Intelligence Learning Network
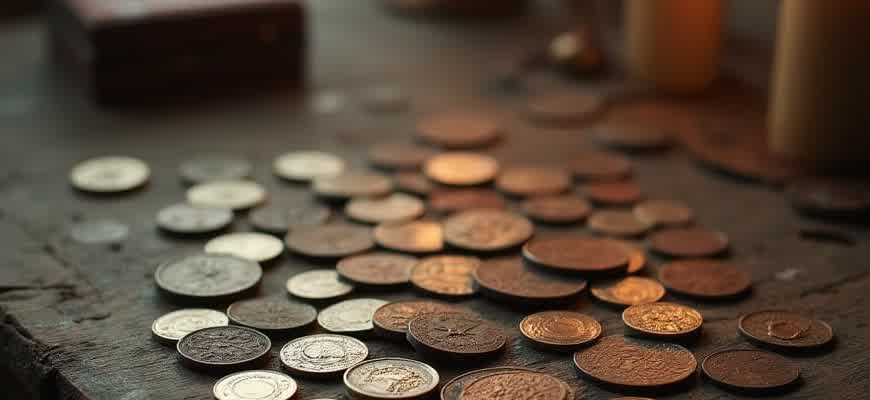
The concept of intelligent learning networks refers to systems that integrate machine learning algorithms, data analytics, and real-time feedback to improve educational processes. These networks utilize interconnected devices, software tools, and platforms to enhance personalized learning experiences. Unlike traditional educational approaches, these networks are adaptive, continuously evolving to meet the unique needs of each learner.
Key components of intelligent learning networks include:
- Data collection and analysis tools
- Real-time feedback mechanisms
- Personalized learning pathways
- Integration of AI-driven technologies
Some examples of how intelligent learning networks operate:
- Personalized course recommendations based on student performance data
- AI-driven tutors providing assistance in real-time
- Automated assessment tools offering instant feedback
"An intelligent learning network is not just a passive repository of content; it actively adapts to learner needs, creating a dynamic and personalized learning journey."
These networks aim to bridge the gap between traditional classroom-based teaching and the evolving demands of the digital age.
Component | Description |
---|---|
Data Analytics | Analyzing student performance to adjust learning paths |
Adaptive Learning | Personalizing the curriculum to the learner's needs |
Artificial Intelligence | Supporting learners through AI-driven tools and tutors |
How to Integrate AI into Your Learning Network
Incorporating AI into your learning network can transform the way knowledge is delivered, personalized, and analyzed. By leveraging AI, organizations and educational institutions can create smarter, more adaptive systems that cater to the individual needs of learners. This allows for the automation of routine tasks and enhances decision-making processes by providing insights based on data analysis.
To successfully integrate AI, it is essential to follow a structured approach. This involves selecting the right tools, creating an infrastructure that supports AI technologies, and training both the system and the users on how to work with them effectively. Below are key steps to ensure the integration of AI into your learning network is both efficient and impactful.
Key Steps to Integrating AI into Your Learning Network
- Identify Learning Objectives: Clearly define the goals you want to achieve with AI, whether it's personalized content delivery, automated assessments, or improved student engagement.
- Choose AI Tools: Select AI-powered platforms or applications that align with your objectives. Popular options include learning management systems (LMS) with AI features or specialized tools for data analysis and feedback automation.
- Prepare Infrastructure: Ensure your network infrastructure can support the demands of AI, including data storage, processing power, and security measures.
- Train Stakeholders: Provide training to instructors, students, and administrators on how to use AI tools effectively, including understanding AI-generated insights and how to adapt learning strategies accordingly.
AI Implementation Strategy
- Data Collection: Gather data from various learning sources, such as student performance, engagement levels, and feedback. This data forms the basis for AI-powered recommendations and analysis.
- AI Model Development: Work with AI developers to create or customize models that can process the collected data and deliver personalized learning experiences or recommendations.
- Monitor and Evaluate: Continuously assess the effectiveness of AI integration by tracking learning outcomes and adjusting AI models based on performance data.
Important Note: Successful AI integration requires ongoing adaptation. The learning network must evolve to meet new challenges and incorporate advancements in AI technology. Regular feedback loops are crucial to maintaining relevance and effectiveness.
AI Integration in Practice
AI Tool | Purpose | Benefits |
---|---|---|
Adaptive Learning Platforms | Personalize learning paths based on individual progress. | Increased engagement, improved retention rates. |
Automated Grading Systems | Grade assignments and assessments using AI algorithms. | Time-saving, objective assessments, real-time feedback. |
Learning Analytics Tools | Analyze learner data to provide insights on performance. | Informed decision-making, tailored support for students. |
Tracking Learner Progress: Tools and Metrics in the Network
In the context of an Intelligence Learning Network, monitoring the progress of learners is crucial to ensure continuous development and effective adaptation of learning paths. The integration of diverse tracking tools allows for the real-time assessment of performance and engagement, making it possible to fine-tune educational experiences based on individual needs. These tools provide valuable insights into learner behavior, understanding, and retention, which are essential for optimizing both content delivery and interaction within the network.
Different types of metrics are employed to track a learner's journey through the system. These metrics focus not only on the quantity of learning but also on the quality of engagement and the depth of understanding. By using various tracking mechanisms, the system can provide detailed reports and feedback, which help instructors or automated systems adjust learning materials dynamically to suit individual learning curves.
Key Tools for Tracking Learner Progress
- Learning Analytics Dashboards: Real-time data visualizations that display learner activity, performance, and engagement levels.
- Competency-Based Progress Tracking: Metrics that evaluate mastery of specific skills or knowledge areas rather than just completion of content.
- Adaptive Learning Systems: Tools that adjust content and difficulty based on the learner’s progress and mastery level.
- Behavioral Analytics: Tracking of learner interactions with the platform, such as time spent on tasks, activity completion, and participation.
Key Metrics Used to Evaluate Learner Progress
- Engagement Rate: Measures how actively learners interact with the learning content, including frequency of logins and participation in discussions.
- Completion Time: Tracks how long learners take to complete modules, assignments, and assessments, providing insight into their understanding pace.
- Assessment Scores: Evaluates the learner's performance on quizzes, tests, and assignments to gauge knowledge retention.
- Feedback Frequency: Monitors the number and quality of feedback provided to learners, helping instructors or automated systems identify potential areas for improvement.
Importance of Tracking Progress
"Continuous monitoring of learner progress is essential for ensuring the educational experience remains adaptive and personalized, allowing each learner to reach their full potential."
Example of Tracking Tools and Metrics
Tool | Metric | Purpose |
---|---|---|
Learning Analytics Dashboard | Engagement Rate | Tracks user activity and interaction with learning materials in real-time. |
Competency-Based System | Skills Mastery | Evaluates the learner’s proficiency in specific competencies or knowledge areas. |
Adaptive Learning Systems | Completion Time | Adjusts content based on the learner’s progress and time spent on tasks. |
Customizing Learning Paths for Different Skill Levels
Adapting learning experiences for various skill levels is crucial for optimizing personal development. Tailored learning paths ensure that learners are not overwhelmed by overly complex material or, conversely, bored with content that is too simplistic. By aligning educational content with individual competencies, the learning process becomes more engaging and efficient.
Effective customization of learning paths involves identifying the learner’s current knowledge, strengths, and areas for improvement. Based on this data, educational frameworks can be designed to progress logically from one level to the next, providing meaningful challenges and opportunities for growth.
Key Considerations for Creating Tailored Learning Paths
- Skill Assessment: Accurate evaluation of existing competencies helps in designing the right starting point.
- Content Progression: Learning paths should evolve in complexity to match the learner’s pace.
- Engagement Strategies: Interactive and varied content types maintain interest across different levels.
Steps for Designing Customized Learning Paths
- Perform a comprehensive skill gap analysis.
- Design modular content that allows for flexibility in progression.
- Integrate adaptive technologies to monitor progress and suggest adjustments.
- Provide regular feedback and assessments to guide learners through each stage.
“Tailoring learning paths to individual skill levels promotes a more personalized and effective educational experience, ensuring optimal outcomes for every learner.”
Example of a Customized Learning Path Structure
Skill Level | Learning Focus | Content Delivery |
---|---|---|
Beginner | Fundamentals and basic concepts | Interactive videos, quizzes |
Intermediate | Application of core principles | Case studies, group discussions |
Advanced | Expert-level challenges and problem-solving | Simulations, real-world projects |
Maximizing User Engagement through Interactive Tools in the Network
Effective user engagement within an intelligence-based learning network is directly linked to the integration of interactive elements. These features are designed to foster active participation, making the experience more immersive and personalized. The key to engagement lies in offering users dynamic tools that not only provide valuable content but also encourage collaboration and communication across the platform.
By leveraging interactive features, users can enjoy a more hands-on approach to learning. These tools promote deeper involvement, allowing learners to customize their experience, track progress, and even contribute content. Let’s explore the most effective features that can be utilized to maximize engagement in such networks.
Key Interactive Features to Boost Engagement
- Real-time Collaboration: Tools like chat rooms, forums, and live webinars encourage immediate interaction among users, enhancing peer-to-peer learning.
- Gamification: Incorporating game elements such as leaderboards, achievements, and rewards can motivate learners to consistently participate and challenge themselves.
- Personalized Dashboards: Customizable interfaces where users can track progress, set goals, and receive tailored content recommendations.
- Interactive Quizzes and Assessments: Providing instant feedback through quizzes and interactive tests helps users retain information and stay engaged.
Strategies for Engaging Users in a Learning Network
- Foster a sense of community: Encouraging group activities and discussions ensures that learners feel connected, enhancing their overall experience.
- Provide timely and relevant feedback: Real-time feedback during interactive activities ensures that users understand their progress and areas for improvement.
- Facilitate continuous learning: Ensure that users are consistently provided with challenges and new opportunities to expand their knowledge.
Important Considerations for Implementation
Feature | Benefit | Example |
---|---|---|
Live Q&A Sessions | Encourages immediate learner engagement and knowledge clarification. | Weekly instructor-led webinars where learners can ask questions in real-time. |
Peer Review Systems | Allows learners to assess each other’s work, promoting critical thinking and collaboration. | Platform where learners submit projects and receive peer feedback. |
Progress Tracking Tools | Helps users monitor their learning journey and stay motivated. | Dashboards showing completed modules, achievements, and milestones. |
Engaging learners through interactive features not only enhances their experience but also ensures they are more likely to return and continue their educational journey.
Scaling Your Learning Network: Best Practices for Growth
As organizations and individuals seek to expand their intellectual capacity, the growth of a learning network becomes essential. Expanding such networks allows for more diverse insights, access to a wider pool of expertise, and better problem-solving capabilities. However, scaling a learning network requires careful planning, a structured approach, and ongoing adjustments to accommodate new members, ideas, and methods of interaction.
Effective scaling involves both technological solutions and human-centered strategies to foster collaboration. Here are several best practices that can help guide the expansion of a learning network:
Key Practices for Scaling
- Leverage Collaborative Tools: Use platforms that allow seamless interaction, such as learning management systems (LMS), discussion forums, and collaborative workspaces.
- Encourage a Culture of Knowledge Sharing: Promote a mindset where sharing expertise is seen as valuable, and reward contributions that benefit the broader community.
- Implement Modular Learning: Break down learning materials into digestible pieces that can be easily updated, scaled, and personalized for diverse members.
- Ensure Diversity of Thought: Actively recruit individuals with diverse skills and perspectives to ensure that the network remains dynamic and innovative.
Step-by-Step Guide to Scaling
- Assess Current Network Strengths and Weaknesses: Before expanding, analyze the current state of your learning network to understand what’s working and what needs improvement.
- Invest in Robust Infrastructure: Ensure that both physical and digital resources are capable of handling increased activity and data flow.
- Foster Engagement Through Incentives: Create systems that encourage members to engage frequently, such as recognition programs, badges, and other gamified elements.
- Regularly Evaluate and Adjust Strategies: Continuously measure the impact of growth strategies and be prepared to adjust as needed.
"Scaling a learning network is not just about increasing size, but enhancing the quality and depth of knowledge exchanges. A larger network should still feel cohesive and supportive."
Considerations for Scaling: Key Metrics
Metric | Importance |
---|---|
Engagement Rate | Measures how actively members are interacting with content and each other. |
Knowledge Retention | Indicates the effectiveness of the learning material and how well it is retained by members. |
Member Growth Rate | Tracks the pace at which new participants are joining the network. |
Content Relevance | Ensures that the materials being shared are up-to-date and aligned with member needs. |