Deep Learning in Education Sector
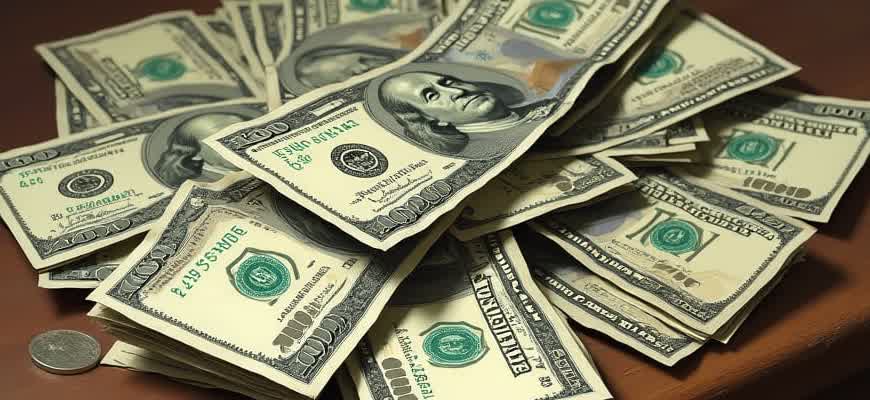
Deep Learning has significantly reshaped how educational systems function. By integrating AI technologies, institutions can now offer more personalized learning experiences and streamline administrative processes. Unlike traditional methods, where learning material is static and one-size-fits-all, deep learning enables the creation of adaptable content based on individual student needs.
Some of the key applications include:
- Personalized Learning: AI systems can assess students' strengths and weaknesses and deliver tailored lessons.
- Automated Grading: Deep learning algorithms can grade assignments with high accuracy, saving time for instructors.
- Predictive Analytics: AI can predict student outcomes and suggest interventions before issues arise.
Benefits of Implementing Deep Learning:
"AI can transform traditional learning environments into dynamic, data-driven ecosystems, enhancing both teaching and learning quality."
Below is a table summarizing some educational tools powered by AI:
Tool | Purpose | Impact |
---|---|---|
Smart Tutors | Offer real-time feedback and tailored lessons. | Improves individual learning outcomes. |
Adaptive Testing | Adjusts difficulty based on student performance. | Ensures optimal challenge for each student. |
Learning Analytics | Tracks student progress and behavior. | Helps identify at-risk students early. |
Optimizing Personalized Learning Paths with Deep Learning Models
Personalized learning paths are essential for catering to individual student needs and improving engagement in educational environments. With the power of deep learning models, the process of customizing learning experiences can be significantly enhanced. These models can analyze vast amounts of data about a learner’s preferences, strengths, and weaknesses to generate tailored educational plans that adapt as the student progresses. By utilizing sophisticated algorithms, educational institutions can provide a more effective and dynamic learning environment that evolves with each student's learning journey.
Deep learning, particularly through neural networks, can process both structured and unstructured data, such as student test scores, behavioral data, and even interaction patterns with digital content. This ability allows for the creation of highly responsive systems that can adjust learning paths in real-time, ensuring that each student receives the most relevant and challenging materials. Such systems can predict a learner's next steps and suggest resources that enhance their strengths or address their weaknesses, making education more efficient and personalized.
How Deep Learning Improves Personalization
- Adaptive Content Delivery: Deep learning models can analyze individual learner's pace and style, adjusting content complexity based on real-time performance.
- Early Detection of Struggling Students: Neural networks can identify patterns that indicate when a student may need additional support, allowing for timely interventions.
- Smart Feedback Loops: Feedback is tailored to the learner’s progress, promoting a more engaging and constructive learning experience.
Key Advantages of Using Deep Learning for Learning Path Optimization
Advantage | Description |
---|---|
Enhanced Engagement | Personalized learning paths keep students engaged by offering materials that are neither too easy nor too difficult. |
Data-Driven Decisions | Deep learning models leverage data to make informed decisions about what content to present next to each learner. |
Scalable Personalization | These systems can handle large numbers of students and provide personalized experiences at scale. |
"Deep learning transforms the way education adapts to individual learning needs, ensuring that no student is left behind in their academic journey."
Enhancing Student Assessment Systems Through AI-Driven Analytics
Artificial intelligence (AI) is revolutionizing the education sector by offering advanced tools for improving student assessment systems. Traditional evaluation methods often fail to capture the full spectrum of a student's abilities, as they primarily focus on exam-based metrics or standardized tests. With AI-driven analytics, institutions can gain deeper insights into student performance, enabling more personalized and accurate assessments.
AI-based assessment systems leverage vast amounts of data, from classroom interactions to online learning behaviors, to provide a more comprehensive view of student progress. These systems can identify patterns, predict future performance, and recommend tailored interventions to help students succeed. The integration of AI in assessment has the potential to make evaluations more dynamic, reflective of real-time progress, and adaptive to each student's unique learning style.
Key Benefits of AI-Enhanced Student Assessment
- Real-time Performance Tracking: AI algorithms can analyze student data continuously, allowing educators to track performance in real time and make adjustments to teaching strategies when necessary.
- Personalized Feedback: AI systems can provide students with specific, actionable feedback based on their learning patterns, helping them improve where they need the most support.
- Predictive Analytics: AI can forecast student outcomes, helping educators identify at-risk students early and intervene before performance issues become critical.
How AI Works in Assessment
- Data Collection: AI systems aggregate data from various sources such as assignments, quizzes, participation, and even student interactions with learning platforms.
- Pattern Recognition: Through machine learning models, AI analyzes this data to detect patterns in student performance and behavior that may not be visible to human instructors.
- Continuous Adaptation: As the system gathers more data, it constantly adjusts the feedback and assessment criteria to reflect individual student needs and learning progression.
AI-Driven Analytics in Action
"AI-powered assessment tools are not just about grading; they are about providing a more nuanced understanding of student abilities, enabling educators to offer support where it truly matters."
Aspect | Traditional Assessment | AI-Driven Assessment |
---|---|---|
Feedback | Generic, often delayed | Specific, real-time, tailored |
Scope of Evaluation | Limited to exams or projects | Holistic view, includes behaviors, participation, and progress |
Intervention | Reactive, after poor results | Proactive, identifies at-risk students early |
Automating Administrative Tasks with Deep Learning Algorithms
Administrative tasks in educational institutions, such as scheduling, grading, and managing student records, often require significant time and effort. Leveraging deep learning algorithms offers an efficient solution to streamline these processes, reducing human workload and improving accuracy. These algorithms can handle vast amounts of data, making them ideal for managing administrative duties that are typically prone to human error.
By implementing deep learning models, tasks like document classification, attendance tracking, and even responding to routine student queries can be automated. The adoption of these technologies allows educational institutions to reallocate resources to more impactful areas, enhancing overall productivity and learning experiences.
Applications of Deep Learning in Administrative Automation
- Automated Document Classification: Deep learning can classify documents such as assignments, reports, and applications, significantly reducing manual review times.
- Attendance Management: Facial recognition algorithms can automate attendance tracking, reducing administrative overhead and increasing the reliability of attendance records.
- Chatbots for Routine Queries: AI-driven chatbots powered by deep learning can provide instant responses to students’ frequently asked questions, improving student service efficiency.
Benefits and Challenges
Deep learning systems enable educational institutions to save time and costs while improving the accuracy and efficiency of administrative tasks. However, challenges such as data privacy concerns and the need for specialized infrastructure still need to be addressed.
Challenges to Overcome
- Data Privacy: Handling sensitive student information requires strict data protection protocols to ensure compliance with privacy laws.
- Infrastructure: Implementing deep learning solutions requires robust computational resources and high-quality datasets.
- Teacher and Staff Training: Ensuring that educators and administrative staff are properly trained to work with AI tools is essential for the successful adoption of these systems.
Potential Cost Savings
Task | Traditional Approach | Automated Approach | Potential Savings |
---|---|---|---|
Attendance Tracking | Manual record-keeping and verification | Facial recognition or RFID-based tracking | Reduced administrative hours |
Grading | Manual grading by instructors | Automated grading via AI tools | Faster feedback and less instructor workload |
Document Processing | Manual sorting and filing | AI-based classification systems | Improved efficiency and accuracy |
Real-Time Feedback Systems for Students Powered by Neural Networks
The rapid growth of artificial intelligence has opened new avenues for enhancing educational practices. One of the most promising innovations is the application of deep learning in providing real-time feedback mechanisms for students. These AI-driven systems can assess student performance, identify areas for improvement, and offer tailored recommendations instantly. With the support of neural networks, educators are now equipped with tools that make assessments more accurate and immediate than ever before.
Real-time feedback systems powered by neural networks help bridge the gap between traditional teaching methods and the need for personalized learning experiences. Unlike conventional methods that rely on delayed or generic responses, these systems provide students with immediate insights into their work. The feedback is based on a detailed analysis of the student's input, processed by advanced algorithms that can evaluate various learning metrics in real-time.
Key Features of Real-Time Feedback Systems
- Instant Evaluation: Neural networks process the student's responses as they submit assignments, allowing for immediate feedback.
- Personalized Recommendations: Based on student performance, the system generates customized suggestions for further improvement.
- Adaptive Learning Paths: As the student progresses, the system adjusts the learning content and challenges to suit their evolving needs.
Benefits of AI-Powered Feedback:
- Enhanced Learning Efficiency: Students receive feedback while they are still engaged in the learning process, leading to better retention and understanding.
- Reduction of Instructor Workload: Automated feedback allows teachers to focus on more complex aspects of instruction while still maintaining engagement with each student.
- Early Identification of Learning Gaps: The system can detect areas where students struggle and alert instructors to intervene before gaps widen.
"Real-time feedback is not just about speed, but also about the depth and relevance of insights provided to the learner."
Technical Implementation
To achieve real-time feedback, neural networks are typically trained on large datasets consisting of student responses, teacher evaluations, and learning outcomes. These systems rely on supervised learning models, where the network is provided with examples of correct and incorrect answers to refine its predictive capabilities. Over time, the model becomes adept at providing precise feedback based on patterns it learns from individual and group behaviors.
Feature | Description |
---|---|
Neural Network Model | Deep learning models trained on student data to predict performance and provide feedback. |
Real-Time Analysis | Continuous processing of student input to provide immediate feedback. |
Personalized Feedback | Adaptive recommendations based on individual performance metrics. |
Enhancing Educational Content Recommendations with Deep Learning Approaches
With the growing availability of online learning resources, one of the challenges in education today is ensuring that students are presented with the most relevant content. Traditional recommendation systems often struggle to accurately match students with the right materials due to the complexity of individual learning styles and preferences. Deep learning models offer a powerful solution to this problem by enabling systems to analyze vast amounts of data and personalize suggestions with greater precision.
By leveraging deep learning techniques such as neural networks, educational platforms can improve the accuracy of content recommendations. These models can account for a wide range of factors, such as the learner’s past behavior, the complexity of the content, and the contextual learning environment. The result is a more tailored and effective educational experience.
Techniques for Optimizing Content Suggestions
- Collaborative Filtering: This method identifies patterns in students' interactions with materials, suggesting content that similar learners have engaged with. Deep learning models enhance this technique by automatically detecting hidden relationships within user data.
- Content-Based Filtering: In contrast to collaborative filtering, this approach focuses on the attributes of the educational content itself. Deep learning models can analyze text, video, and other media to extract features and match them with a student’s learning profile.
- Hybrid Models: Combining collaborative and content-based filtering, these models use deep learning to integrate both user preferences and content characteristics, providing a more holistic approach to content recommendation.
Benefits of Deep Learning for Education Platforms
- Personalization: Deep learning allows systems to predict what resources will be most beneficial for each individual student, adjusting recommendations in real-time based on their evolving needs.
- Scalability: With large-scale data processing capabilities, deep learning models can handle millions of learners and resources simultaneously, making them highly effective for global platforms.
- Continuous Improvement: As more data is collected, deep learning models improve their predictions, creating a system that becomes smarter over time.
Key Considerations for Implementing Deep Learning in Education
Factor | Importance |
---|---|
Data Quality | Deep learning models require large volumes of high-quality data to function effectively. |
Model Transparency | It is essential to ensure that recommendations made by deep learning systems are explainable to educators and students. |
Bias Mitigation | Care must be taken to prevent bias in the data and models, ensuring that recommendations are fair and inclusive. |
"The future of personalized learning depends on how effectively we can harness the power of deep learning to understand and predict the unique needs of each learner."
Predicting Student Performance and Identifying At-Risk Learners with AI
Artificial intelligence (AI) can significantly enhance how educational institutions assess and improve student performance. By leveraging data from various sources, AI systems can predict student outcomes with remarkable accuracy, helping educators identify students who may require additional support. These systems analyze factors such as engagement levels, test scores, and participation to create personalized learning paths and intervene before academic struggles escalate.
AI-powered models use historical data and machine learning techniques to detect patterns and forecast future performance. These insights allow for early identification of at-risk students, ensuring timely intervention. This proactive approach reduces dropout rates and helps educators provide targeted assistance to students in need.
Key Methods for Predicting Performance
- Data collection: Student information, including grades, behavior patterns, and participation, is aggregated into large datasets.
- Data processing: Raw data is cleaned and preprocessed to remove inconsistencies and outliers, ensuring the quality of input for AI models.
- Predictive modeling: Machine learning algorithms, such as regression models and neural networks, are used to forecast future performance based on historical data.
Identifying At-Risk Students
AI tools can identify students at risk of underperforming by analyzing various indicators:
- Low engagement levels: Students who participate less in class activities and assignments are flagged as potentially struggling.
- Declining grades: A drop in academic performance is often a key signal for early intervention.
- Behavioral patterns: Consistent absences or behavioral issues may indicate underlying learning difficulties.
Predictive Models in Action
"By leveraging machine learning algorithms, educators can shift from reactive to proactive interventions, improving student outcomes through personalized support."
Performance Prediction Example
Student ID | Predicted Grade | Engagement Score | Risk Level |
---|---|---|---|
12345 | B+ | 85% | Low |
67890 | C | 60% | Medium |
11223 | F | 40% | High |
Leveraging Natural Language Processing in Intelligent Tutoring Systems
Natural Language Processing (NLP) plays a crucial role in the development of intelligent tutoring systems. By enabling machines to understand and process human language, NLP helps create personalized and interactive learning experiences. These systems can assess students' responses, identify knowledge gaps, and provide tailored feedback, which is vital in a dynamic educational environment. As education increasingly moves towards AI-driven tools, NLP's potential in creating smart tutors is becoming more evident.
Through NLP, smart tutoring systems can facilitate meaningful interactions between students and technology, offering immediate feedback that mirrors the effectiveness of one-on-one tutoring. This technology can be applied in various ways, such as automated essay grading, real-time question answering, and language learning support. Here’s how NLP enhances the learning process:
- Personalized feedback: NLP algorithms analyze student input to deliver tailored guidance based on individual learning styles and needs.
- Language comprehension: These systems can interpret and respond to natural language, making learning more intuitive for students across different subjects.
- Real-time assessments: NLP tools can evaluate student progress during interactions, allowing for immediate corrections and suggestions.
Key Benefits:
Benefit | Description |
---|---|
Scalability | Smart tutoring systems can handle a large number of students simultaneously, offering personalized learning without human intervention. |
Engagement | Interactive dialogue systems keep students engaged by making learning feel more conversational and accessible. |
"The ability of NLP to decode complex student queries and provide actionable feedback positions it as a key driver for the next generation of educational technologies."
Integrating Deep Learning into Remote Education Platforms
The shift to remote learning has created significant opportunities to integrate innovative technologies like deep learning (DL) into education. By utilizing advanced algorithms and data-driven insights, remote education platforms can deliver personalized learning experiences at scale. Deep learning enables systems to analyze vast amounts of student data and tailor content to each individual's learning pace and style, enhancing overall engagement and effectiveness.
Incorporating DL technologies in remote education helps address challenges such as student disengagement and content accessibility. Machine learning models can evaluate students’ performance in real-time and suggest adjustments to course materials, improving retention rates and knowledge transfer. The combination of AI-powered tools and remote platforms can offer continuous feedback, making the learning experience more adaptive and responsive.
Key Benefits of Deep Learning in Remote Education
- Personalized Learning Paths: Deep learning systems can create customized learning trajectories for each student, adjusting difficulty levels based on their progress.
- Real-Time Feedback: Automated assessments allow educators to provide immediate feedback, helping students correct mistakes and improve their understanding.
- Content Optimization: AI algorithms analyze learner behavior and optimize content delivery, ensuring the material is engaging and relevant.
Applications of Deep Learning in Remote Education Platforms
- Intelligent Tutoring Systems: AI-powered tutors can guide students through lessons, answering questions and providing explanations based on the individual’s learning history.
- Automatic Grading: Deep learning models can evaluate written assignments, essays, and even complex problem-solving tasks, saving time for instructors.
- Speech and Text Recognition: These technologies can transcribe spoken lectures into text, making the learning material more accessible to students with hearing impairments.
Important: The integration of deep learning into remote education platforms not only enhances personalization but also provides valuable insights into student performance, allowing educators to refine their teaching strategies more effectively.
Challenges to Consider
Challenges | Impact |
---|---|
Lack of Data | Deep learning models require large datasets for accurate predictions. Without sufficient data, models may underperform. |
Resource Intensity | Training deep learning models demands significant computational power and storage, which may not be available on all platforms. |
Privacy Concerns | Collecting and processing personal data for learning insights may raise privacy and security issues. |