Ai Driven Tutoring Systems
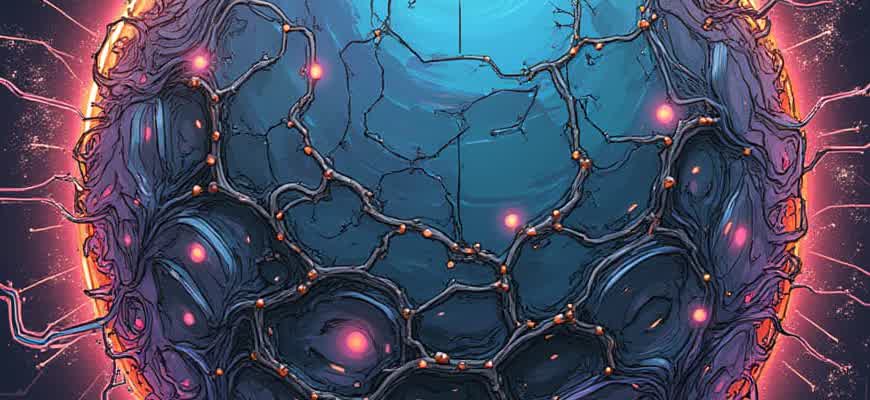
AI-driven tutoring platforms have transformed the educational landscape by providing personalized learning experiences tailored to individual needs. These systems harness advanced algorithms to analyze student performance and adjust content delivery accordingly, creating a dynamic learning environment that evolves as the student progresses.
Unlike traditional methods, AI-supported systems are designed to:
- Offer real-time feedback based on the learner's interactions.
- Adapt to a student's unique pace and learning style.
- Provide targeted content and exercises for skill reinforcement.
"AI-powered tutoring platforms are not simply replacing human tutors, but enhancing learning experiences through immediate, data-driven insights."
These systems integrate various technologies to ensure effective learning:
Technology | Purpose |
---|---|
Natural Language Processing (NLP) | Facilitates communication between the student and the system through conversational interfaces. |
Machine Learning | Analyzes data to customize lessons and predict areas needing improvement. |
Adaptive Learning Algorithms | Adjusts the difficulty and type of content based on the learner’s progress. |
AI-Powered Learning Support: Key Insights for Users
AI-driven tutoring systems are rapidly transforming educational landscapes by providing personalized, scalable learning experiences. These platforms use sophisticated algorithms to adapt to the needs of individual learners, offering tailored feedback and targeted learning resources. With real-time assessments, students can continuously track their progress and receive immediate assistance on areas where they need improvement. This makes AI tutoring a potent tool for enhancing both individual and group learning outcomes.
For users, the practical benefits of AI-based tutoring systems are clear. They can access personalized support at any time, allowing them to learn at their own pace, without the constraints of traditional classroom settings. However, to maximize the effectiveness of these tools, it is important to understand how to interact with them and what advantages they offer over conventional learning methods.
Key Features of AI-Driven Tutoring Systems
- Personalized Learning Paths: AI algorithms assess individual strengths and weaknesses, adjusting the curriculum to provide customized learning experiences.
- Instant Feedback: Students receive immediate responses to their answers, helping to identify areas that require more attention.
- Adaptive Difficulty: The difficulty level of questions adjusts based on the learner's performance, ensuring that challenges are appropriate and motivating.
Best Practices for Using AI Tutoring Systems
- Regular Interaction: Engage consistently with the system to build familiarity and maximize learning outcomes.
- Provide Feedback: AI systems learn from user inputs. Offering feedback can help improve the system's responses over time.
- Monitor Progress: Regularly review your learning progress and adjust goals within the platform to stay on track.
Tip: AI tutors can be especially effective when used as a supplement to traditional learning, combining the benefits of both personalized technology and human instruction.
Considerations When Using AI Tutoring Systems
Pros | Cons |
---|---|
24/7 accessibility | Limited emotional support |
Personalized learning | Relies on algorithm accuracy |
Instant feedback | May not cover all learning styles |
How AI Tailors Learning Experiences for Students
AI-powered tutoring systems are revolutionizing the educational landscape by offering personalized learning experiences. These systems adapt to the individual needs of each student by analyzing their progress, strengths, and weaknesses. The AI uses advanced algorithms to create a learning path that evolves as the student interacts with the system. This continuous adjustment ensures that the student receives the most relevant and effective content at every stage of their learning journey.
One of the key benefits of AI-driven systems is their ability to track and respond to various learning patterns. By collecting data on how a student interacts with the material, the AI can make real-time adjustments to optimize the learning experience. This ensures that the student is neither overwhelmed with difficult content nor bored with material that is too easy.
Methods of Personalization
- Progress Monitoring: AI systems continuously assess student performance, adjusting content difficulty based on real-time results.
- Customized Feedback: Instant, targeted feedback helps students focus on areas of improvement, fostering a deeper understanding of the material.
- Adaptive Learning Pathways: The system modifies the sequence of lessons based on student performance and learning style.
Example of Personalized Learning Path:
Student Performance | AI Response |
---|---|
Poor performance in math concepts | Provides additional exercises focused on foundational math skills. |
Mastery of basic concepts | Introduces more complex problems to challenge the student. |
Strong reading comprehension | Suggests reading material with more complex narratives. |
AI-driven tutoring systems are designed to foster self-paced learning by identifying the optimal point at which a student should advance. These systems ensure that the student's unique learning needs are met, enhancing both engagement and retention.
Understanding the Role of Data in AI-Based Tutoring Systems
AI-driven tutoring systems rely heavily on the data they receive to personalize learning experiences and enhance student performance. The integration of large datasets, including historical student performance, learning styles, and interaction patterns, is critical for the system's ability to adapt and offer targeted educational content. The more precise and relevant the data, the more accurately the AI can tailor lessons to each learner's needs, improving overall engagement and effectiveness.
Data is not only instrumental in identifying students' weaknesses and strengths but also plays a key role in continuously refining the tutoring system. As these systems gather more interaction data, they evolve to become smarter and more efficient, learning from past experiences to improve future outcomes. This process helps ensure that students receive timely and appropriate assistance, optimizing their learning journey.
Types of Data Used in AI-Based Tutoring Systems
- Behavioral Data: Tracks how students interact with the platform, such as time spent on tasks and frequency of corrections made.
- Performance Data: Includes scores, test results, and assessment outcomes that indicate areas of progress or struggle.
- Contextual Data: Information about the learning environment, such as device type or location, can influence the effectiveness of tutoring methods.
- Demographic Data: Age, learning level, and educational background help the system offer more personalized content.
How Data is Processed and Utilized
- Collection: Data is gathered through student interactions with the platform, including responses, clicks, and completion times.
- Analysis: Machine learning algorithms analyze this data to identify trends and patterns that can inform teaching strategies.
- Customization: Based on the analysis, AI systems adjust the difficulty, format, or focus of the material to suit each student's needs.
- Feedback Loop: Continuous data collection enables the system to refine its recommendations and improve its effectiveness over time.
Key Insights from Data-Driven Learning
Data Type | Impact on Learning |
---|---|
Behavioral Data | Helps identify engagement levels and areas where students may lose focus, enabling targeted interventions. |
Performance Data | Provides direct insight into student mastery and highlights topics that require further review. |
Demographic Data | Allows the system to tailor content and pacing according to the learner's background and experience level. |
"The success of AI-based tutoring systems depends not only on the algorithms themselves but also on how well data is collected, analyzed, and used to adapt to the individual learning needs of students."
Cost Comparison: Traditional Tutors vs. AI-Powered Solutions
When comparing the cost of conventional tutoring services and AI-driven educational platforms, several key factors come into play. Traditional tutoring often involves hourly rates, which can accumulate quickly depending on the frequency of sessions. On the other hand, AI-based solutions offer a more scalable and flexible approach, often reducing the financial burden over time by providing continuous access to learning materials and personalized feedback at a fraction of the cost.
Understanding the key differences in cost structure can help learners and parents make an informed decision. Traditional tutors may offer personalized, face-to-face interaction, but their fees can quickly add up, especially when the need for regular sessions arises. AI-powered platforms, however, may offer subscription models or pay-per-use options, allowing learners to access tailored learning experiences without the same ongoing expenses.
Cost Breakdown of Both Options
Factor | Traditional Tutors | AI-Driven Platforms |
---|---|---|
Hourly Rate | $30 - $100+ per hour | Subscription Model ($10 - $30 per month) |
Availability | Limited to scheduled sessions | 24/7 access |
Personalization | Highly personalized, but limited by tutor's expertise | AI adapts to learner's pace and progress |
Key Insights
- Traditional Tutors: Can be expensive due to the hourly billing model.
- AI-Driven Systems: Provide scalable, cost-effective solutions with flexibility and immediate access.
- Flexibility: AI tutoring systems offer 24/7 availability, while traditional tutors are limited by their schedule.
In many cases, AI solutions can provide comparable or even superior levels of personalized learning at a lower overall cost, especially in the long term.
How AI-Driven Educational Platforms Adapt to Varied Learning Styles
AI-powered tutoring systems are designed to cater to the diverse needs of learners by recognizing their unique preferences and adapting the delivery of content accordingly. By leveraging machine learning algorithms, these platforms personalize the learning experience, offering tailored materials and interactive experiences based on each learner's style. The platforms use data-driven insights to identify patterns in the student's interaction with the content, thereby providing more effective support. This ability to accommodate various learning styles is essential for enhancing student engagement and retention.
In educational settings, learners may display different cognitive approaches, such as visual, auditory, reading/writing, or kinesthetic preferences. AI platforms leverage this information to deliver customized content that resonates with each type. These systems are capable of adjusting the format of lessons and exercises to fit the preferred learning style, making the learning experience more intuitive and engaging.
Personalization through Adaptive Learning
AI tutoring platforms employ several techniques to personalize learning and meet individual needs:
- Dynamic Content Adjustment: Based on the student's responses and progress, the AI adjusts the complexity and type of content delivered, ensuring a continuous challenge while avoiding overwhelm.
- Multimodal Learning: Depending on the learner's style, platforms may incorporate a mix of video, audio, and text to engage visual, auditory, and reading/writing learners.
- Real-Time Feedback: AI systems provide immediate responses to student actions, helping them to correct mistakes and deepen understanding promptly.
Engagement and Motivation Techniques
AI platforms utilize strategies to maintain learner motivation and encourage continued progress:
- Gamification: Some AI-driven tutoring systems integrate gamified elements such as points, levels, and rewards to increase learner engagement, especially for kinesthetic learners.
- Progress Tracking: Continuous tracking and reporting on progress allow students to visually track their achievements, fostering a sense of accomplishment and motivating further learning.
"The AI tutoring system adapts in real-time to the student's learning patterns, ensuring that each individual receives the support they need in the format that works best for them."
Example of Learning Style Adaptation
The following table illustrates how an AI tutoring platform might adapt its approach based on different learning preferences:
Learning Style | AI Platform Response |
---|---|
Visual Learners | Interactive diagrams, videos, and infographics are used to present content. |
Auditory Learners | Audio explanations, podcasts, and verbal feedback are incorporated into lessons. |
Reading/Writing Learners | Text-heavy content such as articles, written exercises, and quizzes are emphasized. |
Kinesthetic Learners | Physical activities, interactive simulations, and hands-on tasks are provided to engage learners. |
Enhancing Student Engagement with AI-Driven Feedback Systems
AI-powered feedback systems are rapidly transforming the way educators engage with students. These tools leverage artificial intelligence to provide timely and personalized feedback, improving students' learning experiences and boosting their motivation. By offering instant responses to student queries and assignments, AI systems allow for more interactive and individualized instruction. This results in increased engagement, as students receive guidance tailored to their unique learning needs and progress.
One of the key aspects of AI-driven feedback is its ability to deliver targeted suggestions that can address specific learning gaps. Unlike traditional methods, which often involve generalized comments, AI tools provide real-time, actionable feedback that encourages students to reflect on their performance and make improvements. This approach fosters a growth mindset, where students are motivated to continue learning and refining their skills.
Types of AI Feedback Mechanisms
- Instant feedback: Immediate corrections on assignments or quizzes, enabling students to adjust their understanding in real-time.
- Progress tracking: Continuous monitoring of performance that helps both students and instructors identify areas of improvement.
- Personalized learning paths: Adaptive systems that suggest customized learning activities based on the student's strengths and weaknesses.
Benefits of AI-Enhanced Feedback
- Improved learning retention: Instant, relevant feedback strengthens students' memory retention by reinforcing correct concepts.
- Increased motivation: Timely praise and constructive criticism encourage students to stay engaged and motivated throughout their learning journey.
- Self-paced learning: AI-driven feedback allows students to progress at their own speed, reducing the pressure of traditional classroom settings.
Key Metrics in Feedback Efficiency
Metric | Traditional Feedback | AI-Driven Feedback |
---|---|---|
Response Time | Delayed (up to a few days) | Instantaneous |
Personalization | Generalized Comments | Highly Tailored Suggestions |
Engagement Level | Passive | Active and Interactive |
"The integration of AI in feedback mechanisms allows for real-time guidance, which has proven to significantly enhance student participation and retention in learning activities."
Real-Time Performance Monitoring: Benefits for Educators
In the context of AI-driven tutoring systems, real-time monitoring of student progress offers significant advantages for educators. It provides an efficient way to track and analyze individual performance, enabling educators to adjust their teaching strategies accordingly. This dynamic feedback loop ensures that both students and instructors are aligned in their learning objectives, fostering a more personalized and effective educational experience.
By leveraging AI technology, educators can access a wealth of data that outlines each student's strengths and weaknesses. This information can be used to create targeted interventions, improve lesson planning, and ultimately enhance learning outcomes. Moreover, real-time tracking can help educators identify potential issues early on, which is crucial for preventing students from falling behind.
Key Benefits for Educators
- Instant Feedback: Allows educators to receive immediate data on student performance, enabling timely interventions and adjustments to the curriculum.
- Personalized Learning: Helps in tailoring the learning experience to each student's unique needs, ensuring that they receive the right support at the right time.
- Data-Driven Decisions: Provides educators with accurate and actionable insights that guide instructional decisions, improving overall teaching effectiveness.
- Identification of At-Risk Students: Facilitates early detection of students who may need additional help, reducing the likelihood of them falling behind.
Practical Applications
- Teachers can monitor student engagement levels and adjust pacing to maintain interest.
- Real-time tracking can help in identifying patterns of difficulty, allowing for quick remedial actions.
- AI-driven analytics offer insights into which teaching methods are most effective for different students.
"Real-time performance monitoring empowers educators to create more adaptive and responsive learning environments, ultimately improving student success."
Example of Data Utilization
Metric | Action |
---|---|
Low Engagement | Adjust lesson pacing, introduce interactive activities |
Frequent Mistakes in Specific Topics | Provide additional resources and focused review sessions |
How AI Tutors Adjust to Student Progress and Challenges
Artificial intelligence-driven tutoring systems are designed to track the learner's performance over time and respond accordingly. These systems utilize advanced algorithms that assess not only correct answers but also the types of mistakes made, enabling them to adapt instruction to better meet individual needs. By continuously analyzing responses, the system identifies areas of struggle, providing focused support and offering tailored exercises for improvement.
Through constant evaluation, AI tutors create a personalized learning experience. The system monitors progress through real-time feedback, adjusting the pace and difficulty of tasks. When students demonstrate mastery of a topic, the AI shifts to more challenging materials, while when difficulties arise, it revisits foundational concepts and reinforces understanding in targeted areas.
Key Adaptation Methods
- Dynamic Learning Paths: AI tutors adjust learning routes based on a student's progress. They may skip over already mastered concepts or revisit topics that need more attention.
- Real-Time Feedback: Immediate responses to both correct and incorrect answers help students learn from mistakes and enhance their problem-solving skills.
- Customized Content: Based on the ongoing assessment, the tutor can introduce personalized exercises, allowing students to work on specific weaknesses while reinforcing strengths.
Process of Identifying Areas for Improvement
- Data Collection: The system gathers data on each interaction, including the time taken to complete tasks and error patterns.
- Pattern Recognition: AI identifies patterns in errors, categorizing them into areas that need more focus, such as concepts, problem-solving methods, or time management.
- Focused Exercises: Once challenges are identified, the system creates focused exercises targeting the specific gaps in knowledge.
AI-driven tutoring systems are not static. They continuously evolve based on the student’s unique learning curve, ensuring an individualized experience that fosters success.
Example of AI Tutoring Adjustments
Student Progress | AI Tutor Response |
---|---|
Mastery of basic arithmetic | Introduction of more advanced mathematical problems |
Frequent errors in algebraic equations | Repetition of foundational algebra concepts with hints and step-by-step guides |
Correct answers in geometry, slow response time | Increase in speed-focused practice with timed exercises |
Ensuring Data Privacy and Security in AI Tutoring Platforms
In the era of AI-driven tutoring systems, the protection of personal data is critical. These platforms collect vast amounts of sensitive information, such as academic performance, learning patterns, and even personal details. With this in mind, ensuring robust data privacy and security mechanisms is paramount to maintaining user trust and compliance with regulations like GDPR or HIPAA.
AI tutoring systems must integrate sophisticated measures to safeguard against data breaches and unauthorized access. This includes encrypting all stored and transmitted data, implementing multi-factor authentication (MFA), and adhering to best practices in data anonymization. Additionally, constant monitoring for potential vulnerabilities should be a standard procedure to address emerging threats proactively.
Key Security Measures in AI Tutoring Systems
- Data Encryption: All sensitive data should be encrypted both at rest and during transmission to prevent unauthorized access.
- Access Control: Implement role-based access control (RBAC) to ensure that only authorized personnel or systems have access to sensitive information.
- Continuous Monitoring: Regular audits and real-time monitoring of the platform help to identify and address potential security risks before they escalate.
To further enhance privacy, AI systems must also provide transparency about how data is collected, stored, and used. This includes clear user agreements, regular updates on data handling practices, and the option for users to control or delete their data as needed.
"Transparency and user consent are not just ethical practices; they are legal requirements in many jurisdictions, particularly in the context of AI and data privacy."
Privacy and Security Best Practices
- Regular Software Updates: Keeping the platform's software up to date is essential to mitigate vulnerabilities found in older versions.
- User Education: Users should be informed about the importance of maintaining their own account security through strong passwords and awareness of phishing attempts.
- Compliance with Standards: AI platforms must comply with relevant standards and regulations to protect user data effectively.
Security Measure | Description |
---|---|
Data Encryption | Ensures that all data, both at rest and in transit, is encrypted to prevent unauthorized access. |
Role-based Access Control | Restricts access to sensitive data based on the user’s role within the system. |
Two-factor Authentication | Provides an additional layer of security by requiring two forms of identification to access the system. |