Most Popular Ai Platforms
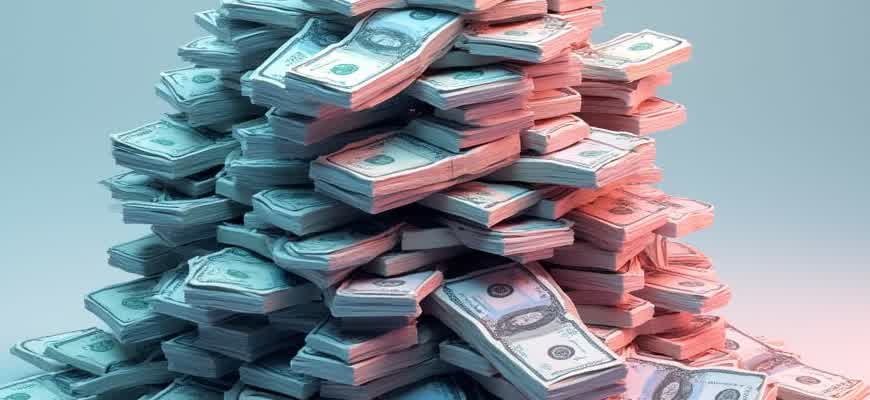
Artificial Intelligence platforms are transforming industries by providing innovative solutions for a range of tasks. These platforms offer advanced machine learning models, automation tools, and data processing capabilities to companies of all sizes. Some of the most widely used platforms in the industry today include:
- Google Cloud AI: Offers a range of services, including AI-powered machine learning models and tools for data analysis.
- Microsoft Azure AI: Provides an extensive set of tools, from cognitive services to machine learning, aimed at developers and enterprises.
- IBM Watson: Focuses on AI solutions for businesses, offering everything from natural language processing to deep learning models.
These platforms are distinguished by their ability to integrate seamlessly with existing systems and provide powerful insights based on vast amounts of data. They cater to both small startups and large enterprises looking to harness the power of AI to enhance productivity and decision-making.
Platform | Key Features | Target Users |
---|---|---|
Google Cloud AI | Advanced machine learning, big data analytics, pre-trained models | Startups, Enterprises |
Microsoft Azure AI | Cognitive services, custom AI models, deep learning | Developers, Enterprises |
IBM Watson | Natural language processing, AI-driven analytics, data security | Businesses, Research Institutes |
"The true value of AI platforms lies in their ability to simplify complex processes and deliver actionable insights that drive innovation."
Leading Artificial Intelligence Platforms
The demand for AI-driven solutions has surged in recent years, and various platforms have emerged as leaders in providing tools for machine learning, data processing, and deep learning. These platforms cater to developers, data scientists, and enterprises looking to build advanced AI models and applications with ease. They offer a range of services, from pre-built models to customizable solutions for various industries, making it easier to adopt AI technology across different sectors.
Among the most widely used AI platforms, each offers a unique set of features, such as scalability, ease of use, and integration capabilities with other technologies. This article highlights some of the most popular AI platforms currently in the market and outlines their strengths and key offerings.
Top AI Platforms
- Google AI Platform – Known for its powerful cloud-based tools, Google's platform enables seamless deployment of machine learning models and integrates with TensorFlow, Keras, and other tools.
- Microsoft Azure AI – Offers a comprehensive suite of AI tools, including cognitive services and Azure Machine Learning, allowing for scalable AI application development.
- Amazon Web Services (AWS) AI – Provides an extensive set of AI tools and machine learning services, including SageMaker for model training and deployment, and tools for natural language processing and computer vision.
- IBM Watson – Focuses on AI-driven analytics, with a strong emphasis on enterprise solutions, natural language processing, and automated machine learning.
Key Features Comparison
Platform | Strengths | Popular Use Cases |
---|---|---|
Google AI Platform | Deep learning tools, TensorFlow integration, cloud infrastructure | AI research, autonomous systems, healthcare AI solutions |
Microsoft Azure AI | Scalable infrastructure, advanced analytics, strong integration with Microsoft tools | Enterprise AI applications, customer service bots, predictive analytics |
AWS AI | Flexible AI tools, scalable machine learning, rich ecosystem | Big data analytics, recommendation engines, image recognition |
IBM Watson | Natural language processing, enterprise AI solutions, conversational AI | Healthcare diagnostics, customer service automation, sentiment analysis |
Note: Each platform has its own set of strengths, so the choice of platform depends on the specific needs of the business or project, such as scalability, cost, and integration requirements.
Choosing the Right AI Platform for Your Business Needs
Selecting the appropriate AI platform is crucial for businesses looking to integrate artificial intelligence into their operations. The right platform can drive innovation, improve efficiency, and reduce costs, while the wrong one may lead to wasted resources and missed opportunities. Factors such as ease of use, scalability, support, and cost-effectiveness should guide the decision-making process. Businesses must evaluate both their immediate needs and long-term objectives when choosing an AI platform.
Before diving into a specific solution, companies need to consider the type of AI technology that best suits their business model. Whether it's machine learning, natural language processing, or computer vision, the platform should align with the organization's goals. Below are key factors to consider when making this choice.
Key Factors to Evaluate
- Ease of Integration: Ensure the platform integrates smoothly with existing tools and systems.
- Scalability: The platform should grow with your business as demands evolve.
- Support and Community: A strong support system and active community can be invaluable for troubleshooting and guidance.
- Cost: Factor in both initial costs and long-term pricing structures, including potential hidden fees.
Comparison of Popular AI Platforms
Platform | Key Feature | Best For |
---|---|---|
Google AI | Scalable machine learning tools, TensorFlow | Large enterprises with deep learning needs |
IBM Watson | Advanced NLP capabilities, pre-built models | Businesses needing NLP and cognitive AI solutions |
Microsoft Azure AI | Comprehensive cloud AI services, strong integration | Businesses requiring cloud-based AI and automation |
Choosing the right AI platform is not just about technology–it’s about finding the best fit for your business objectives and operational needs.
Key Features to Look for in Top AI Platforms
When selecting an AI platform, it is essential to focus on several core features that will determine its effectiveness for your specific use case. These features can vary depending on the platform's purpose–whether it's for machine learning, natural language processing, or computer vision–but some factors remain universally important across all platforms.
Among the most critical attributes to evaluate are ease of use, integration capabilities, scalability, and security. An intuitive user interface and robust documentation are essential for quickly getting started. The ability to scale models without limitations ensures that the platform can grow alongside your needs. Security protocols are crucial to protect sensitive data, especially when dealing with AI applications in sectors like healthcare or finance.
Essential Features to Consider
- User-Friendly Interface: A clear and intuitive UI simplifies the learning curve and boosts productivity.
- Integration Capabilities: The ability to seamlessly integrate with existing systems and APIs ensures smooth deployment.
- Customization and Flexibility: The platform should allow customization of models and workflows to meet specific business requirements.
- Scalability: Look for platforms that offer horizontal and vertical scalability, allowing for increased data volume and complexity.
- Security Features: Robust encryption, user authentication, and compliance with data privacy regulations are essential.
Additional Considerations
- Performance and Efficiency: Evaluate the computational resources required for training and inference, as well as the platform's ability to optimize resource allocation.
- Support and Community: A strong support system, including online communities and customer service, is invaluable when troubleshooting issues.
- Cost Transparency: Ensure the pricing model aligns with your budget and provides clear insights into any additional costs.
"A great AI platform not only provides powerful tools but also ensures ease of use, integration, and scalability to grow with your organization."
Feature | Importance |
---|---|
User-Friendly Interface | Essential for quick adoption and smooth workflow management |
Integration Capabilities | Ensures compatibility with existing systems, boosting efficiency |
Scalability | Supports future growth, allowing for larger models and data sets |
Cost Comparison: AI Platform Pricing and Subscription Plans
When selecting an AI platform, one of the most crucial factors is understanding the associated costs. AI services often come with a variety of pricing structures, and users need to carefully evaluate which plan aligns with their requirements and budget. The pricing models for popular AI platforms typically include pay-per-use, subscription-based plans, or a mix of both, and the rates can vary significantly depending on the services provided and the scale of usage.
To help clarify these differences, we will compare the pricing options for several prominent AI platforms. Each platform offers unique features and different pricing tiers, which cater to various types of users, from small startups to large enterprises.
Pricing Structures Overview
Most AI platforms offer flexible pricing models. Here is a breakdown of common types:
- Pay-Per-Use: Charges based on usage, such as the number of API calls, data processed, or compute resources consumed.
- Subscription Plans: Fixed monthly or yearly fees that offer a certain amount of service usage.
- Freemium: Free tier with limited usage and features, with paid upgrades for additional capabilities.
Comparison Table
AI Platform | Pricing Model | Starting Price | Free Tier |
---|---|---|---|
Platform A | Pay-Per-Use | $0.01 per API call | Yes, limited calls |
Platform B | Subscription | $29/month | No free tier |
Platform C | Freemium | Free (up to 10,000 API calls/month) | Yes, with limits |
Note: The cost of AI platforms can vary significantly based on the specific services and the scale of usage. It's important to assess both the price and the value of features before committing to a plan.
Conclusion
Understanding the pricing structures of AI platforms helps you make an informed decision based on your budget and business needs. Whether you opt for a pay-per-use model, a subscription plan, or a freemium option, carefully reviewing the terms and comparing features can lead to cost savings and ensure that you are getting the most value from your chosen platform.
How to Seamlessly Integrate AI Tools into Your Current Operations
Incorporating AI solutions into your established workflow can be a game changer, boosting productivity and enhancing decision-making processes. However, this integration requires careful planning and a strategic approach to ensure smooth adoption without disrupting existing systems. By choosing the right AI platform and integrating it effectively, businesses can improve automation, streamline data handling, and enhance user experiences.
The process of integrating AI tools into your workflow involves several steps, from selecting the appropriate platform to aligning it with your team's objectives. Below are practical steps to help ensure a successful integration.
Key Steps for Integration
- Identify Your Needs: Clearly define the problem you're trying to solve with AI. Whether it's improving customer service, automating repetitive tasks, or analyzing big data, understanding the problem is essential before choosing the right tool.
- Select the Right Platform: Choose a platform that aligns with your goals. Research and evaluate AI platforms based on compatibility, scalability, and ease of use. Popular choices often offer trial periods or demo versions to test before committing.
- Set Up a Pilot Program: Before full-scale deployment, run a pilot program to test the AI's effectiveness in real-world scenarios. This helps identify potential issues and fine-tune the integration.
- Train Your Team: Invest in training your team on how to use AI tools. Provide support to ease the transition, and encourage feedback to improve the system.
Best Practices for Smooth Implementation
- Ensure Compatibility: Make sure that the AI platform integrates seamlessly with your current software stack. Check for available APIs and integration options to avoid compatibility issues.
- Monitor Progress: Continuously track performance and effectiveness. Use analytics tools to monitor the AI’s impact on key metrics, and adjust as needed to optimize results.
- Maintain Flexibility: Be ready to make adjustments as the system scales. AI integration is not a one-time setup; it requires constant monitoring and optimization.
"AI is most effective when it complements, rather than replaces, existing processes. A hybrid approach often delivers the best results."
Useful Tools and Resources
Platform | Features | Best For |
---|---|---|
TensorFlow | Open-source machine learning framework | Data scientists and developers |
IBM Watson | Advanced AI tools for business automation | Large enterprises with complex needs |
Google Cloud AI | AI-powered cloud solutions | Companies looking for scalable cloud solutions |
Understanding Data Privacy and Security in AI Platforms
Data privacy and security are crucial considerations when working with AI platforms. These platforms often handle large volumes of sensitive data, including personal, financial, and health-related information. Ensuring that this data is processed securely, stored safely, and only accessed by authorized individuals is paramount. AI systems must follow strict protocols to protect against data breaches and unauthorized access. Moreover, compliance with international regulations, such as GDPR or CCPA, is necessary for maintaining user trust and legal standing.
AI platforms typically utilize several mechanisms to safeguard data privacy. These include encryption techniques, user authentication protocols, and data anonymization strategies. It is also important to ensure that AI models do not inadvertently expose sensitive information during training or inference. Understanding these security measures helps organizations mitigate risks associated with data leaks, hacking, and misuse of personal data.
Key Strategies for Ensuring Data Privacy
- Encryption: Ensuring that data is encrypted both in transit and at rest prevents unauthorized access during storage or transfer.
- Access Control: Implementing strict access controls ensures that only authorized individuals or systems can interact with sensitive data.
- Data Anonymization: Removing or masking personally identifiable information (PII) reduces the risk of exposing private data during model training or analysis.
- Regular Audits: Conducting frequent security audits helps detect vulnerabilities before they can be exploited.
Common Risks in AI Platforms
- Data Breaches: Unauthorized access to sensitive data is one of the most significant threats to privacy.
- Model Inversion Attacks: Adversaries may attempt to extract sensitive information from trained AI models.
- Bias and Discrimination: AI systems may inadvertently reinforce societal biases, impacting fairness and privacy.
Important: AI developers must implement security measures from the outset of the design process, not as an afterthought. Privacy by design ensures compliance with laws and minimizes risks.
Security Features in Leading AI Platforms
Platform | Security Feature |
---|---|
Google AI | End-to-end encryption, secure data storage, and compliance with GDPR standards. |
Microsoft Azure AI | Multi-layered security protocols, role-based access control, and advanced data anonymization techniques. |
IBM Watson | Integrated security tools for data encryption, access monitoring, and regulatory compliance support. |
Evaluating AI Platforms for Scalability and Flexibility
When selecting an AI platform, it is essential to assess its scalability and flexibility to ensure that it can adapt to the growing needs of an organization. Scalability refers to the platform’s ability to handle increasing workloads efficiently without sacrificing performance. Flexibility, on the other hand, relates to the platform’s ability to integrate with various tools, data sources, and technologies, ensuring it can be customized to meet specific requirements.
AI platforms vary significantly in their capabilities to scale and adapt to different environments. Some are designed for large-scale deployments, offering robust infrastructure that can expand seamlessly as demands grow. Others are more versatile but may require additional configurations to handle high volumes of data or complex models. In either case, evaluating these aspects early on can save time and resources in the long run.
Key Factors for Scalability and Flexibility
- Infrastructure: Assess whether the platform can support cloud, on-premise, or hybrid deployments.
- Customization: Look for platforms that allow easy modification of models and algorithms to fit specific business needs.
- Integration: Ensure the platform can seamlessly integrate with other tools, databases, and existing IT environments.
- Support for Distributed Computing: Check if the platform supports distributed workloads for faster data processing.
Evaluating Scalability
- Performance Under Load: Test the platform’s ability to maintain performance as the data volume and computational requirements increase.
- Elasticity: Evaluate the system’s ability to automatically scale resources up or down based on real-time needs.
- Cost Efficiency: Consider how the platform handles resource allocation and cost management as it scales.
Evaluating Flexibility
- Support for Diverse Frameworks: Verify whether the platform supports popular AI frameworks such as TensorFlow, PyTorch, or custom-built solutions.
- Data Compatibility: Ensure compatibility with various data formats and sources, including structured and unstructured data.
- Custom Workflow Creation: Assess whether the platform allows for the creation of custom workflows that align with business processes.
"Scalability and flexibility are not just about growing with the business; they’re about adapting to rapidly changing technology and market demands."
Comparison Table
Feature | Platform A | Platform B | Platform C |
---|---|---|---|
Scalability | High, supports auto-scaling | Moderate, requires manual scaling | Very high, elastic scaling in cloud |
Flexibility | Customizable workflows and models | Limited integration options | Supports all major AI frameworks |
Integration | Seamless with various tools | Basic integrations available | Extensive integration options |
Case Studies: Success Stories with Leading AI Platforms
The integration of artificial intelligence in various industries has led to groundbreaking improvements, enhancing productivity and transforming business processes. Leading AI platforms, such as Google Cloud AI, IBM Watson, and Microsoft Azure, have empowered companies to innovate and streamline operations, providing advanced solutions to meet complex challenges. Below are several examples of how these AI solutions have been successfully implemented across different sectors.
One notable example is the retail industry, where AI has enabled businesses to optimize inventory management and enhance customer experiences. By utilizing AI-powered platforms, companies have improved their ability to predict demand, personalize shopping experiences, and increase sales efficiency. Another success story involves the healthcare sector, where AI has been used to improve diagnostics, automate administrative tasks, and enhance patient care.
Retail Industry: AI for Personalization and Demand Forecasting
One of the most significant achievements in the retail sector has been the use of AI platforms for personalized customer experiences and demand forecasting. Retailers are now able to analyze vast amounts of consumer data, leading to more effective inventory management and targeted marketing campaigns.
- Platform Used: IBM Watson
- Key Outcomes: Improved customer personalization, better demand forecasting, reduced waste
“By integrating AI-driven insights, we were able to create a more personalized shopping experience that not only engaged our customers but also increased sales by 15% within the first quarter.” – Retail Executive
Healthcare Sector: AI in Diagnostics and Automation
AI has made significant strides in the healthcare sector, particularly in diagnostics and patient care management. AI-powered platforms are helping medical professionals make more accurate diagnoses and automate time-consuming administrative tasks.
- Platform Used: Google Cloud AI
- Key Outcomes: Improved diagnostic accuracy, faster medical imaging analysis, better patient management
AI Platform | Sector | Results |
---|---|---|
IBM Watson | Retail | 15% sales increase, better inventory management |
Google Cloud AI | Healthcare | Improved diagnostic accuracy, faster imaging |
Future Trends in AI Platforms: What to Expect in the Coming Years
As the world of artificial intelligence continues to evolve, several key trends are emerging that will shape the future of AI platforms. These trends point to a more integrated, user-friendly, and efficient AI ecosystem that is expected to dominate in the coming years. The demand for AI tools that can assist in real-world applications, ranging from healthcare to automation, is increasing. It is crucial for developers and businesses to stay ahead of these trends to ensure they leverage AI’s full potential.
Advancements in AI are likely to lead to platforms that offer better scalability, faster processing times, and greater accessibility. As AI continues to play a central role in industries such as finance, education, and healthcare, companies will need to focus on developing more sophisticated platforms that meet the diverse needs of their users. Here are some of the key trends to watch for in AI development:
Key Future Trends in AI Platforms
- Automation and Self-Improving Systems: AI platforms will increasingly focus on automation of complex tasks, reducing the need for manual intervention. These platforms will be designed to learn from their own performance, improving their capabilities over time.
- Integration of AI and Edge Computing: Expect more AI platforms to integrate with edge computing. This will allow for faster data processing at the point of origin, reducing latency and improving real-time decision-making.
- Ethical AI and Transparency: With growing concerns over AI’s impact on privacy and ethics, there will be an increased push towards transparency in AI systems. Platforms will incorporate mechanisms for ethical decision-making, ensuring fairness and accountability.
Impact on Industry Applications
- Healthcare: AI platforms will play a crucial role in personalizing patient care, improving diagnostic accuracy, and streamlining administrative tasks.
- Finance: Enhanced risk management, fraud detection, and personalized financial services will be driven by AI platforms, providing more secure and efficient solutions.
- Manufacturing: AI-driven automation and predictive maintenance will become more widespread, increasing efficiency and reducing operational costs in manufacturing industries.
"In the coming years, AI platforms will no longer be standalone tools; they will become integral components of virtually every industry, offering seamless integration with existing systems and improving overall productivity."
Table of AI Trends and Expected Impacts
Trend | Impact |
---|---|
Automation and Self-Improving Systems | Increased efficiency and reduced need for human intervention. |
AI and Edge Computing Integration | Faster decision-making and reduced latency in data processing. |
Ethical AI | Improved transparency, fairness, and accountability in AI systems. |