Deep Learning in Higher Education
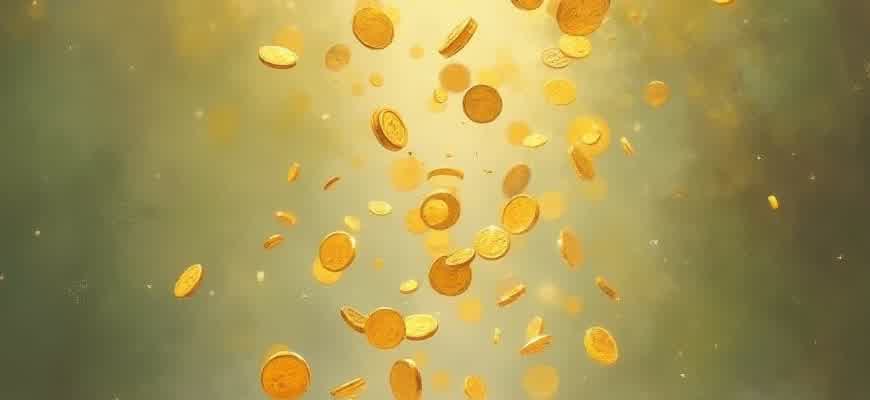
The implementation of machine learning techniques, particularly deep learning, has become increasingly relevant in higher education. By leveraging advanced neural networks, universities are reshaping their teaching methods and research capabilities. These technologies have the potential to not only enhance educational content delivery but also transform administrative processes.
One of the key applications of deep learning in academia is the development of personalized learning experiences. By analyzing student data, deep learning algorithms can predict individual learning needs and provide tailored educational paths. This allows for a more efficient and effective learning process, as well as increased student engagement.
Some significant uses of deep learning in higher education include:
- Automated grading systems: Deep learning models can assess student submissions with higher accuracy and efficiency compared to traditional methods.
- Enhanced research capabilities: Deep learning helps process vast amounts of data, enabling researchers to gain insights faster and more comprehensively.
- Adaptive learning platforms: These platforms adjust content in real-time based on the learner’s progress, enhancing their ability to master complex subjects.
Table 1: Key Areas of Deep Learning Application in Higher Education
Application | Impact |
---|---|
Automated Grading | Reduces administrative workload and improves grading accuracy. |
Personalized Learning | Increases student engagement and learning outcomes. |
Data-Driven Research | Enhances the speed and depth of academic research analysis. |
"The integration of deep learning into higher education is not just about innovation; it is about preparing students for the future by equipping them with the skills and tools required in the rapidly evolving technological landscape."
How Deep Learning Contributes to Research Advancements in Higher Education
Deep learning has emerged as a transformative tool for academic research, enabling universities to enhance their data analysis capabilities and accelerate discovery. By automating complex processes and extracting meaningful insights from large datasets, deep learning models are assisting researchers in various fields such as medicine, social sciences, and engineering. These techniques have allowed scholars to focus on innovative solutions, leaving routine tasks to advanced algorithms.
Moreover, deep learning’s ability to process unstructured data, such as images, videos, and textual information, has expanded the scope of research. The integration of these models into academic projects has resulted in more precise predictions, improved diagnostic tools, and even the development of new theoretical frameworks. Universities are embracing these technologies, incorporating them into curricula, and fostering interdisciplinary collaboration.
Key Benefits of Deep Learning in Academic Research
- Increased Research Efficiency: Automated data analysis accelerates research timelines by processing large volumes of data quickly.
- Enhanced Accuracy: Deep learning models significantly improve the precision of predictions, leading to more reliable research outcomes.
- Cross-Disciplinary Insights: Researchers from different fields can leverage deep learning for collaborative projects, facilitating innovative approaches to solving complex problems.
- Scalability: Deep learning techniques can be applied to a wide range of datasets, from small academic studies to large-scale global research initiatives.
Examples of Deep Learning Applications in University Research
- Healthcare Research: Deep learning models are used to analyze medical images, enhancing diagnostics and treatment planning in academic hospitals.
- Social Sciences: Sentiment analysis tools powered by deep learning help researchers study public opinion and social trends.
- Environmental Studies: Models predict climate changes by analyzing vast datasets related to weather patterns and environmental variables.
Impact on Collaborative Research
"Deep learning facilitates collaboration by providing researchers from different disciplines with a common analytical platform, enabling them to combine insights in ways that were previously not possible."
Research Opportunities in Universities
Field | Application | Benefit |
---|---|---|
Biology | Gene sequencing analysis | Faster identification of genetic markers linked to diseases |
Physics | Particle collision simulation | Improved accuracy in predicting particle behavior |
Literature | Text mining for literary analysis | Uncover hidden patterns in large volumes of text |
Integrating Advanced Neural Networks into STEM Education
Integrating deep learning techniques into STEM education represents a crucial shift in how future professionals are trained. The ability to apply neural networks to complex problems in areas like physics, engineering, and biology opens new pathways for understanding data, predicting outcomes, and solving challenges. Academic curricula must evolve to reflect these developments, incorporating both theoretical concepts and practical applications of machine learning technologies.
For STEM students, mastering deep learning can significantly enhance their problem-solving abilities and equip them with skills highly sought after by industries. However, it requires careful planning to ensure that students gain a well-rounded understanding, from the fundamentals of neural networks to their use in advanced research and industry applications.
Steps for Incorporating Neural Networks into STEM Programs
- Curriculum Revamp: Redesign courses to include key topics like supervised learning, reinforcement learning, and convolutional neural networks (CNNs).
- Hands-On Learning: Offer projects and labs where students can apply deep learning algorithms to real-world datasets.
- Interdisciplinary Approach: Collaborate across departments (e.g., computer science, electrical engineering, biology) to show the diverse applications of deep learning.
Incorporating deep learning requires both the adoption of new tools and methodologies, as well as fostering collaboration between departments to deliver a rich, interdisciplinary learning experience.
"Neural networks have proven to be transformative in fields ranging from climate modeling to medical diagnosis. Their integration into STEM curricula will empower future professionals to drive innovation in ways previously unimaginable."
Key Considerations for Effective Integration
Consideration | Action |
---|---|
Faculty Training | Provide faculty with professional development to keep up with the latest advancements in deep learning. |
Resources & Tools | Ensure access to high-performance computing resources, cloud platforms, and deep learning libraries. |
Collaborative Projects | Encourage cross-disciplinary student teams to apply deep learning to real-world challenges. |
The Impact of AI on Tailored Learning Paths in Higher Education
Artificial Intelligence is revolutionizing the educational landscape by providing students with customized learning experiences. In higher education, AI systems adapt to individual learning styles, making it possible to create more engaging and efficient academic paths. These intelligent systems leverage data to evaluate student progress, identify strengths and weaknesses, and recommend personalized learning strategies. As a result, students can focus on areas requiring improvement while also advancing faster in subjects they excel in.
AI-powered platforms utilize a variety of techniques, including machine learning and natural language processing, to generate personalized educational experiences. These systems continuously learn from student interactions, adjusting content delivery and pacing in real-time. In doing so, AI fosters a more inclusive and accessible learning environment, ensuring that all students, regardless of background or ability, have the opportunity to succeed.
Benefits of AI-Driven Personalization in Education
- Adaptive Learning: AI algorithms adjust course content based on real-time performance, ensuring that students are neither bored by overly easy content nor overwhelmed by difficult material.
- Real-Time Feedback: AI systems provide instant feedback, allowing students to identify mistakes and correct them before moving on to more advanced topics.
- Data-Driven Insights: Educators gain access to detailed analytics that can help identify trends, understand individual student needs, and make data-backed decisions for curriculum improvements.
- Increased Engagement: By offering personalized content, students are more likely to remain engaged, reducing dropout rates and improving overall retention.
Challenges in Implementing AI for Personalization
- Data Privacy Concerns: Collecting and analyzing student data raises important privacy issues that need to be carefully managed to ensure compliance with regulations.
- Cost and Resource Allocation: Implementing AI systems requires significant investment in both technology and training, which may be a challenge for some institutions.
- Quality of Data: AI relies heavily on the quality and accuracy of the data it analyzes, and poor-quality data can lead to suboptimal recommendations or learning pathways.
"AI-driven personalized learning is not just about enhancing student performance, but also about creating a more inclusive and dynamic educational environment that adapts to the needs of each learner."
Example: AI-Powered Personalized Learning System
Feature | Benefits |
---|---|
Adaptive Learning Algorithms | Ensures content is tailored to individual progress, preventing both boredom and frustration. |
Real-Time Feedback | Instant insights help students correct mistakes quickly and improve their understanding. |
Predictive Analytics | Anticipates student needs and suggests additional resources to ensure mastery of topics. |
Automating Administrative Tasks with Deep Learning Models
In recent years, the adoption of deep learning technologies in higher education institutions has significantly streamlined administrative processes. Traditional administrative tasks, such as student enrollment, course scheduling, and grade management, can be time-consuming and prone to human error. By leveraging the power of deep learning models, universities are transforming these processes, improving efficiency and accuracy.
Deep learning algorithms, particularly those based on natural language processing (NLP) and machine learning, can automate many administrative duties that were once manually executed. This not only reduces the workload for staff but also ensures a more consistent and faster service for students and faculty alike.
Key Applications of Deep Learning in Administration
- Automated Enrollment Systems: Deep learning models can process student data and assign courses based on predefined criteria such as prerequisites, availability, and academic performance.
- Grade Prediction and Assessment: AI can assist in grading by analyzing assignments, exams, and projects, offering consistent feedback to students.
- Scheduling Optimization: Deep learning can optimize classroom and exam schedules, taking into account room availability, professor schedules, and student preferences.
Benefits of Automating Administrative Tasks
"By automating repetitive administrative tasks, institutions can focus more on enhancing the learning experience and allocating resources to research and development."
- Time Efficiency: Automation reduces the time spent on manual tasks, allowing staff to focus on more complex, high-value activities.
- Cost Reduction: Less reliance on human intervention can lead to significant cost savings, especially in large institutions.
- Improved Accuracy: Deep learning models minimize the risk of human errors, ensuring higher precision in data processing and decision-making.
Example of Automated Scheduling System
Task | Manual Process | Automated Process |
---|---|---|
Room Assignment | Manual assignment based on availability and capacity | AI model predicts optimal room assignments based on historical data and student preferences |
Course Scheduling | Manual creation of timetables by department | AI system automatically generates schedules considering faculty and student availability |
Leveraging Neural Networks for Forecasting Student Performance
In recent years, the use of neural networks in education has gained traction, particularly in the area of predicting student outcomes. These models, which are capable of processing vast amounts of data, can identify patterns in student behavior, performance, and demographics. By analyzing historical data, neural networks can provide valuable insights into which students are likely to succeed or face challenges, enabling institutions to take proactive steps to support them.
Neural networks, especially deep learning algorithms, have proven to be effective in handling the complexity of educational data. These systems can analyze multiple variables such as grades, attendance, engagement levels, and social factors, combining them to generate predictions about future academic performance. By continuously learning from new data, neural networks refine their accuracy over time, offering increasingly precise forecasts.
How Neural Networks Predict Student Success
Neural networks use a variety of inputs to generate their predictions. These can range from basic demographic information to more intricate data such as engagement metrics and historical academic performance. The following points highlight key factors considered in the prediction process:
- Academic History: Previous grades and performance in different subjects.
- Attendance Rates: Frequency of class attendance, which can indicate student commitment and engagement.
- Behavioral Patterns: Engagement in discussions, participation in extracurricular activities, and other behavioral indicators.
- Psychosocial Factors: Emotional well-being and stress levels, often inferred from surveys or historical data.
Advantages and Applications
The integration of neural networks in predicting student success offers numerous benefits:
- Early Intervention: By identifying at-risk students early, universities can implement targeted interventions.
- Resource Allocation: Neural networks can help institutions allocate resources more effectively, prioritizing students in need of additional support.
- Personalized Learning: Prediction models can also be used to design tailored learning experiences for individual students based on their unique needs.
Example Prediction Model
Input Variables | Weight Assigned | Impact on Success Prediction |
---|---|---|
Attendance Rate | High | Directly correlates with performance trends. |
Previous Grades | High | Strong predictor of future academic success. |
Participation in Extracurriculars | Moderate | Indicates overall engagement and commitment. |
Psychosocial Factors | Moderate | Impacts motivation and study habits. |
"By integrating neural network models, universities can create a more supportive and data-driven environment that benefits both students and educators." – Educational Research Journal
Collaboration Between Universities and Industry in Deep Learning
In recent years, the integration of deep learning technologies has gained significant traction in university-industry collaborations. Universities serve as centers for research and development, while industry partners provide real-world problems and resources. This partnership creates an environment where deep learning models can be applied and refined in practical, innovative ways. Universities benefit from access to large datasets and cutting-edge technology, while industries gain insights into emerging trends and methodologies from academic researchers.
The synergy between academia and industry leads to various collaborative opportunities, including joint research projects, internships, and the development of industry-specific deep learning solutions. The most successful partnerships tend to focus on solving concrete challenges while fostering a knowledge exchange that benefits both sides.
Key Areas of Collaboration
- Joint Research Initiatives: Universities and industries collaborate on long-term research projects, focusing on developing new algorithms and models for real-world applications.
- Data Sharing: Companies provide access to proprietary datasets, allowing academic researchers to improve deep learning techniques using high-quality, domain-specific information.
- Innovation Labs: Many universities establish innovation labs, where students and faculty work with companies to solve specific problems, creating an environment conducive to experimentation.
Benefits of Academic-Industry Partnerships in Deep Learning
- Enhanced Real-World Impact: Research becomes more applicable to current industry needs, accelerating the implementation of deep learning solutions.
- Resource Optimization: Companies gain access to academic expertise, while universities benefit from practical datasets and infrastructure.
- Workforce Development: Students gain valuable industry experience through internships and collaborative projects, equipping them with relevant skills for their careers.
"The fusion of academic knowledge and industry expertise is the key to driving innovation in deep learning, helping to bridge the gap between theoretical advancements and practical applications."
Collaboration Models
Model | Description |
---|---|
Research Consortia | Multiple universities and companies form a consortium to jointly fund and conduct deep learning research on shared topics. |
Internship Programs | Universities provide students with internship opportunities in companies, allowing hands-on experience with deep learning technologies. |
Corporate Sponsorships | Companies sponsor specific university research projects, gaining access to results and contributing to the academic environment. |
Challenges and Solutions in Implementing AI Technologies in Campuses
The adoption of artificial intelligence (AI) in educational institutions presents various hurdles. These challenges encompass technical, infrastructural, and ethical concerns that must be addressed to facilitate seamless integration. One of the primary obstacles is the lack of sufficient technical expertise among faculty and staff to leverage AI tools effectively. Additionally, universities often face issues with the scalability of AI systems, as large campuses require robust and flexible solutions to cater to diverse educational needs.
Moreover, resistance to change from both faculty and students can hinder the progress of AI integration. Concerns about privacy, data security, and the potential loss of human interaction in teaching are also prevalent. Universities must find ways to address these concerns while promoting the benefits of AI technologies in enhancing the educational experience.
Key Challenges
- Technical Expertise Shortage: Many institutions lack the necessary skills and training programs to equip faculty with the knowledge to utilize AI tools effectively.
- Data Security Concerns: AI relies heavily on data, and the handling of sensitive student information can raise concerns regarding privacy and protection.
- Scalability Issues: Implementing AI across large campuses with varying needs requires adaptable systems that can manage the complexities of diverse academic environments.
- Resistance to Change: Traditional educational models can lead to reluctance in adopting AI-driven approaches, especially when they seem to diminish the role of human instructors.
Solutions to Overcome Challenges
- Training and Development: Universities should establish comprehensive training programs to enhance the technical skills of staff and faculty, enabling them to understand and implement AI tools effectively.
- Data Privacy Measures: Institutions must invest in robust cybersecurity frameworks to ensure the protection of student data and build trust in AI technologies.
- Scalable AI Solutions: AI platforms should be designed with flexibility in mind, ensuring that they can be scaled to meet the specific needs of various departments or courses.
- Awareness Campaigns: Educating students and faculty on the benefits and potential of AI in education can help reduce resistance and foster a culture of innovation.
Quote: "Successful integration of AI in education depends not only on technology but on the willingness of educators and students to embrace change and adapt to new ways of learning."
Example of AI Integration in Campuses
University | AI Application | Outcome |
---|---|---|
Stanford University | AI-driven tutoring systems | Improved student engagement and personalized learning experiences |
Massachusetts Institute of Technology (MIT) | AI-powered research assistants | Enhanced research capabilities and time-saving for faculty |