Ai Enabled Analytics
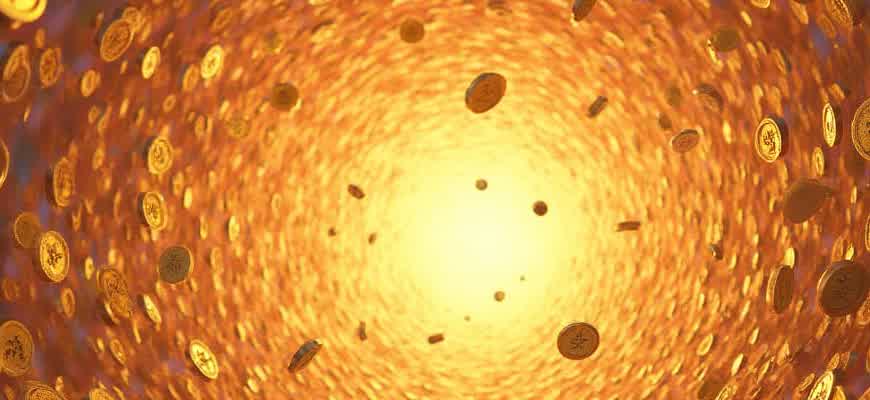
Artificial Intelligence (AI) has significantly transformed the landscape of data analysis, enabling more sophisticated, accurate, and real-time insights. Traditional methods of data analysis often relied on manual processes or basic statistical techniques, which could only process limited volumes of data and lacked the ability to identify complex patterns. AI-powered analytics, on the other hand, leverages machine learning algorithms to analyze vast datasets and predict trends with a level of precision previously unattainable.
Key Components of AI-Driven Analytics:
- Data Collection: Continuous stream of real-time data is gathered from various sources, including IoT devices, sensors, and user interactions.
- Data Processing: AI models automatically clean, filter, and process raw data to ensure it is suitable for analysis.
- Pattern Recognition: Machine learning algorithms detect hidden patterns, correlations, and outliers that traditional methods might miss.
Types of AI Analytics Applications:
- Predictive Analytics: Uses historical data to forecast future trends and outcomes.
- Prescriptive Analytics: Recommends actions to optimize decision-making based on data insights.
- Descriptive Analytics: Summarizes past data to understand what happened and why.
AI-powered tools enhance the speed and accuracy of analysis, allowing businesses to make data-driven decisions in real-time, increasing both operational efficiency and competitive advantage.
Below is an overview of the capabilities of AI-based analytics tools:
Capability | Description |
---|---|
Real-time Analysis | AI systems can process and analyze data as it is collected, enabling immediate decision-making. |
Scalability | AI tools can handle large datasets, providing insights across diverse business functions. |
Automated Reporting | AI tools can generate detailed, insightful reports without manual input, saving time and resources. |
AI-Driven Analytics: Unlocking Business Potential
AI-driven analytics is rapidly transforming the way businesses derive insights from data. By combining artificial intelligence with data analysis, companies can now uncover patterns, predict trends, and automate decision-making processes more efficiently than ever before. This shift is enabling organizations to stay ahead of competitors by leveraging data as a strategic asset.
With AI-enabled analytics, businesses can enhance their operations across various domains, including marketing, customer service, and supply chain management. By integrating advanced algorithms, businesses can optimize their strategies and discover new growth opportunities that were previously hidden within vast datasets.
Key Benefits of AI-Driven Analytics
- Improved Decision Making: AI models can process large volumes of data and identify patterns that might not be apparent to human analysts, leading to more informed and accurate business decisions.
- Predictive Insights: AI-powered tools can forecast future trends and behaviors, helping businesses proactively respond to market shifts and customer needs.
- Operational Efficiency: Automation of repetitive tasks and data processing helps reduce costs and frees up resources for more strategic activities.
Real-World Applications of AI-Driven Analytics
- Customer Segmentation: AI models analyze consumer behavior to identify distinct customer groups, enabling businesses to tailor marketing efforts.
- Supply Chain Optimization: By predicting demand and optimizing inventory, AI-driven analytics help companies reduce waste and improve delivery timelines.
- Risk Management: AI helps in detecting potential risks by analyzing historical data, allowing companies to take preventive actions.
AI-driven analytics empowers businesses to unlock previously hidden opportunities and streamline their operations, resulting in a significant competitive advantage.
AI Analytics in Action
Application | Benefit | Impact |
---|---|---|
Customer Insights | Improved targeting | Increased sales and customer loyalty |
Fraud Detection | Enhanced security | Reduced losses from fraud |
Operational Efficiency | Cost savings | Higher profitability |
Integrating AI Analytics into Your Existing Systems
Incorporating AI-powered analytics into your current systems can significantly enhance decision-making processes by providing more accurate insights and predictions. However, it requires careful planning and execution to ensure a seamless integration. The process involves aligning AI capabilities with your existing data infrastructure, ensuring compatibility with business workflows, and adapting to evolving technological standards.
The first step is to identify the key areas where AI can deliver value. This could include automating repetitive tasks, providing predictive insights, or optimizing resource allocation. Once the opportunities are clear, you can move forward with integration in a structured manner, balancing innovation with system stability.
Steps to Integrate AI Analytics
- Assess Current System Capabilities: Evaluate your existing data infrastructure, tools, and workflows to identify gaps and opportunities for AI integration.
- Choose the Right AI Tools: Select AI solutions that are compatible with your current system, considering factors such as scalability, flexibility, and data requirements.
- Data Preparation: Cleanse and preprocess your data to ensure it is in a format suitable for AI models. This includes eliminating noise and filling in missing values.
- Implementation & Testing: Deploy the AI models in stages, conducting thorough testing at each phase to ensure that they function as expected without disrupting current processes.
- Monitor & Optimize: Continuously track the performance of AI models and optimize them based on feedback and evolving business needs.
Integrating AI analytics is not a one-time project, but an ongoing process that requires constant optimization to maximize its value.
Key Considerations
- System Compatibility: Ensure the AI tools integrate smoothly with existing software solutions and infrastructure.
- Data Security: Safeguard sensitive data during AI model deployment and ensure compliance with regulations such as GDPR.
- Scalability: Plan for the future by choosing AI tools that can grow with your business demands.
Example of AI Integration in Analytics
Step | AI Integration Approach | Expected Outcome |
---|---|---|
Data Preparation | Data cleansing and feature engineering using AI algorithms | Improved accuracy and reliability of data inputs |
AI Model Implementation | Deploying machine learning models for predictive analytics | Real-time, actionable insights for business decisions |
Continuous Monitoring | AI models are continually optimized based on new data | Enhanced model performance and ROI |
How AI Enhances Data Processing Speed and Precision
Artificial Intelligence has significantly reshaped the way organizations handle large datasets, offering improved processing speeds and enhanced accuracy. By utilizing machine learning models, AI can automatically identify patterns, make predictions, and process vast amounts of data much faster than traditional methods. This automation reduces human error and speeds up decision-making processes across industries such as finance, healthcare, and marketing.
One of the key advantages of AI in data processing is its ability to handle both structured and unstructured data. AI systems can quickly analyze raw data, extract meaningful insights, and even clean and organize data in real-time, allowing businesses to make faster and more informed decisions. As a result, companies can stay ahead of the competition by processing large volumes of data with minimal delay.
Key Benefits of AI in Data Processing
- Faster Decision Making: AI enables real-time data processing, which accelerates decision-making and reduces the time spent on manual data analysis.
- Enhanced Accuracy: AI algorithms minimize human error, leading to more accurate data interpretations and predictions.
- Scalability: AI systems can process exponentially larger datasets, making it ideal for big data applications.
How AI Improves Accuracy in Data Processing
AI systems, through continuous learning, adjust to new data patterns, reducing the likelihood of errors and improving predictive accuracy over time.
- Automated Data Cleaning: AI tools can automatically clean data by identifying inconsistencies and outliers, ensuring high-quality input for analytics.
- Predictive Models: By training on historical data, AI models predict future outcomes with higher precision, reducing the risk of forecasting errors.
- Real-Time Error Detection: AI systems can instantly detect anomalies or errors in datasets, correcting them before they impact results.
AI Processing Speed vs. Traditional Methods
Processing Method | Speed | Accuracy |
---|---|---|
Traditional Data Processing | Slow, often requires manual input | Susceptible to human error |
AI-Driven Processing | Fast, real-time analysis | High accuracy with continuous learning |
Using Artificial Intelligence to Detect Emerging Business Patterns in Real-Time
Artificial intelligence (AI) has revolutionized the way businesses analyze trends by providing real-time insights. Through the use of advanced machine learning algorithms, AI can process vast amounts of data quickly and detect patterns that would take humans significantly longer to identify. By integrating AI-powered analytics tools, companies can gain a competitive edge by responding to market changes faster and more accurately.
One of the primary advantages of AI in trend detection is its ability to continuously monitor multiple data streams and make predictions based on historical and current data. This enables businesses to adjust their strategies on the fly, improving decision-making processes across various departments, from marketing to supply chain management.
Key Benefits of AI-Driven Trend Identification
- Speed: AI systems can analyze data in real-time, providing instant insights into emerging trends and customer behavior.
- Scalability: Unlike traditional methods, AI can handle large volumes of data, ensuring businesses can scale their analytics operations as their data grows.
- Accuracy: AI algorithms improve over time, offering more precise trend predictions based on accumulated data and feedback loops.
AI in Action: Real-Time Trend Analysis Examples
- Customer Sentiment Analysis: AI can evaluate social media posts, reviews, and customer feedback to gauge sentiment shifts, helping businesses adapt marketing strategies accordingly.
- Market Demand Forecasting: AI-driven models can predict demand spikes for products or services by analyzing real-time consumer behavior and external factors like economic conditions or weather.
- Competitive Intelligence: AI tools can scan competitor activity and market conditions, providing businesses with early alerts on emerging competitive threats.
Real-Time Analytics Dashboard
Feature | Description | Impact |
---|---|---|
Trend Detection | AI analyzes customer interactions and market data to identify emerging trends in real-time. | Enables quick action to capitalize on new opportunities. |
Predictive Analytics | Machine learning models forecast future business outcomes based on historical and live data. | Improves decision-making with data-driven insights. |
Anomaly Detection | AI flags outliers or unexpected behavior in datasets. | Prevents potential business risks by catching irregularities early. |
"AI allows businesses to remain agile by detecting and responding to new trends as they unfold, without the delay of traditional analysis methods."
Predictive Modeling: Leveraging AI for Future Insights
Predictive modeling has become a cornerstone in the application of AI-powered analytics. By utilizing historical data and advanced machine learning algorithms, businesses are now able to forecast future trends and behaviors with high accuracy. This process involves building statistical models that can predict outcomes such as customer purchases, market shifts, or operational disruptions, providing invaluable insights for decision-making.
The power of AI in predictive modeling lies in its ability to process vast amounts of data and identify hidden patterns that would be impossible for humans to discern. As a result, organizations can act proactively, making informed strategic choices based on predictions rather than reactive responses. The growing integration of AI into business analytics is transforming industries ranging from retail to healthcare by optimizing resource allocation and improving customer experiences.
Key Benefits of AI-Driven Predictive Analytics
- Enhanced Accuracy: AI models leverage large datasets, which increases the precision of forecasts compared to traditional methods.
- Real-Time Insights: AI systems can analyze data continuously, offering real-time predictions that enable immediate action.
- Cost Efficiency: Predictive models help organizations optimize resources, reducing waste and improving ROI.
Common Techniques Used in Predictive Modeling
- Linear Regression: A statistical method for predicting numerical values based on the relationship between variables.
- Decision Trees: A flowchart-like model that splits data into branches to make predictions based on various conditions.
- Neural Networks: Complex algorithms inspired by the human brain, used for recognizing patterns and making predictions in large datasets.
"Predictive analytics enables companies to act on foresight rather than hindsight, offering a competitive edge in an increasingly data-driven world."
Application Areas of Predictive Modeling
Industry | Use Case |
---|---|
Retail | Forecasting customer demand and optimizing inventory levels. |
Healthcare | Predicting patient outcomes and improving treatment plans. |
Finance | Assessing risk and predicting market trends. |
AI-Driven Data Visualization Tools: Enhancing User Understanding
In recent years, artificial intelligence has revolutionized data analysis by introducing advanced visualization tools that significantly improve the way users interpret and interact with complex datasets. These AI-powered tools analyze vast amounts of data and present it in intuitive graphical formats, which help users quickly identify patterns and make informed decisions. By leveraging machine learning algorithms, AI-driven solutions can automatically generate insightful visual representations of data, making them an invaluable resource for businesses and researchers alike.
The integration of AI into data visualization tools has reduced the cognitive load on users by providing more interactive, dynamic, and real-time visualizations. As opposed to traditional charts and graphs, these intelligent platforms offer adaptive visual outputs, based on the data trends and user input. This helps users grasp key insights more efficiently and take proactive actions.
Key Benefits of AI-Powered Data Visualization Tools
- Automated Insights: AI algorithms can instantly analyze data, identify patterns, and suggest trends, allowing users to focus on high-priority tasks.
- Dynamic and Interactive Visuals: These tools provide customizable visualizations that adapt to data changes in real time, making analysis more engaging.
- Enhanced Decision-Making: AI-powered visuals highlight critical data points, helping users make quicker and more informed decisions.
AI-driven tools eliminate the need for manual data interpretation, enabling users to uncover hidden insights with minimal effort.
AI-Enabled Visualization Features
- Predictive Analytics: AI tools can forecast future trends based on historical data and display these predictions in clear, understandable charts.
- Anomaly Detection: These tools can automatically highlight outliers or unusual patterns in data, improving the accuracy of analyses.
- Data Integration: AI solutions can merge and process data from multiple sources, creating a unified visualization that provides a holistic view of the data.
Example of AI-Powered Data Visualization
Feature | Benefit |
---|---|
Real-Time Updates | Immediate insights with minimal delay, improving reaction time in decision-making. |
Automated Trend Detection | Quick identification of key trends without manual intervention, saving valuable time. |
Automating Decision-Making with AI Analytics
Artificial Intelligence is revolutionizing the way businesses approach decision-making by leveraging advanced analytics. AI-driven systems are able to process vast amounts of data in real-time, providing accurate insights that help organizations make informed decisions faster. Through machine learning algorithms, AI models can learn from historical data and adapt to new trends, ensuring that the decision-making process remains agile and data-driven.
The automation of decision-making using AI analytics enables organizations to reduce human error, optimize resource allocation, and respond to market changes with increased speed. By automating repetitive tasks and data processing, businesses can free up valuable time for strategic initiatives and focus on high-level decisions that require human judgment.
Key Benefits of AI in Decision-Making
- Faster Insights: AI can analyze complex datasets in seconds, allowing for quicker response times in critical business situations.
- Reduced Human Bias: AI removes subjective influence from decisions by relying solely on data-driven insights.
- Increased Efficiency: Automating decision-making processes minimizes manual work, freeing up resources for more impactful tasks.
Steps to Implement AI-Powered Decision-Making
- Data Collection: Gather historical and real-time data to train AI models.
- Model Development: Create machine learning algorithms to identify patterns and trends from the data.
- Integration: Incorporate AI models into existing decision-making systems for seamless automation.
- Continuous Monitoring: Regularly evaluate and adjust AI models to ensure they adapt to evolving data.
"AI systems can autonomously make decisions, allowing businesses to optimize operations, predict trends, and respond to market shifts effectively."
Example: AI-Powered Decision-Making in Marketing
AI Task | Result |
---|---|
Customer Segmentation | AI divides customers into distinct groups based on buying behavior, enhancing targeting strategies. |
Campaign Optimization | AI adjusts marketing strategies in real-time based on campaign performance and customer interactions. |
AI-Powered Analytics for Customizing User Experiences
As businesses aim to enhance user engagement, artificial intelligence is increasingly employed to refine customer experiences by analyzing behavior, preferences, and interactions. By leveraging AI-driven data analysis, companies can deliver tailored offerings and personalized services, boosting customer satisfaction and loyalty. These technologies allow businesses to transition from generic service models to individualized customer journeys.
Through advanced AI models, organizations are able to track customer actions across multiple touchpoints, creating a detailed profile of each user. This dynamic data is then processed to predict future needs and preferences, enabling the development of customized recommendations and marketing strategies.
Key Benefits of AI in Personalized Customer Interactions
- Real-Time Personalization: AI tools can analyze customer behavior instantly and adapt the service or content displayed.
- Behavioral Insights: Identifying patterns in user actions allows businesses to predict customer needs and preferences.
- Enhanced Customer Retention: Delivering relevant experiences based on past interactions increases the likelihood of repeat customers.
"AI empowers businesses to move beyond static, one-size-fits-all approaches by utilizing real-time data to create adaptive customer experiences."
Example Use Cases
- Recommendation Systems: AI analyzes past purchases and browsing patterns to suggest products users are more likely to buy.
- Personalized Content: AI-driven analytics adjusts website or app content to match the interests of individual users.
- Customer Support Chatbots: AI chatbots deliver highly relevant responses based on previous customer interactions, enhancing user satisfaction.
Data-Driven Approach to Customer Segmentation
Customer Segment | AI-Powered Insight | Personalization Strategy |
---|---|---|
Frequent Shoppers | Predictive models suggest upcoming products or offers based on past purchases. | Exclusive discounts or loyalty rewards tailored to their preferences. |
New Visitors | AI identifies patterns in browsing behavior to determine interests. | Display introductory offers and personalized product suggestions. |
Measuring the Impact of AI-Driven Analytics
Implementing AI-driven analytics can significantly enhance business decision-making, but to justify the investment, measuring its return on investment (ROI) is crucial. Unlike traditional methods of measuring performance, AI analytics requires a different approach due to its complexity and long-term benefits. To evaluate the success of an AI analytics system, businesses need to consider both immediate and long-term factors that contribute to improved performance.
Key performance indicators (KPIs) such as increased revenue, cost savings, and efficiency gains are essential in determining the ROI of AI analytics. However, tracking the financial impact alone may not provide a complete picture. Companies should also look at indirect benefits such as customer satisfaction and brand reputation improvements.
Key Metrics for Evaluating ROI
- Operational Efficiency: Reductions in time spent on manual data analysis and improved decision-making speed.
- Revenue Growth: Sales increases driven by more accurate forecasting and personalized marketing strategies.
- Cost Reduction: Reduced overhead costs and fewer resources needed for data processing and analysis.
- Customer Retention: Improved customer satisfaction through better-targeted services and products.
For a comprehensive ROI assessment, companies should integrate both quantitative and qualitative results. Financial benefits should be paired with strategic outcomes such as market positioning and brand loyalty.
Steps to Calculate ROI from AI Analytics
- Define Objectives: Clearly outline the business goals that AI analytics is expected to address.
- Measure Baseline Performance: Assess key metrics before AI implementation to establish a comparison point.
- Track Progress: Continuously monitor performance indicators post-implementation and gather data on improvements.
- Quantify Gains and Costs: Assess both the direct and indirect financial impact, including the costs of AI tools, training, and integration.
- Analyze Results: Calculate the ROI by comparing the net benefits against the costs over a predefined period.
Example ROI Calculation Table
Metric | Pre-AI Value | Post-AI Value | Change (%) |
---|---|---|---|
Revenue | $5,000,000 | $6,200,000 | 24% |
Operational Costs | $1,000,000 | $750,000 | -25% |
Customer Satisfaction | 75% | 85% | 13% |