Machine Learning in 2025
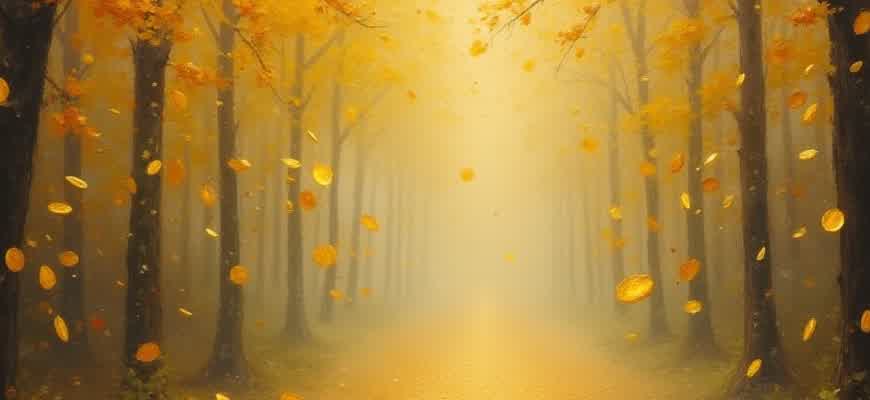
By 2025, machine learning has transitioned into an essential tool across various industries, unlocking new possibilities in data processing, automation, and decision-making. Below is an overview of the key developments that have reshaped this field:
- Increased automation: AI systems now autonomously handle tasks that once required human input, from medical diagnostics to customer service.
- Smarter algorithms: Machine learning models have become significantly more efficient, enabling real-time data analysis at unprecedented speeds.
- Better data integration: The ability to seamlessly integrate structured and unstructured data has opened new avenues for predictive analytics.
“Machine learning in 2025 is not just about processing data–it's about understanding and predicting patterns with remarkable precision.”
Here are the primary areas where machine learning is making its most significant impact:
- Healthcare: AI-driven tools are improving patient care through personalized treatment plans and early disease detection.
- Finance: Financial institutions leverage ML for fraud detection and risk management, ensuring more secure transactions.
- Autonomous Vehicles: Machine learning systems power self-driving cars, enabling safer roads and more efficient transportation networks.
Sector | Impact |
---|---|
Healthcare | AI-based diagnostic systems enhance accuracy and reduce human error. |
Finance | Improved fraud detection, predictive analytics, and smarter investment decisions. |
Transportation | Efficient traffic flow and reduced accidents through autonomous driving technologies. |
Advancements in Automated Data Processing for Business Applications
The automation of data processing in business environments has seen remarkable improvements over the past few years. By 2025, businesses are leveraging more sophisticated tools that streamline the way data is collected, analyzed, and applied to decision-making. These advancements are driven by the increased use of AI models, enhanced algorithms, and cloud infrastructure, which allow for faster, more accurate processing of large datasets. This shift is especially impactful in industries such as finance, healthcare, and marketing, where timely and precise data insights are critical for operational efficiency and competitive advantage.
Automated systems have significantly reduced the time required to process vast amounts of data, enabling businesses to make informed decisions in real-time. These systems not only analyze historical data but also offer predictive insights that can guide future strategies. The integration of machine learning into these processes ensures continuous improvement in data accuracy and relevance, further boosting the value businesses gain from their data assets.
Key Technological Developments
- AI-Driven Data Cleaning: AI technologies are now capable of automatically detecting and correcting errors in datasets, ensuring higher data quality for businesses.
- Real-Time Data Processing: Advanced algorithms allow for immediate analysis and insights, enabling faster decision-making and operational adjustments.
- Cloud-Based Solutions: Businesses now rely on cloud platforms to handle the computational load of data processing, enabling scalability and flexibility.
Applications in Various Industries
- Finance: Real-time fraud detection and risk management are enhanced through automated data processing, enabling swift identification of suspicious activities.
- Healthcare: AI models process patient data to predict medical outcomes, recommend treatments, and improve patient care efficiency.
- Retail: Businesses leverage customer behavior analysis to personalize marketing strategies and optimize inventory management.
Benefits of Automated Data Processing
Benefit | Description |
---|---|
Increased Speed | Real-time data processing enables quicker insights and faster decision-making. |
Cost Efficiency | Automation reduces manual labor and operational costs by streamlining processes. |
Improved Accuracy | AI and machine learning algorithms enhance data accuracy by eliminating human errors. |
"The future of data processing is automated, allowing businesses to shift focus from data management to strategic decision-making."
Implementing Real-Time Predictive Analytics for Competitive Advantage
The application of real-time predictive analytics in 2025 is transforming how organizations approach decision-making and business operations. By integrating advanced machine learning algorithms into their processes, companies can now predict outcomes, identify trends, and optimize strategies in real time. This capability not only helps in reducing operational inefficiencies but also allows businesses to stay ahead of competitors by responding to market changes faster than ever before.
Real-time analytics provide immediate insights into various aspects of business performance. From inventory management to customer behavior forecasting, the possibilities for leveraging these technologies are vast. Companies that can effectively implement real-time predictive models gain the ability to adjust their strategies dynamically, enhancing their overall competitiveness in the market.
Key Benefits of Real-Time Predictive Analytics
- Increased Responsiveness: Companies can react to changes in demand or market conditions instantly, ensuring optimal decision-making and resource allocation.
- Enhanced Customer Experience: By predicting customer preferences and behaviors, businesses can deliver personalized experiences and improve satisfaction.
- Improved Operational Efficiency: Predictive analytics can identify bottlenecks or inefficiencies, enabling real-time adjustments that streamline operations.
Steps to Implement Real-Time Predictive Analytics
- Data Collection: Gather high-quality, real-time data from various sources, such as IoT devices, CRM systems, and social media.
- Model Training: Develop machine learning models using historical and real-time data to identify patterns and make predictions.
- Deployment and Monitoring: Integrate the models into the business environment and continuously monitor their performance to ensure accuracy.
- Continuous Improvement: Regularly update the models based on new data and feedback to refine predictions and enhance their effectiveness.
“In 2025, companies that harness the power of real-time predictive analytics will be able to make more informed decisions, leading to a stronger competitive edge.”
Example: Predictive Analytics in Retail
Aspect | Traditional Approach | With Real-Time Analytics |
---|---|---|
Customer Demand Forecasting | Based on historical sales data, updated periodically. | Based on real-time consumer behavior and external factors (e.g., weather, events). |
Inventory Management | Replenishment based on past trends and forecasts. | Dynamic adjustments in inventory levels in response to current demand patterns. |
Pricing Strategy | Fixed pricing based on traditional market conditions. | Dynamic pricing based on real-time competitor analysis and customer behavior. |
Impact of Quantum Computing on Machine Learning Models
Quantum computing promises to revolutionize the way machine learning algorithms process and analyze data. By leveraging the principles of quantum mechanics, it introduces new computational capabilities that could significantly enhance the performance and efficiency of machine learning models. Quantum computing's ability to handle complex calculations in parallel could drastically reduce the time required for training models, especially when dealing with large datasets.
The integration of quantum computing into machine learning models could also lead to the development of entirely new algorithms. These quantum-enhanced models would leverage quantum superposition and entanglement to explore data in ways traditional computers cannot, potentially uncovering patterns and insights previously inaccessible.
Potential Advantages
- Increased Speed: Quantum processors can perform multiple calculations at once, drastically reducing the time to train machine learning models.
- Enhanced Optimization: Quantum computers are well-suited for solving optimization problems, which are common in machine learning tasks like feature selection and hyperparameter tuning.
- Improved Data Analysis: Quantum computers can process vast amounts of data simultaneously, enabling more comprehensive and accurate analysis.
Challenges to Overcome
- Quantum Hardware Limitations: Current quantum computers are still in the early stages, with issues like qubit stability and error rates affecting their practical use.
- Algorithm Development: Many machine learning algorithms need to be adapted to quantum systems, requiring new approaches and strategies.
- Scalability: As the number of qubits increases, so does the complexity of managing them, leading to potential scalability issues.
Key Considerations for Machine Learning Models
Quantum computing has the potential to accelerate machine learning by offering new ways to solve complex optimization and data analysis problems, but substantial challenges must be addressed before it becomes mainstream in the field.
Comparison: Classical vs Quantum Computing for Machine Learning
Aspect | Classical Computing | Quantum Computing |
---|---|---|
Processing Power | Limited by traditional binary systems | Exponential growth due to quantum states |
Data Handling | Sequential processing of data | Parallel processing using qubits |
Optimization | Can handle small to medium optimization problems | Solves large and complex optimization problems more efficiently |
Development Complexity | Well-established algorithms and techniques | Requires new quantum algorithms and research |
How Autonomous Systems Are Shaping the Future of Machine Learning
Autonomous systems have become a driving force in the evolution of machine learning, particularly in areas requiring high levels of decision-making, adaptation, and real-time processing. These systems are revolutionizing industries such as transportation, healthcare, and robotics by enabling machines to learn and adapt independently, often without direct human intervention. With AI models embedded into autonomous systems, the demand for advanced machine learning techniques has skyrocketed, prompting continuous innovation in areas like reinforcement learning and unsupervised learning.
As these systems become increasingly sophisticated, their ability to operate autonomously across a wide range of environments is changing how machines learn, interact with the world, and improve their performance over time. The integration of deep learning models into autonomous systems, paired with real-time data processing, is paving the way for more efficient, accurate, and scalable AI solutions.
Key Impacts of Autonomous Systems on Machine Learning
- Continuous Learning: Autonomous systems learn from real-time experiences, refining their models as they gather more data from their environment.
- Data Efficiency: These systems improve the efficiency of data usage, requiring less manual intervention while simultaneously increasing the quality of the input data they process.
- Improved Decision-Making: By leveraging reinforcement learning and adaptive algorithms, autonomous systems can make decisions that are better suited to dynamic, unpredictable scenarios.
Challenges and Opportunities in Autonomous System Development
- Data Security and Privacy: As autonomous systems become more widespread, the need for secure data handling and privacy protection becomes more critical.
- Model Transparency: Ensuring that autonomous systems provide explainable and interpretable decisions will be crucial in building trust with users and stakeholders.
- Integration with Existing Infrastructure: Autonomous systems need to seamlessly integrate with legacy systems, which often requires overcoming significant technical and regulatory hurdles.
Autonomous Systems in Machine Learning: A Comparative Overview
Aspect | Traditional Systems | Autonomous Systems |
---|---|---|
Data Usage | Dependent on large datasets curated by humans | Learn from real-time data, improving efficiency |
Decision-Making | Requires human intervention for most complex tasks | Can make decisions without human input, based on learned experiences |
Adaptability | Limited adaptability to changing conditions | Highly adaptive, able to learn from new and dynamic environments |
"Autonomous systems not only redefine how machines learn but also enhance the scope and accuracy of AI applications across diverse sectors."
Challenges in Training Large-Scale AI Models in 2025
As AI models continue to grow in complexity and scale, the challenges associated with their training have become more pronounced. In 2025, several factors are influencing the efficiency and feasibility of training large AI systems. These challenges are not only related to hardware and computation but also to data management, ethical concerns, and the increasing demand for model interpretability.
One of the main obstacles is the enormous computational cost required for training such models. This has led to a reliance on highly specialized infrastructure, such as advanced GPUs and distributed computing systems, which can handle the processing requirements. However, as the demand for larger models increases, so does the difficulty in scaling this infrastructure while keeping costs under control.
Key Challenges in Training Large AI Models
- Resource Constraints: AI models are becoming so large that traditional computing hardware struggles to keep up. The need for high-performance GPUs and specialized accelerators requires significant investment in infrastructure.
- Data Quality and Availability: Large-scale models require vast amounts of high-quality data, which often presents challenges in sourcing, cleaning, and ensuring privacy standards.
- Ethical Considerations: As AI models become more advanced, ensuring fairness, transparency, and accountability in their decisions becomes harder, especially when training data may introduce biases.
Technological Barriers
- Energy Consumption: The carbon footprint of training large-scale AI models is a growing concern. More efficient algorithms and hardware are needed to reduce energy consumption during training.
- Scalability of Algorithms: While parallel computing has made strides, scaling algorithms to efficiently use thousands of processing units without sacrificing performance remains a challenge.
- Latency Issues: As models increase in size, real-time application performance can degrade, leading to slower responses and delays in deployment.
The cost of training AI models is expected to rise exponentially as the size of the models increases, making it unsustainable for smaller organizations to compete in the AI race.
Infrastructure Costs Breakdown
Resource Type | Cost Estimate (USD) |
---|---|
High-Performance GPUs | $100,000+ per unit |
Cloud Computing Services | $10,000 per month for large-scale models |
Data Storage and Management | $50,000+ per year |
AI-Driven Personalization: Changing Customer Experiences
In 2025, businesses are leveraging advanced machine learning algorithms to create deeply personalized experiences for their customers. These AI-driven systems analyze vast amounts of data, uncovering unique patterns in user behavior, preferences, and interactions. With this information, companies can offer tailored content, services, and recommendations that feel more natural and relevant to the individual.
The shift toward personalization is reshaping industries such as e-commerce, entertainment, and healthcare. Rather than relying on broad, one-size-fits-all strategies, businesses are adopting AI to create custom-tailored journeys that enhance customer satisfaction and foster brand loyalty.
Key Advantages of AI-Powered Personalization
- Real-time adaptation: AI systems continuously analyze user data to adjust recommendations instantly, ensuring the experience evolves in real time.
- Enhanced user engagement: Personalized content results in higher levels of interaction and customer retention.
- Increased conversion rates: By delivering relevant suggestions, businesses can increase the likelihood of customer purchases and decision-making.
"AI personalization isn’t just about showing the right products – it’s about building a relationship with the customer that feels intuitive and personal."
Example: E-Commerce Recommendations
In e-commerce, AI-powered recommendation engines are a prime example of personalized experiences. These systems use machine learning algorithms to suggest products based on previous browsing history, purchase patterns, and even social media activity.
Data Type | Action Taken | Result |
---|---|---|
Browsing History | Suggest related products | Increased likelihood of purchase |
Purchase Patterns | Offer discounts or bundles | Higher average order value |
Social Media Activity | Recommend products based on shared interests | Improved customer engagement |
Ethical Implications of Machine Learning Algorithms in 2025
As machine learning technologies continue to evolve, it is essential to address the ethical challenges they present. In 2025, machine learning algorithms will have greater autonomy in decision-making processes, impacting various sectors, including healthcare, finance, and public safety. With the growing reliance on AI for decision support, ensuring that these algorithms act in a fair, transparent, and accountable manner becomes increasingly crucial.
The ethical concerns surrounding machine learning algorithms revolve around fairness, privacy, and accountability. Bias in training data and decision-making processes can lead to harmful consequences, such as reinforcing existing inequalities. Furthermore, the opacity of some advanced algorithms makes it difficult to trace decisions back to their causes, undermining trust and transparency. In addition, the collection of sensitive data by AI systems raises significant privacy risks, especially when that data is mishandled or misused.
Key Ethical Considerations
- Bias and Discrimination: Machine learning models may perpetuate or even exacerbate societal biases if trained on skewed data.
- Privacy Concerns: Collecting large volumes of personal data without proper consent can result in violations of individual privacy rights.
- Accountability and Transparency: The complexity of certain algorithms makes it difficult to understand how decisions are made, which raises concerns about accountability when errors occur.
- Job Displacement: Automation driven by AI has the potential to disrupt labor markets and displace workers, raising ethical questions about societal responsibility and economic inequality.
Approaches to Mitigate Ethical Risks
- Ensuring Fairness: Incorporating fairness metrics into model training to identify and mitigate biases before deployment.
- Data Privacy Protection: Implementing robust data encryption and ensuring user consent for data usage in line with evolving privacy laws.
- Promoting Transparency: Developing explainable AI models that allow for clear understanding of decision-making processes.
- Regulation and Oversight: Establishing frameworks for monitoring and regulating AI systems to ensure ethical standards are upheld.
"Ethical machine learning in 2025 will require a combination of technological innovation, policy development, and active engagement with diverse stakeholders to ensure that AI benefits society as a whole."
Potential Impact on Society
Sector | Ethical Concerns | Impact |
---|---|---|
Healthcare | Bias in diagnostic tools | Unequal access to care and misdiagnosis |
Finance | Unfair credit scoring models | Increased inequality in financial access |
Public Safety | Inaccurate risk assessment algorithms | Discrimination and wrongful decisions |