative, and aligned with societal values.” – A.I. Responsibility Expert
Table of Cross-Disciplinary Team Roles and Responsibilities
Role | Primary Responsibilities |
---|---|
Ethicist | Assess the ethical implications of A.I. systems and guide decision-making from a moral standpoint. |
Legal Expert | Ensure compliance with relevant laws and regulations, and address emerging legal challenges in A.I. |
Data Scientist | Monitor data quality, ensure fairness in algorithmic decision-making, and minimize biases. |
Engineer | Develop A.I. systems that are transparent, explainable, and align with ethical standards. |
Sociologist/Psychologist | Examine societal impact and human behavior in relation to A.I. technologies. |
Utilizing AI Responsibility Labs for Sustainable Innovation
AI responsibility labs serve as collaborative spaces where multiple stakeholders, including researchers, developers, policymakers, and ethicists, come together to shape the future of AI. These environments are crucial for fostering long-term innovation by ensuring AI technologies are developed with accountability, transparency, and ethical considerations in mind. Such labs not only support the exploration of new AI capabilities but also help anticipate and mitigate potential risks associated with their widespread deployment.
By engaging in open dialogue and cross-disciplinary collaboration, these labs create the conditions for innovative solutions that prioritize human well-being and societal benefit. Long-term success hinges on continuously integrating responsible AI practices into development processes, fostering a culture of sustainable innovation, and addressing ethical dilemmas proactively rather than reactively.
Key Strategies for Leveraging AI Responsibility Labs
- Ethical Frameworks: Establishing clear guidelines for responsible AI use ensures that all developments adhere to legal and ethical standards.
- Collaboration Across Disciplines: Involving diverse experts leads to more balanced perspectives and solutions that align with societal needs.
- Transparent Development: Transparency in the development process builds public trust and provides a foundation for addressing concerns early in the process.
Practical Approaches to Long-Term Innovation
- Proactive Risk Management: Identifying potential risks and challenges before AI technologies are widely adopted allows for mitigation strategies to be integrated into the design phase.
- Continual Monitoring: Ongoing evaluation and auditing of AI systems help identify and address unintended consequences that may arise over time.
- Inclusive Decision Making: Ensuring diverse voices contribute to the decision-making process ensures that AI technologies meet the needs of all stakeholders, avoiding bias and inequity.
"AI responsibility labs act as incubators for the next generation of AI innovations, where accountability and creativity work hand in hand for the benefit of society."
Example of Key Components in AI Responsibility Labs
Component | Purpose |
---|---|
Ethical Guidelines | Provide a framework for responsible AI deployment, ensuring that ethical considerations are embedded in the development process. |
Cross-Disciplinary Teams | Bring together experts from various fields to address complex issues and create holistic AI solutions. |
Transparency Mechanisms | Ensure that AI systems' operations and decision-making processes are accessible and understandable to the public. |