Ai Based Adaptive Learning Systems
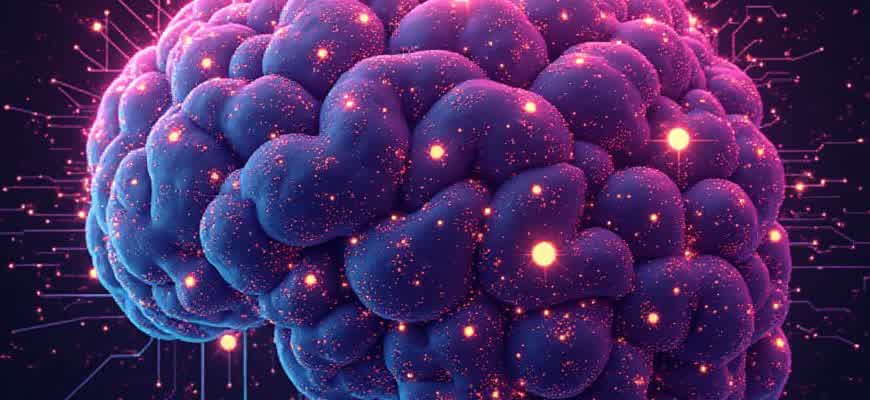
Artificial intelligence (AI) has made significant strides in transforming the education sector, particularly through personalized learning platforms. These systems use machine learning algorithms to analyze students' interactions and adapt educational content accordingly. By leveraging data from learners' performance, AI can provide a tailored learning experience, adjusting in real-time to meet individual needs and preferences.
Key Features of AI-Based Adaptive Learning:
- Personalized content delivery based on user progress and learning style.
- Continuous assessment through data collection to optimize learning outcomes.
- Real-time feedback to guide learners through challenging concepts.
Benefits:
Adaptive learning systems enhance engagement by offering a customized educational experience that is responsive to the individual pace of the learner.
Feature | Description |
---|---|
Content Customization | AI tailors lessons, quizzes, and assignments to the learner's strengths and weaknesses. |
Data-Driven Insights | Learning platforms analyze student data to make real-time adjustments to teaching strategies. |
AI-Driven Personalized Learning Systems: Practical Insights
AI-based adaptive learning systems have revolutionized the way educational content is delivered by tailoring learning experiences to individual needs. These systems analyze student performance, behavior, and preferences, adjusting the curriculum dynamically to maximize engagement and knowledge retention. Unlike traditional one-size-fits-all approaches, adaptive learning leverages advanced algorithms to provide personalized content, real-time feedback, and appropriate challenges, ensuring that each learner progresses at their own pace.
While the concept of AI-powered education has been widely discussed, practical implementation reveals several key insights. Successful integration of adaptive learning technologies requires not only robust algorithms but also a clear understanding of how to interpret data and use it to enhance the learning process. Below are some critical aspects of AI-based adaptive learning systems:
Key Practical Insights
- Real-Time Adaptation: AI systems continuously monitor learner progress and modify the content based on current performance, ensuring learners are neither overwhelmed nor under-challenged.
- Data-Driven Insights: The system generates actionable insights, allowing educators to identify knowledge gaps and personalize support for each learner.
- Scalability: These systems can scale across large numbers of students, providing personalized learning paths without requiring proportional increases in human resources.
“Adaptive learning is not about making content easier or harder, but about ensuring that learners engage with material at the right level of challenge for their current ability.”
Challenges and Considerations
- Data Privacy: Collecting and processing learner data raises significant concerns about privacy and security, especially when it comes to minors.
- Teacher Involvement: AI does not replace the teacher but augments their ability to monitor and guide students. Teachers must be trained to effectively use these systems.
- Algorithm Bias: If not properly designed, AI systems can perpetuate biases present in training data, leading to unfair learning outcomes for certain groups.
Comparison of AI-Based Systems and Traditional Methods
Feature | AI-Based Adaptive Learning | Traditional Learning |
---|---|---|
Personalization | Tailors content to individual needs in real time | One-size-fits-all approach |
Data Utilization | Uses learner data for continuous improvement | Limited use of data; relies on periodic assessments |
Scalability | Can handle large numbers of students without loss of quality | Requires proportional increase in resources for scaling |
How AI Customizes Learning Paths for Individual Learners
AI-powered learning systems utilize data-driven techniques to assess individual student performance and create personalized educational pathways. These systems gather real-time insights based on interactions, preferences, and historical progress, which allows them to adjust content delivery according to the unique needs of each learner. By analyzing patterns in student behavior, AI can predict areas of difficulty and strength, allowing for dynamic alterations in the curriculum to ensure optimal engagement and effectiveness.
The ability to tailor learning paths means that students experience a more fluid, adaptive environment. For example, if a student excels in a particular area, the system can provide advanced materials to challenge them further, while offering additional resources or exercises in areas where they are struggling. This level of personalization helps maintain student motivation and prevents frustration due to a one-size-fits-all approach.
Adaptive Learning Strategies
- Content Adjustment: AI monitors student progress and updates the difficulty of tasks based on performance metrics.
- Learning Pace: Students progress at their own pace, allowing for a deeper understanding of concepts before moving forward.
- Feedback Loops: Continuous feedback helps students to track their progress and improve over time.
AI-based systems adjust the learning trajectory in real-time, offering personalized challenges and support tailored to each learner's pace and progress.
Key Factors in Personalizing Learning
Factor | Role in Personalization |
---|---|
Data Collection | Continuous monitoring of student actions, choices, and performance informs the system’s adjustments. |
Behavioral Patterns | AI identifies trends and adapts the learning material to fit each student's learning style. |
Adaptive Algorithms | Algorithms ensure that the right content is delivered to the right student at the right time based on individual needs. |
Through the integration of data-driven insights, AI systems create a personalized learning journey that evolves based on the learner’s strengths and challenges.
Key Features of Adaptive Algorithms in AI Learning Systems
Adaptive algorithms in AI learning systems are designed to personalize the learning process by adjusting to individual user needs and learning styles. These algorithms analyze learner data in real-time and adjust the content, difficulty, and pace of instruction to enhance the learner's experience. By continuously monitoring performance and engagement, adaptive systems ensure that each learner is provided with the most relevant and effective learning path. The integration of these systems is essential for creating efficient, scalable educational environments in both academic and professional settings.
One of the most important aspects of adaptive learning algorithms is their ability to evolve based on data-driven insights. They rely on feedback loops that gather information from user interactions, such as progress rates, time spent on tasks, and error patterns, to dynamically adjust learning content. This results in a more customized and effective learning journey for every individual, catering to various learning speeds and styles.
Key Features
- Real-Time Adaptation: The system modifies the learning path based on continuous data input, such as user performance and engagement.
- Personalization: Tailors the difficulty, content, and pace of learning according to individual user profiles.
- Data-Driven Feedback: Collects data from user interactions to refine the learning experience and predict future learning outcomes.
- Scalability: Adaptive algorithms can manage large groups of learners, providing a personalized experience to each learner simultaneously.
- Efficiency: Optimizes the use of time by focusing on areas where the learner needs the most improvement, reducing unnecessary repetition.
"Adaptive algorithms create a dynamic and flexible learning environment where every user gets a learning experience tailored to their specific needs."
Types of Adaptive Algorithms
- Rule-Based Systems: Use predefined rules to determine content adjustments based on learner progress.
- Machine Learning Models: These models analyze learner behavior to predict and adapt learning paths using complex data patterns.
- Bayesian Networks: Leverage probabilistic models to predict the most likely areas where the learner may need additional support.
Comparison of Algorithm Features
Algorithm Type | Adaptation Method | Key Strength |
---|---|---|
Rule-Based Systems | Predefined rules based on learner performance | Simplicity and transparency |
Machine Learning Models | Data-driven predictions and real-time adjustments | Highly personalized and flexible |
Bayesian Networks | Probabilistic modeling for decision making | Effective in predicting learning needs |
Integrating AI-Driven Learning Systems in Classroom Environments
To successfully implement AI-based learning tools in educational settings, it is crucial to first understand how these systems can enhance both individualized and collective learning. These technologies can assess students' performance in real-time, adapting to their unique needs, learning styles, and paces. The key to effective integration is a structured approach, which includes aligning AI capabilities with curriculum goals, teacher involvement, and continuous assessment.
AI-powered systems rely on data to identify patterns in student behavior and learning progress, providing insights that can be used to tailor instruction. By leveraging these systems, educators can provide more personalized experiences, ensuring that each student’s learning journey is both engaging and effective.
Steps to Integrate AI into Classroom Learning
- Evaluate Educational Needs: Identify specific areas where AI can be most beneficial–whether it's in content delivery, assessment, or tracking student progress.
- Select the Right AI Tools: Choose platforms or software that are designed to enhance classroom engagement and can be easily integrated with existing teaching methods.
- Teacher Training: Educators must be trained to use AI tools effectively, understanding both their functionalities and their role in the learning process.
- Set Clear Objectives: Determine what outcomes are expected from the integration of AI, such as improved student engagement, personalized feedback, or mastery of specific skills.
- Monitor and Adapt: Continuously assess the effectiveness of AI tools, making adjustments as needed based on student feedback and learning data.
Key Features of AI in Classroom Settings
Feature | Benefit |
---|---|
Personalized Learning Paths | Allows students to progress at their own pace, ensuring no one is left behind. |
Real-Time Feedback | Helps students understand their mistakes immediately, reinforcing learning. |
Data-Driven Insights | Provides educators with actionable data to identify learning gaps and strengths. |
Note: It is important that AI tools complement–not replace–traditional teaching methods. Teachers should still play an active role in guiding and supporting their students.
Measuring Student Progress with Adaptive Learning Technologies
Adaptive learning technologies have revolutionized the way student progress is measured. Unlike traditional assessment systems, which often rely on fixed tests or standard evaluations, adaptive learning platforms track each learner’s unique path, adjusting content based on their strengths, weaknesses, and learning pace. This real-time data collection enables instructors to gain insights into not only a student's current understanding but also their learning trajectory over time.
To accurately measure student progress, adaptive learning systems employ a variety of metrics and techniques, offering a more personalized and dynamic view of academic achievement. These technologies rely on continuous feedback loops, which capture data from various interactions, including responses to practice questions, engagement with multimedia content, and overall performance across different modules.
Key Metrics for Tracking Student Performance
- Response Accuracy: Measures how well students answer questions, giving insight into their understanding of the material.
- Learning Speed: Tracks how quickly students grasp concepts and move through lessons, indicating their efficiency in mastering content.
- Engagement Levels: Monitors time spent on tasks and the frequency of interactions with learning materials, reflecting student commitment and interest.
- Skill Mastery: Evaluates the depth of understanding on specific topics, adjusting the difficulty of subsequent material based on prior performance.
Adaptive Learning Feedback and its Impact
“With adaptive learning technologies, instructors no longer have to wait for end-of-term exams to assess student comprehension. Continuous tracking and real-time feedback allow for immediate intervention and tailored support.”
How Progress is Evaluated in Adaptive Systems
- Continuous Assessments: Instead of single-point exams, assessments occur throughout the learning process, providing up-to-date data on student performance.
- Personalized Adjustments: Based on assessment results, the system adapts the difficulty of future content, ensuring a consistent challenge that aligns with the learner's abilities.
- Data Analytics: Advanced algorithms analyze patterns from multiple data points, helping educators understand the overall progress and specific areas where students may need additional help.
Student Performance Overview
Metric | Student A | Student B | Student C |
---|---|---|---|
Response Accuracy | 85% | 92% | 78% |
Learning Speed | Moderate | Fast | Slow |
Engagement | High | Medium | Low |
Skill Mastery | Good | Excellent | Needs Improvement |
Overcoming Challenges in Scaling AI Learning Systems for Large Institutions
Scaling AI-driven educational platforms within large organizations presents numerous obstacles, from technical constraints to organizational resistance. As these systems aim to provide personalized learning experiences at a massive scale, both infrastructure and adaptability must be addressed. The integration of AI requires not only high computational power but also flexible software solutions to handle the diverse needs of a large institution's student base, faculty, and administration.
Beyond the technical complexities, there are challenges in ensuring that these AI systems are effectively implemented across various departments. A cohesive strategy must be developed to align AI with institutional goals, overcome data silos, and ensure that all stakeholders can benefit from the technology. Moreover, the scalability of the AI system must be ensured to handle the growing data inputs and user interactions while maintaining high performance and accuracy.
Key Obstacles in Scaling AI Learning Systems
- Data Management: Large institutions often face challenges in managing vast amounts of diverse educational data. Ensuring accurate, high-quality data flow into AI systems is critical for personalization and system training.
- Computational Resources: AI models require significant computational power to process data and deliver real-time feedback. Scaling the infrastructure to support these demands can be both costly and technically complex.
- Integration with Existing Systems: Many institutions operate on legacy systems that are not designed to work with modern AI technologies. Seamless integration of AI into these systems can be a significant hurdle.
Approaches for Effective Scaling
- Cloud Computing Solutions: Leveraging cloud infrastructure allows institutions to scale computational resources dynamically based on demand without the need for large upfront investments in hardware.
- Modular AI Systems: Developing modular AI systems that can be customized and scaled independently allows institutions to implement AI incrementally while avoiding a complete overhaul of existing infrastructure.
- Continuous Data Monitoring: Implementing real-time data monitoring mechanisms ensures that AI systems stay accurate and relevant as new data flows in. Regular updates and retraining of models are essential for long-term effectiveness.
"The integration of AI in educational institutions is not just about scaling technology; it's about creating an environment where both learners and educators can benefit from its insights and capabilities."
Example of Scaling Challenges in Action
Challenge | Solution |
---|---|
Data Integration | Utilizing a data lake architecture to centralize diverse educational data sources. |
Computational Load | Adopting hybrid cloud models to manage peak usage times efficiently. |
User Resistance | Investing in training programs and demonstrating AI’s value to stakeholders. |
Integrating AI Learning Tools with Existing Education Platforms
Adapting AI-powered educational tools into current learning management systems (LMS) is a complex yet beneficial process. These integrations allow for a more personalized, responsive learning environment, leveraging the strengths of both AI and traditional educational infrastructures. By enhancing existing platforms, educational institutions can provide students with real-time feedback, tailored learning experiences, and data-driven insights. This synergy between AI tools and LMS aims to improve student engagement, performance, and overall learning outcomes.
The challenge lies in ensuring seamless integration without disrupting the established workflows or requiring extensive re-training for educators. The integration process must consider compatibility with current software, ease of use, and the capacity to scale as the institution's needs evolve. Addressing these concerns is critical to fostering a productive relationship between AI tools and educational platforms.
Key Steps for Integration
- Identify the right AI learning tools that complement existing educational resources.
- Ensure seamless data flow between AI tools and the LMS.
- Integrate AI features without overwhelming users or disrupting existing workflows.
- Provide ongoing support and training for both educators and students.
Benefits of Integration
"AI-enhanced educational platforms provide dynamic learning experiences tailored to each student's unique pace and style."
- Personalization: AI adapts learning paths based on student performance, offering customized resources to address individual strengths and weaknesses.
- Efficiency: Automation of administrative tasks frees up educators’ time, allowing for more focus on teaching.
- Real-Time Analytics: Teachers receive actionable insights to track progress and adjust lessons accordingly.
Integration Considerations
Consideration | Description |
---|---|
Compatibility | Ensure that AI tools work with existing LMS software without technical issues. |
Usability | Maintain an intuitive interface for both educators and students, minimizing the learning curve. |
Data Privacy | Ensure the secure handling of student data, complying with relevant privacy regulations. |
Ensuring Data Privacy and Security in AI Learning Environments
In AI-powered adaptive learning systems, data privacy and security are crucial to maintain the trust of users and comply with regulatory frameworks. These systems rely heavily on large datasets, including sensitive personal information, to customize learning experiences. Therefore, ensuring that this data is protected from unauthorized access, manipulation, and misuse is of paramount importance. Without robust safeguards, the risk of data breaches, identity theft, and other malicious activities increases significantly, potentially undermining the effectiveness of the learning environment itself.
To address these concerns, several strategies are employed within AI learning systems. From data encryption and anonymization to secure access controls, these measures help safeguard data throughout its lifecycle. The following key practices are commonly implemented in such systems:
- Data Encryption: All sensitive information is encrypted both at rest and in transit, making it unreadable to unauthorized entities.
- Access Control: Only authorized personnel and systems are granted access to sensitive data, preventing unauthorized manipulation.
- Data Minimization: Only the necessary data is collected and retained, reducing the risk of exposure.
- Regular Audits: Regular security audits are conducted to detect vulnerabilities and ensure compliance with privacy regulations.
Important Considerations:
AI-based learning systems must comply with regional privacy laws such as the GDPR in Europe or the CCPA in California, ensuring that personal data is handled appropriately.
Furthermore, implementing AI-driven security protocols helps to identify unusual patterns in data access and usage, allowing for proactive measures in case of a potential breach. Machine learning models can be used to monitor and flag anomalies, ensuring that any threat is detected in real-time.
Key Security Measures in AI Learning Systems
Measure | Description |
---|---|
Encryption | Secures data by converting it into an unreadable format, ensuring only authorized users can decrypt it. |
Tokenization | Replaces sensitive data with non-sensitive placeholders, reducing the exposure of personal information. |
Authentication | Ensures that only authorized users can access the system through multi-factor authentication and strong password policies. |
AI-driven Monitoring | Utilizes machine learning models to detect and respond to unusual data access patterns. |