Ai in Rvb
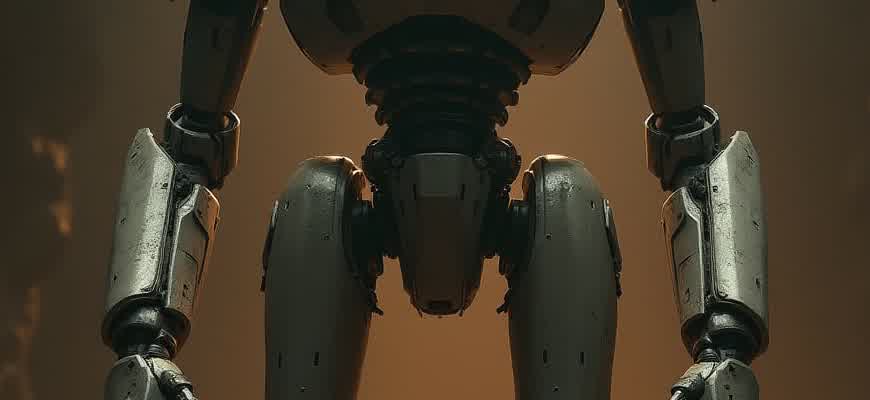
AI-driven technologies are revolutionizing the use of RGB in various industries, enhancing the functionality of devices and systems that rely on color recognition and manipulation. The integration of machine learning and AI models has opened up new possibilities for RGB processing, from gaming to design and even medical imaging.
AI's role in RGB applications can be categorized into several key areas:
- Color Detection and Calibration
- Image Enhancement
- Real-Time Processing
- Automation in Creative Fields
"Machine learning algorithms are now capable of not only processing raw RGB data but also making predictions about optimal color adjustments and generating images based on user preferences."
Below is a table illustrating the advancements in AI for RGB processing:
Technology | Application | Impact |
---|---|---|
Deep Learning | Image recognition and enhancement | Improved image quality and color accuracy |
Neural Networks | Color-based automation in design | Faster production cycles and better customization |
Generative Adversarial Networks (GANs) | RGB image generation from minimal input | Enhanced creative possibilities with fewer resources |
AI in Rvb: Practical Applications and Benefits
Artificial Intelligence (AI) has found numerous practical applications within the Rvb (Red, Green, Blue) spectrum, particularly in fields like image processing, color correction, and automated design. AI models analyze RGB values to enhance visual content in real time, providing both creative and technical solutions for professionals across various industries. These tools can optimize the color grading process, automate image adjustments, and even create new visuals based on given parameters.
In modern workflows, AI-driven solutions are transforming tasks that once required manual labor into streamlined, efficient processes. These applications not only save time but also introduce a level of precision that can improve the overall visual quality of projects. This is particularly beneficial in areas such as advertising, entertainment, and digital media production.
Practical Uses of AI in RGB Processing
- Color Correction: AI algorithms can adjust RGB values to achieve perfect color balance, reducing human error and enhancing visual accuracy.
- Image Enhancement: AI can upscale low-resolution images, improving detail while maintaining color integrity across the entire spectrum.
- Automated Design: AI-driven software can generate new color schemes, offer design suggestions, and create aesthetically pleasing combinations based on RGB values.
Benefits of AI in RGB Workflows
- Time Efficiency: Tasks such as manual color adjustments and retouching are automated, reducing the time spent on repetitive processes.
- Consistency: AI ensures that color grading and corrections are applied uniformly across all media assets, improving visual consistency.
- Scalability: AI solutions are capable of handling large volumes of visual content, making them ideal for industries like film production and marketing.
"By leveraging AI for RGB processing, professionals can unlock new creative possibilities, improve workflow efficiency, and maintain high-quality results across various media types."
AI-Driven RGB Tools in Action
Tool | Application | Benefit |
---|---|---|
Adobe Sensei | Color correction, image enhancement | Automatic adjustments and improved quality |
DeepAI | Image upscaling and enhancement | Improved image detail and resolution |
Runway | Automated design generation | Creative suggestions and color scheme automation |
How AI Optimizes Rvb in Digital Marketing Campaigns
AI technologies have brought significant transformations in digital marketing, particularly in optimizing Return on Marketing Investment (Rvb). By leveraging machine learning algorithms, artificial intelligence can process vast amounts of data in real time, providing insights that are crucial for improving campaign efficiency. AI-driven systems help marketers to predict customer behavior, personalize content, and automate complex tasks that previously required manual intervention.
In the context of Rvb, AI offers tools that not only enhance targeting and segmentation but also fine-tune the allocation of resources, ensuring that every marketing dollar is spent effectively. By analyzing historical data, consumer preferences, and market trends, AI can recommend strategic adjustments that lead to increased engagement, conversions, and ultimately, a higher return on investment.
Key Benefits of AI in Rvb Optimization
- Improved Targeting: AI helps marketers identify and segment their audience with precision, allowing for more personalized campaigns.
- Dynamic Budget Allocation: AI algorithms can adjust marketing budgets in real-time based on campaign performance, optimizing ad spend.
- Predictive Analytics: Using historical data, AI can forecast future trends and behaviors, helping to adjust strategies proactively.
AI Applications in Rvb Enhancement
- Real-Time Data Analysis: AI tools analyze user interactions across different platforms and channels to provide actionable insights.
- Personalized Content Creation: AI can generate tailored content that resonates with specific audience segments, increasing engagement.
- Automated Campaign Management: AI handles the execution and optimization of marketing campaigns, saving time and reducing human error.
“By integrating AI into digital marketing strategies, businesses are able to optimize their Rvb by ensuring that each marketing dollar works harder, providing measurable and actionable results.”
Impact of AI on Conversion Rates and ROI
Factor | Impact of AI |
---|---|
Customer Segmentation | Increases precision, leading to more effective targeting and higher conversion rates. |
Campaign Adjustments | Real-time optimizations lead to improved performance and better resource allocation. |
Personalized Experiences | Enhanced customer engagement results in higher ROI from personalized content. |
Integrating AI for Real-Time Data Analysis in Rvb Systems
In modern Rvb (Radar, Video, and Beamforming) systems, the ability to process vast amounts of data in real-time is critical for accurate decision-making. AI, particularly machine learning algorithms, can significantly enhance these systems by offering faster data processing, anomaly detection, and predictive analysis. The integration of AI allows Rvb systems to automatically identify patterns within the incoming data streams, improving situational awareness and reducing human intervention in complex environments.
The real-time capabilities of AI in Rvb systems are based on the rapid analysis of sensor data, which is necessary for immediate responses in applications such as autonomous vehicles, security monitoring, and military operations. AI models can process the raw data from radar sensors, cameras, and other devices, enabling them to deliver real-time insights that were previously not possible with traditional computing methods.
Key Benefits of AI Integration in Rvb Systems
- Improved Data Processing Speed: AI algorithms can process large datasets in fractions of a second, providing real-time insights.
- Enhanced Accuracy: By learning from past data, AI systems can identify patterns and anomalies more accurately than manual methods.
- Adaptive Systems: AI models continuously improve over time, adjusting to new patterns and environments without requiring human reprogramming.
Steps for Implementing AI in Rvb Systems
- Data Collection: Gather data from various sensors (radar, cameras, etc.) that are part of the Rvb system.
- Data Preprocessing: Clean and format the data for use in AI models to ensure high-quality input.
- Model Training: Train machine learning models using historical and real-time data to detect relevant patterns and predict future outcomes.
- Real-Time Deployment: Implement the trained models within the Rvb system for live data analysis and decision-making.
"AI empowers Rvb systems to go beyond simple data collection, enabling intelligent processing that can adapt to dynamic environments."
Example of AI-Driven Data Flow in Rvb Systems
Step | Process | AI Contribution |
---|---|---|
1. Data Input | Raw data from radar, video, and sensor feeds. | AI identifies key features for further processing. |
2. Preprocessing | Data is cleaned and formatted. | AI selects relevant data points for analysis. |
3. Real-Time Analysis | Data is analyzed for real-time decision-making. | AI detects anomalies and predicts future events. |
4. Actionable Insights | Insights are fed back into the system or control interface. | AI provides recommendations for immediate action. |
Enhancing Customer Experience with AI-Driven Rvb Tools
Artificial intelligence (AI) is reshaping the way businesses interact with their customers. In the context of Rvb (Research and Value-Based) tools, AI is driving personalization and optimization, allowing companies to tailor their offerings more precisely to customer needs. These advanced systems provide businesses with valuable insights and recommendations, enhancing overall user satisfaction. By leveraging AI technologies, Rvb tools can automatically adapt to changing consumer behaviors, creating more responsive and efficient interactions.
AI-powered Rvb tools empower organizations to streamline customer touchpoints and improve decision-making processes. Automation of data processing and analysis enables faster responses to customer needs, while machine learning algorithms refine the experience over time. As a result, businesses can provide a more seamless and intuitive experience that fosters loyalty and trust, which is essential in today’s competitive market.
Key Benefits of AI-Powered Rvb Tools
- Personalization at Scale: AI can analyze vast amounts of customer data to tailor experiences, making each interaction feel unique and relevant.
- Faster Decision Making: AI tools enable businesses to make quicker decisions based on real-time data analysis, improving response times.
- Continuous Improvement: Machine learning algorithms learn from past interactions, constantly enhancing customer engagement strategies.
- Cost Efficiency: Automation reduces the need for manual processes, which can significantly lower operational costs.
How AI Enhances Customer Interaction
- Automated Customer Support: AI chatbots and virtual assistants can handle routine inquiries, providing instant responses to customers 24/7.
- Predictive Analytics: By predicting future customer behavior, AI tools help businesses make proactive decisions, ensuring that services are always aligned with customer expectations.
- Content Recommendation: AI systems suggest personalized content, products, or services based on past behavior and preferences, increasing engagement.
Example AI-Driven Rvb Tool Features
Feature | Benefit |
---|---|
Natural Language Processing (NLP) | Improves customer interactions by understanding and responding to queries in natural language. |
Sentiment Analysis | Analyzes customer emotions to tailor responses and improve customer satisfaction. |
Machine Learning Algorithms | Personalizes experiences and refines strategies based on past interactions and data insights. |
"AI-powered Rvb tools provide not only the efficiency needed for scaling businesses, but also the human touch that customers value in modern interactions."
AI-Powered Automation: Enhancing Rvb Workflow Efficiency
The integration of artificial intelligence into the RVB (Rendering, Visualization, and Broadcasting) workflow is reshaping how creative and technical tasks are executed. AI-driven automation optimizes processes by reducing the need for manual intervention, which allows professionals to focus on more complex, creative aspects of their work. This shift is particularly beneficial in environments where speed and precision are critical, such as in live broadcasts or time-sensitive media production projects.
By leveraging machine learning and data-driven algorithms, AI automates tasks like image processing, video editing, and scene recognition. This leads to a more efficient production pipeline, improving not only the speed but also the consistency of output. The result is a seamless workflow that can scale with demand and adapt to various project requirements.
Key Benefits of AI-Based Automation in Rvb
- Faster Production Times: AI can handle repetitive tasks such as color correction and scene optimization, reducing the time required for each phase of production.
- Improved Accuracy: Automated processes ensure consistent results, minimizing human error and ensuring that high standards are maintained throughout the project.
- Cost Efficiency: By cutting down the number of manual interventions, AI-driven tools lower labor costs and free up resources for more specialized tasks.
How AI Optimizes Key Stages of RVB Workflow
- Data Preprocessing: AI tools are used to preprocess large volumes of data, organizing and categorizing assets to be easily accessed during production.
- Rendering Optimization: Machine learning algorithms predict the most efficient rendering paths, improving speed and resource management.
- Real-time Adjustments: During live broadcasts or dynamic visual productions, AI can make real-time decisions on color grading, lighting adjustments, and scene transitions.
"By automating routine tasks, AI allows creative professionals to focus on innovation and problem-solving, unlocking new levels of productivity in the RVB industry."
Example of AI Application in RVB
Task | AI-Powered Automation | Result |
---|---|---|
Color Grading | AI algorithms analyze footage and adjust hues, shadows, and brightness automatically. | Consistent color accuracy across all scenes, reducing manual correction time. |
Scene Detection | AI-powered software scans video frames to identify key moments and segment scenes. | Faster editing process, enabling real-time scene adjustments and faster content delivery. |
Enhancing Personalization in Rvb with Machine Learning Techniques
Machine learning models are rapidly transforming the way users interact with online platforms, particularly in the realm of personalized recommendations. In the context of Rvb (Red vs Blue), these models offer an innovative approach to tailor content to each player's preferences and behavior. By analyzing massive amounts of data, machine learning algorithms can predict what users are likely to enjoy based on their past interactions, providing a more immersive and engaging experience.
Incorporating these technologies can dramatically improve how Rvb adapts to individual playstyles, offering more dynamic and relevant content. This can range from personalized in-game experiences to targeted content suggestions, increasing user engagement and satisfaction. However, achieving this level of personalization requires a thoughtful integration of machine learning models into the Rvb ecosystem.
Key Methods for Implementing Machine Learning in Rvb Personalization
- Collaborative Filtering: This technique analyzes user behavior to recommend content based on what similar users have enjoyed.
- Content-Based Filtering: Machine learning models identify patterns in the content players interact with, suggesting similar items based on their interests.
- Reinforcement Learning: This approach adapts content in real-time based on feedback, optimizing user experience as players interact with the game.
Benefits of Personalization in Rvb
"Personalized experiences not only increase player satisfaction but also help maintain long-term engagement in a competitive environment like Rvb."
- Increased Player Engagement: Personalization ensures players receive relevant content, keeping them engaged longer.
- Tailored In-Game Experiences: Players can experience customized challenges and scenarios that reflect their individual skills and preferences.
- Improved Retention Rates: With better content relevance, players are more likely to return, reducing churn.
Challenges and Solutions
Challenge | Solution |
---|---|
Data Overload | Implementing efficient data preprocessing and feature selection techniques to extract valuable insights. |
Model Overfitting | Utilizing cross-validation methods to ensure that the model generalizes well to unseen data. |
Real-Time Adaptation | Leveraging reinforcement learning to allow for continuous adaptation based on user interaction. |
AI-Enhanced Forecasting for Rvb Strategic Choices
Incorporating AI-driven tools into the forecasting process allows Rvb organizations to gain an edge in their decision-making strategies. Predictive analytics powered by artificial intelligence can process vast amounts of historical and real-time data, offering insights that guide more informed decisions. This shift towards data-centric decision-making helps organizations adapt quickly to market changes, predict trends, and optimize resource allocation.
AI-based predictive models enable teams to move away from traditional decision-making processes and leverage automated systems to predict potential outcomes. By employing machine learning algorithms and data analytics, Rvb professionals can identify correlations and patterns that were previously difficult to discern. This approach results in more accurate, data-driven forecasts and improved risk management.
Key Benefits of AI-Powered Predictive Analysis
- Improved Forecast Accuracy: Machine learning algorithms can analyze complex datasets to predict future outcomes with higher precision.
- Resource Optimization: AI can suggest the most efficient allocation of resources based on predictive models.
- Real-Time Insights: AI systems process real-time data, providing up-to-the-minute insights for quick decisions.
- Risk Mitigation: Predictive models can assess potential risks and suggest strategies to mitigate them.
Steps for Implementing AI in Predictive Decision-Making
- Data Collection: Gather historical and real-time data relevant to Rvb operations.
- Model Development: Train machine learning models using the collected data to predict future scenarios.
- Analysis and Reporting: Analyze the output of predictive models and integrate the insights into decision-making processes.
- Continuous Improvement: Continuously refine models based on feedback and updated data.
AI-powered predictive analytics enable Rvb teams to shift from reactive decision-making to proactive strategies, enhancing efficiency and competitiveness.
Example of Predictive Analytics Application in Rvb
Scenario | AI-Powered Insight | Outcome |
---|---|---|
Supply Chain Disruptions | Predictive model forecasts delays based on historical weather patterns and supplier data. | Proactive adjustments in inventory management, reducing delays by 30%. |
Market Demand Shifts | AI identifies patterns in customer behavior and forecasts demand changes. | Optimized product allocation, increasing sales by 15% during high-demand periods. |
Customizing Rvb Solutions with Artificial Intelligence for Precise Results
Artificial Intelligence (AI) is transforming the way customized solutions in the Rvb (Red, Green, Blue) color space are developed. By leveraging machine learning models, organizations can now create tailored color processing solutions that meet specific requirements for visual representation, enhancing user experience and ensuring more accurate and effective outputs. AI's role in this field is expanding, making it possible to refine Rvb solutions in ways that were previously not achievable through traditional methods.
Machine learning algorithms can now be trained to adjust color schemes, balance lighting, and optimize the overall color accuracy based on precise target outcomes. This ability to apply AI models allows for dynamic changes in real-time, making Rvb applications in design, video production, and other fields more adaptive and intelligent. Whether it's for a gaming environment or visual branding, AI-powered systems can create highly specific results based on users' needs.
Key Techniques for Customization
- Pattern Recognition: AI can analyze color patterns in various environments, adjusting Rvb values based on existing visual data.
- Context-Aware Optimization: AI can adapt color schemes to suit different contexts, such as lighting conditions or viewer preferences.
- Real-time Adjustments: Machine learning models enable real-time color modification, creating dynamic visuals that respond to changes in user input.
"AI helps designers fine-tune color parameters to achieve precise, user-centered outcomes that were previously difficult to realize."
Process for Implementing AI in Rvb Customization
- Data Collection: Gather sample color datasets from diverse environments to train AI models.
- Model Training: Use machine learning to teach models how to interpret and adjust Rvb values for various situations.
- Testing & Refining: Continuously test and refine the system to ensure the generated colors meet specific aesthetic or functional goals.
Example of AI Customization in Rvb Solutions
Scenario | AI Application | Result |
---|---|---|
Video Production | Real-time color adjustments for lighting variations | Enhanced visual quality and consistency across different scenes |
Game Design | Adaptive Rvb values based on user preferences | Increased user engagement and satisfaction |
Digital Branding | Personalized color optimization based on viewer data | Stronger brand identity and user connection |