Adaptive Learning with Ai
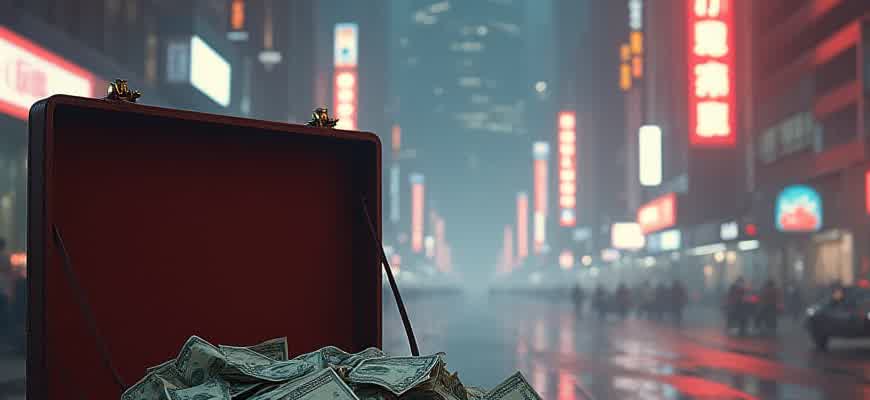
AI-powered adaptive learning systems leverage advanced algorithms to personalize the educational experience, ensuring that each learner receives content tailored to their individual needs. This dynamic approach contrasts with traditional one-size-fits-all teaching methods by responding to students' progress and adjusting the difficulty of tasks, pacing, and resources in real time.
Key Benefits of AI in Adaptive Learning:
- Personalized learning paths
- Real-time feedback and assessment
- Improved student engagement
- Data-driven insights for educators
These systems continuously monitor learner behavior, identifying patterns and areas where students struggle. The data is then used to adjust the curriculum to help learners master concepts before moving on to more complex material.
Adaptive learning systems help both students and instructors by identifying specific knowledge gaps, fostering a more efficient and effective learning process.
Example Features of Adaptive Learning Systems:
Feature | Description |
---|---|
Personalized Recommendations | AI suggests resources based on the learner's unique progress and learning style. |
Real-Time Feedback | Students receive instant responses to their performance, guiding them to the correct answers. |
Dynamic Pacing | The system adjusts the pace of content delivery based on the learner's mastery level. |
Adaptive Learning with AI: A Practical Guide
Artificial intelligence has significantly transformed how educational content is delivered. Adaptive learning systems leverage AI algorithms to personalize learning experiences, ensuring that each student receives the most effective content tailored to their specific needs. This personalized approach maximizes learning efficiency and improves overall outcomes. AI-driven platforms continuously monitor a learner's progress and adjust the difficulty, type, and pacing of the material in real-time, providing a truly customized learning journey.
However, implementing adaptive learning systems in education requires an understanding of how AI works, the tools available, and the impact it has on both learners and instructors. The following practical guide outlines key elements to consider when incorporating AI-based adaptive learning systems in an educational setting.
Key Components of Adaptive Learning Systems
- Data Collection: AI relies on vast amounts of data to understand student behavior and predict learning patterns. This data includes responses to quizzes, assignments, and interaction with learning materials.
- Real-Time Feedback: The system provides immediate feedback on learner performance, which can guide the next steps in the learning process.
- Personalized Content: Based on collected data, the AI adjusts content to match the learner's current proficiency level and preferred learning style.
How Adaptive Learning Systems Work
- Initial Assessment: At the start of a course, learners may take a diagnostic test that helps the system evaluate their knowledge level.
- Learning Path Creation: The AI generates a personalized learning path based on the results, focusing on areas where the learner needs the most improvement.
- Continuous Adaptation: The system continuously monitors learner performance and adjusts the content delivery accordingly. It can increase difficulty when the learner is excelling or provide additional resources when they're struggling.
Benefits of Using AI for Adaptive Learning
Benefit | Description |
---|---|
Individualized Learning | Each learner receives tailored content, ensuring that they are neither overwhelmed nor under-challenged. |
Efficiency | AI saves time by automatically adjusting content based on real-time analysis of performance, allowing learners to progress at their own pace. |
Scalability | Adaptive learning systems can be implemented on a large scale, benefiting both small classes and large groups of learners. |
"AI-powered adaptive learning systems are revolutionizing education by creating personalized pathways for each learner, making education more accessible and effective."
How AI-Driven Personalization Enhances Student Involvement
AI-driven personalized learning platforms offer tailored experiences based on individual student needs, preferences, and learning styles. These systems adapt content and assessments in real-time, ensuring that each student remains engaged by delivering the right level of challenge and support. By analyzing past performance, learning pace, and behavior patterns, AI can dynamically adjust resources, ensuring that students are never bored or overwhelmed.
The ability of AI to personalize learning experiences fosters deeper student involvement. With relevant and timely feedback, students are more likely to stay engaged in their studies. Furthermore, AI systems track progress continuously, identifying areas for improvement and offering targeted interventions, which helps keep learners on track and motivated.
Key Benefits of AI-Powered Personalization
- Real-time Adaptation: AI adjusts the learning path according to the student’s performance and learning style.
- Targeted Feedback: Personalized, instant feedback keeps students informed about their progress, improving retention and satisfaction.
- Enhanced Engagement: Students are presented with content that matches their interests and difficulty level, fostering intrinsic motivation.
Examples of AI Personalization in Action
- Dynamic Questionnaires: AI generates customized quizzes that focus on weak areas, ensuring practice in areas that need improvement.
- Learning Pace Adjustments: AI identifies when a student is struggling or excelling and adjusts the pace accordingly to keep the student engaged.
- Interactive Content: AI-powered tools create engaging multimedia content that caters to various learning preferences, such as videos, interactive simulations, and gamified elements.
"AI enables learning to be as unique as each student, making it possible for them to engage in ways that were never before possible."
Impact on Long-Term Learning Outcomes
Aspect | Impact with AI Personalization |
---|---|
Engagement | Increased as students receive tailored content and relevant challenges. |
Retention | Improved due to constant feedback and adjustments based on progress. |
Motivation | Higher motivation as students are presented with content that suits their level of mastery and interests. |
Integrating Personalized Learning Systems into Existing Educational Platforms
Integrating adaptive learning systems into traditional educational platforms can significantly enhance personalized instruction. These systems leverage AI to analyze learner data, adjusting content delivery based on individual needs, strengths, and weaknesses. The goal is to provide a more customized learning experience that keeps students engaged while ensuring they meet their educational goals. However, this integration requires careful planning and thoughtful adjustments to existing infrastructures.
To achieve successful integration, educational platforms must focus on compatibility, scalability, and data security. Incorporating AI-based systems into legacy platforms might require significant updates to the backend architecture and user interfaces. Additionally, it is essential to provide support for instructors and students to smoothly transition to the new, adaptive learning methods.
Steps for Effective Integration
- Assessment of Current Infrastructure: Before integrating AI-driven learning systems, evaluate the current platform’s technical capabilities and compatibility with adaptive technologies.
- AI System Selection: Choose an adaptive learning system that aligns with the platform’s educational goals and student needs. Consider factors such as content types, ease of use, and customization features.
- Training for Educators: Provide comprehensive training for instructors to help them use the new system effectively and incorporate adaptive techniques into their teaching practices.
- Continuous Monitoring: Implement a feedback loop to continuously monitor system performance and make adjustments based on data collected from users.
Key Benefits of Integration
Benefit | Description |
---|---|
Personalized Learning Pathways | Adaptive systems tailor content to each learner's needs, improving engagement and outcomes. |
Data-Driven Insights | AI generates actionable insights for both educators and students to enhance learning strategies. |
Scalability | Adaptive platforms can scale to accommodate growing numbers of students without compromising quality. |
"Integrating AI into learning environments creates a dynamic and responsive experience that benefits both learners and educators."
Maximizing Learning Outcomes with Real-Time Data and AI Feedback
Adaptive learning systems powered by artificial intelligence (AI) offer personalized, real-time feedback that helps students progress at their own pace. By leveraging real-time data, these systems dynamically adjust the learning experience, ensuring that each learner receives tailored content that suits their individual needs and abilities. This creates an environment where students can achieve optimal learning outcomes, enhancing both engagement and retention of knowledge.
AI-driven systems continuously track learner performance and use this data to make immediate adjustments to instructional strategies. This ensures that learners are not only challenged appropriately but also provided with timely support when necessary. By delivering personalized interventions, real-time data can reduce frustration and increase the chances of mastering complex topics efficiently.
Key Features of Real-Time AI Feedback in Adaptive Learning
- Instant Performance Tracking: AI algorithms track a learner’s progress continuously, offering immediate insights into areas of strength and weakness.
- Personalized Learning Paths: Based on real-time data, the system adapts the curriculum to match the learner’s pace and proficiency level.
- Contextual Feedback: Learners receive feedback specific to the task or concept they are working on, helping to target knowledge gaps quickly.
Benefits of Real-Time Data Integration
- Improved Engagement: Real-time feedback motivates students by providing them with constant updates on their progress and next steps.
- Efficient Learning: By focusing on areas that need attention, learners can avoid wasting time on concepts they have already mastered.
- Targeted Interventions: AI systems can pinpoint when learners need help and offer instant resources, preventing them from falling behind.
Real-Time Data Analysis in Action
Time | Student Action | AI Response |
---|---|---|
Initial Test | Student completes a quiz on new material | AI identifies weaknesses and adjusts the learning path |
Mid-Learning | Student struggles with a specific concept | AI delivers targeted explanations and resources |
Final Assessment | Student completes a final test | AI evaluates progress and suggests areas for further study |
"Adaptive learning systems, when fueled by real-time data, can dramatically transform how students engage with content, providing them with the right resources exactly when they need them."
Developing Scalable AI-Driven Learning Models for Extensive Online Courses
Designing adaptive learning systems for large-scale online courses presents unique challenges. One of the primary concerns is the ability to tailor educational content to individual learners while simultaneously managing large volumes of data. AI-powered models must be robust enough to handle real-time adjustments to content based on student performance, yet scalable to accommodate thousands or even millions of users without compromising the learning experience.
Effective scalability of adaptive learning systems requires a combination of advanced machine learning techniques and efficient data management strategies. Key components include dynamic content delivery, personalized feedback mechanisms, and predictive analytics. By leveraging these elements, learning platforms can optimize both individual learning paths and the overall course experience for a diverse student base.
Key Strategies for Scalable Adaptive Learning
- Modular Content Design: Breaking down course material into smaller, manageable modules allows for easier adaptation to varying learning speeds and styles.
- Real-Time Analytics: Using AI to analyze student interactions and performance data enables the system to make quick adjustments to the learning path.
- Automated Feedback Systems: Personalized, instant feedback enhances student engagement and ensures that learners stay on track.
Implementation Considerations
- Data Collection and Management: Ensuring efficient data storage and processing techniques for large datasets is crucial for maintaining performance.
- AI Model Optimization: Regular fine-tuning of the machine learning algorithms is necessary to ensure accuracy in adapting the learning experience.
- Infrastructure Scaling: Cloud-based solutions can be employed to scale resources dynamically based on user load, ensuring the platform remains responsive.
Important Metrics for Adaptive Learning Success
Effective adaptive learning systems must be evaluated based on learner retention, engagement, and performance improvements over time. These metrics help to determine the success of the personalized learning pathways created by AI models.
Metric | Importance |
---|---|
Engagement Rate | Indicates the level of student interaction with the platform and its content. |
Retention Rate | Shows how well students retain knowledge and stay enrolled in the course. |
Performance Improvement | Measures the progression of student performance over time as a result of personalized learning interventions. |
Assessing the Effect of AI-Driven Personalized Learning on Retention Rates
The integration of AI-based adaptive learning systems has had a significant impact on student engagement and retention in educational settings. Personalized learning pathways, powered by AI, adapt to the needs and progress of individual learners, ensuring that they receive the appropriate level of challenge and support. This customization enhances the learning experience, which can, in turn, increase the likelihood of students staying in the program and successfully completing it. AI’s ability to analyze data from student interactions helps identify learning patterns and address areas where students struggle, reducing the likelihood of disengagement and dropout.
Recent studies have shown a strong correlation between the use of adaptive learning technologies and improved student retention rates. By tailoring content to the unique learning styles and paces of individual students, these systems foster a deeper understanding and engagement with the material. As a result, students are more likely to continue their studies and complete courses or programs. Below are key factors contributing to the positive influence of AI on retention.
Key Factors Influencing Retention
- Personalized Learning Experience: AI systems can create unique learning paths for each student, ensuring that they stay engaged and challenged at the right level.
- Real-Time Feedback: Immediate, data-driven feedback helps students understand their strengths and areas for improvement, which encourages continuous learning.
- Support for At-Risk Students: AI tools can identify students at risk of falling behind and offer targeted interventions before they disengage.
- Adaptive Content: By adjusting the difficulty and nature of the content to match each learner’s needs, AI can reduce frustration and promote a sense of progress.
Measuring the Impact: Evidence from Research
Studies have quantitatively measured the impact of AI on retention through various metrics, such as completion rates, student satisfaction, and academic performance improvements. One common method involves comparing student outcomes in courses using adaptive learning platforms with those in traditional, non-adaptive environments.
Metric | Traditional Learning | Adaptive Learning with AI |
---|---|---|
Course Completion Rate | 72% | 85% |
Student Satisfaction | 68% | 90% |
Retention Rate | 60% | 78% |
"AI-driven adaptive learning platforms are not only improving student performance, but they are also helping to significantly reduce dropout rates by providing continuous, personalized support." – Educational Technology Researcher
Overcoming Technical Challenges in AI-Driven Adaptive Learning Platforms
Adaptive learning platforms powered by artificial intelligence face numerous technical hurdles that need to be addressed to ensure effective personalization of educational content. One of the primary challenges is the ability to gather and process large amounts of data in real-time, while maintaining the accuracy of predictions and recommendations. This data-driven process requires advanced algorithms capable of adjusting the content dynamically based on the learner’s behavior and progress.
Another significant obstacle is the integration of AI models with existing educational technologies. These systems must be interoperable with diverse platforms, tools, and content management systems, which often operate on different standards. Ensuring seamless communication and data flow between all components requires sophisticated technical infrastructure and robust software engineering.
Key Technical Issues
- Data Processing: Efficiently collecting, analyzing, and utilizing large datasets without overburdening system resources.
- Real-Time Adaptation: Updating content dynamically based on learner performance in real-time can be complex and resource-intensive.
- Scalability: Ensuring the platform can handle a growing number of users while maintaining high-quality adaptive features.
"Adaptive learning systems are only as effective as the data they process, making the development of precise AI algorithms critical."
Approaches to Overcome These Challenges
- Improved Data Management: Optimizing data storage and retrieval methods to minimize delays and bottlenecks during data processing.
- Advanced AI Algorithms: Utilizing machine learning models that improve over time and refine predictions as more data is collected from users.
- Cloud-Based Solutions: Leveraging cloud technologies to enhance scalability, ensuring the platform can expand without compromising performance.
System Requirements for AI-Driven Platforms
Component | Requirement |
---|---|
Data Storage | Scalable cloud storage solutions with fast access speeds. |
Processing Power | High-performance servers with dedicated AI computation resources. |
Algorithm Training | Continuous learning models capable of adapting based on user interaction data. |
Enhancing Teacher Roles Through AI: From Instructor to Learning Facilitator
The integration of artificial intelligence into education systems has significantly shifted the way teachers interact with students. With AI tools offering personalized learning experiences, the teacher's role is no longer confined to traditional instruction. Instead, educators are evolving into facilitators of learning, guiding students as they navigate through adaptive learning paths tailored to their individual needs and strengths. This transformation opens up opportunities for a more dynamic and responsive approach to teaching, where the focus is on empowering students to take ownership of their learning journey.
AI enables teachers to spend more time focusing on mentorship, problem-solving, and emotional support, rather than on administrative tasks or one-size-fits-all lectures. By leveraging AI-driven insights, educators can track student progress in real time, providing personalized recommendations and interventions that are aligned with each student's pace and abilities. This shift in focus from instruction to facilitation allows teachers to engage with students more effectively and foster deeper learning outcomes.
Key Aspects of the Teacher's Evolving Role
- Personalized Guidance: Teachers use AI to tailor learning experiences based on individual student data, ensuring that each learner receives the right level of support.
- Real-Time Analytics: Teachers can access detailed reports on student performance, enabling them to make data-driven decisions about when to intervene and how to adjust learning strategies.
- Collaboration and Mentorship: With AI handling routine tasks, teachers can focus on building relationships with students, offering personalized mentorship and fostering collaboration.
Benefits of AI in Facilitating Teacher Roles
"AI technology empowers teachers to move from traditional instruction to dynamic learning facilitation, enhancing student engagement and ensuring tailored educational experiences."
- Improved Student Engagement: By adapting lessons to the unique needs of each student, AI encourages active participation and greater enthusiasm for learning.
- Efficiency and Time Savings: Teachers can automate grading, track progress, and manage content distribution, freeing up time to focus on higher-value activities like coaching and guiding.
- Data-Driven Support: AI offers detailed insights into student learning patterns, helping educators identify areas for improvement and implement targeted interventions.
Teacher's Evolving Responsibilities: A Comparative Table
Traditional Role | AI-Enhanced Role |
---|---|
Delivering lectures and content | Facilitating personalized learning experiences |
Grading assignments manually | Using AI to automate grading and assessments |
Providing generic feedback | Delivering data-driven, customized feedback |
Managing classroom logistics | Focusing on student development and mentorship |